News and Agenda Archive
News
ICLR award for Cristian Meo (SPS)
- Monday, 5 May 2025
The paper "Masked Generative Priors Improve World Models Sequence Modelling Capabilities" -https://lnkd.in/d6MbVTEa, was awarded the Outstanding Paper Award at the World Models: Understanding, Modelling & Scaling Workshop at ICLR 2025. The first author is Cristian Meo, a PhD student of Justin Dauwels.
ICLR is one of the top-3 machine learning conferences.
(The compute resources needed to generate the results in this paper were provided by a grant for the Snellius national supercomputer, amounting to over 50 kE.)
More ...
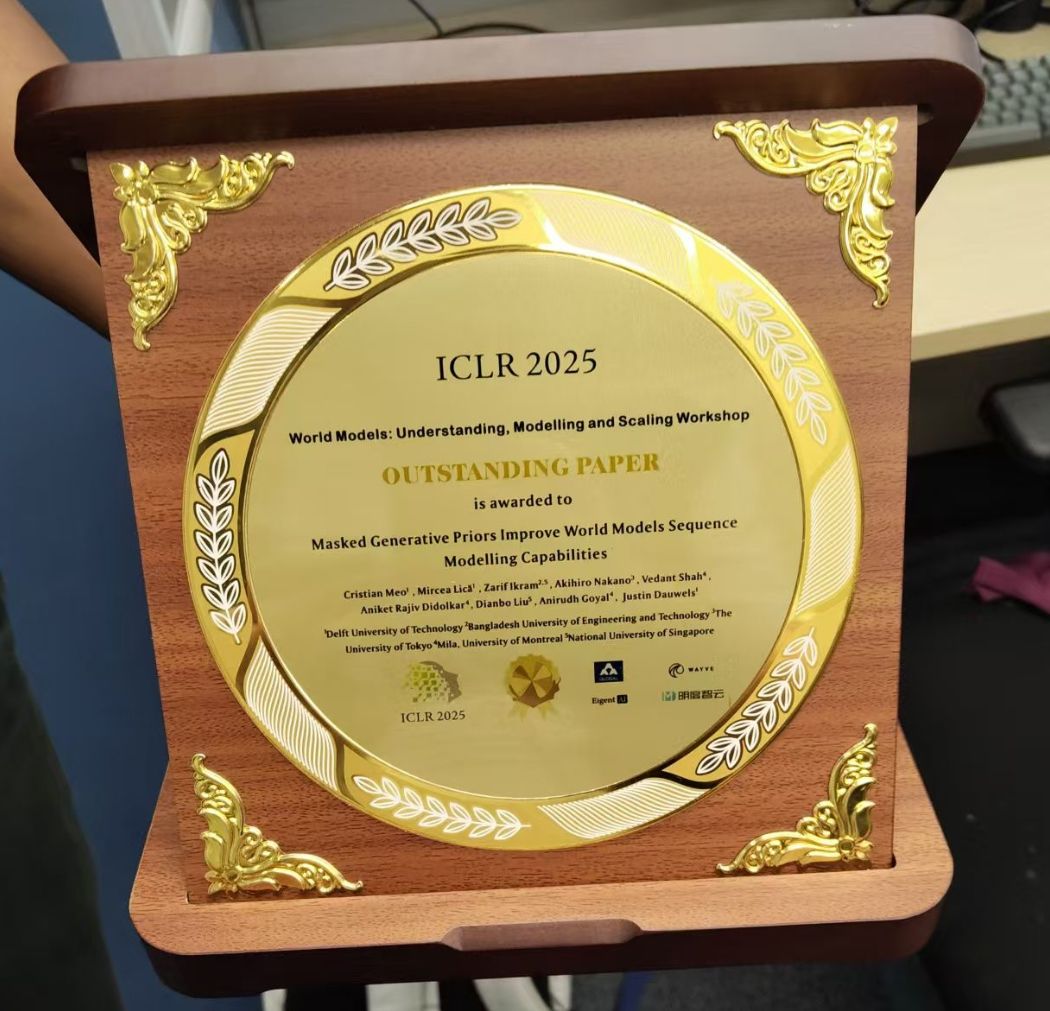
Where is everyone?
- Friday, 11 April 2025
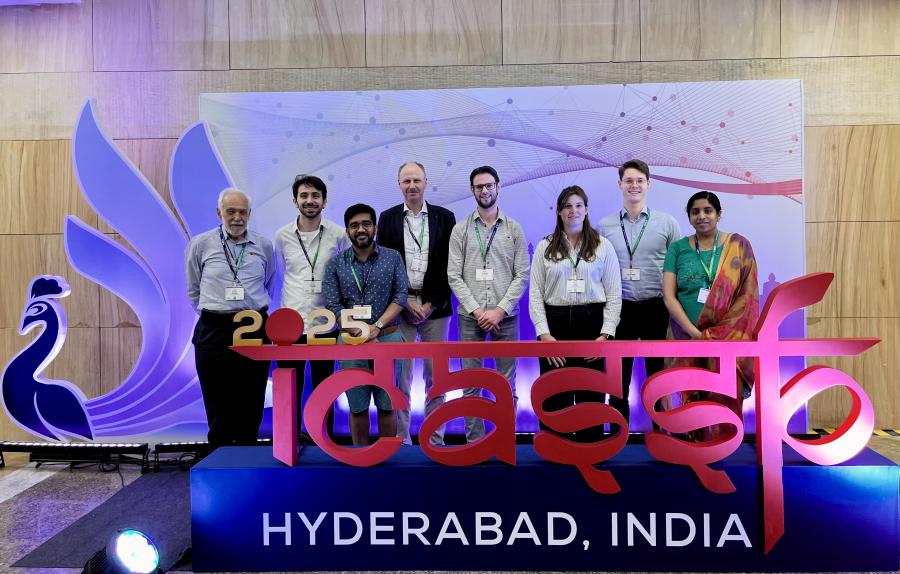
Greetings from ICASSP 25 Hyderabad! Where Geert collected his TSP best paper award. Special guest: Patrick Dewilde (former head of the section until 2006).
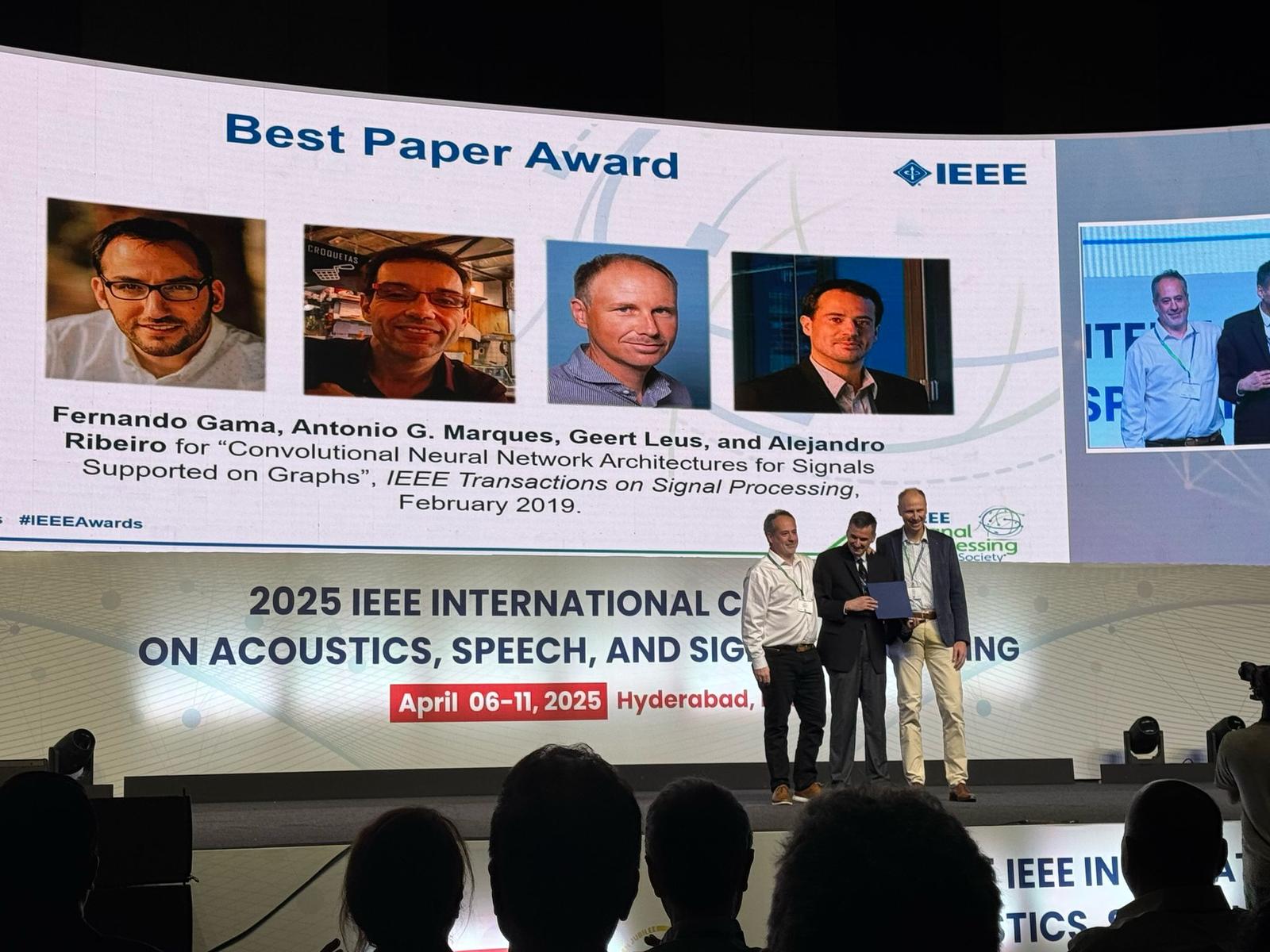
Bishwadeep Das receives ICASSP 2025 Best Paper Award
- Friday, 11 April 2025
At ICASSP 2025 in Hyderabad, SPS Postdoc Bishwadeep Das received the Best Paper Award. For this conference, more than 7000 submissions were received, so it's quite an accomplishment. Congratulations!
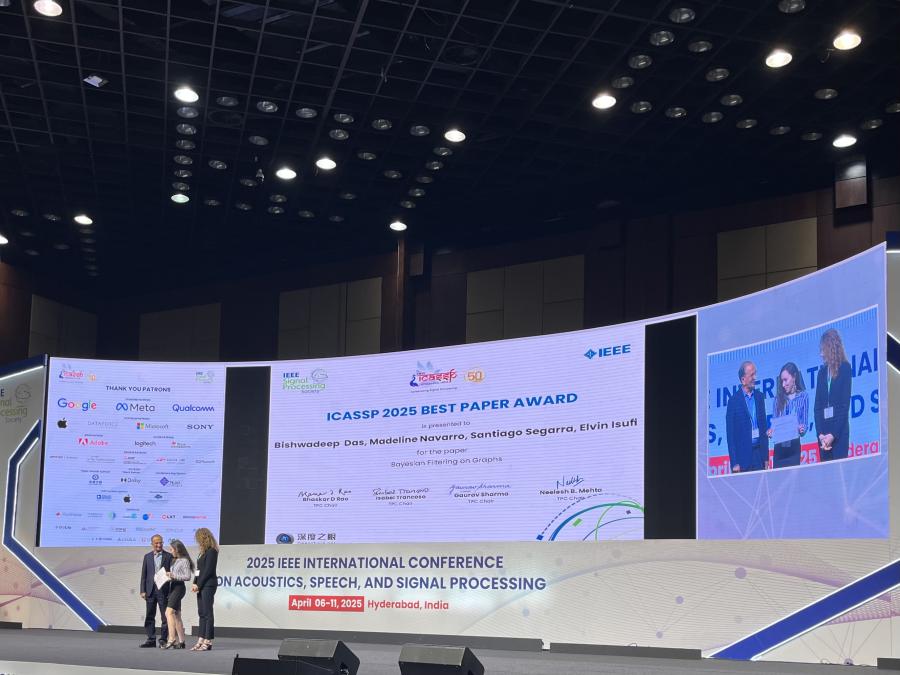
Geert Leus receives IEEE TSP Best Paper Award
- Friday, 11 April 2025
In December 2024, it was announced that a paper coauthored by Geert has been selected for the 2024 IEEE SPS Best Paper Award for the below noted paper.
Fernando Gama, Antonio G. Marques, Geert Leus, and Alejandro Ribeiro
"Convolutional Neural Network Architectures for Signals Supported on Graphs"
IEEE Transactions on Signal Processing, VOL. 67, NO. 4, FEBRUARY, 15 2019
https://ieeexplore.ieee.org/document/8579589
The award was handed to him during the award ceremony at ICASSP'25 Hyderabad.
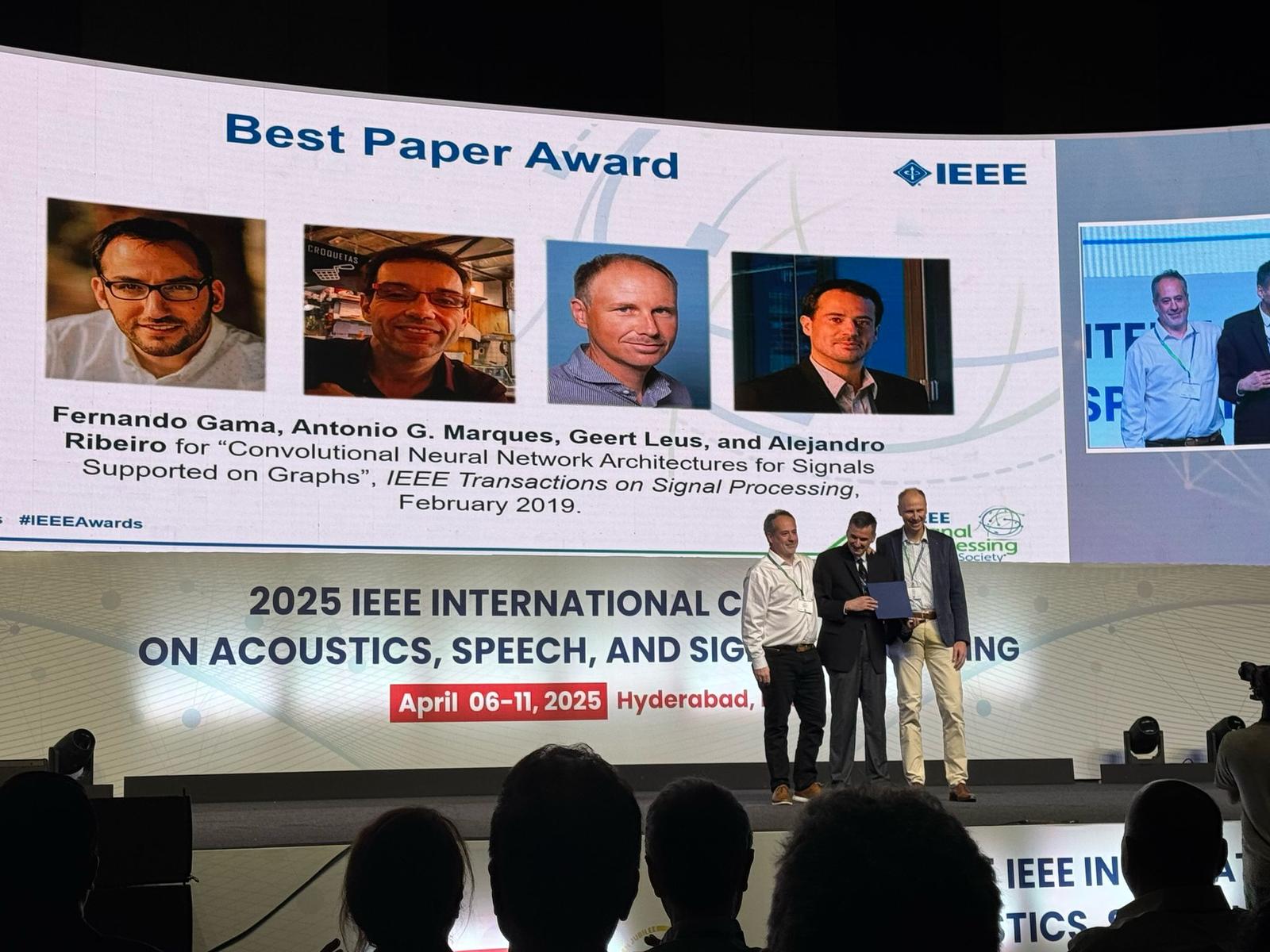
The Model-Driven Decisions Lab (MoDDL) officially opened
- Wednesday, 12 February 2025
Director: Justin Dauwels
More ...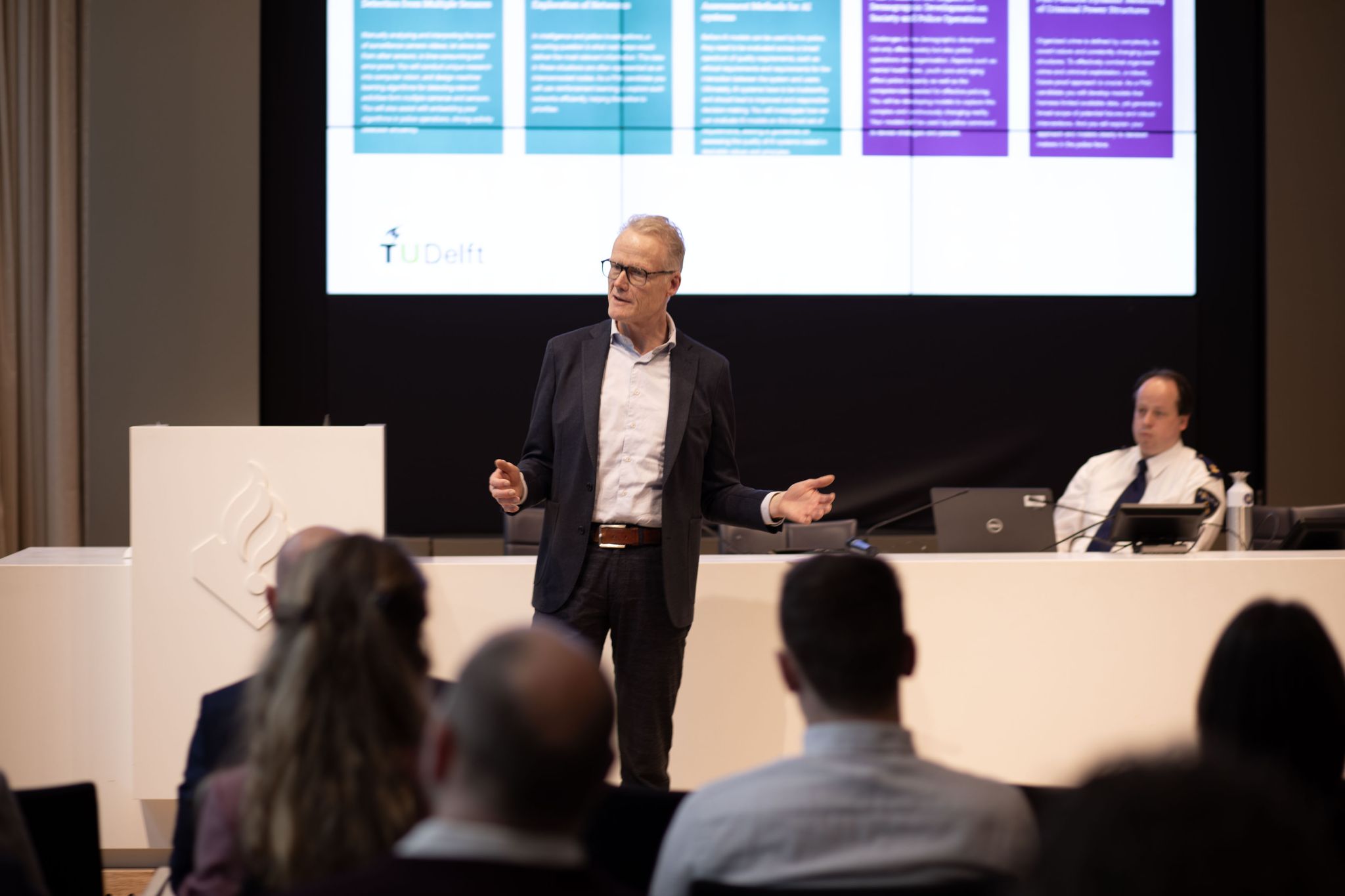
Geert Leus (SPS group) won the 2024 IEEE SPS best Paper award
- Tuesday, 17 December 2024
A paper coauthored by Geert has been selected for the 2024 IEEE SPS Best Paper Award for the below noted paper.
Fernando Gama, Antonio G. Marques, Geert Leus, and Alejandro Ribeiro
"Convolutional Neural Network Architectures for Signals Supported on Graphs"
IEEE Transactions on Signal Processing, VOL. 67, NO. 4, FEBRUARY, 15 2019
https://ieeexplore.ieee.org/document/8579589
The award will be handed during the award ceremony at ICASSP'25 Hyderabad.
More ...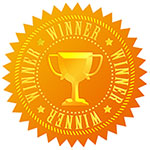
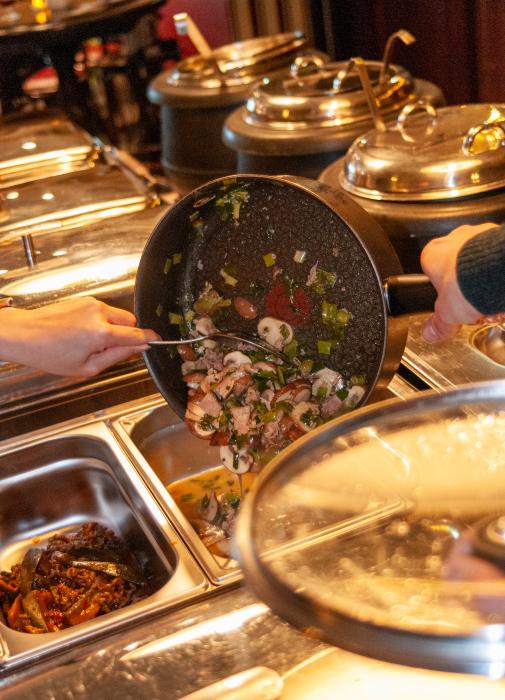
Congratulations Aybuke
- Monday, 9 December 2024
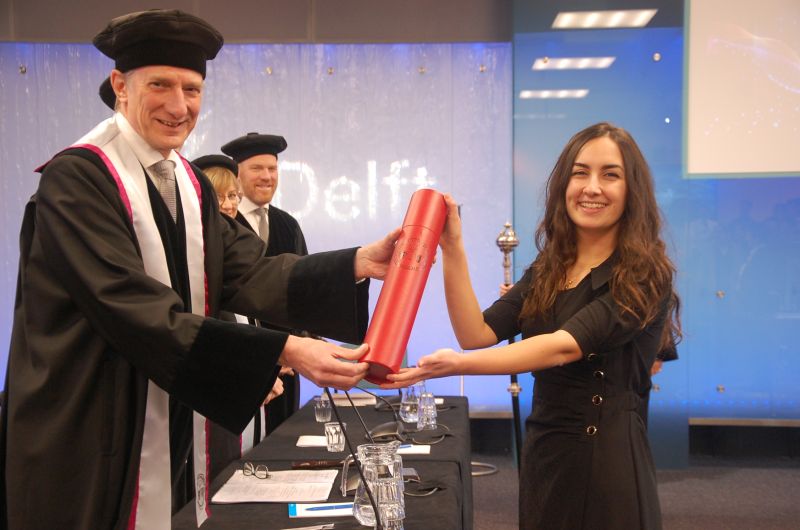
On 3 December 2024, Aybuke Erol successfully defended her PhD thesis on "Modeling spatio-temporal variability of brain responses in functional ultrasound", and thereby earned her PhD diploma. Congratulations!
Also congratulations to Bori Hunyadi for delivering her second PhD student at TU Delft, both in 2024. More to come!
More ...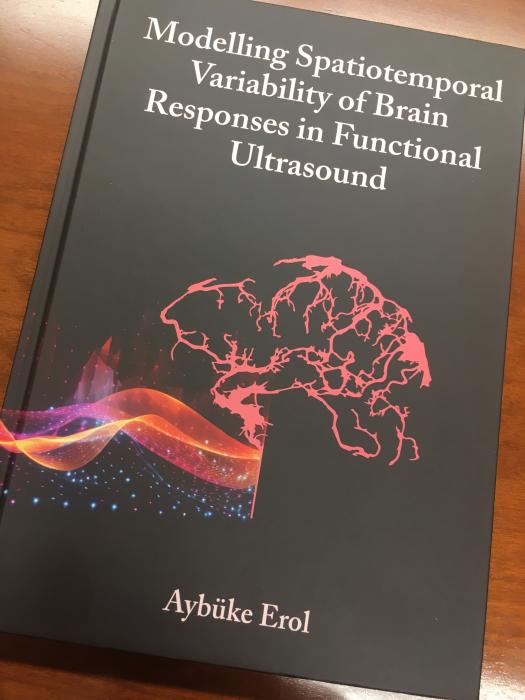
New book by Alle-Jan van der Veen
- Monday, 9 December 2024
Time-Variant and Quasi-separable Systems
Patrick Dewilde, Klaus Diepold, and Alle-Jan van der Veen;
Cambridge University Press, DOI: https://doi.org/10.1017/9781009455640
November 2024.
This book evolved from the PhD thesis of Alle-Jan van der Veen (1993), where Patrick Dewilde was promotor; a first edition of this book (1998) was used for teaching at TU Munich by Klaus Diepold. Over the years, material was added, while content was also adjusted to fit an MSc (advanced-level) course.
The result is this unique book, which links matrix theory to that of linear time-varying systems. E.g., matrix inversion, LU factorization, QR factorization, and even SVD are connected to state-space representations of time-varying systems, and the calculations to obtain these factorizations are also carried out in state-space. This results in both familiar recursions to compute matrix factorizations/inversions, but also in completely new ones.
More ...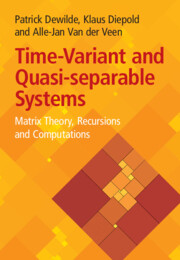
Chenyang Yan (MSc student SPS) receives Best Student Paper Award
- Wednesday, 6 November 2024

For his MSc thesis, Chenyang Yan worked with Microflown on a new type of cover for their acoustic vector sensor. This cover allows for detecting more sources with a better performance. For this work Chenyang was awarded the Best Student Paper Award at the 2024 Asilomar Conference on Signals, Systems, and Computers.
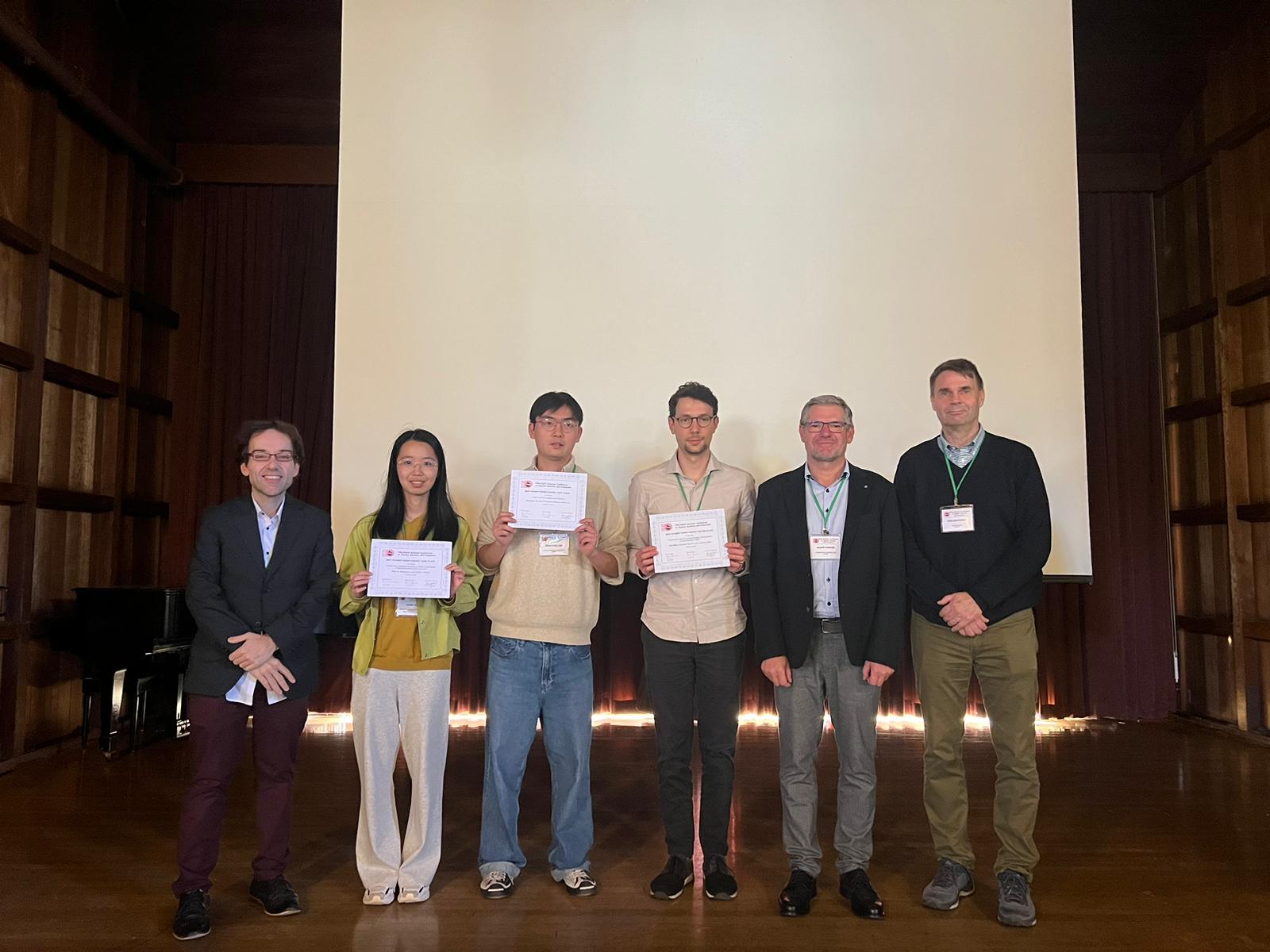
Bori Hunyadi wins Vidi award
- Wednesday, 23 October 2024
NWO has awarded 102 researchers a Vidi grant. Among the eight promising TU Delft researchers is Bori Hunyadi (SPS).
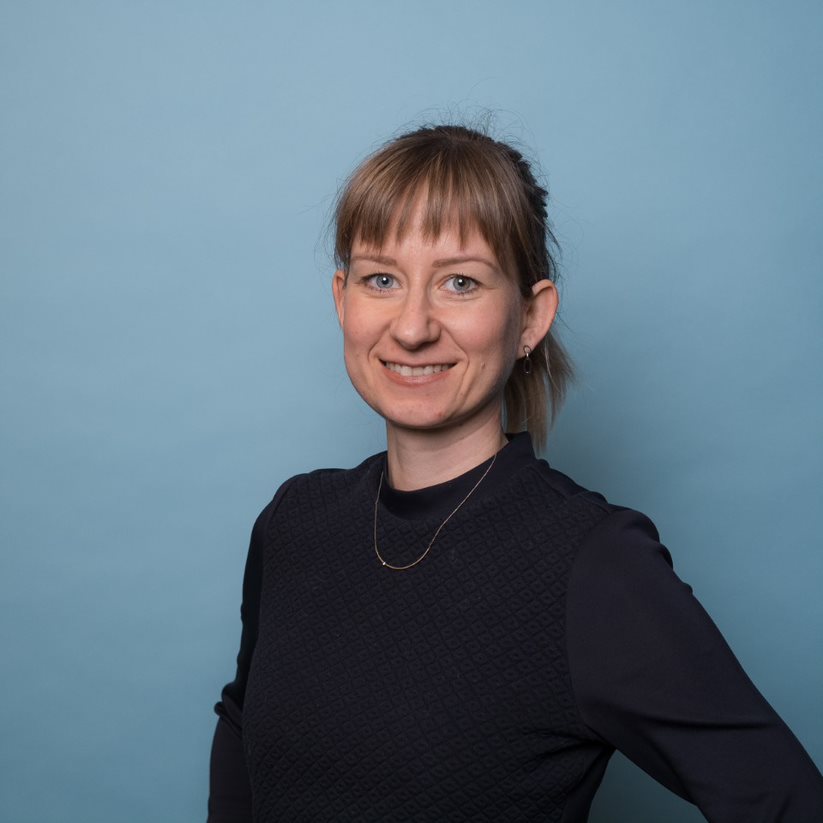
'Best Innovation Booth' award at the international Astronautical Congres
- Tuesday, 22 October 2024
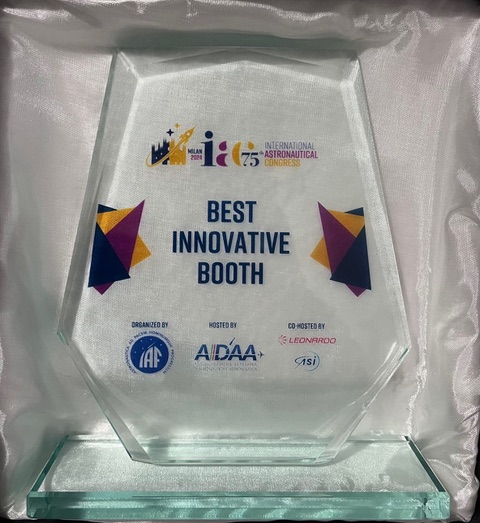
The TU Delft team of Chris Verhoeven and Raj Rajan attended the International Astronautical Congress (2024) https://www.iac2024.org/ , and won the “Best Innovation Booth” award demonstrating the “Autonomous path planning of Lunar Zebro”, and further presented the Moonshot project. Congratulations!

Geert Leus has been awarded the Dr. Ramkumar IISc Distinguished Visiting Chair Professorship
- Tuesday, 17 September 2024
Prof.dr.ir. Geert Leus has been awarded the Dr. Ramkumar IISc Distinguished Visiting Chair Professor position in the Department of Electrical Communication Engineering of the Indian Institute of Science (IISc), Bengaluru, India
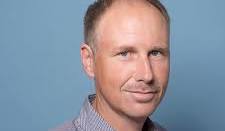
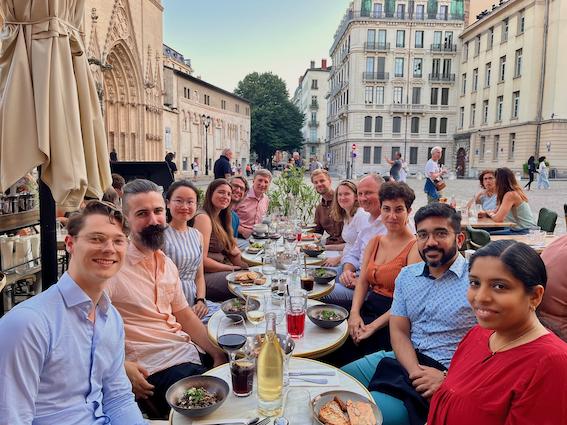
Alle-Jan van der Veen wins EURASIP Technical Achievement Award
- Thursday, 29 August 2024
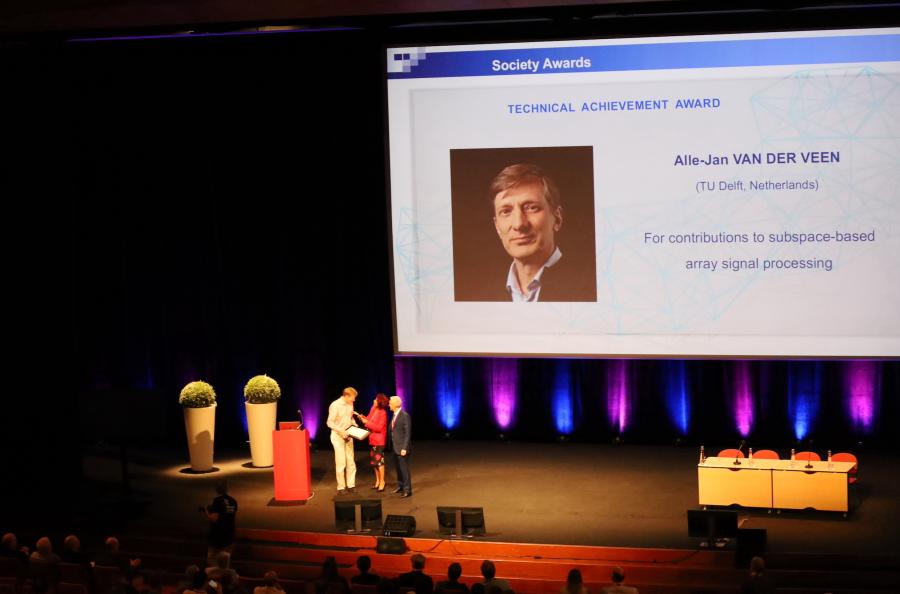
Alle-Jan has been awarded the prestigious Technical Achievement Award of EURASIP for his “contributions to subspace-based array signal processing”. EURASIP is the European Association for Signal Processing. The award was presented at EUSIPCO'2024 in August in Lyon.
The EURASIP Technical Achievement Award honors a person who, over a period of years, has made outstanding technical contributions to theory or practice in technical areas within the scope of the Society, as demonstrated by publications, patents, or recognized impact in this field.
More ...

Congratulations Hanie!
- Friday, 21 June 2024
On 18 June 2024, Hanie Moghaddasi successfully defended her thesis "Model-based feature engineering of atrial fibrillation". Congratulations!
More ...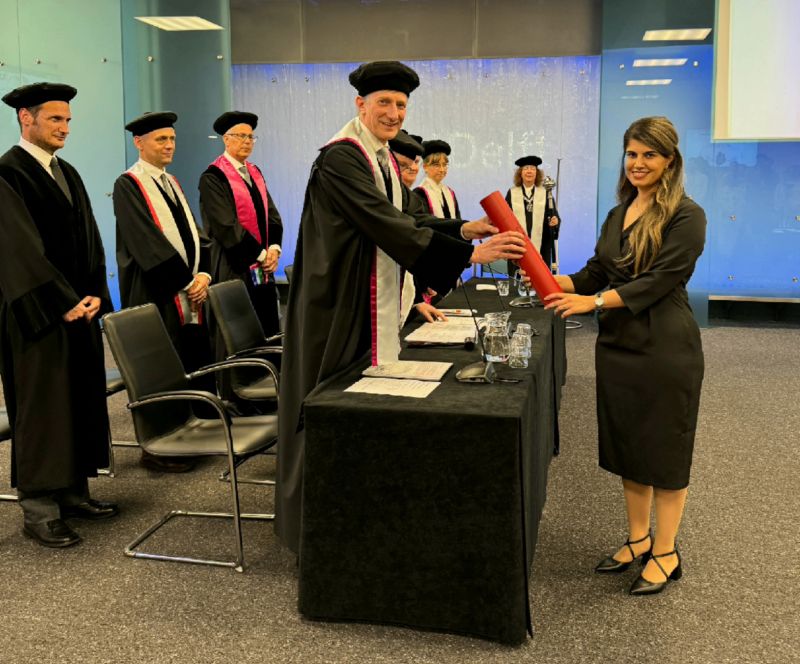
Swarming lab opened!
- Friday, 21 June 2024
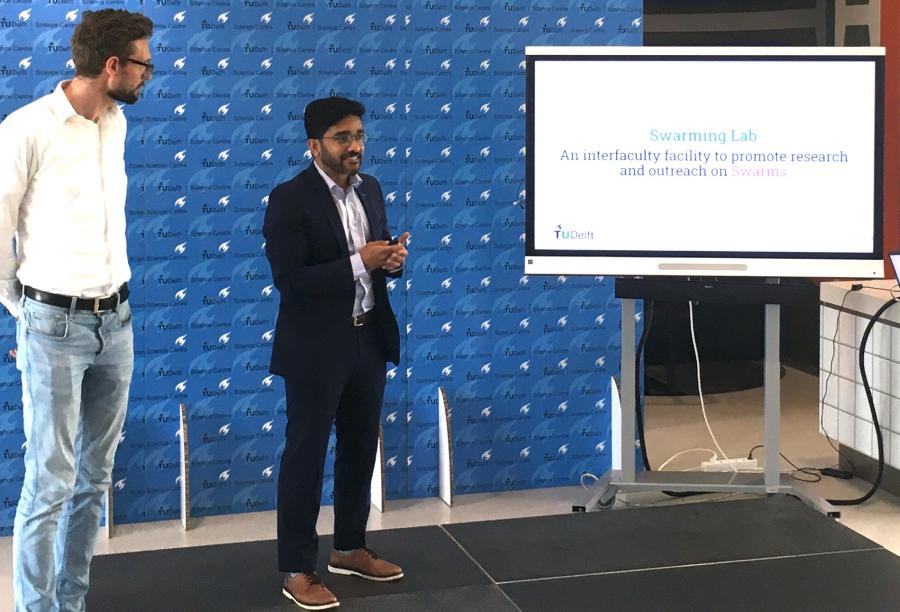
The TU Delft Swarming Lab is an interfaculty facility between Delft Robotics Institute (DRI), Faculty of EEMCS, Faculty of AE and MAVLab, established to foster research collaboration and community building on swarms.
Although the lab has been operational since 2023, it was formally opened on 20 June 2024 by Guido de Croon and Raj Rajan (co-directors). It is housed in the TU Delft Science Centre, which also gives it large visibility to the general public.
The opening presented the facilities as well as some of the amazing students and researchers who have contributed to the lab thus far.
More ...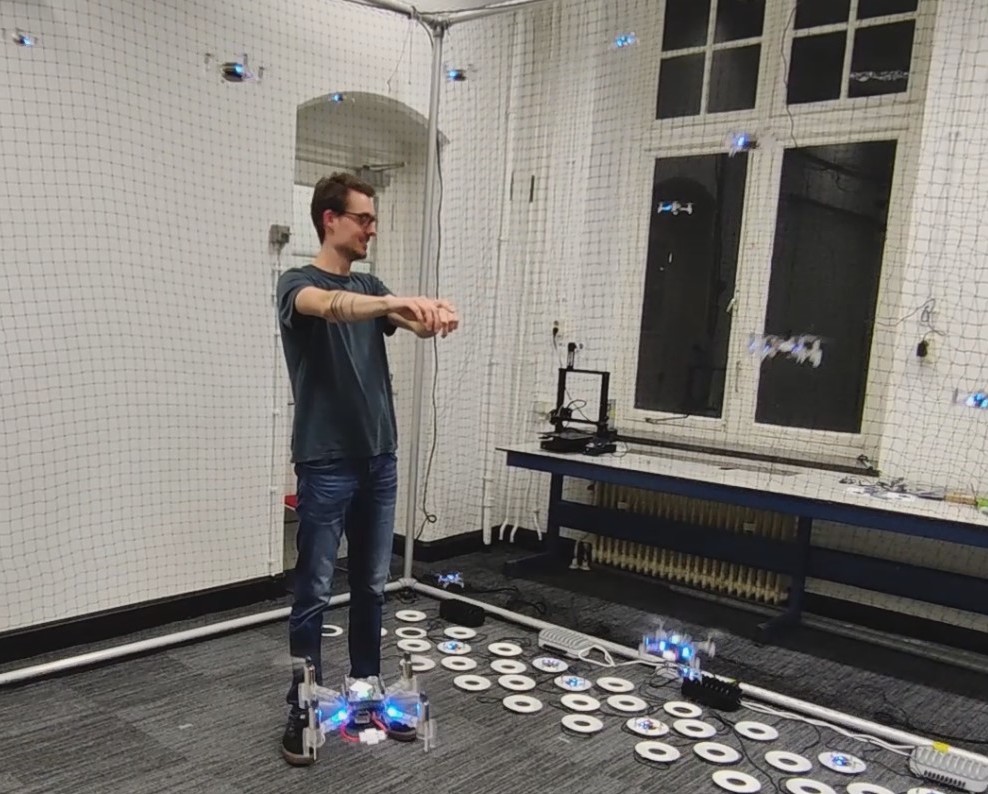
Justin Dauwels appointed (co)director of TU Delft Safety & Security Institute
- Monday, 27 May 2024
Justin Dauwels is appointed as codirector of the TU Delft Safety & Security Institute, together with Eleonora Papadimitriou.
One of the first activities was the signing of a framework agreement with the Dutch police to work on safety & security issues, which led to the establishment of a joint Model-Driven Decisions Lab (MoDDL). Several PhD projects will be funded in the context of this Lab.
More ...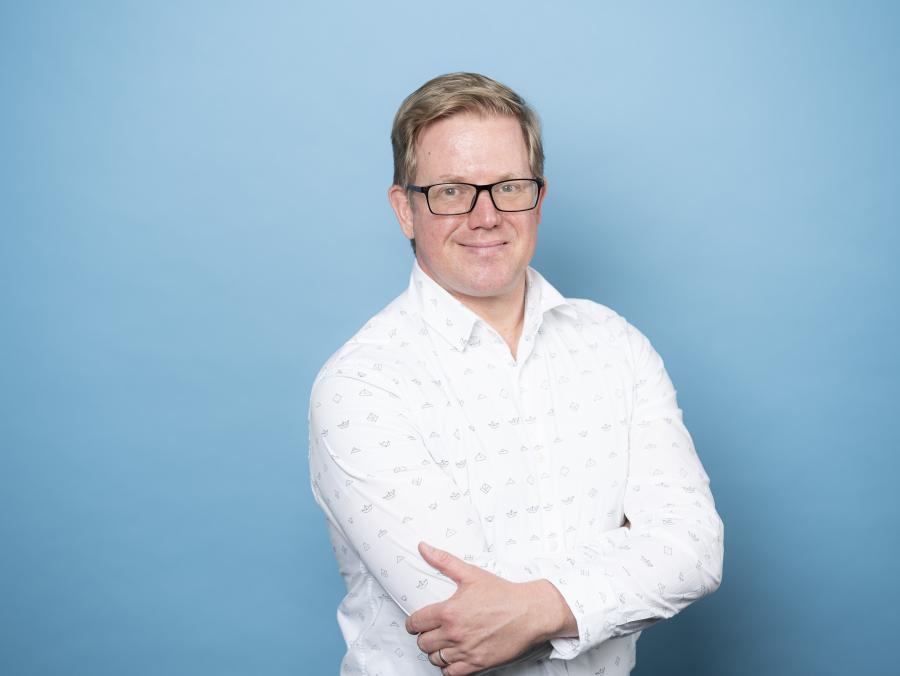
Greetings from ICASSP'24 Seoul
- Monday, 22 April 2024
A small but high-level delegation of the SPS group went to ICASSP'24 Seoul this year, along with over 4400 participants! It's been hard to find each other in this crowd, but still we managed to make this "group" picture.
More ...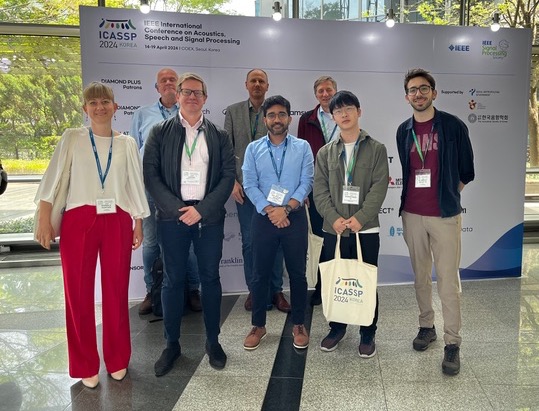
STOI incorporated in Matlab
- Friday, 22 March 2024
STOI is a widely used measure for "short-time objective intelligibility" in (compressed) speech. It was developed by Cees Taal, Richard Hendriks (SPS), Richard Heusdens, and Jesper Jensen in 2010.
It allows to assess the performance of speech compression algorithms, without using listening panels. It is currently popular as a target feature in machine learning algorithms.
STOI already won best paper awards, and has now been incorporated in the 2024 edition of Matlab.
More ...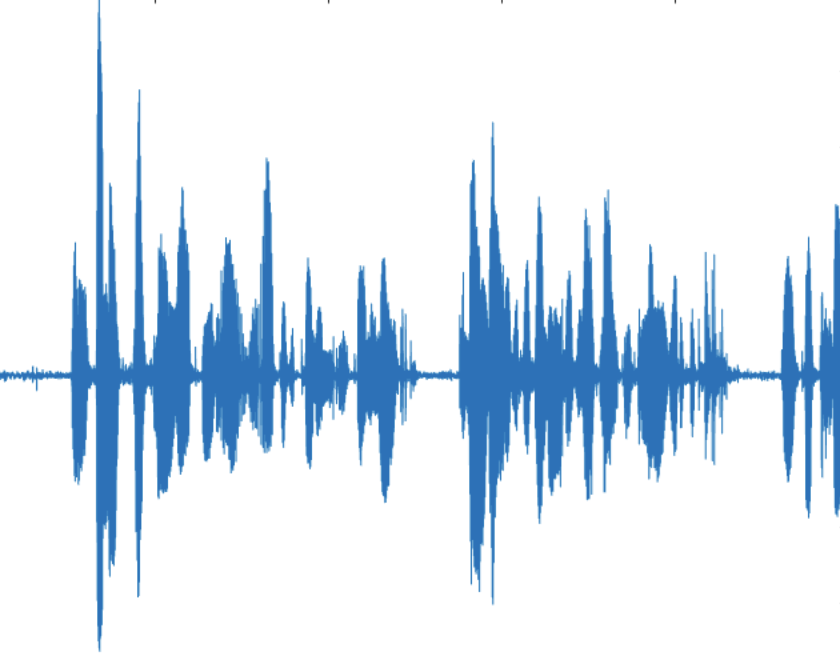
Startup Innatera in the news
- Thursday, 7 March 2024
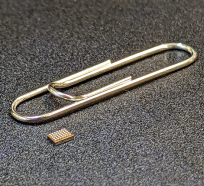
Innatera is a startup that originated in 2019 in the SPS group and has now grown to 65 employees. Recently they completed a neural network-based microcontroller, based on spiking neural networks. This enables highly power efficient processing (e.g. real-time image recognition) for Internet-of-Things applications.
The chip was demonstrated at the Consumer Electronics Show (CES) in Las Vegas.
More ...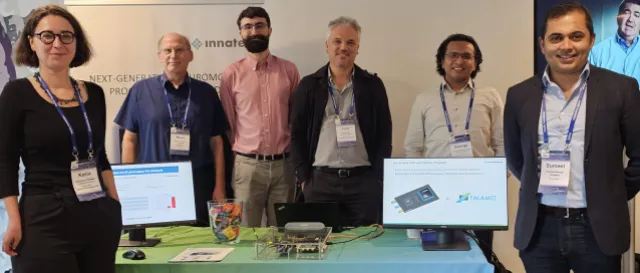
Alle-Jan van der Veen wins EURASIP Technical Achievement Award
- Friday, 1 March 2024
Alle-Jan has been awarded the prestigious Technical Achievement Award of EURASIP for his “contributions to subspace-based array signal processing”.
The EURASIP Technical Achievement Award honors a person who, over a period of years, has made outstanding technical contributions to theory or practice in technical areas within the scope of the Society, as demonstrated by publications, patents, or recognized impact in this field.
EURASIP is the European Association for Signal Processing. The award will be presented at EUSIPCO'2024 in August in Lyon.
More ...
Portrait of Medical Delta Professor Johan Frijns (appointed at EEMCS, Bioelectronics group)
- Friday, 16 February 2024
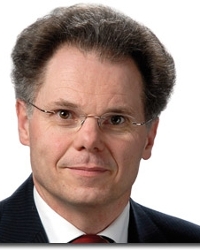
Portrait of Medical Delta Professor Johan Frijns
More than 800,000 people in the Netherlands are hard of hearing. They suffer so much from hearing loss that it limits their daily lives. Prof. Dr. ir. Johan Frijns treats people with hearing loss, conducts research on hearing implants, and gladly shares his knowledge about electrical stimulation of the nervous system. "We shouldn't want to reinvent everything in every little corner. What we learn in one place, we can also use in another."
Johan Frijns is a professor of Otology and Physics of Hearing in the Department of Otorhinolaryngology at LUMC. He heads the Center for Audiology and Hearing Implants Leiden (CAHIL) and the Cochlear Implant Rehabilitation Centre Leiden (CIRCLE). He was recently appointed as a Medical Delta professor with a position at the Faculty of Electrical Engineering, Mathematics, and Computer Science at TU Delft.
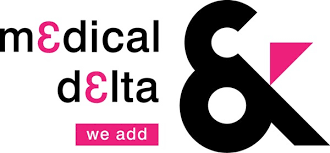
New book with contribution of Geert Leus
- Wednesday, 24 January 2024
Sparse Arrays for Radar, Sonar, and Communications
More ...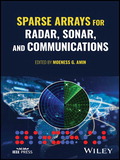
Science Advances paper co-authored by Geert Leus
- Friday, 19 January 2024
"Four-dimensional computational ultrasound imaging of brain hemodynamics" by Michael D. Brown et al. appeared in Science Advances on 17 January, 2024.
Read the paper at the following link.
More ...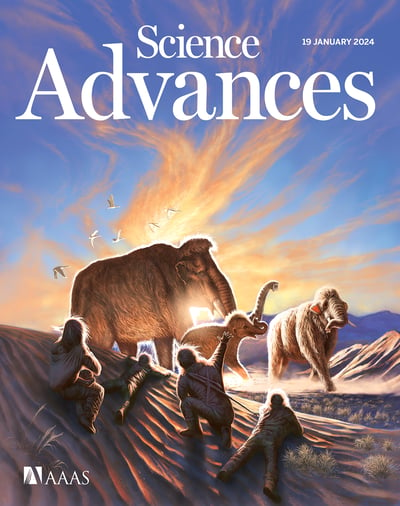
Mario Coutino wins best PhD thesis award
- Tuesday, 12 December 2023
Mario Coutino has won the IEEE Signal Processing Society Best PhD Dissertation Award. Mario received his PhD in Apr 2021 with a dissertation titled "Advances in graph signal processing: Graph filtering and network identification" (promotor: Geert Leus (SPS)).
Congratulations!
More ...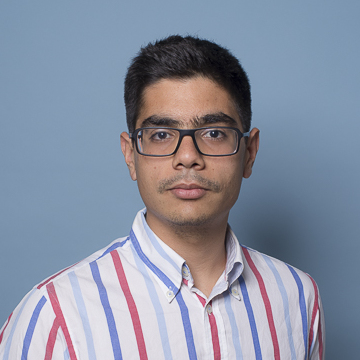
Justin Dauwels named IEEE SPS Distinguished Lecturer for 2024
- Tuesday, 28 November 2023
Justin Dauwels has been named IEEE SPS Distinguished Lecturer for 2024. The Distinguished Lecturer Program provides means for IEEE chapters to have access to individuals who are well known educators and authors in the fields of signal processing. The selection is quite rigorous and only 5 persons are appointed as DL each year.
Lecture topics for Justin are:
- Deep Generative AI
- Machine Learning for Applications in Neurology
- Machine Learning for Applications in Psychiatry
- Perception Error Modelling for Autonomous Driving
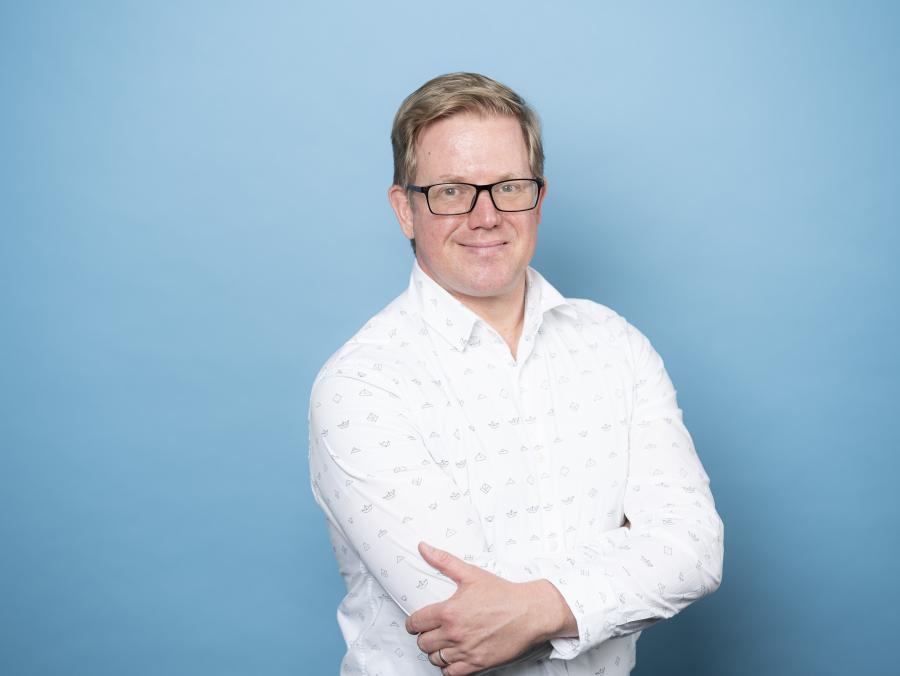
New NWO-TTW project for Geethu and Justin (SPS)
- Monday, 27 November 2023
Laser satellite communications is a promising technology to support worldwide access to telecommunications services. A major technological challenge is atmospheric turbulence impacting the propagation of the laser beams. Its effect can be mitigated by adaptive optics and geographic diversity. The DAILSCOM project aims to provide a map of the effective optical channel performance over Europe. This map is needed to design ground network technology and estimate communications service availability. Since our current understanding and ability to estimate the channel performance are limited, we will develop novel physics-informed machine learning algorithms to formulate the optical link performance map.
Project PIs are: Rudolf Saathof (AE), Justin Dauwels, Geethu Joseph, Sukanta Basu (CiTG)
More ...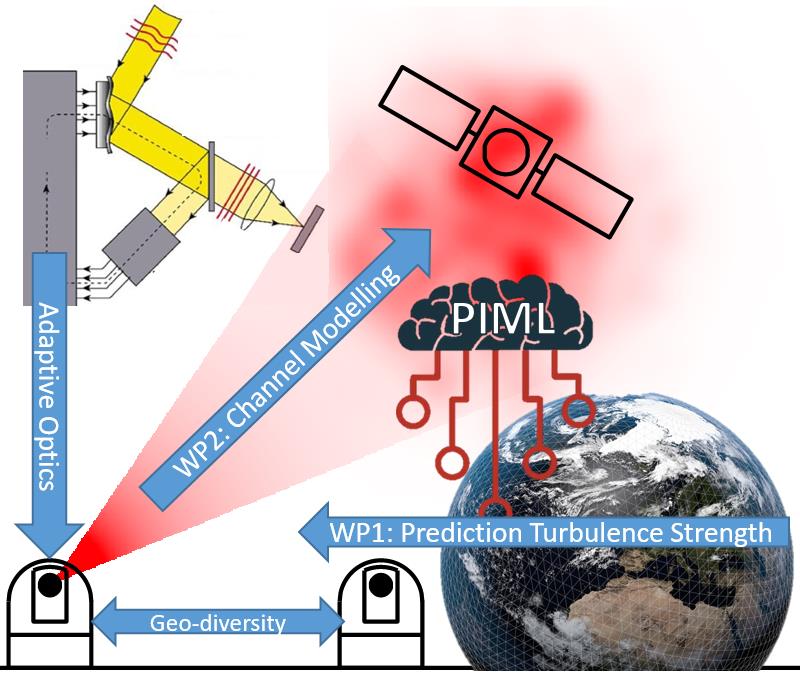
Justin Dauwels IEEE Signal Processing Magazine Area Editor
- Monday, 27 November 2023
Justin Dauwels has been appointed Columns & Forums Area Editor for IEEE Signal Processing Magazine. Congratuations!
More ...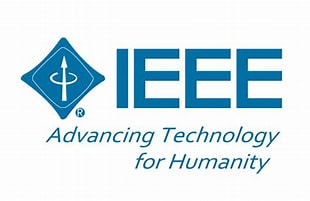
Richard Hendriks Senior Associate Editor
- Friday, 10 November 2023
Richard Hendriks has been appointed as Senior Associate Editor of IEEE Transactions on Audio Speech and Language Processing. Congratulations!
More ...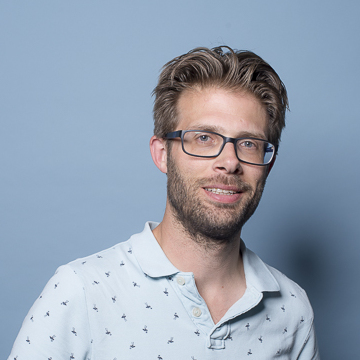
Model-Driven Decisions Lab initiated
- Friday, 29 September 2023
On 28 September, a Memorandum of Understanding was signed between the TU Delft and the national police, to collaborate on research around safety and security, and forensic research. Part of this initiative will be a new research lab, the "Model-Driven Decisions Lab". This lab will start up with 5 PhD positions. Scientific director is Justin Dauwels (SPS).
More ...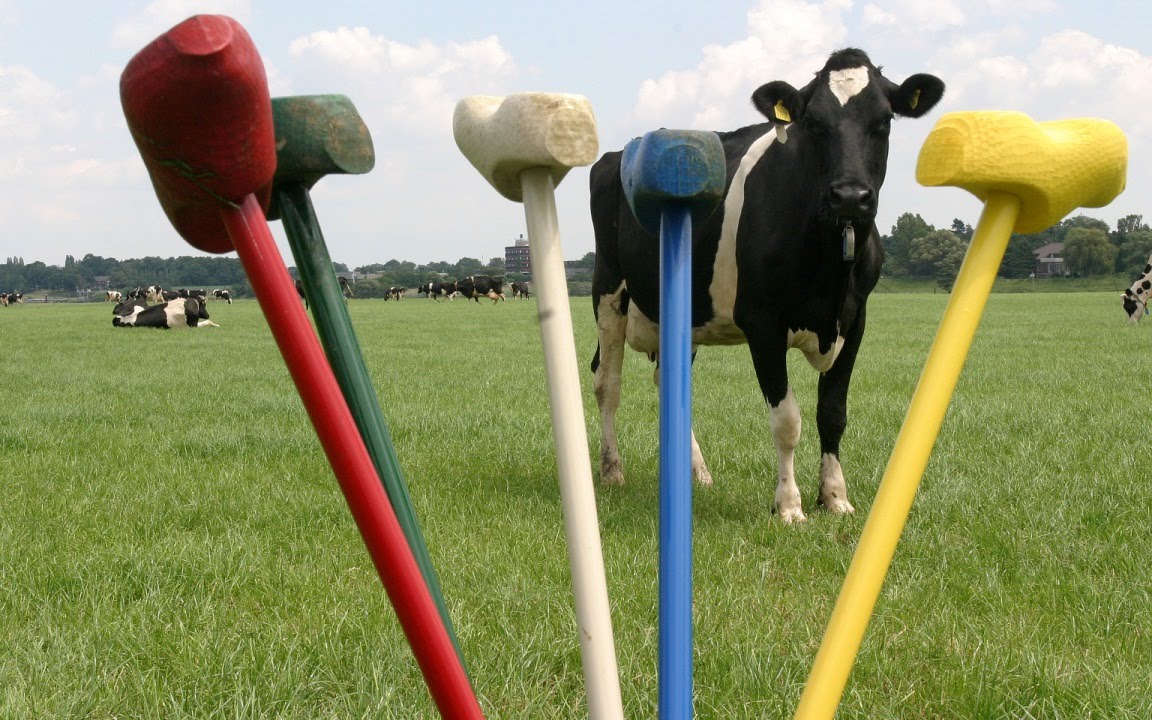
Best paper award for Costas Kokke
- Wednesday, 14 June 2023
Costas Kokke received the best contribution award at ISCS, the International Symposium on Computational Sensing. Congratulations!
More ...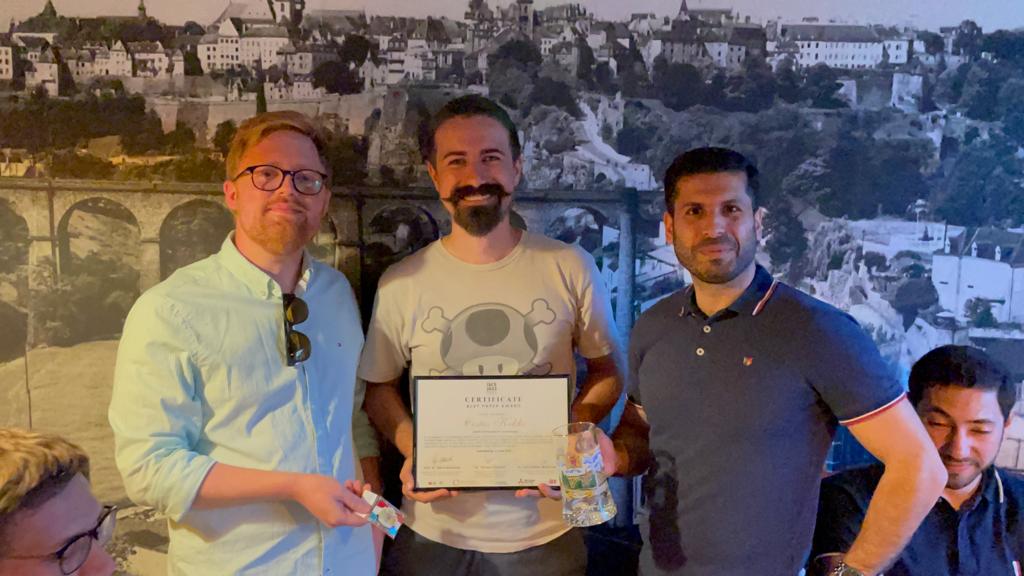
IEEE SPS Best Thesis Award for Geethu Joseph
- Wednesday, 14 June 2023
Geethu Joseph received the IEEE Signal Processing Society Best PhD Thesis Award at ICASSP'23. Annually, there are 2 such awards. The 2nd award went to Elvin Isufi, former PhD student of Geert Leus. Congratulations to both!
These awards were installed 3 years ago; until now, there have been 3 recipients.
More ...
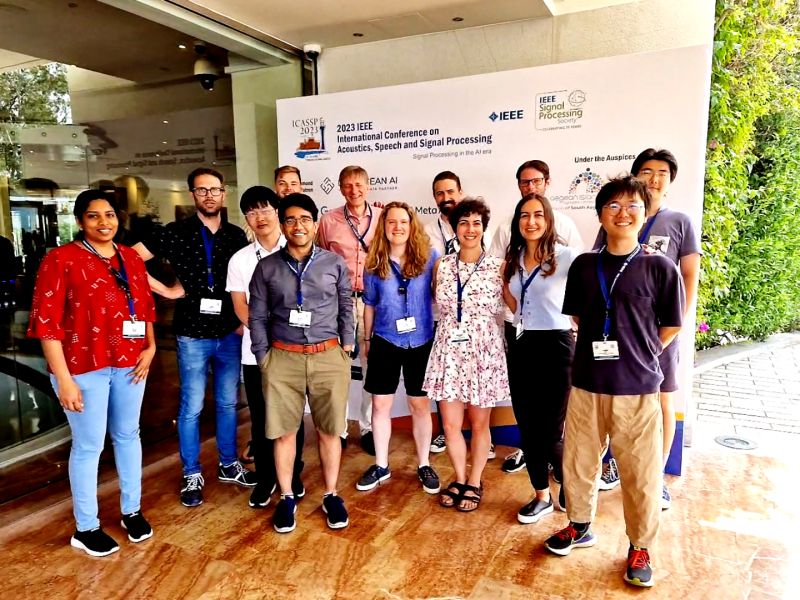
Best paper award for Sofia Kotti!
- Monday, 12 June 2023
We are proud of our PhD student Sofia-Eirini Kotti who won the best paper award for her work “Modeling nonlinear evoked hemodynamic responses in functional ultrasound” at the Data Science and Learning Workshop during #icassp2023. Special thanks to Aybüke Erol for her contributions to this research!
More ...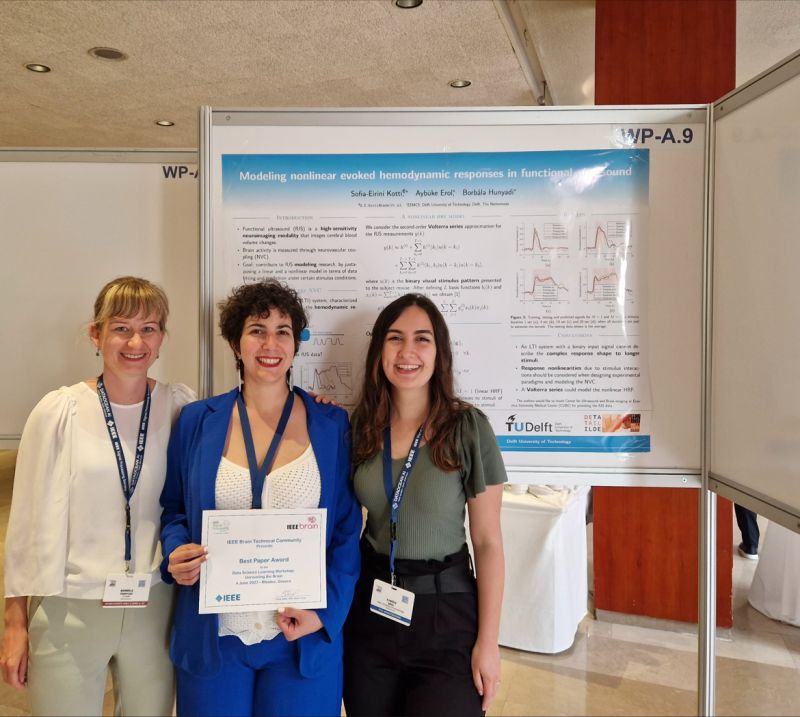
Best Paper Award at the SITB'23 symposium
- Monday, 15 May 2023
At the 43rd Symposium on Information Theory and Signal Processing in the Benelux, our MSc student Alan Hamo has won the award for Best Student Presentation, for the paper "Machine learning algorithm to predict cardiac output based on arterial pressure measurement". Congratulations!
Paper abstract
Cardiac output (CO) plays a crucial role in determining the delivery of oxygen to tissues and is a key metric in hemodynamic optimization. The gold standard method for measuring cardiac output is through thermodilution using pulmonary artery catheter, but it is an invasive procedure associated with complications during placement and the need for a skilled expert to perform the measurements. An alternative approach is to estimate cardiac output by utilizing arterial blood pressure (ABP) measurements, which is a minimally invasive technique. However, the relationship between ABP and CO is not yet fully understood. In this study, we aim to utilize regression-based machine learning techniques and feature engineering to estimate CO from ABP. Hemodynamics and waveform features, along with demographic information of the patient, were integrated to enhance the accuracy of the model.
MSc thesis supervisor: Justin Dauwels. Joint work with Drs. Niki Ottenhof and Jan-Wiebe Korstanje of the Erasmus MC.
More ...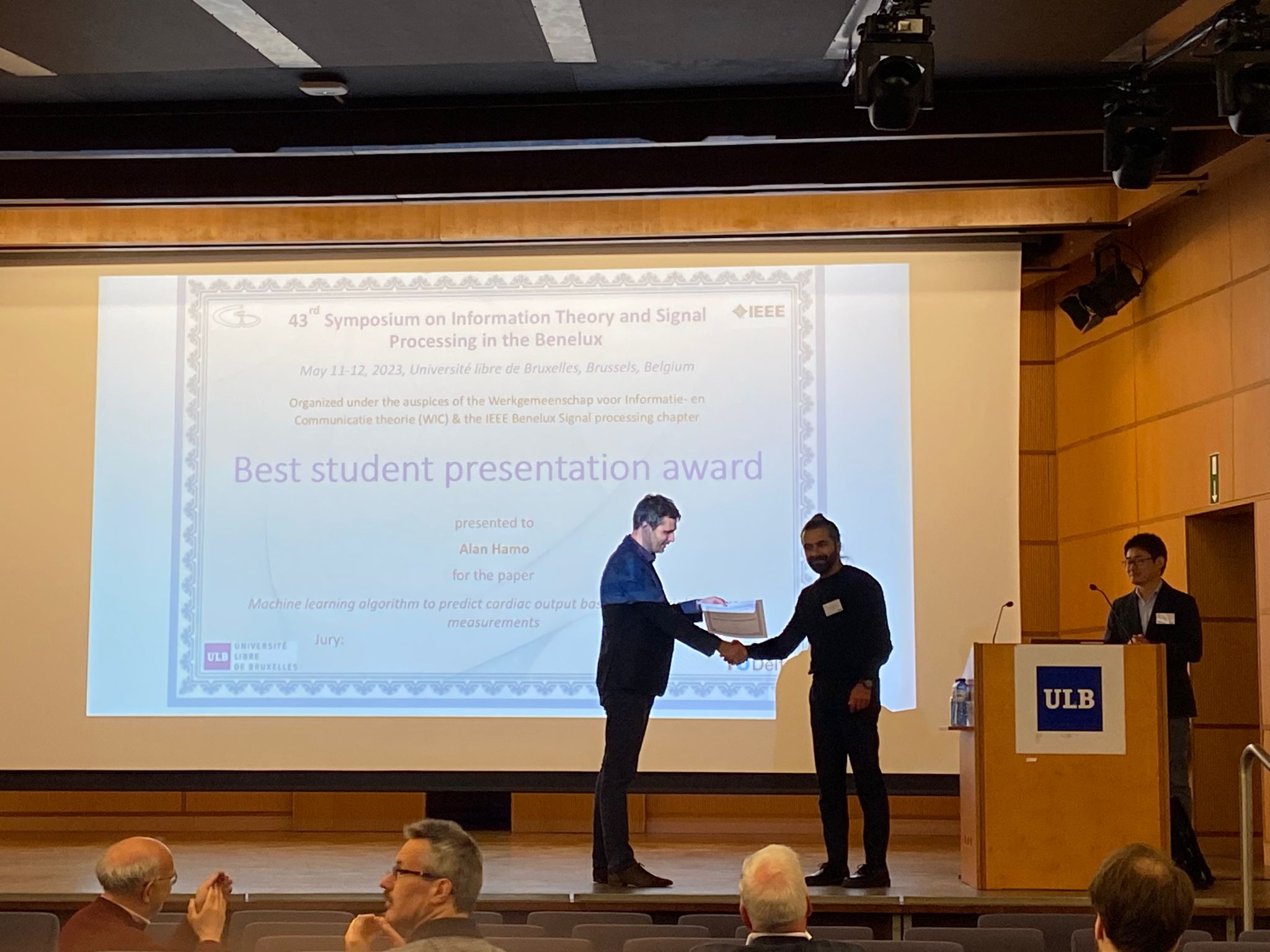
Where is everyone?
- Friday, 12 May 2023
43rd Symposium on Information Theory and Signal Processing in the Benelux (SITB 2023)
More ...
Assistant professor Raj Rajan appointed secretary of "De Nederlandse Vereniging voor Ruimtevaart" (Netherlands Space Society)
- Wednesday, 19 April 2023
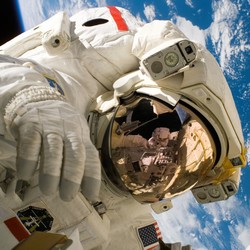
NVR has approximately 1200 members. They include aerospace professionals, students, and people with a personal interest. As of today, the association has been around for over 70 years. Yet, it remains in touch with the newest Dutch and international developments surrounding space and astronautics. The NVR aims to be an association for all ages, and the network for anyone excited by modern aerospace and space travel.
Raj Rajan has joined the Board of NVR as Secretary, starting Feb 2023.
More ...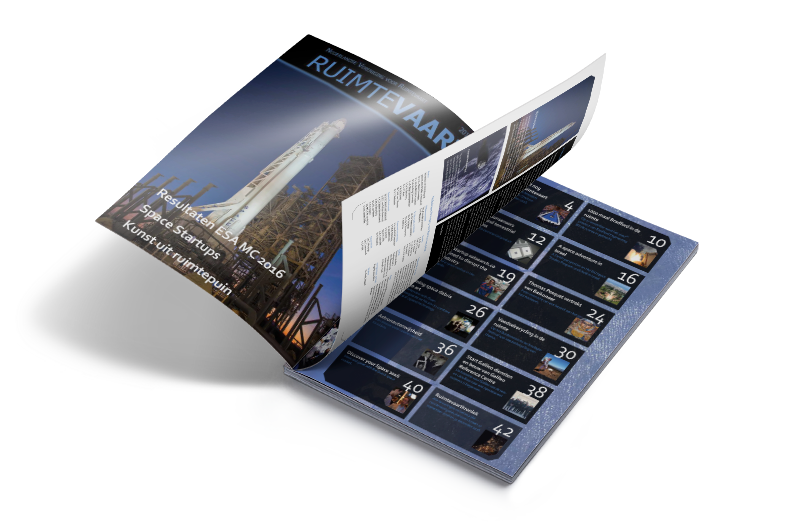
IEEE SPS Student Scholarship Program
- Thursday, 2 March 2023
The IEEE Signal Processing Society (SPS) awards scholarships of up to a total of US$7,000 for up to three years of consecutive support to students who have expressed interest and commitment to pursuing signal processing education and real-world career experiences.
Students and graduate students from all 10 IEEE Regions are encouraged to apply!
This is not a full scholarship but rather a supplementary fund in order to support the signal processing field. Apply before 30 June 2023.
More ...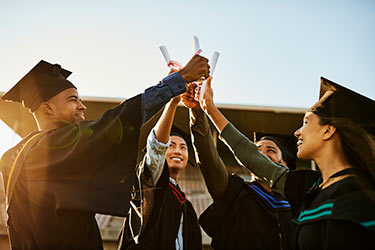
Congratulations Class of 2022!
- Saturday, 31 December 2022
In 2022, SPS (CAS) graduated 48 MSc students, an all-time record for us! In particular, Justin Dauwels (12) and Richard Hendriks (8) had very popular topics, while also biomedical is seeing increasing interest. Four graduates stayed with us to to a PhD.
Congratulations to all the young Engineers!
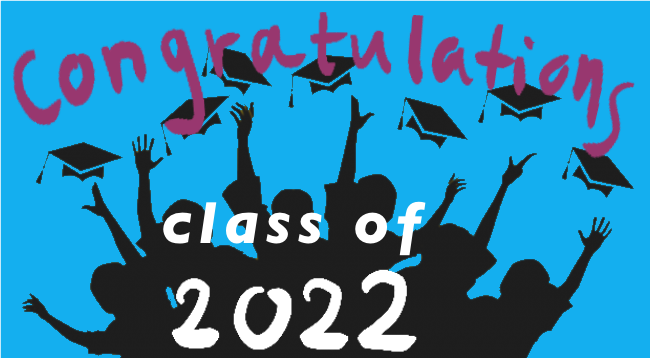
CAS becomes SPS
- Wednesday, 28 December 2022
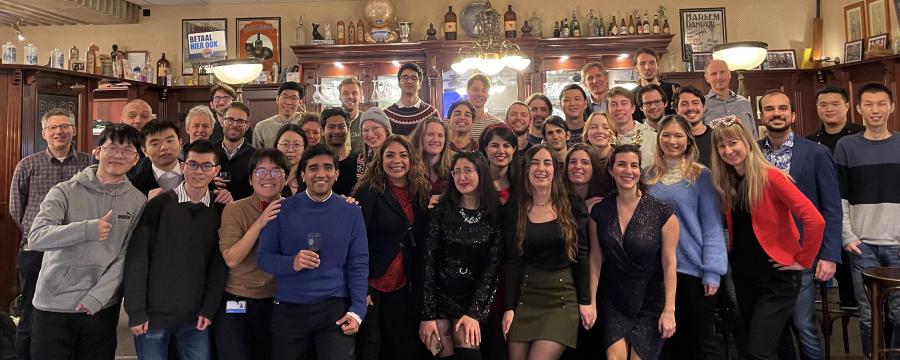
The Circuits and Systems (CAS) group was formed over 20 years ago out of a merger of the Electronic Techiques and the Network Theory group. The resulting group had a focus on system theory and digital VLSI design, in particular design and verification tools.
Over these years, the signal processing part of the group has grown, to a point where now it is time to change our name. Starting from 1 January 2023, the group is called Signal Processing Systems (SPS). Happy New Year!
More ...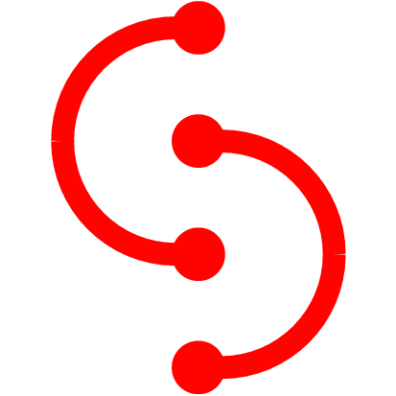
Nature paper for Gerard Janssen
- Thursday, 17 November 2022
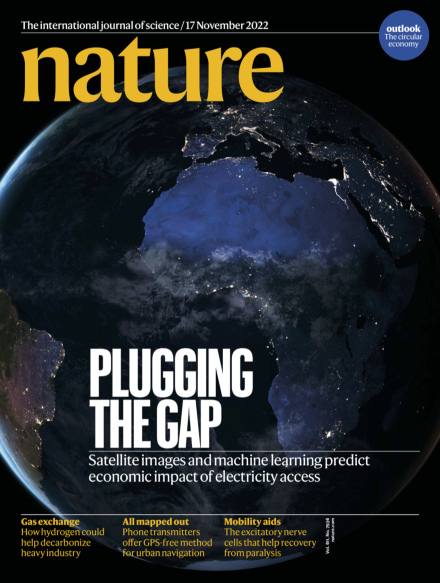
GPS signals are not available everywhere. Especially in urban environments, the satellites may be blocked or the signals experience multipath, leading to timing errors and inaccurate positions in areas where accuracy matters most.
In the SuperGPS project of Gerard Janssen e.a., it is shown how relatively inaccurate wireless transceivers can be networked; the use of wideband signals and carrier phase recovery techniques results in a localization accuracy at decimeter-scale or better; two orders of magnitude better than GPS in certain situations. The perspective is that such transceivers can be embedded in 4G/5G mobile communication networks (the employed protocols are compatible), such that it is relatively inexpensive to roll out this solution.
In the Nov issue of Nature, the project partners describe experimental results acquired on a testbed at The Green Village at TU Delft. An associated article provides an introduction and a perspective. The topic was also covered by IEEE Spectrum.
More ...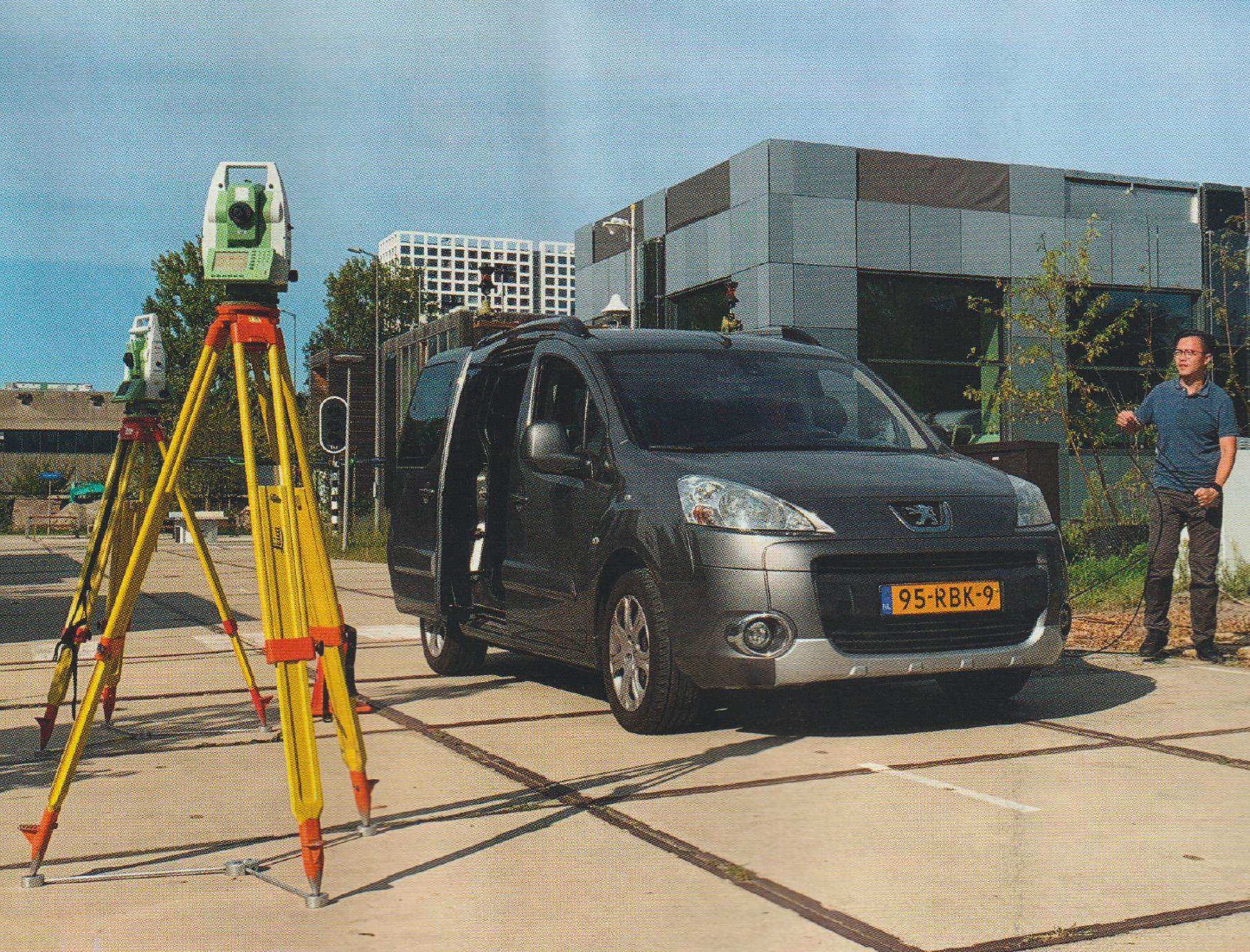
2022 4TU.NIRICT Community Day, November 14
- Thursday, 20 October 2022
Identified are 3 themes around which we would like to bring NIRICT researchers together to discuss and to explore per theme the opportunities for interdisciplinary collaboration. The themes are related to the societal challenges we are currently facing and require interdisciplinary effort in order to be addressed effectively. We believe that productive discussions during the event could be instrumental in forming new research lines, but also project consortia around various national R&D investment programs addressing the knowledge and innovation agenda’s (KIAs) of the Netherlands (https://www.topsectoren.nl/missiesvoordetoekomst).
Themes of the day:
• Health and ICT People live longer, more healthcare is needed. Work pressure in healthcare is rapidly increasing. More data is collected by smart devices. How can the ICT community contribute solutions to keep our society healthy?
• Energy and ICT The current explosion of the energy prices shows that we are facing an unstable situation with respect to energy production and consumption. We produce more solar energy on one hand and consume more energy due to air conditioners and electric cars. We need smart solutions both in production and consumption. Moreover, due to its significant share in energy consumption, ICT systems from the end user devices to power-hungry data centers need to become more energy efficient.
• Agriculture and ICT We just had another summer with extreme heat waves and droughts. The climate changes we are facing have tremendous consequences for agriculture. The population is growing and the pressure on nature is increasing. What smart solutions do we see to deal with the challenges to keep our planet healthy and at the same time to keep the food production in line with the population growth?
The Community Day starts at 12:30hrs with a walk-in lunch. At 13:30hrs the official part will start with a number of short presentations, followed by several parallel sessions regarding the three themes of the day. You can indicate your preference on the registration form. Afterwards there will be a networking drink from 17:00-18:00hrs.
It would be great to welcome many of you on November 14th, because together we strengthen the ICT community of The Netherlands, so join us for the 4TU.NIRICT Community Day 2022, November 14, in Van der Valk Hotel Utrecht and register here by November 6, 2022.
Your data will only be used for registration and will be discarded within one week after the 4TU.NIRICT Community Day. If you have any questions, don’t hesitate to contact us: m.mommers@tue.nl
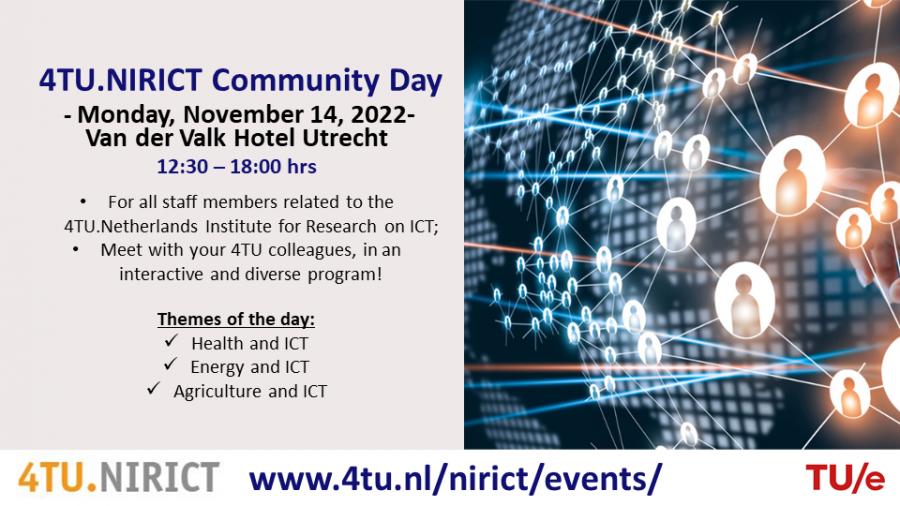
Congratulations Ids
- Friday, 9 September 2022
CAS PhD student Ids van der Werf won the Best Presentation Award at the 2022 IEEE-SPS / EURASIP Summer School "Data and Graph Driven Learning for Communications and Signal Processing". Congratulations!
Ids started just 3 weeks ago as a PhD student; the presentation was actually based on his MSc thesis work on Sound Field Reconstruction.
More ...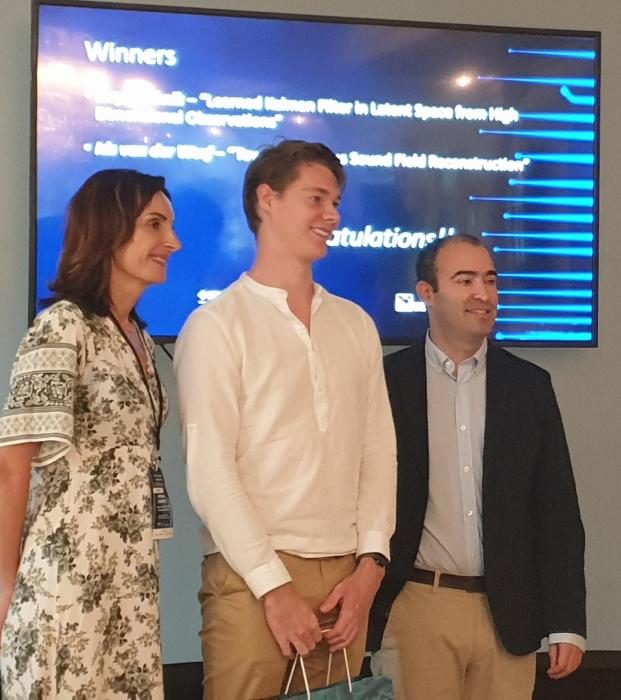
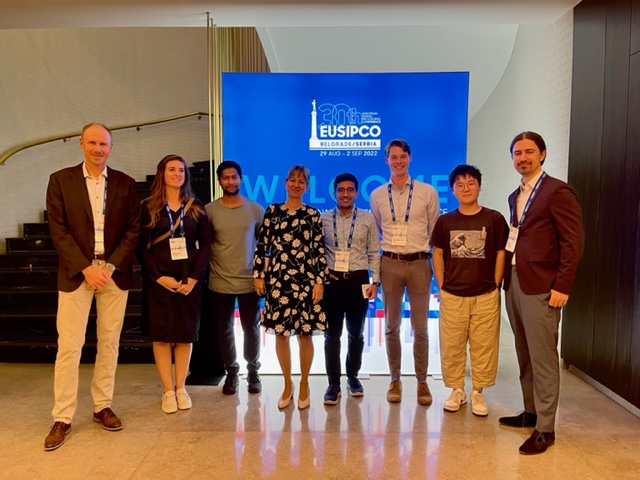
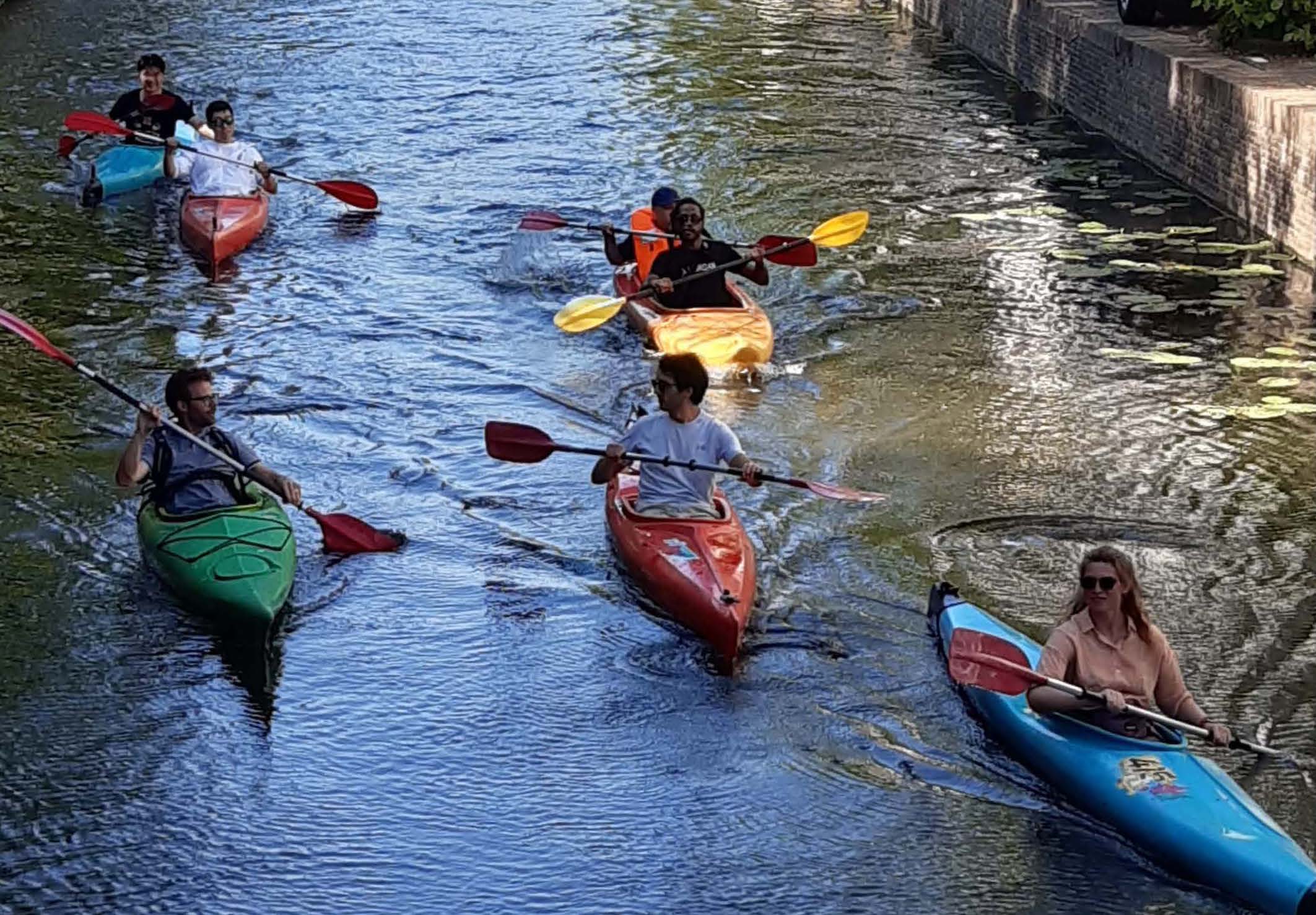
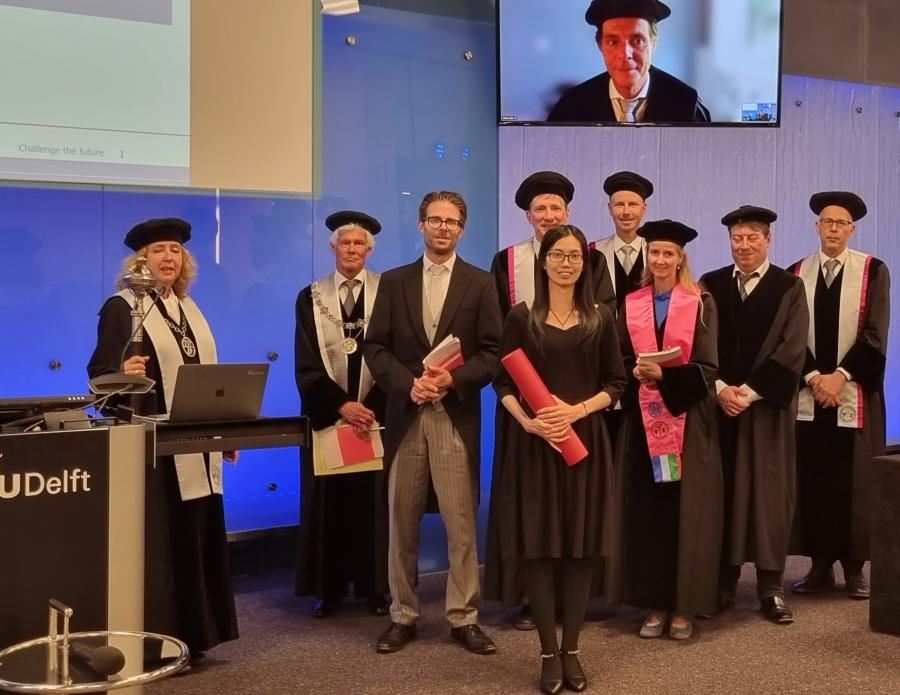
New NWO project: GRASPA
- Friday, 17 June 2022
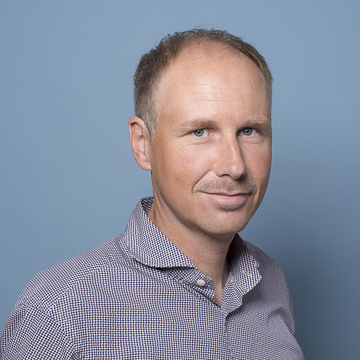
Six research projects receive funding within the Open Technology Programme (OTP) this month. The projects receive a total of about 4 million euros from NWO; organisations involved in the research projects invest a total of 1.3 million euros. Among these 6 projects is a project from prof. Geert Leus from the CAS group; Graph Signal Processing in Action (GraSPA).
Graph signal processing (GSP) is the exciting research field that extends concepts from traditional signal processing to signals living in an irregular domain that can be characterized through a graph. GSP is extremely promising for applications in transportation networks, smart grid, wireless communications, social networks, brain science and recommender systems, to name a few. This project focuses on the non-trivial extension of GSP to time-varying or dynamic networks, where either the connections or the nodes can change. We will develop innovative tools to estimate such time-varying graphs from data and devise new graph filtering schemes for denoising, interpolation, and prediction. The developed techniques will be applied to brain activity monitoring, which is crucial to understand the working of the brain, as well as recommender systems, which are omnipresent in our daily lives.
More information: Four million euro for six technological research projects | NWO
More ...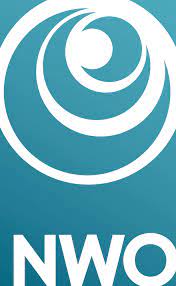
New NWO-TTW project: GraSPA
- Friday, 3 June 2022
Graph signal processing (GSP) is the exciting research field that extends concepts from traditional signal processing to signals living in an irregular domain that can be characterized through a graph. GSP is extremely promising for applications in transportation networks, smart grid, wireless communications, social networks, brain science and recommender systems, to name a few. This project focuses on the non-trivial extension of GSP to time-varying or dynamic networks, where either the connections or the nodes can change. We will develop innovative tools to estimate such time-varying graphs from data and devise new graph filtering schemes for denoising, interpolation, and prediction. The developed techniques will be applied to brain activity monitoring, which is crucial to understand the working of the brain, as well as recommender systems, which are omnipresent in our daily lives.
This project will fund 2 PhD students. PIs are Geert Leus and Elvin Isufi.
More ...Happy Secretaries Day!!!
- Thursday, 21 April 2022
Our congratulations and best wishes!
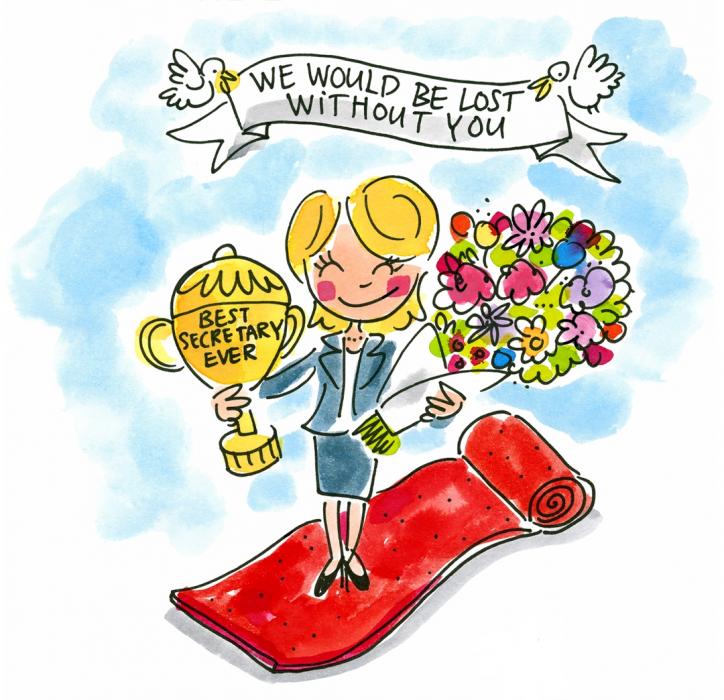
Raj Thilak Rajan elected to the IAF-SCAN committee
- Wednesday, 16 March 2022
Raj Thilak Rajan was elected to the Space Communications and Navigation Committee (SCAN) of the International Astronautical Federation (IAF).
The SCAN committee is responsible, within the IAF, for all aspects of satellite-based communication and navigation systems and technology. The committee will organise the SCAN symposium at each IAC and, when appropriate, will organise specialised plenary sessions and Highlight Lectures. It will provide annual inputs to the IAF report to the United Nations.
For more information: see link.
More ...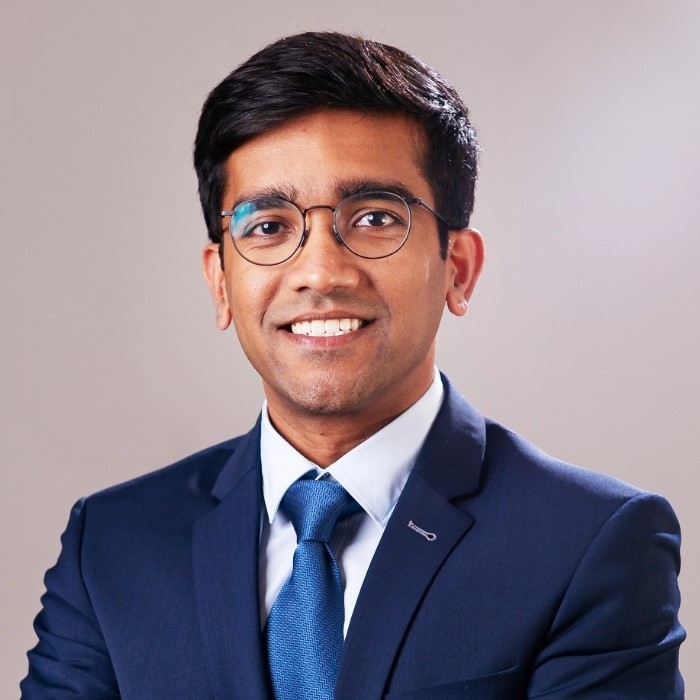
Justin Dauwels wins the Vincent Bendix Automotive Electronics Engineering Award
- Wednesday, 2 February 2022
A paper by Justin Dauwels (from the time he was in Singapore) has won the Vincent Bendix Automotive Electronics Engineering Award.
SAE is a foundation for automotive engineers, founded in 1904 by Henri Ford, and with 100,000+ members.
More ...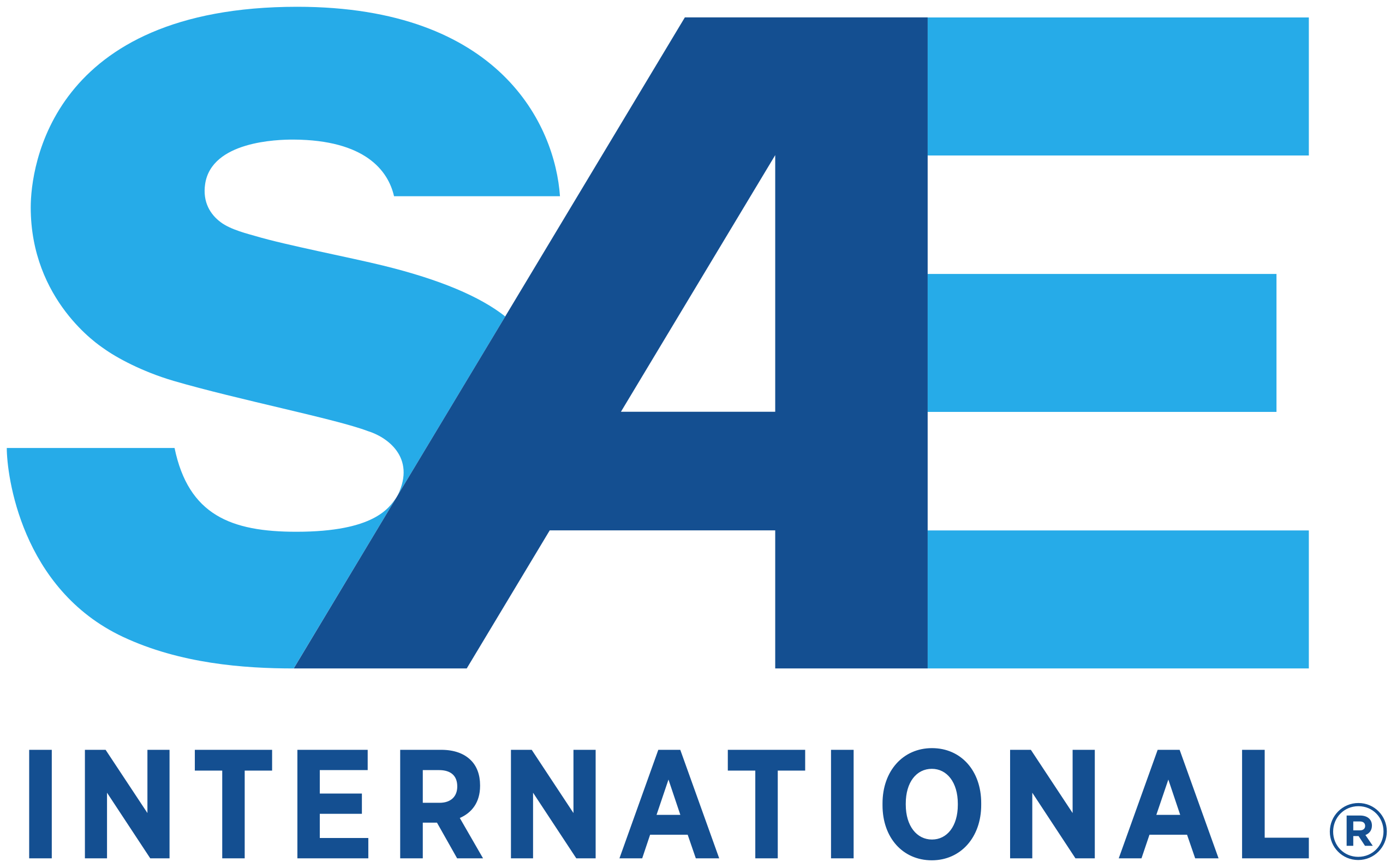
Alle-Jan van der Veen appointed IEEE Signal Processing Society Vice President
- Wednesday, 26 January 2022
Alle-Jan van der Veen has been appointed IEEE Signal Processing Society Vice President for Technical Directions, for the term 2022-2024. In this capacity, he is member of ExCom and chairs the Technical Directions Board (comprising the 12 Technical Committees of the SP Society).
More ...
Minister of Education "meets" Jorge
- Friday, 21 January 2022
On 20 January, our new Minister of Education Robbert Dijkgraaf interviewed a few young scientists. Jorge Martinez was one of them.
On twitter: "Jonge wetenschappers inspireren mij enorm. Ik sprak ze over hun ideeën voor de toekomst van de wetenschap en hun werkdruk en de werkcultuur. Daarnaast vertelden zij over interne en externe stressfactoren en het pad dat zij voor zich zien, ook buiten de wetenschap. Wordt vervolgd!"
More ...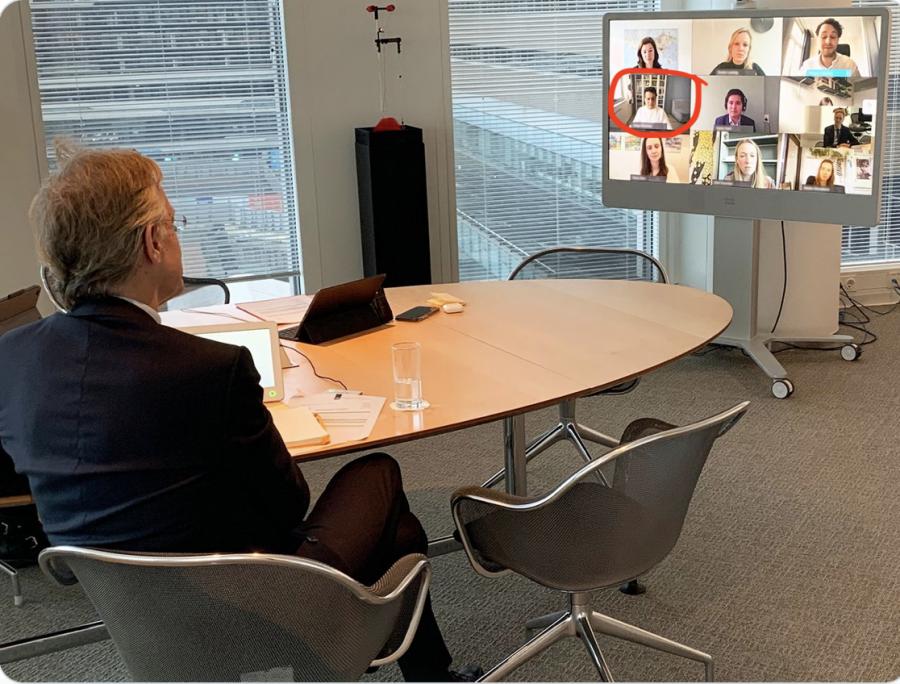
ISOCC 2021 ISE President Best Paper Award
- Wednesday, 8 December 2021

At this year's edition of ISOCC 2021, our former excellent bachelor and master student, Jun Feng, obtained the "2021 ISE President Best Paper Award" for his paper: "A Versatile and Efficient 01.-to-11 Gb/s CML Transmitter in 40-nm CMOS."
Ph.D. candidates Mohammadreza Beikmirza and Milad Mehrpoo contributed to Jun's outstanding work. Jun is now a Ph.D. candidate at KU-Leuven. We congratulate Jun, Mohammadreza, and Milad for this award and send our best wishes for their future research.
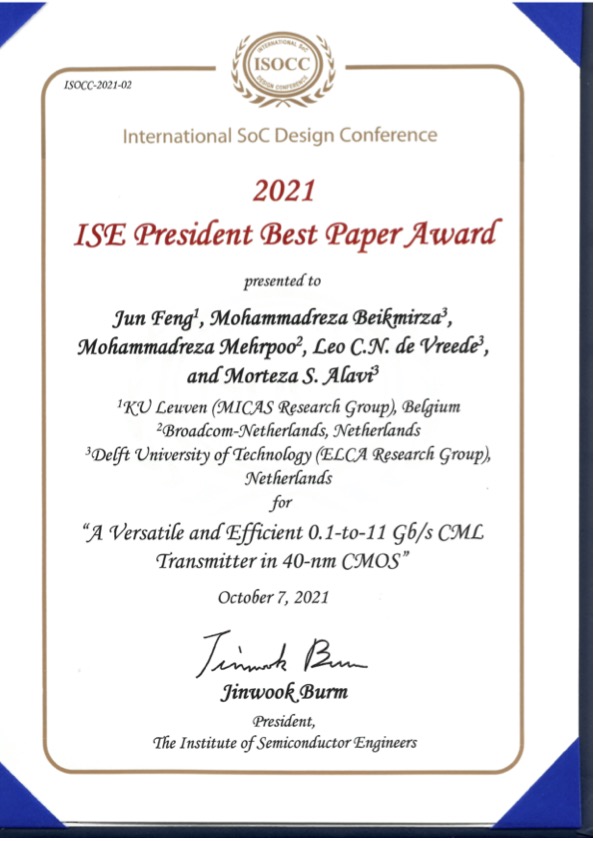
New DAI Labs for Raj Rajan
- Thursday, 11 November 2021
Raj was awarded a DAI Labs, with 3ME (Manon Kok). This will fund 2 PhD positions for CAS, and generate some TU-wide publicity.
The awarded proposal is "Sensor AI" and investigates sensor networks, sensor fusion, distributed learning, sensor swarms. The 3ME part also looks at human motion estimation and including physical knowledge into learning systems.
More ...New Medical Delta professors
- Friday, 5 November 2021
Congratulations Wouter Serdijn, Natasja de Groot and Andrew Webb on your inauguration as Medical Delta Professor
More ...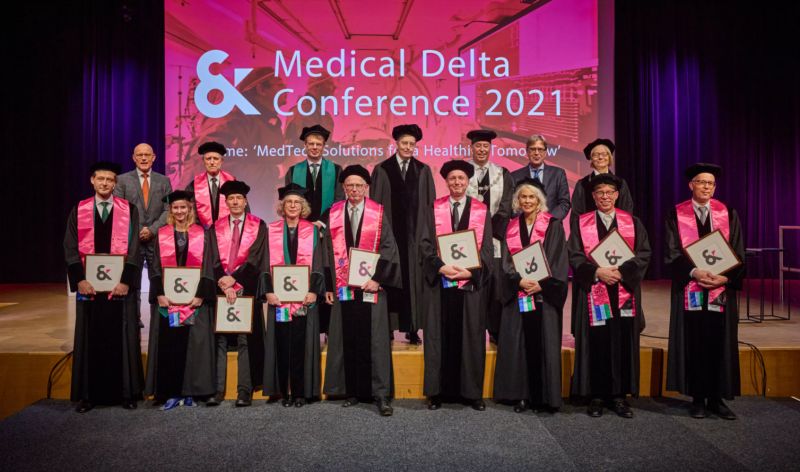
Innatera: Spiking neural network architecture
- Wednesday, 6 October 2021
A short video about the Spiking Neural Hardware Architecture Innatera is working on. (Innatera is a startup originating in the CAS group.)
More ...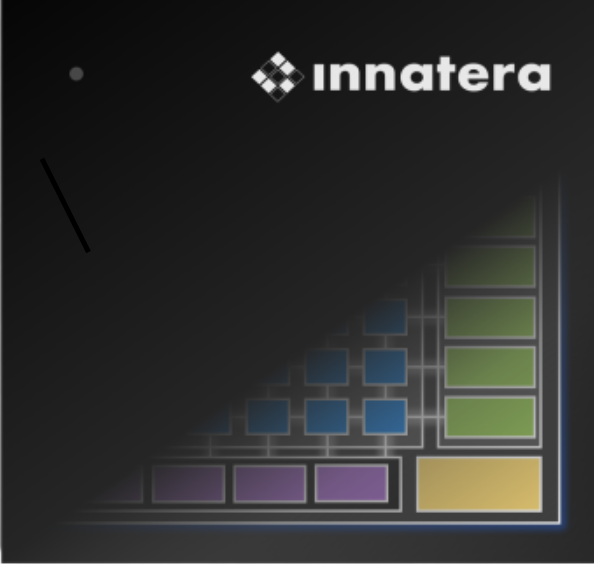
Welcome Geethu Joseph
- Monday, 16 August 2021
The Department of Microelectronics and CAS are welcoming dr. Geethu Joseph, who starts in August 2021 as a new Assistant Professor. Her expertise is in signal processing for communications, in particular machine learning, compressed sensing and sparse recovery on graphs and networks, as well as dynamical systems theory.
More ...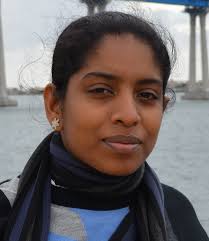
Rising star: Justin Dauwels
- Thursday, 1 July 2021
As associate professor within the Circuits and Systems group at the faculty of Electrical Engineering, Mathematics and Computer Science, rising star Justin Dauwels is fascinated by how data-driven intelligent systems can tackle many of society’s problems. Neural networks and graphical models are his hammer, and he applies it to topics ranging from autonomous cars to digital health and beyond. But neuroscience has always been a main theme ever since his Erasmus exchange to the Institute of Neuroinformatics in Zürich. ‘I apply the models that I studied as an engineer to help unravel and understand human behaviour,’ he says.
Healthcare for the underprivileged
In one of his long-standing projects, he developed machine learning tools to reliably diagnose epilepsy and other related brain conditions from EEGs – recordings of electrical activity on the scalp – allowing neurologists to spend more time with their patients. He has also used audio and video recordings between psychiatrists and their patients to help diagnose various mental health indications such as depression and schizophrenia as well as the severity of a patient’s symptoms. His drive in developing these tools is to bring world-class healthcare to the underprivileged, such as at Sion’s public hospital in Mumbai which he frequently visited. ‘It serves the local community, with doctors who are severely underequipped and completely overburdened,’ he says. ‘The various intellectual properties have been licensed to a startup focused on brain health to make sure these developments reach the patients.’We want to be able to answer what-if questions prior to the operation; if I remove some tissue here, what will that accomplish?
Planning epilepsy operations
A more recent project, and one that he will continue to pursue at TU Delft, is to establish a paradigm shift in the planning of operations for epilepsy patients for whom medication alone leads to an insufficient reduction in symptoms. ‘Currently, the decision on where to operate is primarily based on abnormalities observed on a CT-scan,’ he says. ‘We want to be able to answer what-if questions prior to the operation; if I remove some tissue here, what will that accomplish?’ Using advanced imaging, electrical measurements, and anatomical knowledge, Dauwels and collaborators buildt a very simple mathematical model of the patient’s brain. They can subsequently perform virtual resections and run the model to see if it reduces epilepsy attacks. Using only a basic model, this technique was more than 80% correct in a small cohort of patients for whom the outcome of the actually performed surgery was known. ‘We will now improve our model and analyse more patients.’
Next generation deep learning
TU Delft was already on Dauwels’ radar at the time of his master’s thesis, but he chose to first sharpen his skills in Japan, the USA and Singapore. But now, TU Delft is the perfect setting for him. Partially because it is much closer to his parents, who still live in Belgium, and especially because Medical Delta and the ongoing convergence with Erasmus University and ErasmusMC allow him to cooperate with many top-level clinicians. ‘Pretty much everyone in our group has ties with ErasmucMC, meaning there are many opportunities to apply my hammer,’ he says.But it is not all about applications. He will also delve into the fundamentals of deep learning. ‘People start to realise that neural networks, on their own, won’t be able to address all problems. I have been working on graphical models for more than twenty years and these are a promising path towards next generation deep learning systems. It’s about building a better hammer, one that combines the fast, instinctive thinking of neural networks with the slow thinking of graphical and logical models. Just like in humans, it makes sense to verify an intuitive answer and to correct it if necessary.’
More ...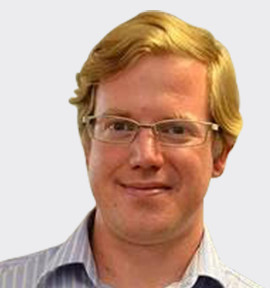
New TTW project for Richard Hendriks and Jorge Martinez
- Thursday, 24 June 2021
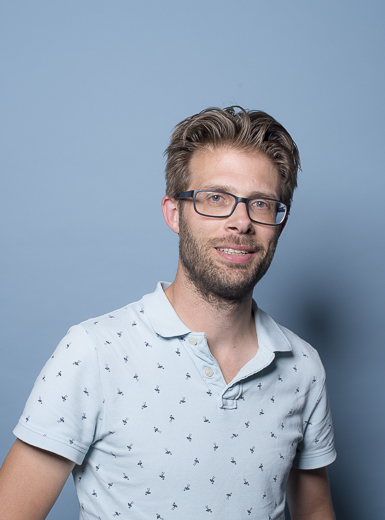
This week Richard Hendriks received the news that his project proposal 'Personalized Auditory Scene Modification to Assist Hearing Impaired People' was granted funding from NWO TTW. Co-applicant is Jorge Martinez. The project will fund 2 PhD students. Congratulations!
Hearing impairment has become a serious problem for a large and increasing portion of the population as is shown by its prevalence: 11 % of the Dutch population suffers from severe hearing loss. These numbers show that hearing impairments form an important societal problem. It comes with many daily life problems in both private situations and working environments. Moreover, hearing-impaired people are often less confident in practical situations, need more assistance and have a worse quality of life. The underlying reasons are that hearing-impaired people suffer from a) the inability to understand speech in acoustic challenging situations, and, b) the inability to correctly localize sound sources. The goal of this project is to make the hearing impaired user fully benefit from an improved intelligibility and sound localization. To do so, we will develop an algorithm to personalize the presented auditory scene for improved speech intelligibility and sound localization for hearing impaired users.
More ...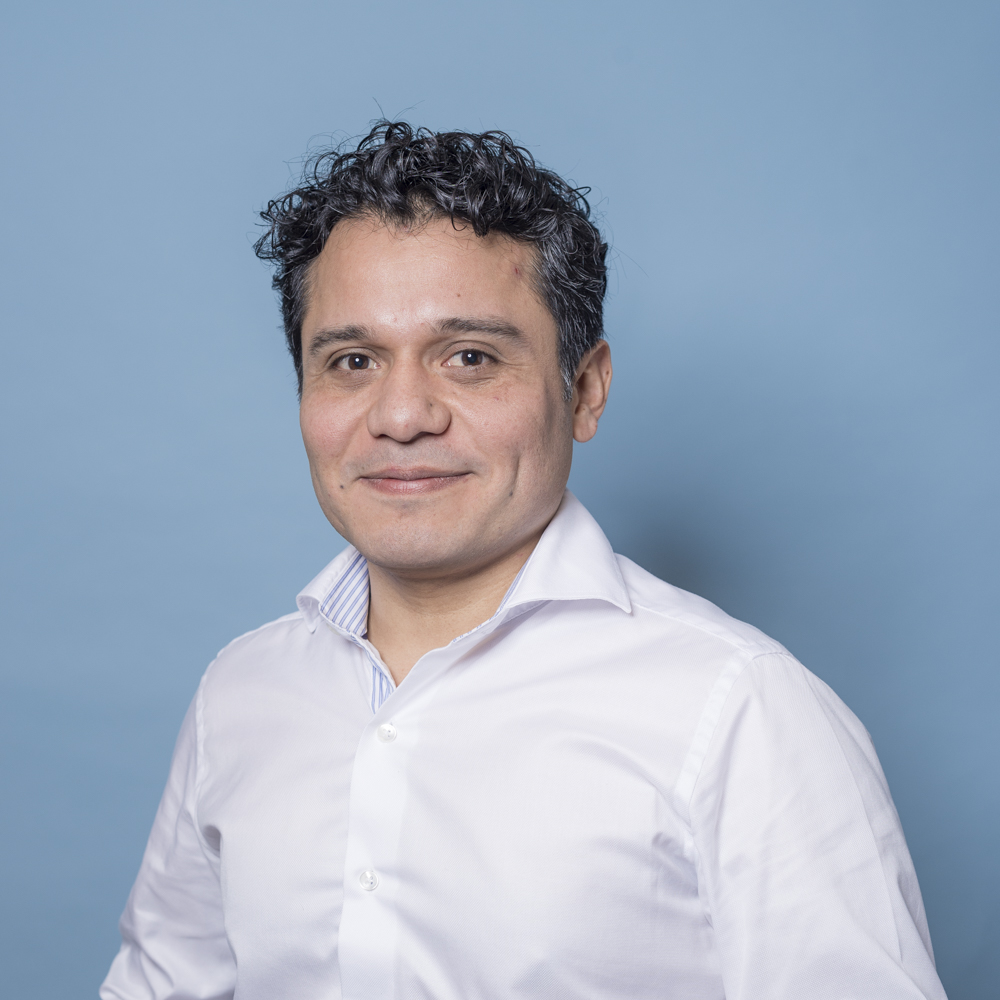
The rising stars of the TU Delft, featuring Dante Muratore
- Tuesday, 8 June 2021
After his PhD in what he calls “hardcore analogue microelectronics”, rising star Dante Muratore knew he wanted to continue his career working on systems that are closer to an actual application. A postdoc position at Stanford University, in which he worked on the electronics for an artificial retina to treat medical conditions leading to the loss of vision, brought him just that. Then, wanting to come back to Europe and to continue doing bioelectronics at the highest level possible, an opening at TU Delft crossed his path. ‘It was the easiest choice I ever made,’ he says.
Read more about Dante in the link below!
More ...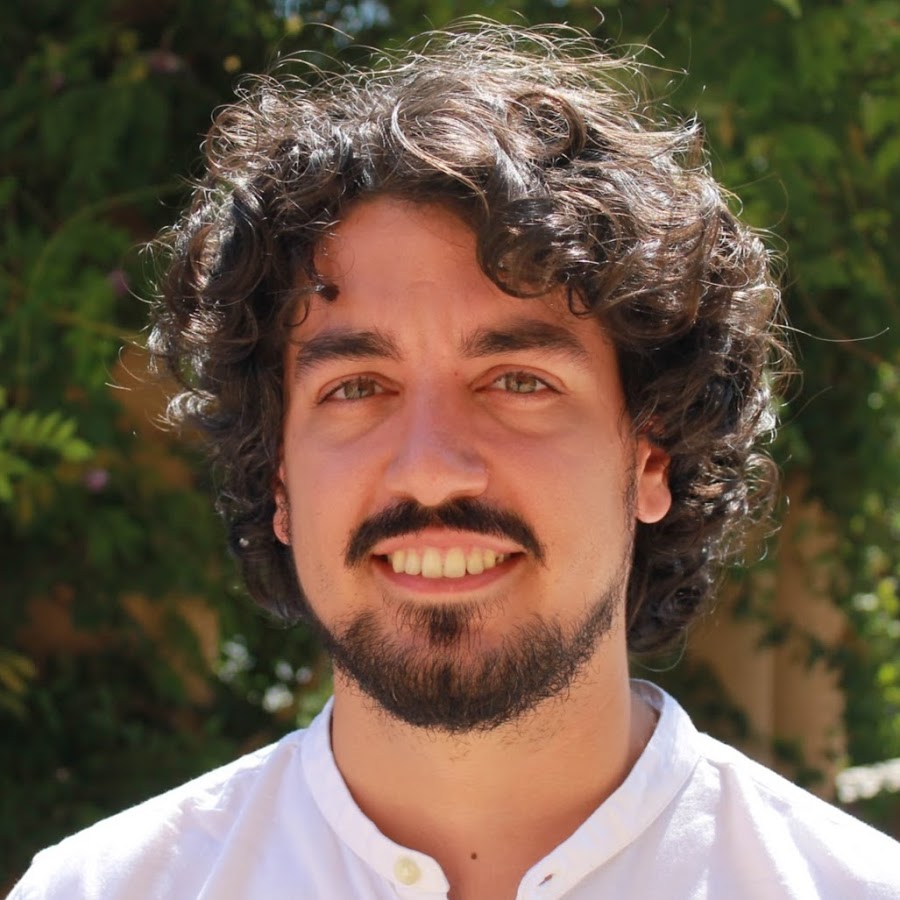
Mario: cum laude PhD defense
- Wednesday, 21 April 2021
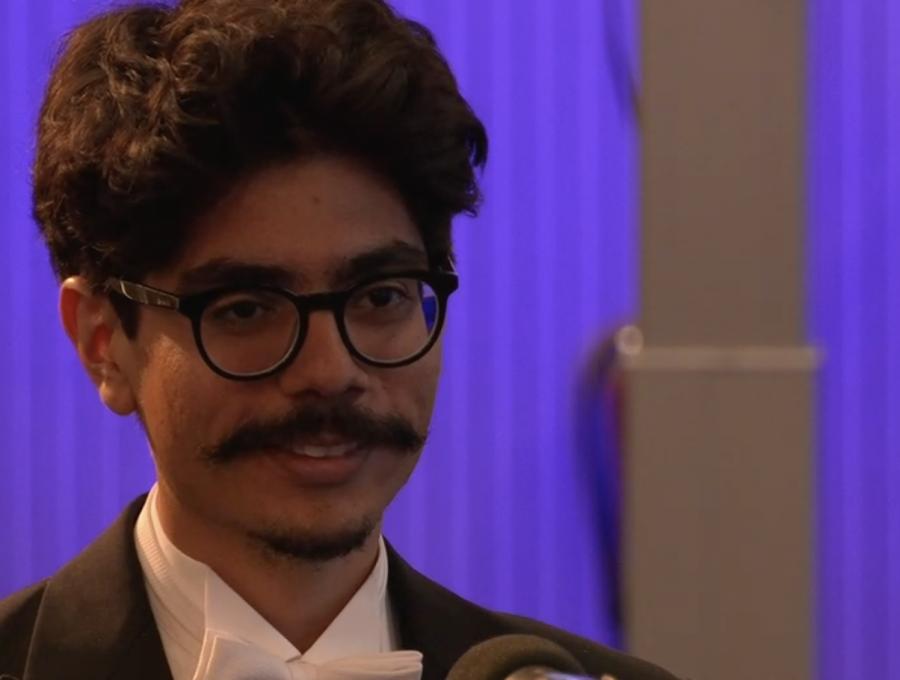
Today Mario Coutino defended his PhD thesis, and received the title "cum laude". Congratulations!
More ...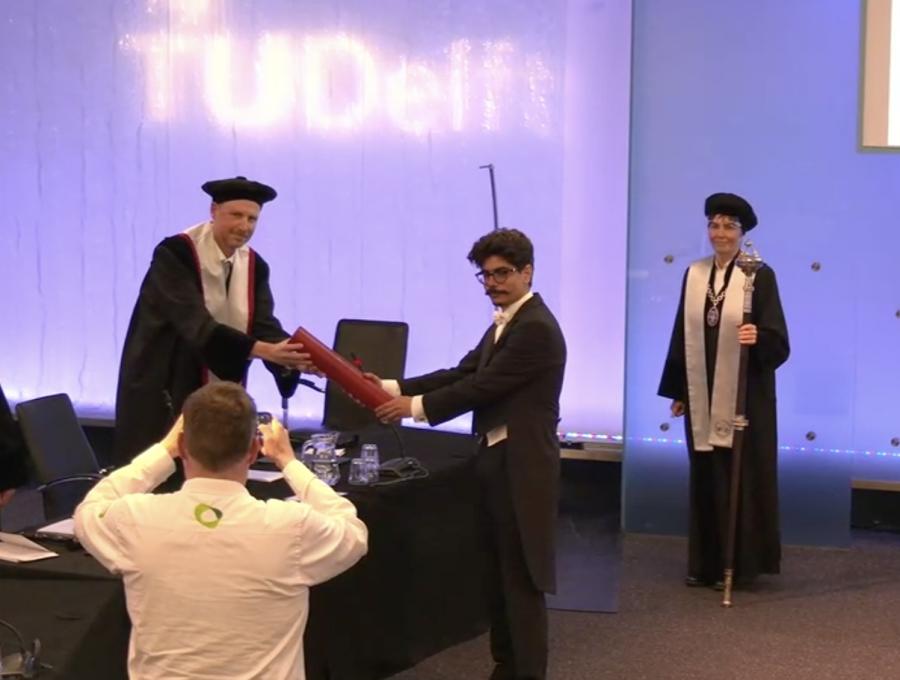
"Tech for Health" featuring Natasja de Groot
- Sunday, 28 March 2021
Please donate to our research on better understanding of cardiac arrhythmia
More ...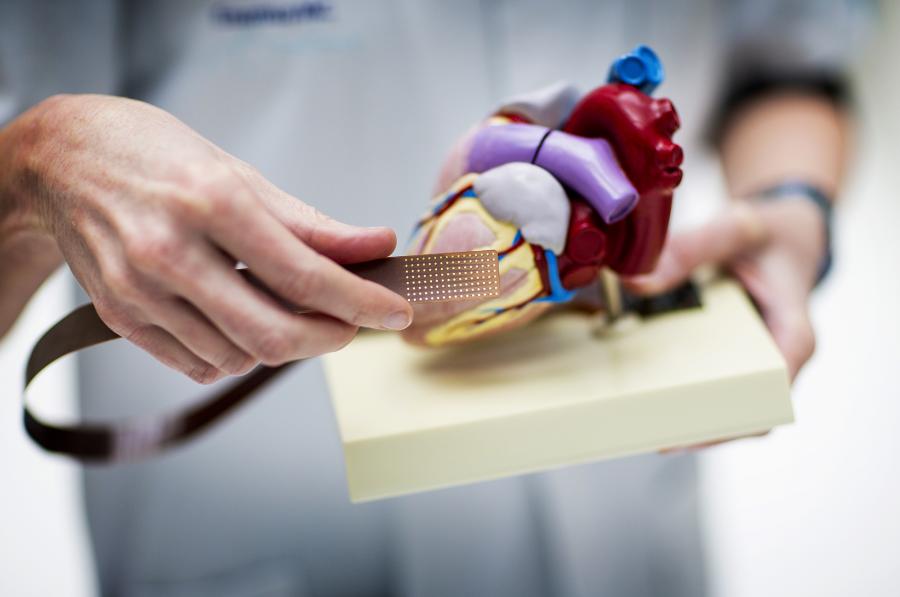
Geert Leus wins EURASIP Technical Achievement Award
- Friday, 26 February 2021
Geert Leus
will be this year's recipient of the European Association for Signal Processing (EURASIP)'s Technical Achievement Award.
The
award will be presented at EUSIPCO 2021 Dublin, Ireland.
As noted in the announcement by Maria Sabrina Greco, EURASIP Director for Awards,
The Technical Achievement Award honors a person who, over a period of
years, has made outstanding technical contributions to theory or
practice in technical areas within the scope of the Society, as
demonstrated by publications, patents, or recognized impact in this
field.
This year’s recipient is Geert Leus, “For contributions to signal processing for communications and sensing".
More ...
Cardiac mapping of very young children reveals conduction disorders related to atrial fibrillation
- Friday, 8 January 2021
Research of Medical Delta professor prof. dr. Natasja de Groot (Erasmus MC and TU Delft)
More ...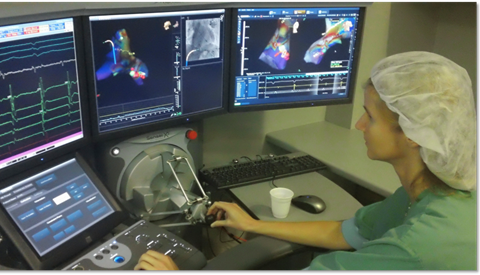
New Associate Professor: Justin Dauwels
- Saturday, 12 December 2020
Per 1 January 2021, Prof. Dauwels starts at CAS. His expertise is in statistical signal processing and machine learning. Welcome!
More ...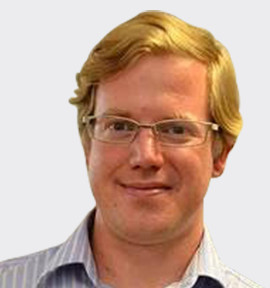
EUSIPCO 2020 - virtual event on 18-22 January 2021
- Sunday, 6 December 2020
The organizing committee of EUSIPCO 2020 has decided that EUSIPCO 2020 will be a full virtual conferencedue to the on-going COVID-19 pandemic and resulting travel restrictions.
We are excited to be able to provide a virtual venue for EUSIPCO 2020 and hope you will join us and learn about the latest developments in research and technology for signal processing.
Please register today for a reduced fee of E 50, or sign up for one of the tutorials for E 25.
More ...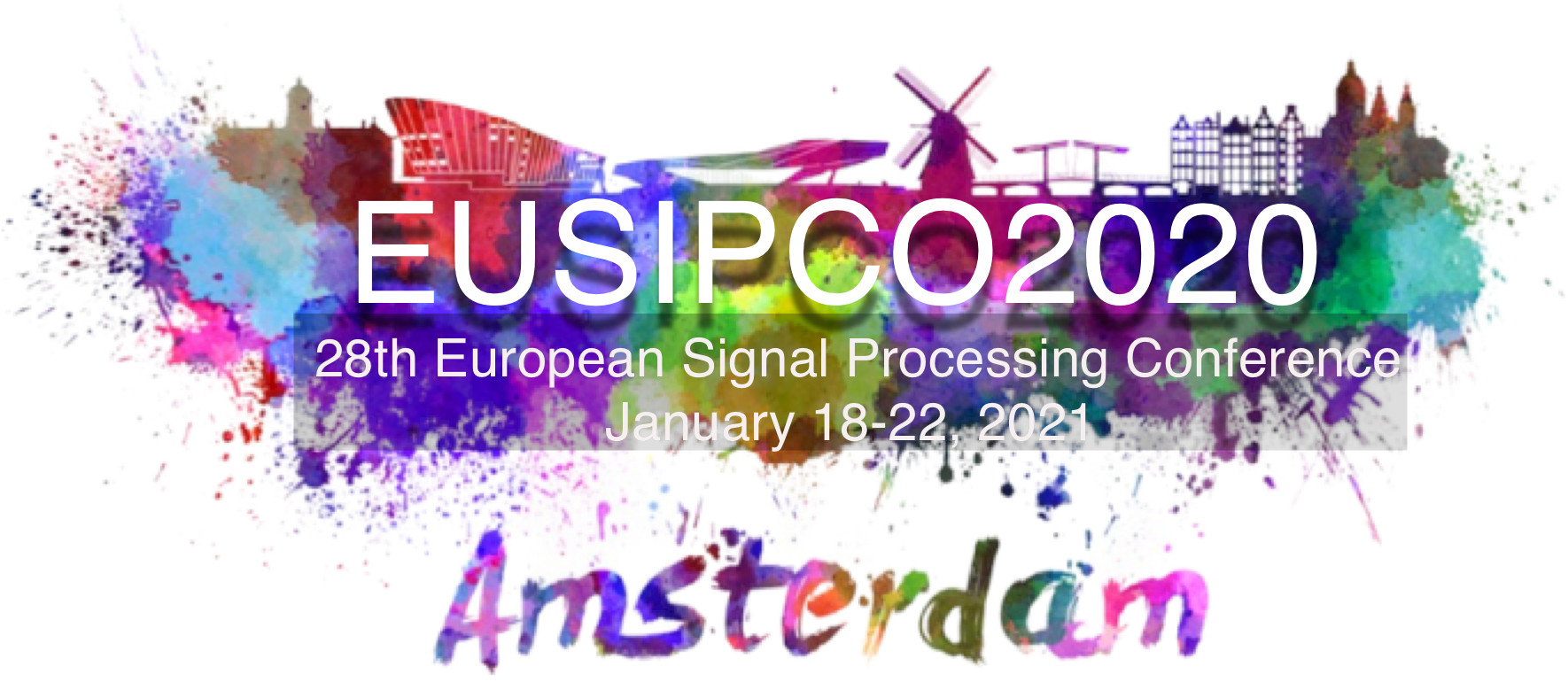
CAS startup Innatera Nanosystems raises €5 million
- Wednesday, 25 November 2020
Innatera, started by CAS members Sumeet, Amir and Rene, is making the next step
More ...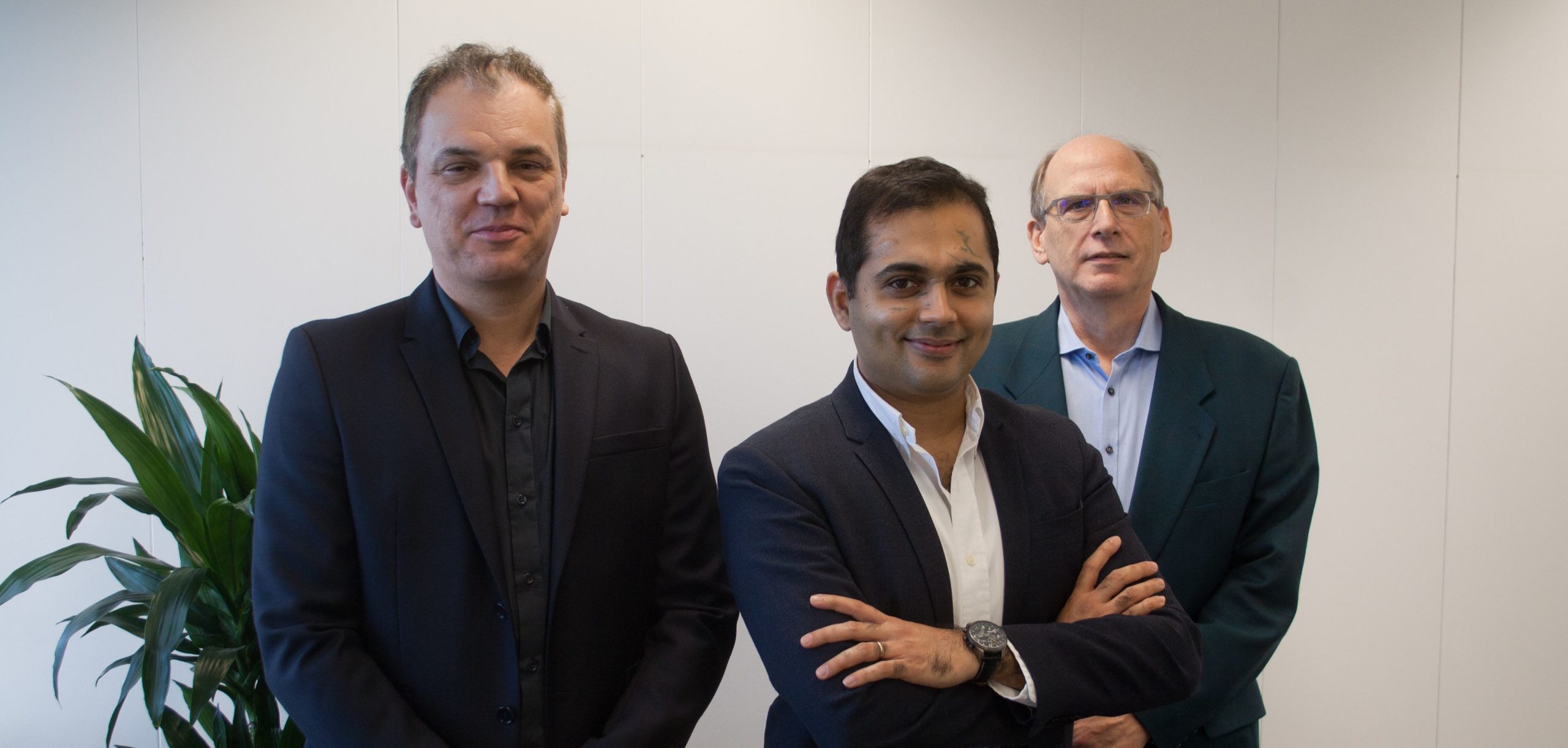
Congratulations for Aydin
- Thursday, 22 October 2020
On 20 October, Aydin defended his PhD thesis. Congratulations!
More ...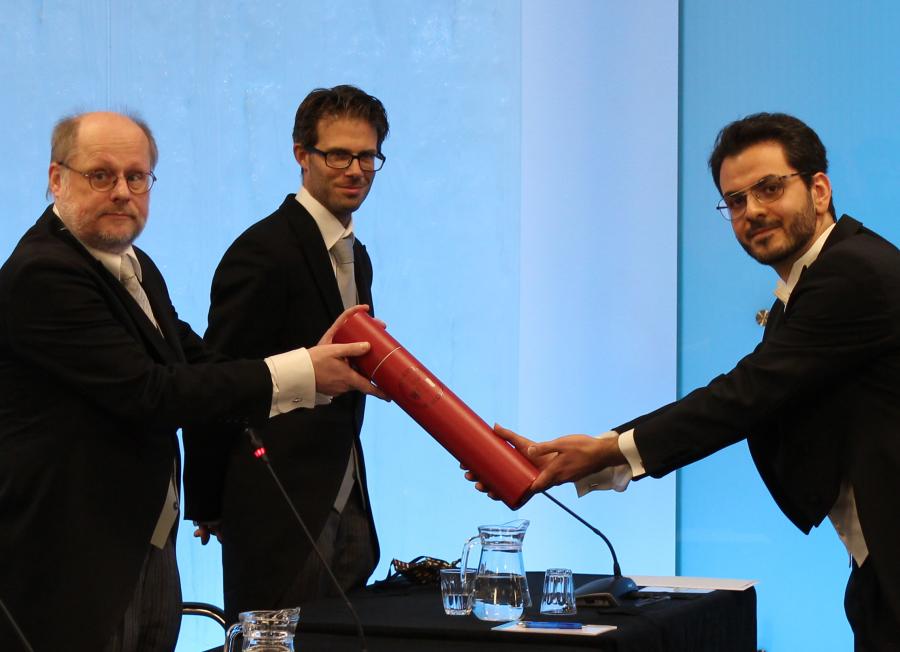
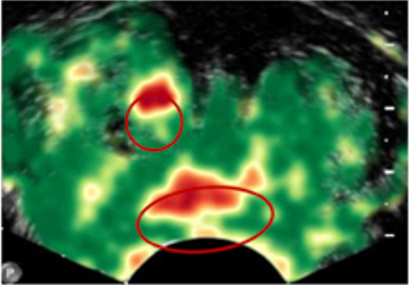
SSCS WYE Webinar
- Tuesday, 4 August 2020
Webinar: To Academia, or to Industry, That is the Question. Presented by: Kofi Makinwa and Shin-Lien Lu
Abstract:
You are about to finish graduate school or perhaps a young or seasoned professional, contemplating a career transition. Which is better - a career in academia or industry? What are the pros and cons of one versus the other? How can you start exploring and build up your career accordingly? In this webinar, we will interview Dr. Linus Lu, a professor-turned-industry veteran, and Prof. Kofi Makinwa, an industry veteran-turned-professor, who will share their insights and perspectives from their personal journeys in both academia and industry careers. They will also address what triggered their transitions, how they staged their transitions, and offer their crystal ball projections on present and future career prospects in the solid-state-circuits profession. More ...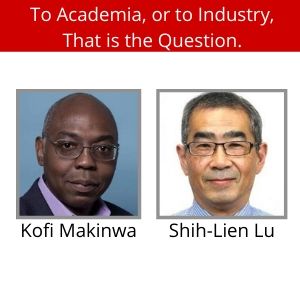
Medical Delta Professors appointed
- Monday, 22 June 2020
Medical Delta appointed 9 new MD Professors, with joint appointments at LUMC and TU Delft, or Erasmus MC and TU Delft. Three of these are connected to the MicroElectronics Department: Wouter Serdijn, Andrew Webb, and Natasja de Groot.
Prof. Dr. Natasja de Groot (Erasmus MC, TU Delft) researches the use of sensors and catheters to more accurately diagnose and treat cardiac arrhythmias. At TU Delft, she will have an affiliation with CAS and BE.
Prof. Dr. ir. Wouter Serdijn (TU Delft, Erasmus MC) researches the use of bioelectronics in medical research. At EMC, he will have an affiliation with Neuroscience.
Prof. Dr. Andrew Webb (LUMC, TU Delft) researches how imaging can be more widely available for medical purposes. He is a professor in MRI at LUMC, and already had a part-time appointment at CAS.
The new Medical Delta professors introduce themselves and their research in a short video. This can be viewed here:
More ...
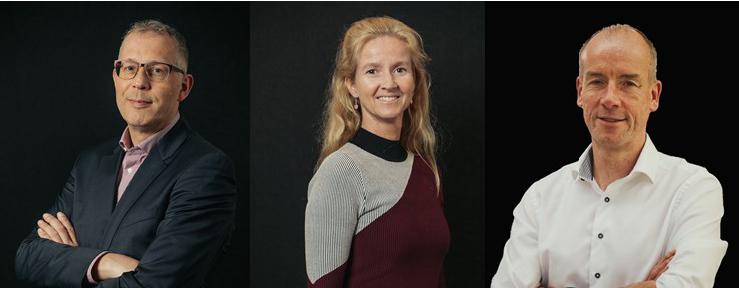
TU Delft launches first eight TU Delft AI Labs
- Wednesday, 17 June 2020
TU Delft is setting up eight new AI Labs to investigate how artificial intelligence (AI) can accelerate scientific progress. To this end, scientists researching AI will be working together with scientist who use AI in their research. The first of these eight interdisciplinary AI labs will be followed by another sixteen in the course of 2020 and 2021.
Rapid developments in AI, data science and digitalisation can accelerate scientific progress in all fields, ranging from medical science to infrastructural research, and across all levels, from fundamental to applied research. TU Delft is boosting collaboration between AI scientists and scientists in other domains, with the launch of a series of TU Delft AI Labs.
Within the MACHINA Lab, for example, researchers in machine learning work together with materials scientists on the analysis of existing materials and the development of new materials. Within the AidroLab, researchers in geometric deep learning are working with researchers in water management on subjects such as how to improve flood forecasting in the urban environment. Researchers in the CiTyAI-Lab will use a wide variety of data sources to map the impact of the city's 'fabric' on its inhabitants in order to improve the living environment.
Education
AI-related knowledge is indispensable for future generations of engineers and scientists. That is why the labs also aim to strengthen education in the field of AI, data sciences and digitalisation, and to create links with educational programmes in various scientific domains.Investments
TU Delft plans to double its budget in the field of AI, data & digitalisation to 70 million euros per year. These funds will be used for the recruitment of talented researchers, the establishment of research units, the development of educational programmes on AI, data and digitalization, and on the strengthening of collaborations, partnerships and networks.
DeTAIL: Delft Tensor AI Lab
The DeTAIL research lab was proposed by Bori Hunyadi (CAS) and Kim Batselier (3mE), and studies training and innovation in tensor-based AI methods for biomedical signals.Real-life biomedical data is often high-dimensional. Current signal processing solutions artificially segment such high-dimensional data into shorter one- or two-dimensional arrays, causing information loss by destroying correlations between these data. At the same time, advances in biomedical sensor and imaging technology – such as substantially larger recording durations of wearable sensor technology and the unprecedented increase in spatial and temporal resolution of the latest neuroimaging techniques – have led to ever increasing data sets. Tensors (multi-dimensional arrays) are the data structure of choice in artificial intelligence research to exploit the full potential of these data in a timely manner.
Within the DeTAIL Lab, we focus on both the development and application of novel low-rank tensor methods for biomedical signal processing, thereby enabling a much faster training of AI models from large datasets without any loss of accuracy.
We will exploit an as of yet unused property of real-life data; the fact that different modes of data may be correlated. Using tensor decompositions, we can find these correlations as well as compress the data, speeding up computations significantly.
Our findings will, for example, be applied to detect events, such as epileptic seizures, through the classification of multichannel time series data based on labelled training data. We also aim to reveal hidden structure, such as functional networks, in neuroimaging data. As biomedical innovation is a defining characteristic of the TU Delft, we will develop an interfaculty elective course on AI tensor methods to satisfy the expected continual increase in demand for such knowledge
More ...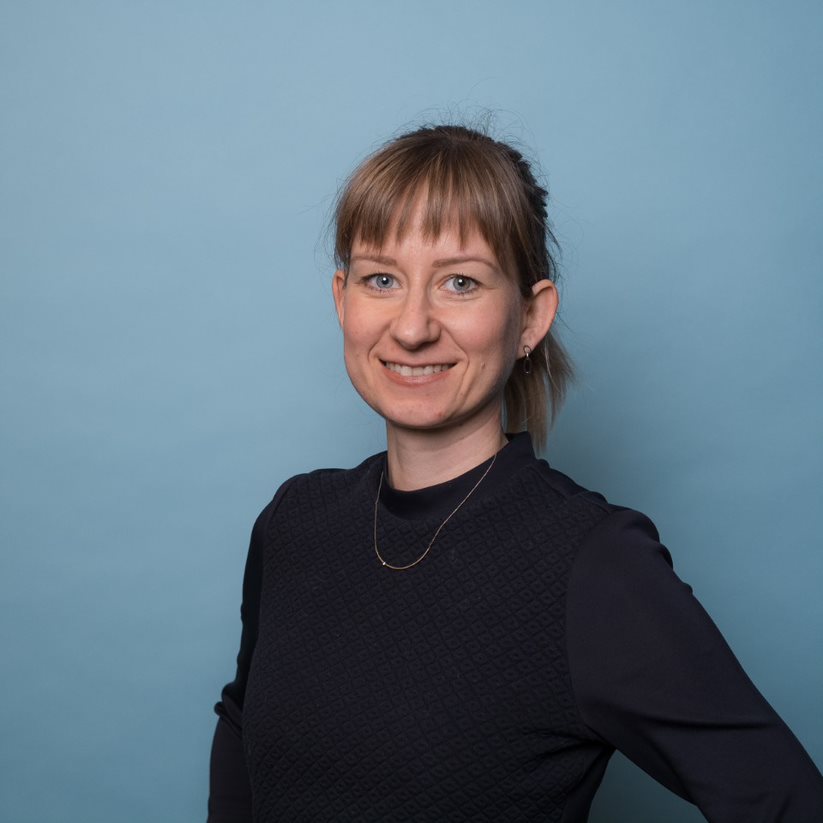
Opening Airport Technology Lab
- Tuesday, 26 May 2020
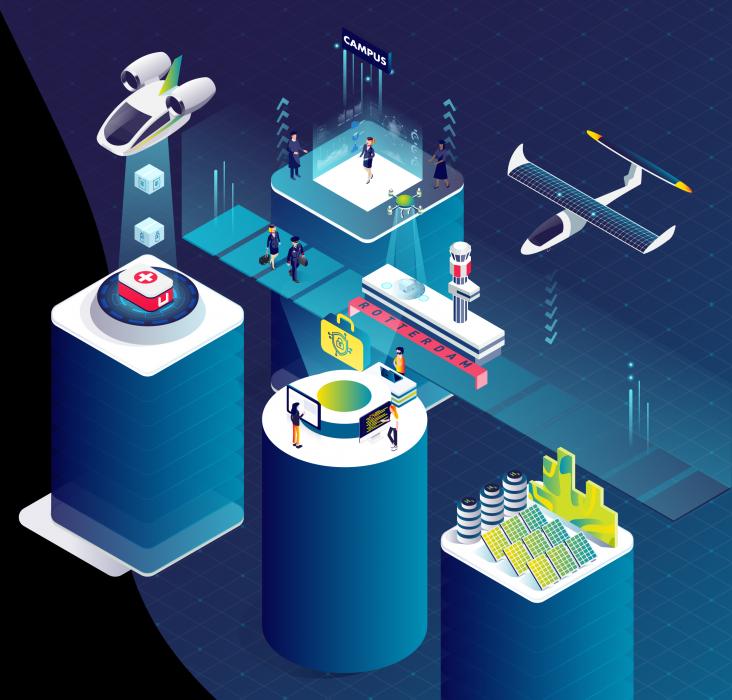
This Friday, May 29 at 15:30, the opening of the Airport Technology Lab will be done by Ron Louwerse (Director of the Rotterdam The Hague Airport), Prof. dr. ir. Tim van der Hagen (President of the Board and Rector Magnificus of TU Delft) and Henk Jan Gerzee (Chief Digital Officer of the Royal Schiphol Group).
The Microwave Sensing, Signals, and Systems section (Department of Microelectronics, EEMCS faculty), chaired by Professor DSc. Alexander Yarovoy, participates in the Laboratory and is going to use their professional expertise and advanced radar facilities to develop modern remote sensing techniques to extend the sensing capability of airport radars for constant weather monitoring. This real-time monitoring with high spatial and temporal resolution is aiming to improve air traffic planning (departing/arriving on time, less fuel, less noise, less pollution, etc.) and safety.
The Airport Technology Lab (ATL) is an organization for the development, test, and demonstration of the innovative products and services for airports. The digitization of everything that happens at airports and the application of Artificial Intelligence is increasing exponentially. With all that data and the right IT platform, companies can create and test new services and products. The most successful innovations will help to provide passengers with more comfort and convenience, make the airliners maintain smoother and more efficient flights, and the handlers will optimize their processes. Ultimately, the airport also contributes (extra) to its social responsibility to reduce air pollution, CO2 emissions and noise pollution.

New project: ADACORSA
- Tuesday, 25 February 2020
Autonomous drone flight, beyond visual line of sight, safe and reliable
More ...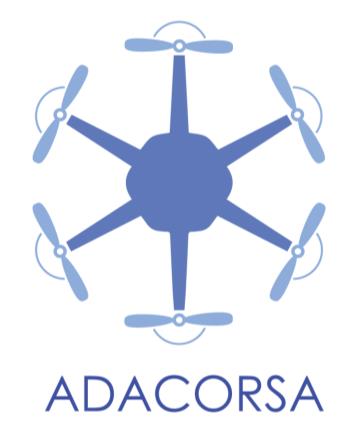
Wouter Serdijn appointed theme leader of Delft Health Initiative 2.0's NeuroTech theme
- Wednesday, 4 September 2019
The Delft Health Initiative has laid the foundation for connected health-oriented research at TU Delft and will continue to focus expertise, develop talent and to connect researchers to national and international initiatives. Wouter Serdijn will lead this for Neurotechnology.
More ...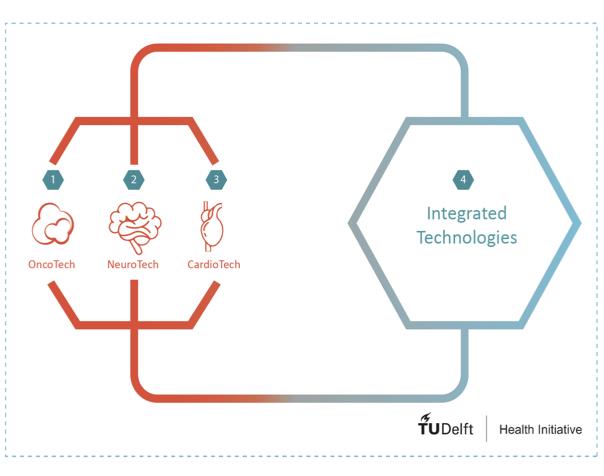
NWA Idea Generator grant for Richard Hendriks
- Tuesday, 9 July 2019
Restored sound localization for hearing impaired people
Dr. Ir. R.C. HendriksThe inability of hearing impaired people to localize sound has a big impact on their well-being and self- reliance. Compared to normal-hearing people, hearing-impaired people cannot efficiently use the same localization information. In this project will be investigated whether inaudible localization information can be transformed into a different audible form.
With a sum of 50,000 euros each, NWO granted 37 out-of-the-box research ideas with the potential to make an impact in society.
The applicants receive funding from the Idea Generator programme of the Dutch National Research Agenda (NWA). A total of 1.85 million euros was available.
More ...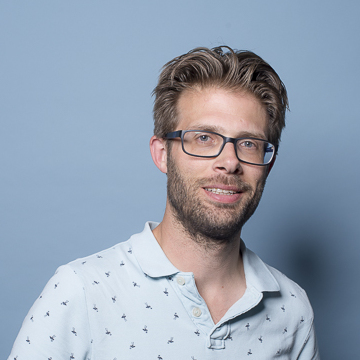
Congratulations for Miao
- Friday, 31 May 2019
Best presenter at the WIC/IEEE Benelux Symposium, Ghent
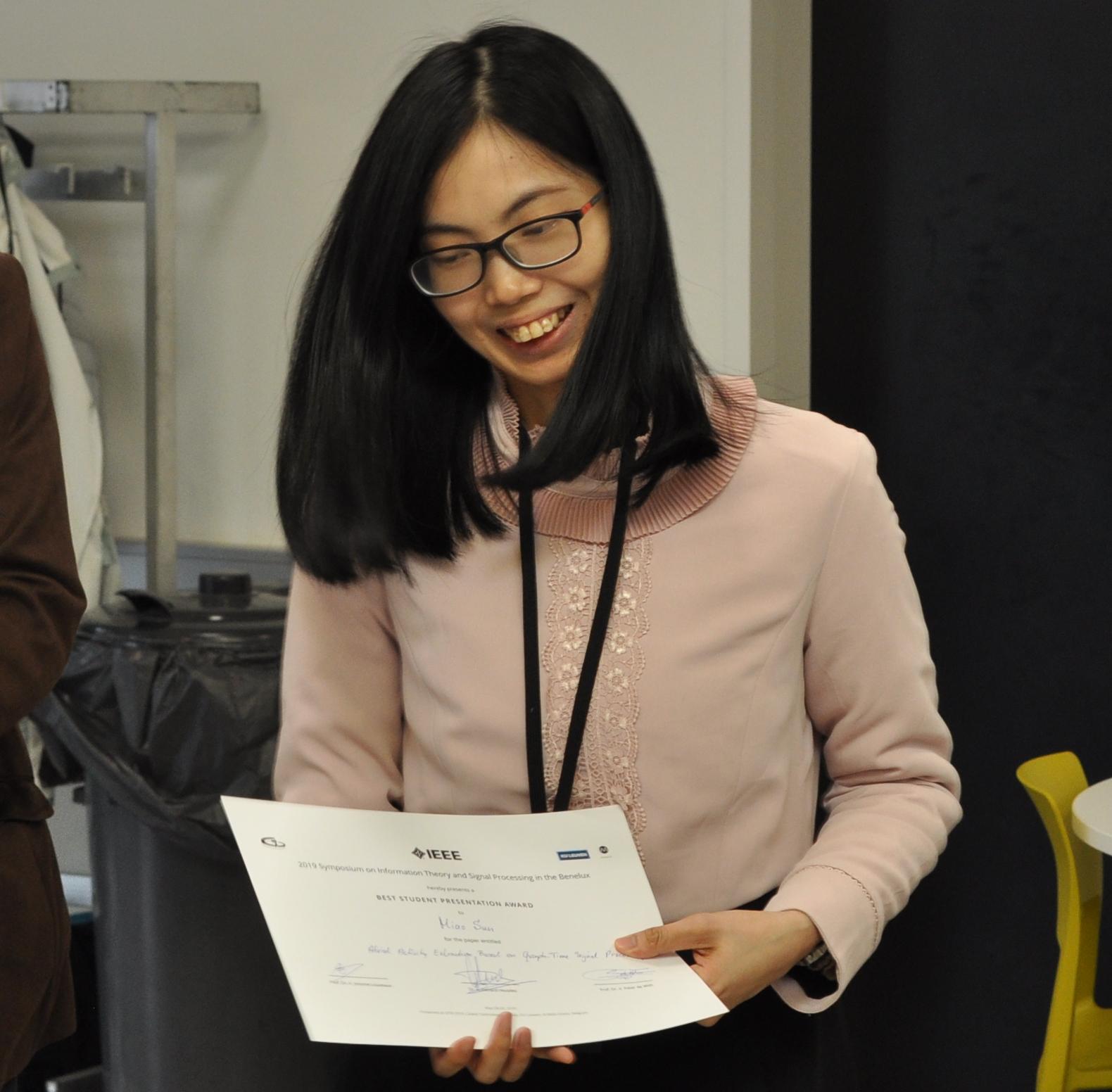
First Microelectronics Synergy Grants
- Friday, 22 February 2019
According to Professor Geert Leus who heads the ME Research Committee, the Synergy Grants are also intended to kick-start the research of young faculty, as it can be quite challenging for them to obtain funding at the beginning of their research careers. The grants cover half the costs of a PhD candidate, with the rest coming from existing research funding. ‘The submitted proposals were carefully evaluated by the ME Research Committee on the basis of their scientific quality, their clarity and feasibility, the synergy between the participating sections, and the relationship to the departmental themes. The ME Management Team (MT) then decided to award Synergy Grants to the top three proposals.’
Changes
The aim of the grants is to encourage newly emerging combinations of technologies and to facilitate cross-overs between them, thus strengthening and broadening the department's research portfolio. This goal fits seamlessly within the research strategy of ME, which has defined itself around the four themes of Health & Wellbeing, XG, Safety & Security and Autonomous Systems to better address societal challenges.
Winners
Last week, the winners were received by the ME MT. They received flowers from the head of the department (Kofi Makinwa) and had the opportunity to briefly present their proposals to the assembled MT. Below are short descriptions of the successful proposals.
Akira Endo & Sten Vollebregt: ‘The aim of our project TANDEM: Terahertz Astronomy with Novel DiElectric Materials is to develop advanced dielectric materials to realize superconducting microstrip lines with very low losses in the frequency ranges of 2-10 GHz and 100-1000 GHz. The PhD candidate will combine the dielectric deposition, characterization, material expertise and facilities of the ECTM group and the Else Kooi Laboratory, and the submillimetre wave device measurement capability of the THz Sensing Group and SRON. The aim is not only to realize low loss dielectrics, but also to understand the underlying physics that governs these losses. If successful, these microstrips will be immediately applied to enhance the sensitivity of the DESHIMA spectrometer on the ASTE telescope in Chile.’
Bori Hunyadi: ‘On one hand, the vast complexity of the human brain (10^11 neurons and 10^14 connections) enables us to process large amounts of information in the fraction of a second. At the same time, imperfections of the wiring in this vast network cause devastating neurological and psychiatric conditions such as epilepsy or schizophrenia. Therefore, understanding brain function is one of the greatest and most important scientific challenges of our times. Brain function manifests as various physical phenomena (electrical or e.g. metabolic) at different spatial and temporal scales. Therefore, the PhD candidate working on this grant will develop a novel multimodal and multiresolution brain imaging paradigm combining EEG and a novel imaging technique, fUS. The specific engineering challenge is to understand and describe the fUS signal characteristics, deal with the large amount of data it records using efficient computational tools; and finally, formulate the specification of a dedicated non-invasive, multimodal, wearable EEG-fUS device.’
Virgilio Valente & Massimo Mastrangeli: ‘The seed money of the Synergy Grant will partially support a joint PhD candidate to investigate the tight integration of an heart-on-chip device with dedicated electronic instrumentation in the same platform. Our aim is to bring sensing and readout electronics as close as possible to a cardiac tissue cultivated within a dedicated micro physiological device. The grant helps promoting the logical convergence between current departmental research activities at ECTM and BE and within the Netherlands Organ-on-Chip Initiative (NOCI) on the development of instrumented organ-on-chip devices.’

New STW project: TOUCAN
- Friday, 15 February 2019
Develop cheap 3D ultrasound using compressive sensing
More ...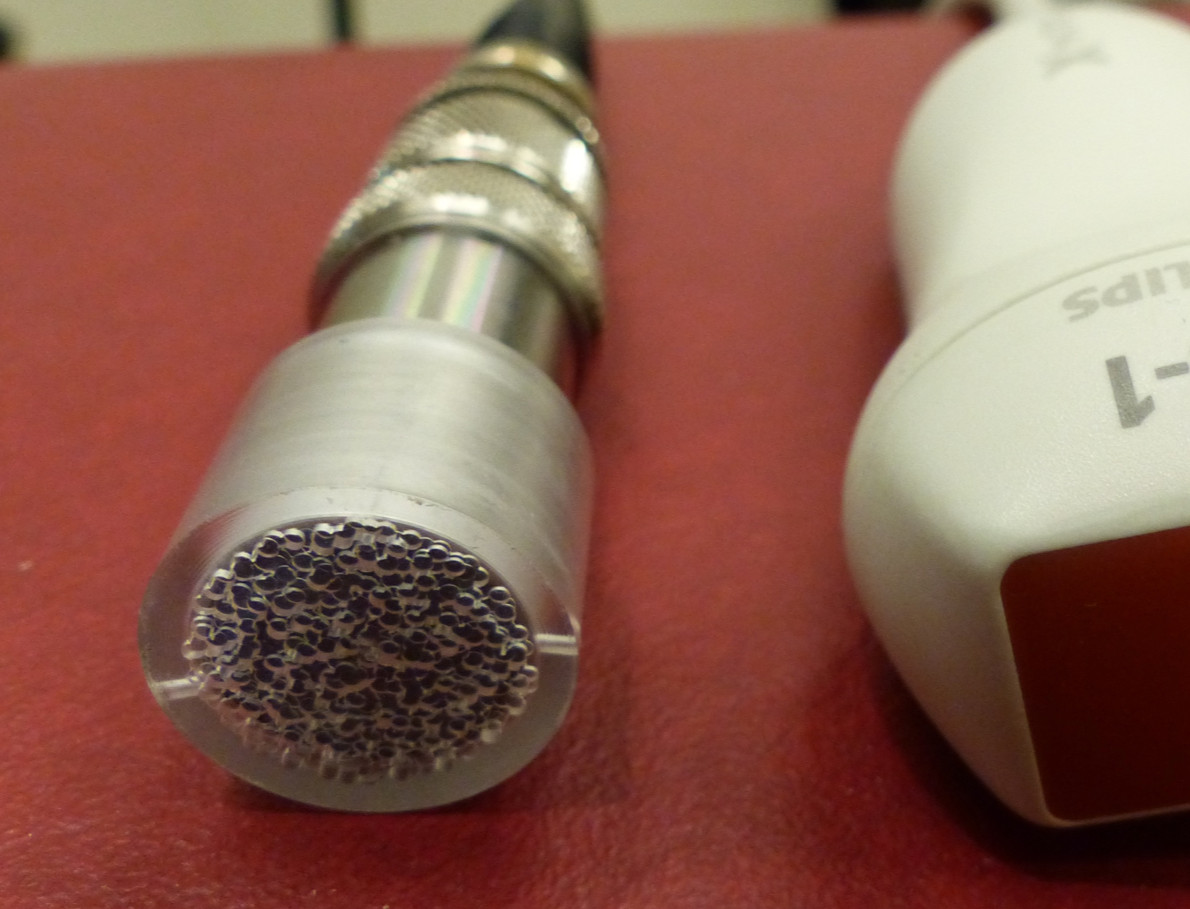
New postdoc: David Aledo
- Friday, 4 January 2019
CAS welcomes David Aledo as a new postdoc, working with Rene van Leuken on the Prystine project. David received his PhD from the Polytechnic University of Madrid (UPM), Spain.
More ...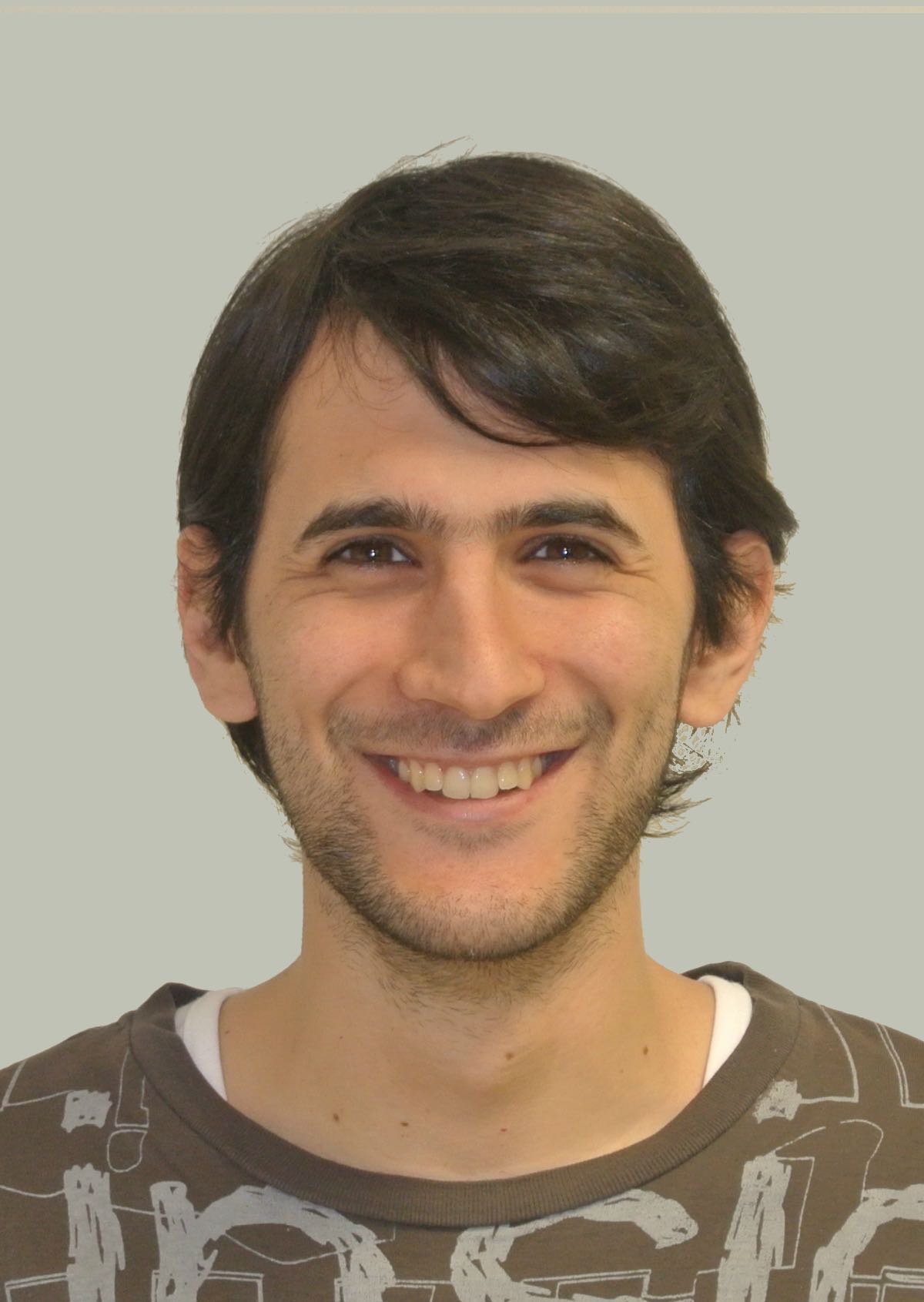
New project: Medical Delta Cardiac Arrhythmia Lab
- Thursday, 3 January 2019
with Erasmus MC; Medical Delta will fund 2 PhD students as part of a larger program aimed to unravel and target electropathology related to atrial arrhythmia
More ...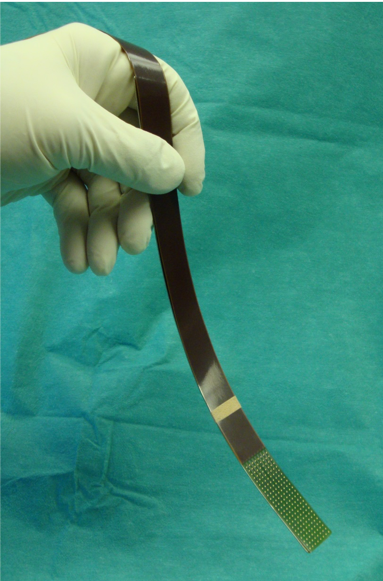
Image formation for future radio telescopes
- Monday, 24 December 2018
Radio astronomy is an interesting application area for array signal processing. We developed a new image formation tool called PRIFIRA, inspired by Sparse Bayesian Learning. Featured in ETV Maxwell 22.1.
More ...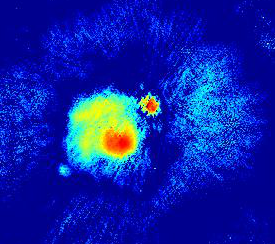
New Assistant Professor
- Wednesday, 3 October 2018
CAS welcomes Borbala Hunyadi, a new Assistant Professor, working on Bio Signal Processing
More ...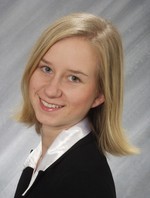
Guillermo Ortiz selected as "Best 2017/2018 Graduate of EEMCS"
- Friday, 21 September 2018
Guillermo Ortiz is selected as "Best 2017/2018 Graduate of EEMCS" by the Dean, and is nominated to compete for the Best Graduate of TU Delft (election on 6 November).
Guillermo did his thesis work on the topic of Graph Signal Processing, which was graded with a 10. Part of his work is already accepted for publication in the GlobalSIP 2018 conference, and has been submitted to an IEEE journal.
Congratulations!
More ...
Shahrzad Naghibzadeh EURASIP 3MT contest finalist
- Sunday, 9 September 2018
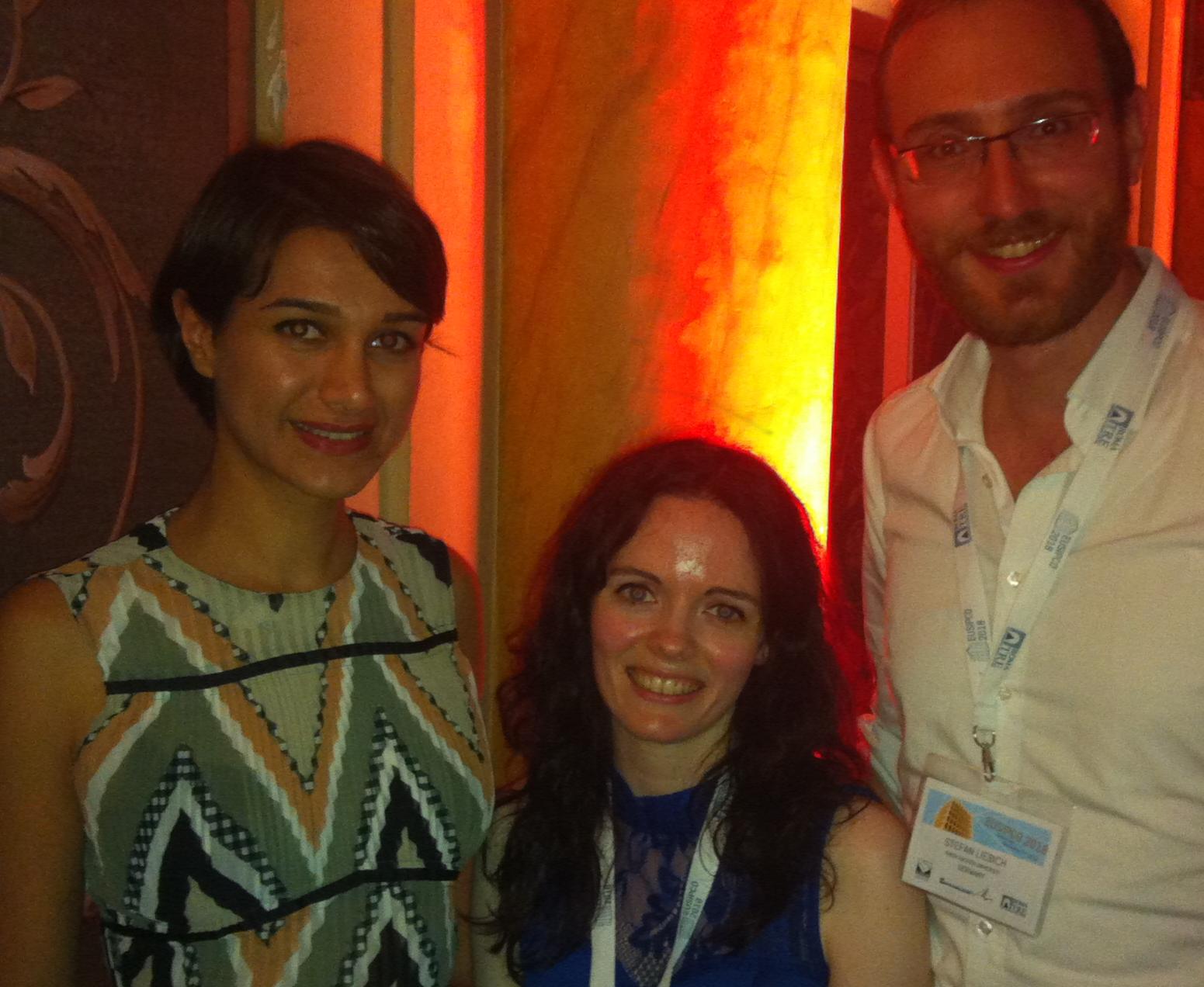
The 3MT (three minute thesis) contest is an international contest for students to explain their PhD thesis within 3 minutes. It is held across many universities and countries. In the EURASIP version, students in Signal Processing are invited to submit their 3-minute video, and the best ones are invited to present their work on stage during the EUSIPCO conference.
In 2018, Shahrzad Naghibzadeh was one of 10 selected students to present her work, in the conference auditorium. By ballot of the over 200 people in the audience, she ended up in the top-three (see picture). The final selection was done by an award committee; the #1 place went to Virginie Ollier.
Congratulations!
More ...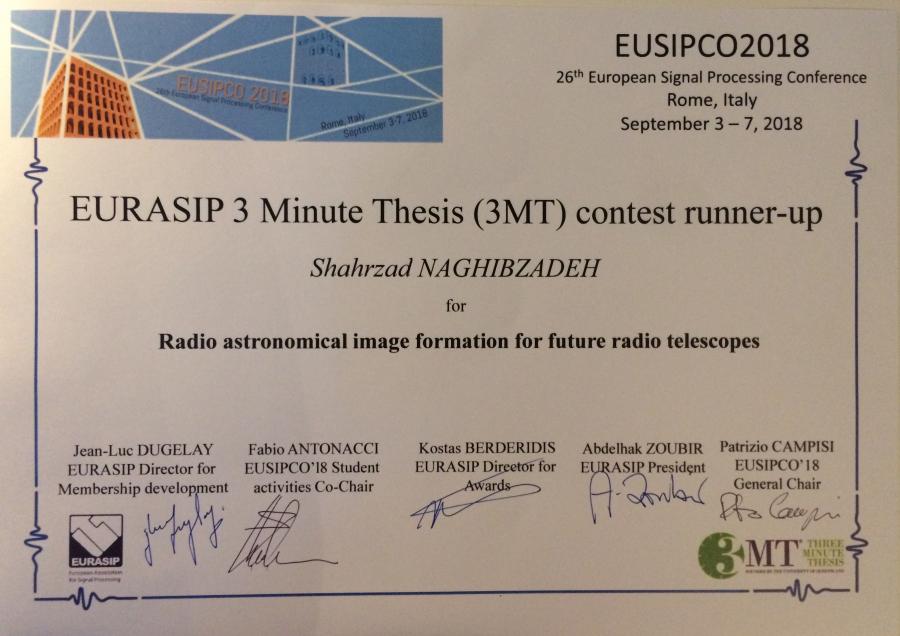
Geert Leus in IEEE ICASSP Top Downloads
- Wednesday, 15 August 2018
An 2015 ICASSP paper co-authored by Geert Leus made it to the Top-20 Downloads list of ICASSP papers over 2015-2017.
The paper is "Compressed Sensing Based Multiuser Millimeter-Wave Systems: How Many Measurements Are Needed?", by Ahmed Alkhateeb, Geert Leus, and Robert Heath. Last year, the related journal paper also won a best (young author) paper award.
The overview of top-downloaded papers was published in IEEE Signal Processing Magazine, July 2018.
More ...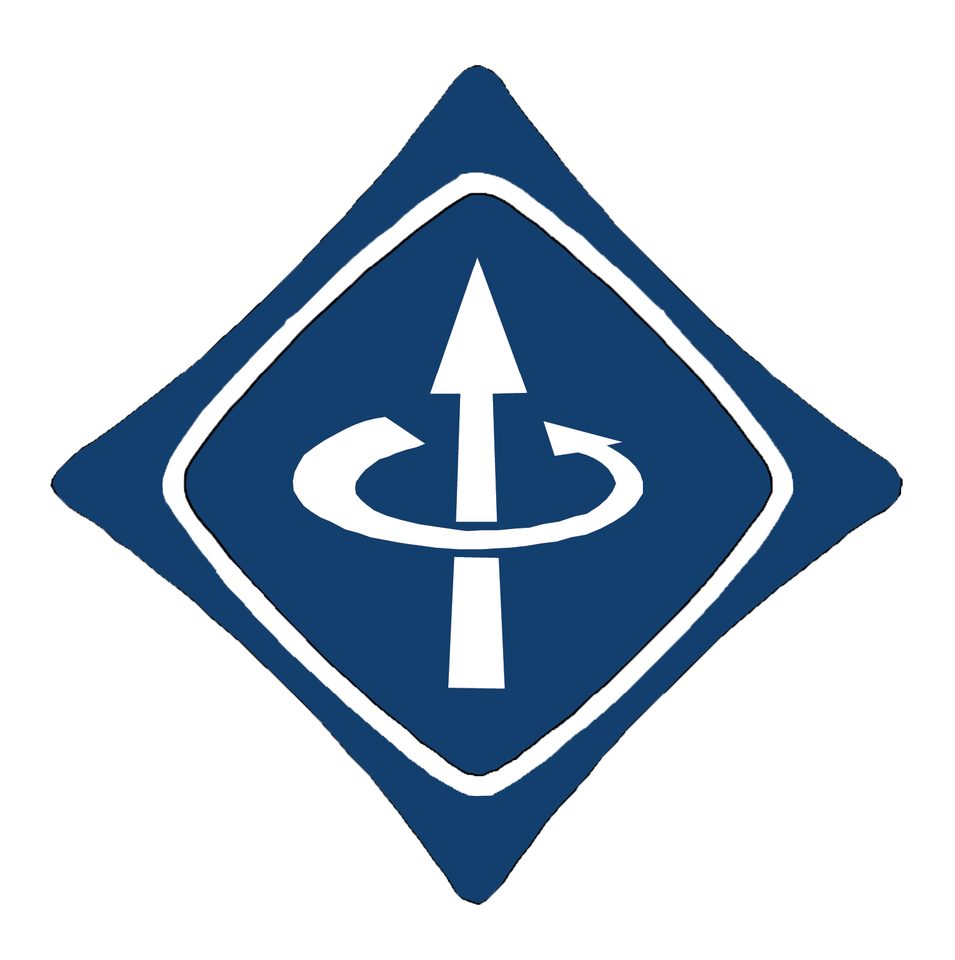
Jie Zhang wins best student paper award
- Thursday, 12 July 2018
On 10 July, Jie Zhang won one of the three best student paper awards of the IEEE Sensor Array Multichannel Signal Processing workshop (175 att.), for the paper "RATE-DISTRIBUTED BINAURAL LCMV BEAMFORMING FOR ASSISTIVE HEARING IN WIRELESS ACOUSTIC SENSOR NETWORKS", coauthored with Richard Hendriks and Richard Heusdens. Congratulations!
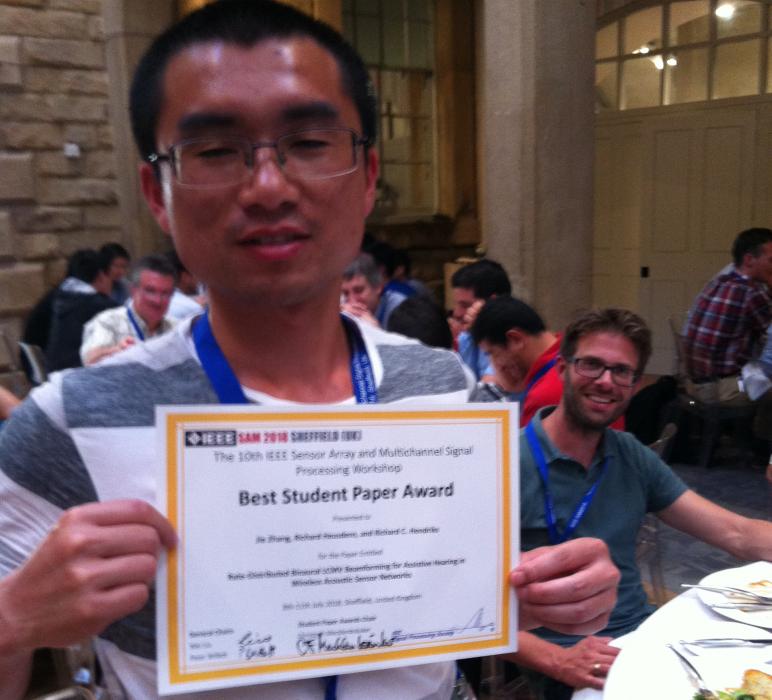
Best paper award for Aydin
- Friday, 8 June 2018
Aydin Rajabzadeh was selected as winner of the best student paper presentations at SPIE's Photonics Europe International Symposia held 22-26 April 2018 in Strasbourg, France, with the paper "A computationally efficient approximation of the transfer matrix model for analysis of FBG reflected spectra". Congratulations!
More ...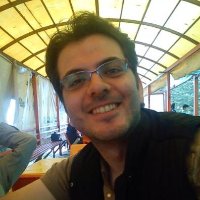
Leiden Marathon
- Monday, 28 May 2018
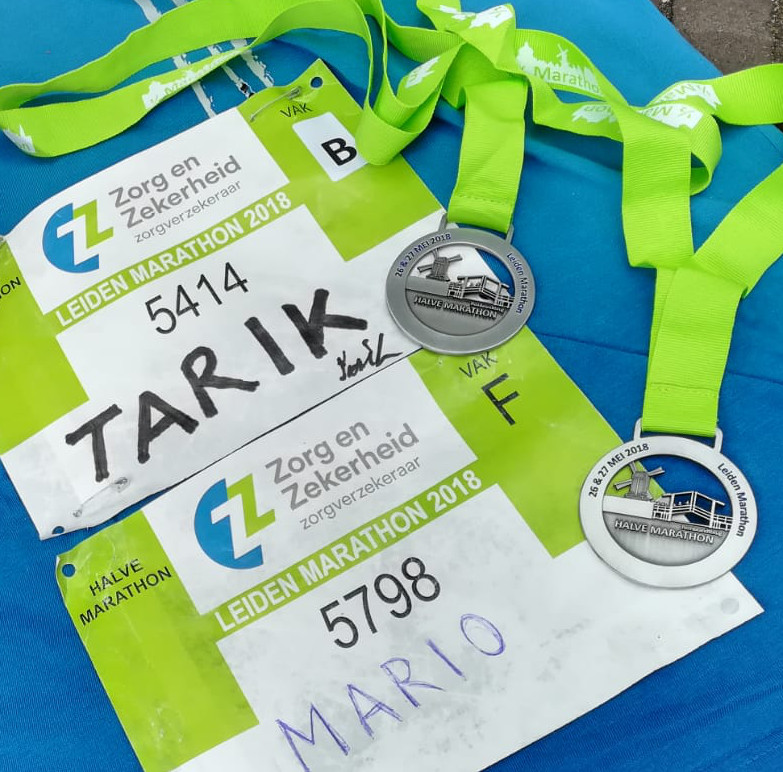
This weekend, Mario and Tarik ran the half-marathon of Leiden, and Tuomas even the full one!
(This is the event where several people had to be hospitalized because of the heath...)
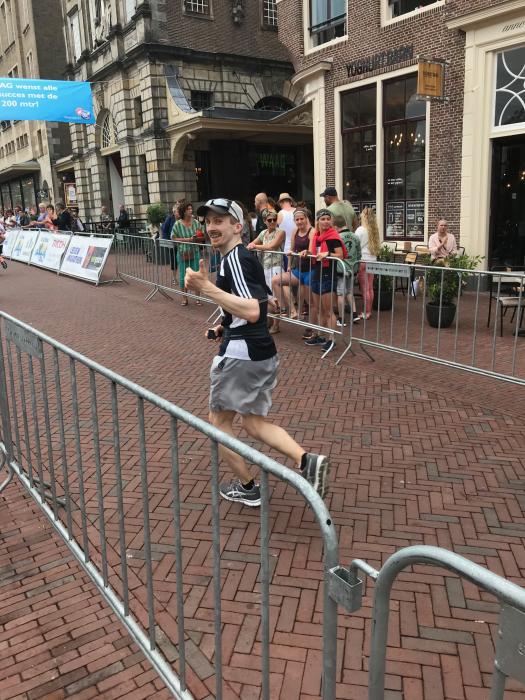
CAS on the run
- Monday, 12 March 2018
Success for Tarik, Mario, Thom and Elvin after the (half) marathon of The Hague on 11 Mar 2018.
More ...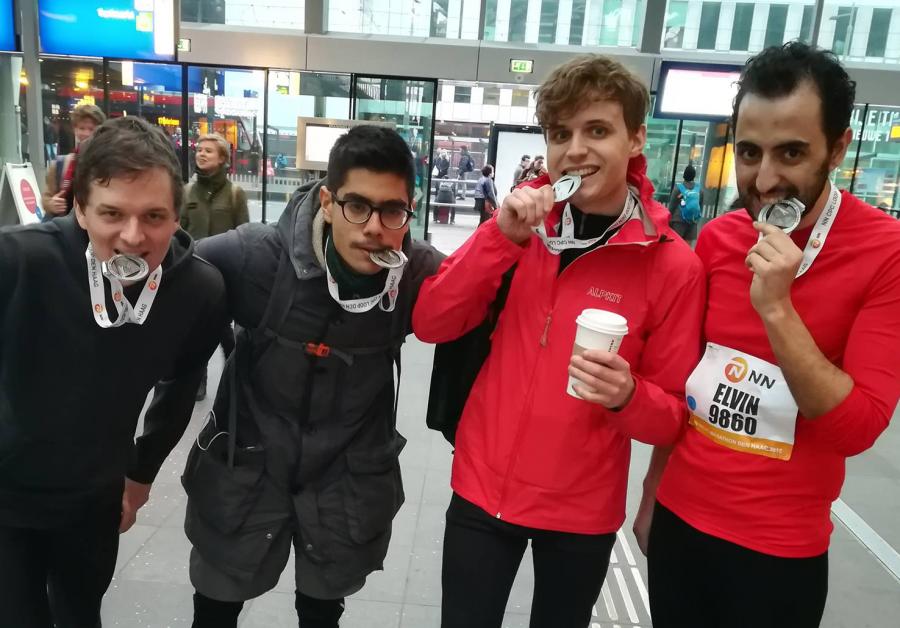
URSI Benelux Forum-2018 poster awards
- Friday, 26 January 2018
The Annual Benelux URSI meeting took place on January 25 in Delft. Around 20 posters were presented. Sharzad and Patrick won the first and second Student Poster Award.
Shahrzad Naghibzadeh proposed "PRIFIRA - General regularization using priorconditioning for fast radio interferometric imaging". while Patrick Fuchs presented "Local Maxwell two-dimensional first order electrical properties tomography".
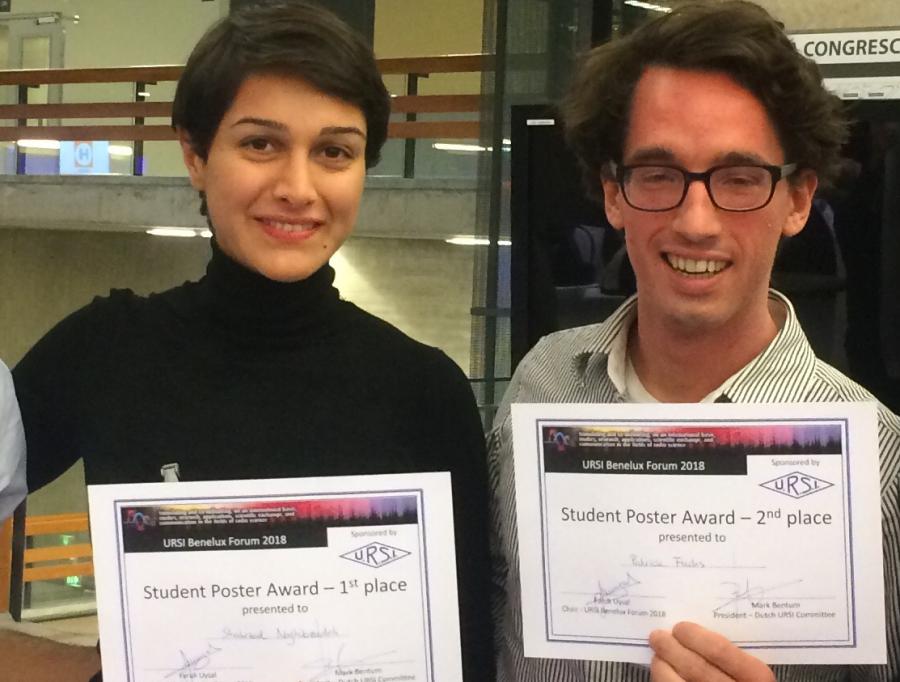
New project: PRYSTINE
- Monday, 22 January 2018
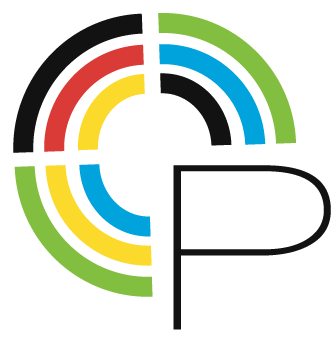
Rene van Leuken, together with his team (Sumeet Kumar, Amir Zjajo), and Said Hamdioui at CE acquired part of a new EU project "PRYSTINE". The aim is to design programmable compute hardware for automatic driving functions, across two application targets: data fusion for robust perception; and acceleration of AI frameworks for decision making. The emphasis is on low-power compute platforms.
While the overall budget of the project is 50 ME and spans over 60 partners, Delft will sign up for 1.8 ME.
More ...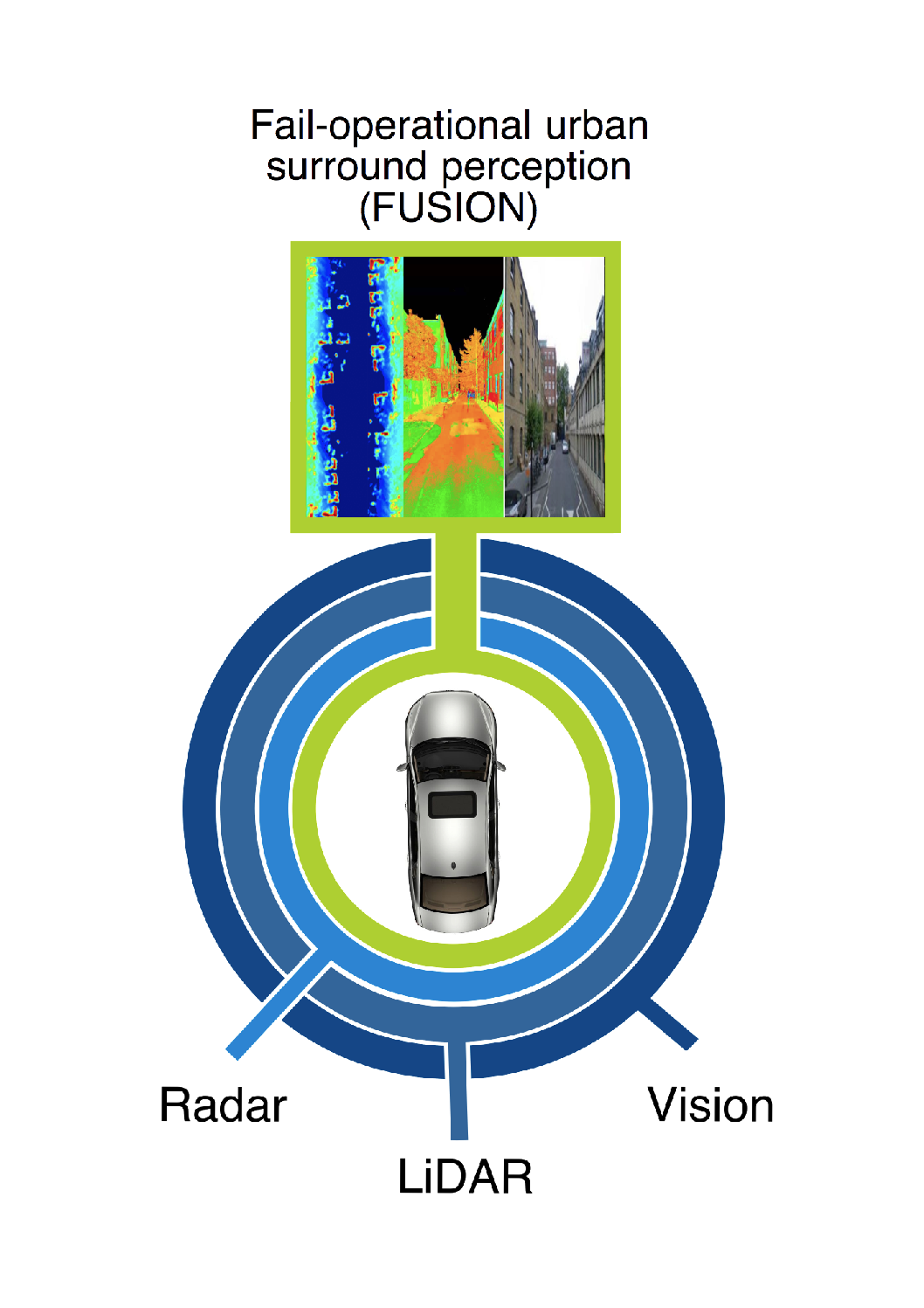
Geert Leus Editor-in-Chief of Elsevier Signal Processing
- Friday, 22 December 2017
Starting 1 January 2018, Geert is the new Editor-in-Chief of Elsevier Signal Processing (IF: 3.1)
More ...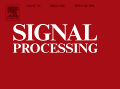
Best student paper award for Mario Coutino and Elvin Isufi
- Monday, 11 December 2017
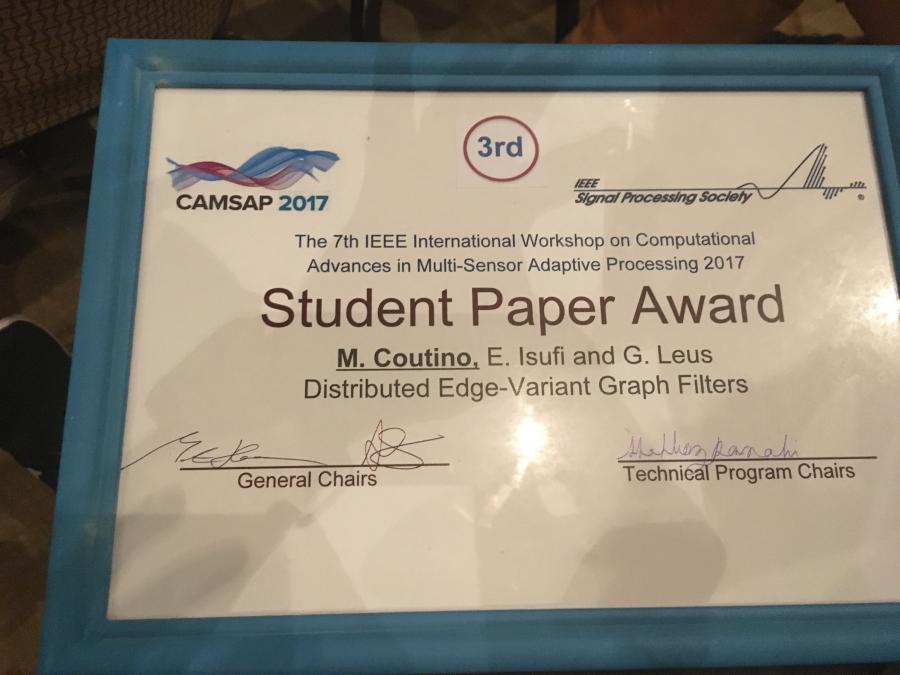
Mario and Elvin win the Student Paper Award contest (3rd place) at CAMSAP’17 for their paper on "Distributed Edge-Variant Graph Filters".
More ...
3D Ultrasound using a single transducer element
- Saturday, 9 December 2017
Pim van der Meulen, Geert Leus and our collaborators at Erasmus MC show how this is possible using a phase mask and compressed sensing,
More ...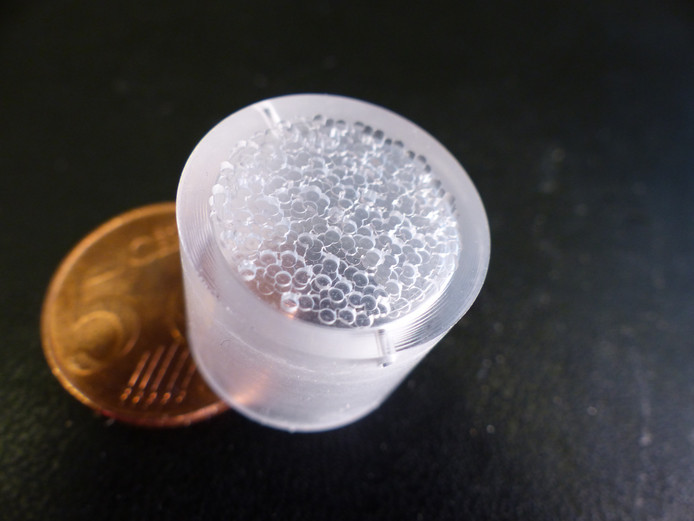
Tadashi and Geert won best paper award
- Monday, 23 October 2017
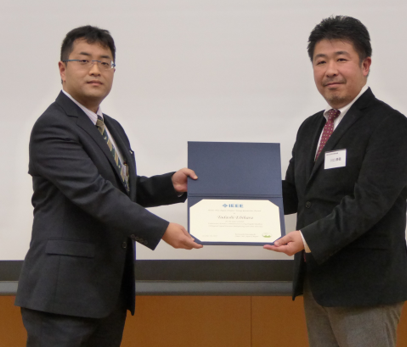
The IEEE/OES Japan Chapter Young Researcher Award was won by Tadashi Ebihara for the paper "Doppler-Resilient Orthogonal Signal-Division Multiplexing for Underwater Acoustic Communication", T. Ebihara; G. Leus; IEEE Journal of Oceanic Engineering, Volume 41, Issue 2, pp. 408-427, 2016.
Tahashi was a 3-month visitor at CAS in 2013 when he worked on this paper with Geert.
More ...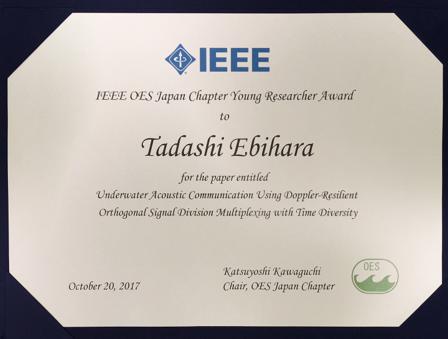
TU Delft "Female Fellowship" Tenure Track Academic Positions
- Monday, 16 October 2017
All academic levels; apply before Jan 8, 2018.
More ...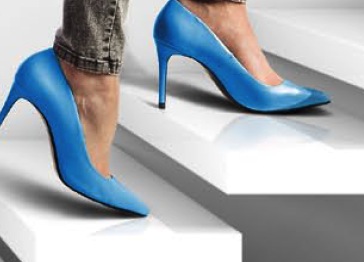
Geert Leus appointed IEEE SPS Distinguished Lecturer
- Monday, 4 September 2017
Geert Leus has been selected to serve as an IEEE Signal Processing Society Distinguished Lecturer for the term 1 January 2018 through 31 December 2019. This is considered a prestigious sign of recognition.
More ...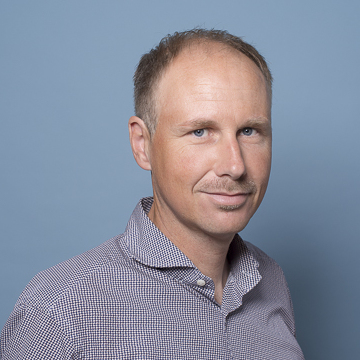
Best Student Presentation Award for Jamal Amini
- Tuesday, 30 May 2017
At the 2017 Symposium on Information Theory and Signal Processing (Delft, 11-12 May), organized by the IEEE Benelux Chapter, Jamal Amini received a best student presentation award. Congratulations!
More ...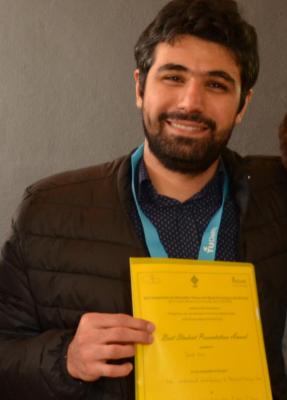
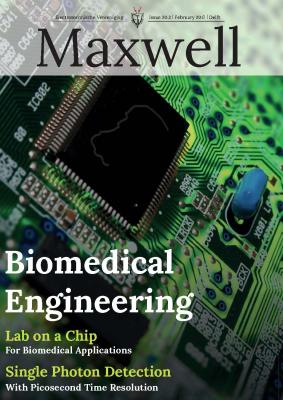
Rob Remis appointed Associate Professor
- Thursday, 26 January 2017
Per 1 January, Rob Remis has been promoted by the Dean to the rank of UHD (Associate Professor). Congratulations!
More ...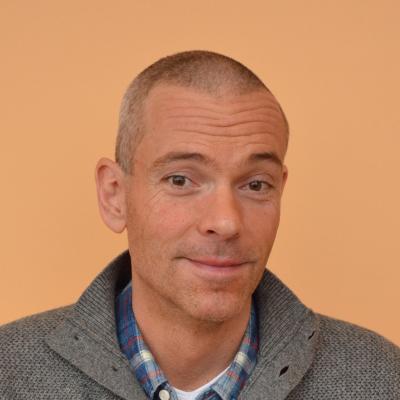
Two IEEE SPS Best Paper Awards
- Monday, 12 December 2016
Selected for the 2016 IEEE Signal Processing Society Best Paper Award:
Cees H. Taal, Richard C. Hendriks, Richard Heusdens, and Jesper Jensen
“An Algorithm for Intelligibility Prediction of Time–Frequency Weighted Noisy Speech”
IEEE Transactions on Audio, Speech, and Language Processing, Volume
19, No. 7, September 2011
Selected for
the 2016 IEEE Signal Processing Society Young Author Best Paper Award:
Ahmed Alkhateeb, Omar El Ayach, Geert Leus and Robert W. Heath, Jr.
“Channel Estimation and Hybrid Precoding for Millimeter Wave Cellular
Systems”
IEEE Journal of Selected Topics in Signal Processing, Volume 8, No. 5,
October 2014.
The awards will be presented at the Awards Ceremony at ICASSP 2017 in New Orleans, LA
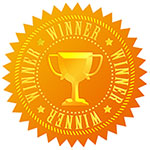
Rob Remis wins STW Open Mind 2016 award
- Monday, 28 November 2016
At their annual congres, STW awarded 5 grants (each 50 kE) to research teams to enable them to explore 'risky research' ideas. Martin van Gijzen, Andrew Webb and Rob Remis presented one of the winning proposals: an affordable MRI instrument based on permanent magnets (as opposed to superconducting magnets) for detecting hydrocephalus.
Short movie presenting the idea.
More ...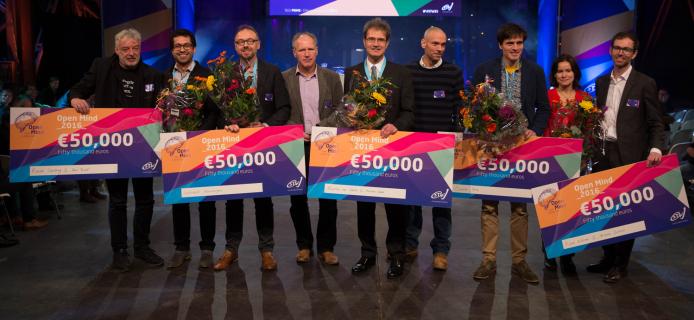
Vacancy: Team manager for Electrical Engineering Education (EEE)
- Thursday, 6 October 2016
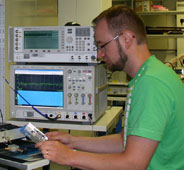
The Faculty of EEMCS is creating a special team to fully focus on teaching using our unique and innovative ‘Delft method’. This method integrates practical and theoretical electrical engineering education and trains students to be hands-on, theoretically versed electrical engineers ready for a future career in science or industry.
We are looking for a team manager specialising in Electrical Engineering Education (EEE) who will be both a group leader and a teacher in his/her capacity as the role model of EE Education.
More information
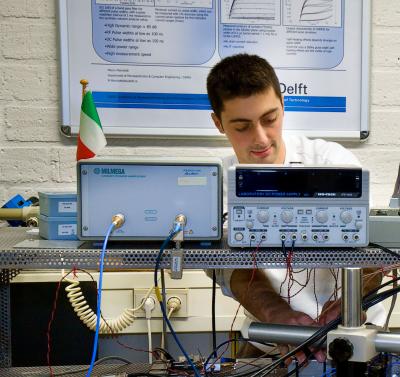
Geert Leus named 2016 EURASIP Fellow
- Tuesday, 6 September 2016
To recognize outstanding achievements in the broad field of Signal Processing, each year the European Association for Signal Processing (EURASIP) elevates a select group of up to maximum four signal processing researchers to "EURASIP Fellow", the Association's most prestigious honor.
The EURASIP Board of Directors (BoD) has awarded prof. Geert Leus as one of the 2016 Fellows, "for contributions to signal processing for communications".
The award consists of a certificate presented during the Opening and Awards Ceremony at EUSIPCO 2016, held in Budapest (Hungary) on August 30, 2016.
More ...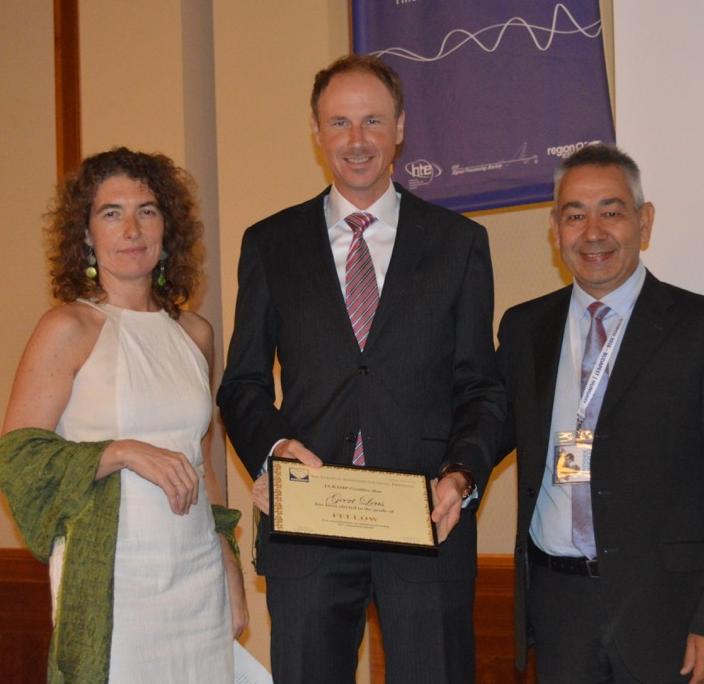
Best paper award for Geert Leus
- Monday, 18 July 2016
IEEE Sensor Array and Multichannel Signal Processing Workshop, Brazil ("Stationary Graph Processes: Nonparametric Spectral Estimation" with Antonio Marques en Alejandro Ribeiro)
7 July 2016: Opening of CryoLab for Extremely Sensitive Electronic Measurements
- Friday, 8 July 2016
.jpg)
The CryoLab of TU Delft's Faculty of EEMCS has been opened on Thursday 7 July by the dean Rob Fastenau. TU Delft scientists from the Tera-Hertz Sensing Group, Jochem Baselmans and Akira Endo, will be leading a team of young scientists and engineers working in the lab on astronomical instrumentation. The first instrument, DESHIMA (Delft SRON High-redshift Mapper), is being developed to be operated on the ASTE telescope in the Atacama Desert in Chile. The goal of the research is to create 3D charts of so-called submillimetre galaxies that, in contrast to 2D charts, also show distance and time.
The large number of superconducting detectors, and the advanced electronics developed at SRON, allows DESHIMA to map a very large volume of space at once. While Endo leads the development of DESHIMA, Baselmans will soon install the next cryostat for testing novel THz array antennas, that will enable his upcoming instrument MOSAIC to target multiple galaxies at once. In the future, the CryoLab is envisioned to also host new coolers from QuTech. Superconducting electronics used for astronomical instrumentation and quantum electronics have much in common, because they both push the limits of what can be observed.
.jpg)
Rob Remis elected best teacher at EWI
- Friday, 10 June 2016
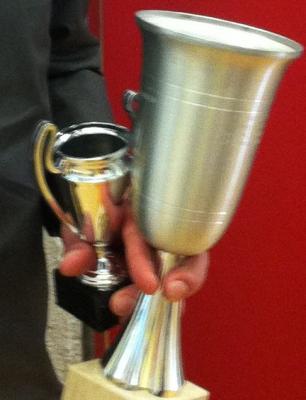
By student election (1700 votes), Rob Remis was elected as best teacher for Fac. EWI in 2016. A decade ago, Rob won already once the title 'Best teacher in EE'. This has now been extended to comprise the full faculty (EE, Mathematics, Computer Science). Later this year, Rob will compete for the title of 'Best teacher of TU Delft'.
The annual election is organised by the student associations of the Faculty (ETV, Christiaan Huygens), based on voting and written motivations.
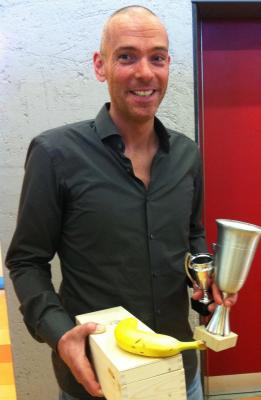
New project "tASk-cognizant sParse sensing for InfeREnce" approved
- Tuesday, 24 May 2016
STW project by Geert Leus
More ...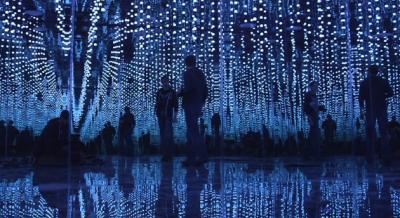
New project "Earlier recognition of cardiovascular diseases" approved
- Wednesday, 20 April 2016
Atrial fibrillation (AF) is a progressive disease and associated with severe complications such as stroke. Early treatment of AF is of paramount importance as it inhibits disease progression from the treatable (recurrent intermittent) to the untreatable (permanent) stage of AF. However, early treatment is seriously hampered by lack of accurate diagnostic instruments to recognize patients who will develop new onset AF or progress to a severer form of the disease.
The goal of this project is to develop age and gender based, bio-electrical diagnostic tests, the invasive and non-invasive AF Fingerprint, which consists of electrical atrial signal profiles and levels of atrial specific tissue/blood biomarkers. In daily clinical practice, this novel diagnostic instrument can be used for early recognition or progression of AF by determination of stage of the electropathology. As such, AF Fingerprinting enables optimal AF treatment, thereby improving patient?s outcome.
The project is a collaboration between Erasmus University (Dept. Cardiology), VU Medical Center (Dept. Physiology), and TU Delft (Sections CAS and Bioelectronics), and will fund 4 PhD students.
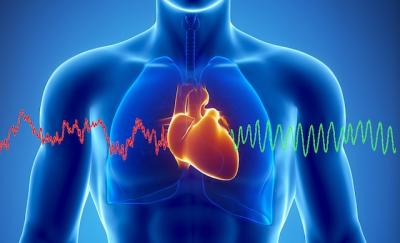
New book by Amir Zjajo: Brain-Machine Interface
- Friday, 11 March 2016
low-power analog front-end circuits for brain signal conditioning and quantization and digital back-end circuits for signal detection
More ...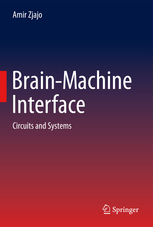
Michel Antolovic granted PicoQuant Young Investor Award
- Thursday, 3 March 2016
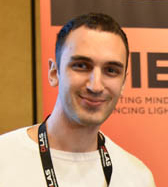
On February 14, 2016, Michel Antolovic was granted the prestigious PicoQuant Young Investigator Award at Photonics West in San Francisco for his paper titled 'Analyzing blinking effects in super resolution localization microscopy with single-photon SPAD imagers�. The paper shows the first localization super resolution images obtained with a SPAD camera. The analysis includes specific timing properties of fluorescing molecules in vitro with unprecedented accuracy thanks to one of the world�s single-photon fastest cameras that was created in the AQUA laboratory. The timing properties are aimed to be used for optimizing fluorophore blinking or separation of fluorophores, enabling multichannel super resolved imaging.
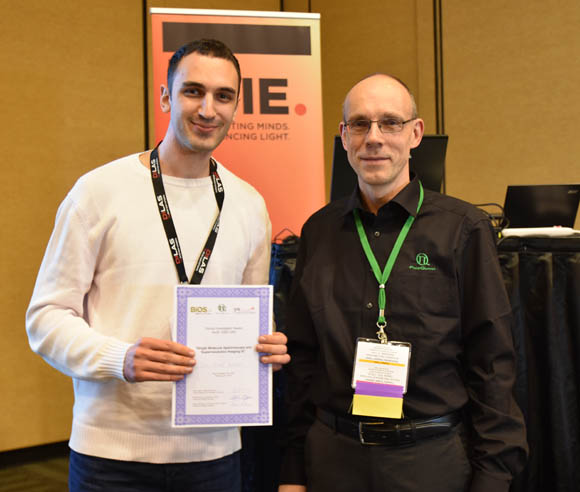
Sundeep's PhD Defense
- Thursday, 28 January 2016
On 25 January, Sundeep Chepuri defended his PhD thesis on "sparse sensing". Here are some pictures
More ...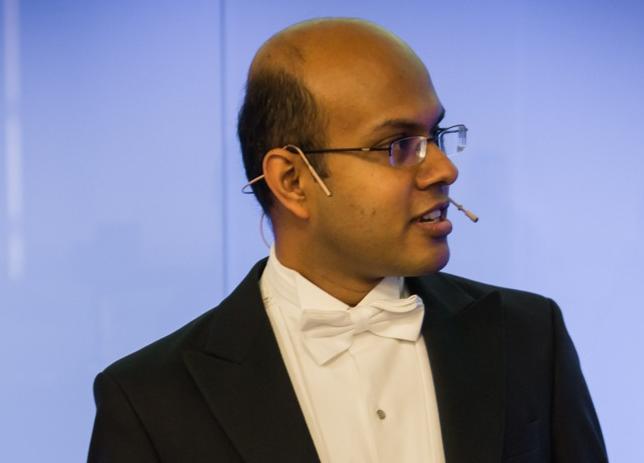
Happy 2016!
- Thursday, 14 January 2016
Here are some pictures of the New Year Reception of the Microelectronics department
More ...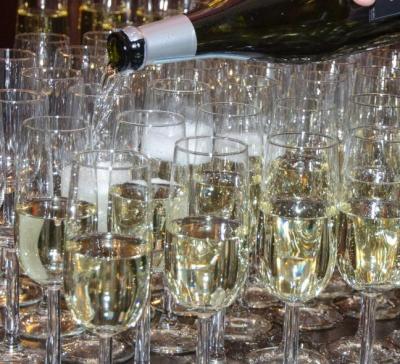
TU Delft Female Fellowship Tenure Track Openings
- Friday, 9 October 2015
Academic openings at all professor levels
More ...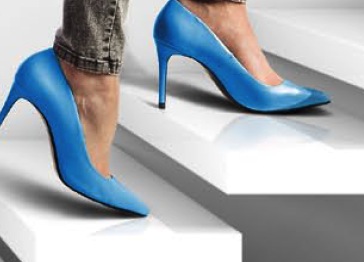
QuTech enters in collaboration with Intel
- Thursday, 3 September 2015
Intel and QuTech, the quantum institute of TU Delft and TNO, have finalised plans for a ten-year intensive collaboration, along with financial support for QuTech totalling approximately $50 million.
More ...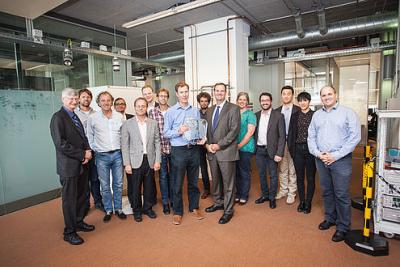
New STW project: "Good vibrations"
- Thursday, 9 July 2015
Today STW announced that Rob's proposal "Good Vibrations" in the Open Technology Program will receive funding. The project will utilize the power of so-called Krylov subspace reduction techniques and develop solution methodologies for wave field problems in complex media.
The project will fund 1 PhD student: Jorn Zimmerling.
More ...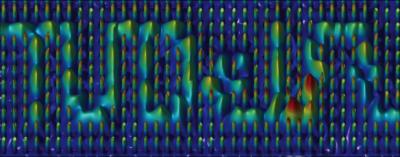
CAS Outing a big success.
- Saturday, 4 July 2015
The CAS outing this year focused on socializing, team building and having a great time and it was a big success. The group went to Outdoor Valley in Bergschenhoek for a bit of serious fun. Thanks all for participating and a big hug for the organizers.
More ...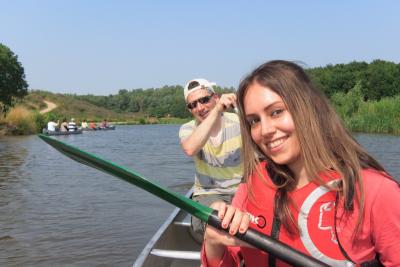
Sundeep Chepuri wins ICASSP Best Student Paper Award
- Tuesday, 21 April 2015
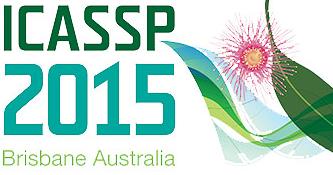
The ICASSP paper "SPARSE SENSING FOR DISTRIBUTED GAUSSIAN DETECTION" by Sundeep Chepuri and Geert Leus won the best student paper award. This is quite a prestegious achievement. Congratulations!
More ...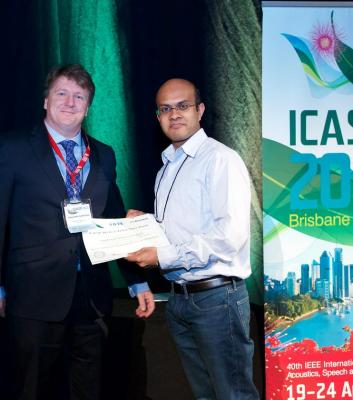
Else Kooi Award ceremony at ICT Open
- Wednesday, 25 March 2015
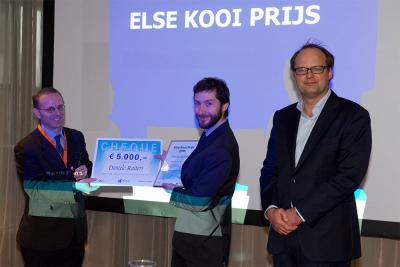
The 2015 Else Kooi Award has been granted to Dr Daniele Raiteri for his scientific research on Technology-Aware Circuit Design for Smart Sensors on Plastic Foils. The Else Kooi Award is an annual award for young researchers in the field of applied semiconductor research conducted in the Netherlands. The award comes with a prize of 5,000 euros.
Raiteri has received the award during a special ceremony at the ICT.OPEN symposium on March 25th. The award was presented by the board of the Else Kooi Award foundation professor Nauta, chair of the foundation (TU Twente) and professor Edoardo Charbon. Edoardo Charbon from the microelectronics department of the EEMCS faculty holds the position of secretary of the Else Kooi Award Foundation.
Dr Raiteri?s research is focused on organic semiconductors. This emerging technology has specific features which severely complicate the design of circuits and systems, such as low transconductance, gain and speed, as well as high component variability. Dr Raiteri has devised several new solutions that have shown to be extremely robust to variability, achieving significantly better gain-bandwidth products in amplifiers and exceptional signal-to-noise ratios in voltage-controlled oscillators.
Photos by: Thijs ter Hart
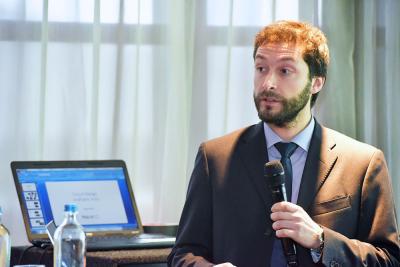
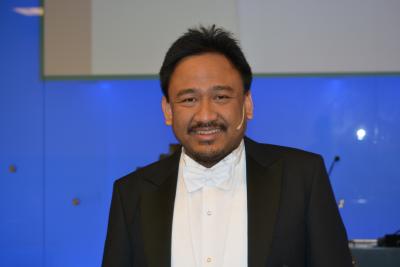
New STW project: "SuperGPS"
- Tuesday, 17 February 2015
Gerard Janssen acquired, with his colleagues Jeroen Koelemeij (VU Amsterdam, PI) and Christian Tiberius (CiTG), a new STW project called SuperGPS.
The project addresses the problem that currently, GPS is not sufficiently accurate and reliable to enable autonomous driving. The central question is: "?How do we realize highly accurate and reliable positioning using extremely accurate time-frequency reference signals, distributed through hybrid optical-wireless networks??.
The project aims at a hybrid optical-wireless system for accurate positioning, navigation, and network synchronization, to complement or even replace satellite navigation technology. This system is accomplished through a terrestrial grid of radio antenna ?pseudolites?, synchronized with extreme accuracy through the fiber-optic telecommunications network. The key deliverable of the project is a pilot demonstration of SuperGPS technology under real-life circumstances.
The technology will be developed with support and feedback from potential users in telecommunications (Royal KPN N.V.), mobility (TNO and Volvo), and Dutch high-tech manufacturers, as well as stakeholders from the scientific and R&D community, including the Dutch metrology institute VSL, the Dutch ?keepers of atomic time? UTC, and physicists and astronomers in need of better time and frequency signals.
More ...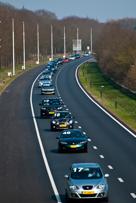
Best paper award for Amir, Carlo and Rene
- Monday, 9 February 2015
Amir Zjajo, Carlo Galuzzi and Rene van Leuken won the Best Paper Award for the paper "Noise Analysis of Programmable Gain Analog to Digital Converter for Integrated Neural Implant Front End" at the International Conference on Biomedical Electronics and Devices (Biodevices 2015; Rome, Italy).
More ...New STW project "From coil to antenna"
- Sunday, 21 December 2014
The STW project "From coil to antenna: development of innovative transmit array elements for MRI of the body at 7 Tesla" has been granted funding. Rob Remis is one of the applicants. The PI is Prof.dr. A.G. Webb (Leids Universitair Medisch Centrum), also Univ. Utrecht is involved.
More ...L3SPAD honored
- Thursday, 18 December 2014
The STW HTSM "L3SPAD: A Single-Photon, Time-Resolved Image Sensor for Low-Light-Level Vision" program has received funding. The program is led by Edoardo Charbon.
Description
Low-light-level (LLL) image sensors have been receiving great attention because they have various applications ranging from fluorescence microscopy to automotive sensing, from safety monitoring to 3D vision for robots. Traditionally, however, LLL image sensors have been used for military purposes because of their prohibitive costs. The appearance of monolithic solid-state complementary metal-oxide-semiconductor (CMOS) processes for the design and fabrication of photon counting image sensors has paved the way to enable low-cost and high-performance LLL image sensors. In this project, we will realize a gated 1.3Mpixel photon-counting image sensor in a standard CMOS process. The target sensor, with high timing resolution, low noise, and high photon detection efficiency, is the perfect candidate to meet all these technical and cost specifications.?More ...Best MSc student of TU Delft
- Thursday, 13 November 2014
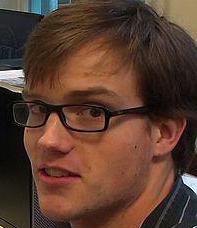
This afternoon, Jorn Zimmerling won the competition for best MSc student of TU Delft of this year. Jorn was an MSc student of Rob Remis and Paul Urbach, and is now a PhD student with Rob at CAS.
TU Delft news article (in dutch)
More ...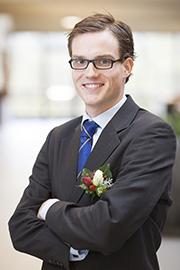
QuTech appointed as 'national icon'
- Friday, 7 November 2014
The Ministery of Economic Affairs has named 4 innovations as 'national icon'; QuTech is one of them. "National icons are innovations which generate future welfare and help to solve mondial problems." The icons will receive a national support podium, including a minister or secretary of state as ambassador.
In the Department of Microelectronics, prof. Edoardo Charbon and dr. Ryochi Isihara are 2 of the 5 EWI faculty members directly involved in QuTech.
More ...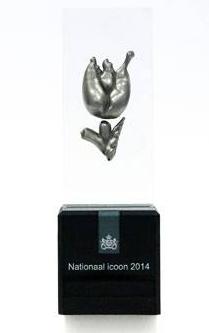
MP Jan Vos visits PARSAX
- Friday, 7 November 2014
On Friday 7 November, Jan Vos, MP for the PvdA, visited the TU Delft Climate Institute. The theme of the visit was climate change, TU Delft's research and the usefulness of and need for climate monitoring. The programme included a demonstration of cloud simulations in the Virtual Lab and a visit to the PARSAX radar. Thanks to the rain, it was possible to obtain good live measurements.
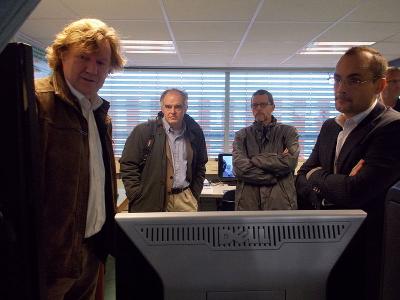
Board of Directors of EURASIP
- Thursday, 4 September 2014
On Sep 1, Alle-Jan was elected as incoming member of the Board of Directors of EURASIP, with a 4-year term starting 1 January 2015. EURASIP is the European Signal Processing association.
More ...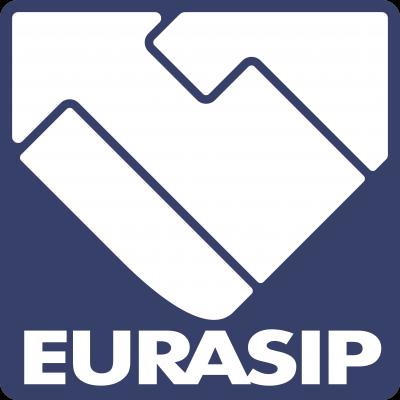
Stefan Wijnholds finalist for Christiaan Huygensprijs 2014
- Friday, 27 June 2014
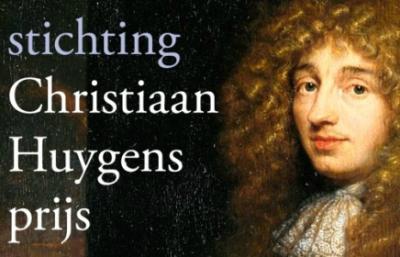
Yesterday, 25 June 2014, Stefan Wijnholds received an "honorable mention" as finalist for the Christiaan Huygensprijs 2014, rewarding the best PhD thesis work in ICT over the past 4 years. The awards were handed by the Minister of Education (dr. Jet Bussemaker).
After a tough preselection by each university, a list of 32 candidates at a national level were judged by the jury. Out of these, 4 finalists were nominated who received a certificate in a ceremony in Voorburg.
Stefan received the honor for his PhD thesis on calibration and imaging for the LOFAR radio telescope. He was a PhD student with Alle-Jan van der Veen in 2006-2010, while being employed by ASTRON in Dwingeloo.
More ...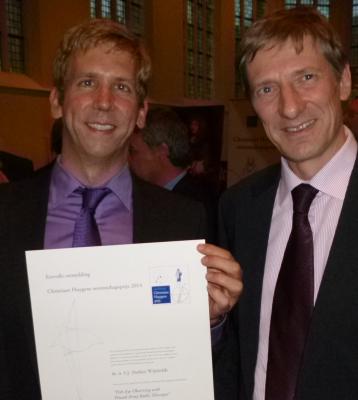
New STW project for Rob Remis
- Tuesday, 20 May 2014
Rob Remis was granted an STW project "Dielectric enhanced MRI". The main applicant of this project is Andrew Webb (Leiden Univ.), coapplicants are Rob Remis (CAS) and Bert-Jan Kooij (MS3). This will fund 2 PhD students in Delft.
The project aims to improve MRI imaging by inserting "bags" with dielectric materials between the magnets and the body. This should provide better illumination, in particular when using high-tesla fields. This has already been applied in practice but the effect is theoretically poorly understood. The project should provide the EM theory related to this case.
More ...Millad's SAM'14 paper in final selection for award
- Monday, 5 May 2014
Millad's paper "Application of Krylov based methods in Calibration for Radio Astronomy" has made it to the final round of the IEEE Sensors and Multichannel (SAM) 2014 student paper competition. This selection has been made based on ranking by the TPC members. The final poster competition is Sunday, June 22, 2014 in A Coru?a.
More ...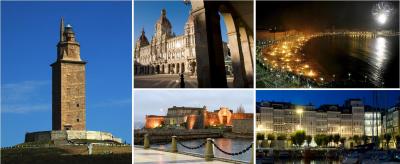
New project: DRIFT
- Friday, 2 May 2014
The future SKA radio telescope will produce large amounts of correlation data that cannot be stored and needs to be processed quasi real-time. Image formation is the main bottleneck and requires order 350 peta-flops using current algorithms. Another bottleneck is the transportation of station data (samples) to the central location where they are correlated.
The project aims to reduce the transportation bottleneck by time-domain compressive sampling techniques, allowing the recovery of full correlation data from significantly subsampled antenna signals, and to introduce advanced algebraic techniques to speed up the image formation. Ideally, we would even skip the intermediate covariance reconstruction.
The project is funded by NWO in the "Big Bang, Big Data" program and is carried out in context of the ASTRON-IBM DOME project.
More ...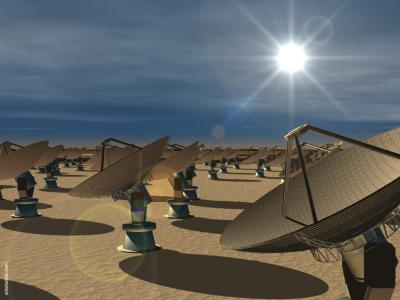
Prof. Bastiaan Kleijn in Delft for 2 months
- Thursday, 1 May 2014
Professor Bastiaan Kleijn is a part-time professor in the CAS group. He will be physically present in the period 1 May-1 July 2014.
His expertise is speech and audio signal processing. He will be collaborating with Richard Heusdens and Richard Hendriks.
More ...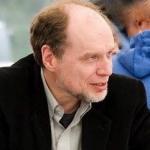
New project on improved hearing aids
- Thursday, 1 May 2014
Richard Hendriks has acquired a new STW project aimed at improving the intelligibility of speech for users of hearing aids.
With a prevalence of about 11 %, severe hearing loss has become a serious problem in our society. While the current generation of hearing aids can be of a great help in certain situations, they generally are not able to provide the hearing-aid user a natural impression of the acoustical scene. An often-reported problem for hearing impaired people is the inability to understand speech in complex acoustical environments as well as the inability to localize sound.
Due to the development of wireless technology, it is possible to equip hearing aids with more powerful noise reduction algorithms to further increase the intelligibility. However, these more powerful multichannel noise reduction algorithms sacrifice naturalness of the sound environment, also when state-of-the-art binaural noise reduction algorithms are used.
This project aims at developing signal processing algorithms to help hearing aid users in these situations, by providing them a natural impression of the acoustical scene.
More ...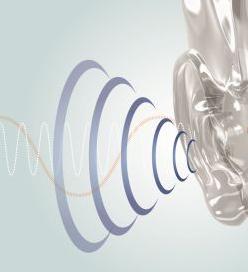
European Conf Antennas Propagation
- Thursday, 10 April 2014
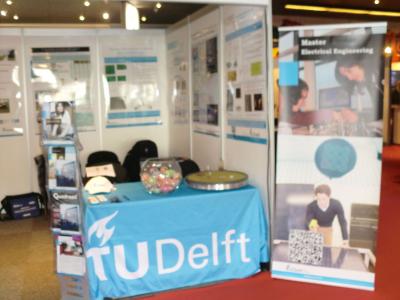
TU Delft is platinum sponser and exhibitor at the EuCAP 2014 - The 8th European Conference on Antennas and Propagation, to be held at the World Forum in The Hague, The Netherlands, on 7 to 11 April 2014.
The Microelectronics (ME) department from the faculty of Electrical Engineering, Mathematics and Computer Science, includes research groups actively engaged on teaching and research in the field of antennas and propagation.
Located within the microelectronics department, the mission of the THz Sensing Group is to introduce breakthrough antenna technology that will revolutionize THz Sensing for Space based and Earth based applications. In the long term the research will enable multi Tera-bit wireless communications.
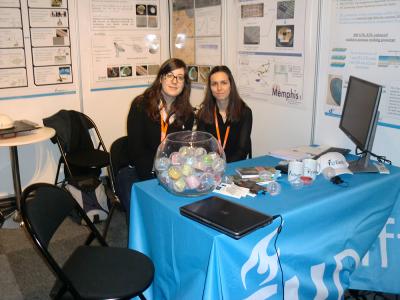
Alle-Jan van der Veen appointed EURASIP Fellow
- Saturday, 5 April 2014
The award is for contributions to array signal processing applied to communications and radio astronomy. In 2014, four researchers have been recognized as Fellow.
More ...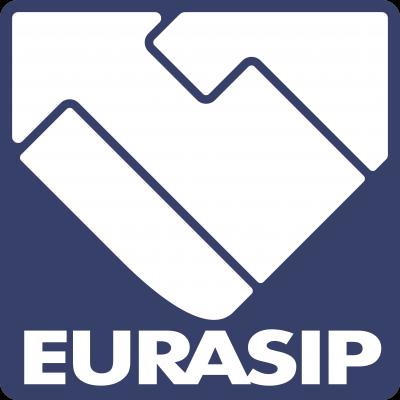
New CAS website
- Saturday, 5 April 2014
Today, CAS switched to a new website. Please enjoy, and let us know if something is not working right (contact: Alle-Jan van der Veen). CAS members can apply for a user account to maintain their own bio-page.
More ...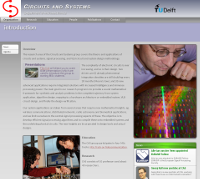
Georg Kail new postdoc at CAS
- Tuesday, 4 March 2014
Georg Kail is a new postdoc at CAS, working with Geert Leus on distributed localization
More ...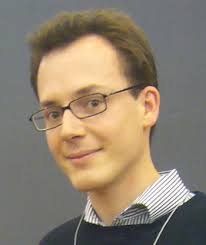
Przemek Pawelczak new Assistant Professor
- Friday, 17 January 2014
In July 2012, Przemek Pawelczak was awarded a VENI research grant from NWO. This grant (EUR 250k) allows the researcher to fund his own research for up to 3 years. The topic of the research is "Intelligent spectrum use in emergency networks", and it will explore statistical methods to guarantee quality of communication in Cognitive Radio Emergency Networks.
Following this, Przemek was appointed as Assistant Professor in the Embedded Software group and started in January 2013.
More ...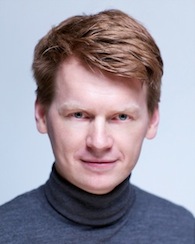
A new professor
- Tuesday, 14 January 2014
On 26 Sept 2012, the Board of Directors of TU Delft has decided to appoint Geert Leus as Antoni van Leeuwenhoek Professor in the CAS group. This is a `personal' full professorship aimed to promote young, excellent academics to Professor at an early age so that they can develop their academic careers to the fullest possible extent
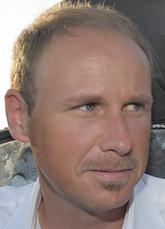
Agenda
MSc SPS Thesis presentation
- Monday, 26 May 2025
- 13:00-15:00
- HB17.140 (Seminar room)
DoA Estimation for Arrays with Phase Incoherencies
Shipeng LiuNXP Semiconductors
Automotive radar is an important sensor technology for self-driving cars and Advanced Driver-Assistance Systems (ADAS). Current automotive radars lack the ability to classify and categorize objects due to their limited angular resolution. A new generation of automotive radar systems, known as automotive imaging radars, proposes to overcome this limitation by using larger apertures with more antenna elements. During the radar operation, automotive imaging radar systems face challenges in the accurate estimation of the Direction-of-Arrival (DoA) due to phase incoherency in the spatially sampled information caused by hardware imperfections, temperature variations, and aging effects. This work proposes a method combining convex optimization and alternating updates to first jointly calibrate the phase incoherencies and estimate the DoAs, and then update them iteratively. It further derives the Cramér-Rao Bound (CRB) and investigates the impact of the phase incoherency on DoA estimation using the CRB. In the proposed approach, the MUltiple SIgnal Classification (MUSIC) algorithm is applied to estimate the DoAs after each calibration. Additionally, the eigenvalue decomposition process in MUSIC is replaced by the Projection Approximation Subspace Tracking (PAST) algorithm to reduce computational complexity while maintaining the accuracy of DoA estimation. Experimental results illustrate the effectiveness of these techniques, highlighting their potential in improving next-generation automotive radar systems.
MSc SPS Thesis presentation
- Thursday, 22 May 2025
- 13:00-15:00
- HB17.140 (Seminar room)
Acoustic array processing with planar coded cover
Yuan yuan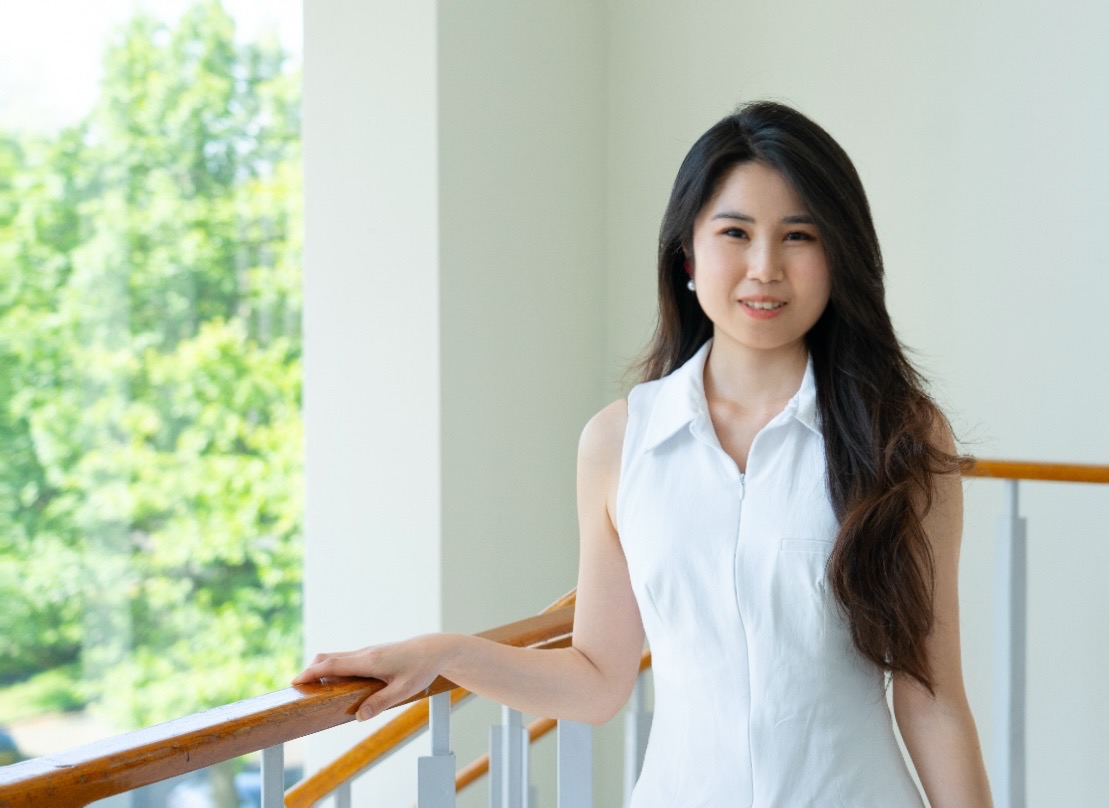
While the success of improving direction of arrival (DOA) estimation with linear coded covers using a single acoustic vector sensor (AVS) has been established, the extension of this theory to array-based systems remains unexplored. To address this gap, we employ a specially designed coded cover and leverage compressed sensing (CS) and compressed covariance sensing (CCS) methods, extending their application from single AVS systems to an array-based acoustic measurement system. Our results demonstrate that a 14×10 coded cover with 12 PU probes enables accurate localization of 100 sound sources in 3D, even at a signal to noise ratio (SNR) as low as 10 dB, showcasing the scalability and robustness of this approach.
To further enhance localization accuracy, we implement a self-calibration method in the covariance domain to correct phase and gain errors in each receiving channel. Additionally, we combine self-calibration with CCS to improve resolution and reduce side lobes. For geometric mismatch, we first investigate the sparsity-cognizant total least-squares (STLS) with multiple measurement vectors (MMV) variant of the fast iterative shrinkage-thresholding algorithm (FISTA) method. Then a grid-searching strategy is employed to compensate for these mismatches, ensuring better estimation accuracy. Experimental validation confirms that these techniques significantly enhance DOA estimation under non-ideal conditions, contributing to the advancement of acoustic sensing and localization methodologies.
ME colloquium
- Thursday, 20 March 2025
- 16:00-17:00
- EEMCS, lecture hall Chip
Bridging Communications, Sensing and Graphs in Signal Processing
Geert Leus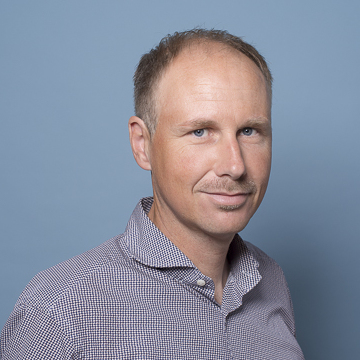
Prof. Leus will provide an overview of key research topics within the Signal Processing Systems group, spanning communications, sensing, and graph signal processing. In the field of communications, The work on a specific modulation format for underwater acoustic communications will be discussed. This type of signal modulation has been independently developed by different research groups under various names, including V-OFDM, OSDM, and OTFS. Regarding sensing, He will present our coded cover designs to boost the performance of acoustic vector sensors, which can also be applied to other types of sensors and sensor arrays. Additionally, he will share our latest findings on optimal array and waveform design for co-located multiple-input multiple-output (MIMO) radar. Interestingly, these designs deviate from conventional notions of optimality. Finally, prof. Leus will introduce the field of graph signal processing, highlight our pioneering contributions, and outline potential directions for future research.
Additional information ...
MSc SPS Thesis presentation
- Friday, 14 March 2025
- 11:00-13:00
- HB17.140 (Seminar room)
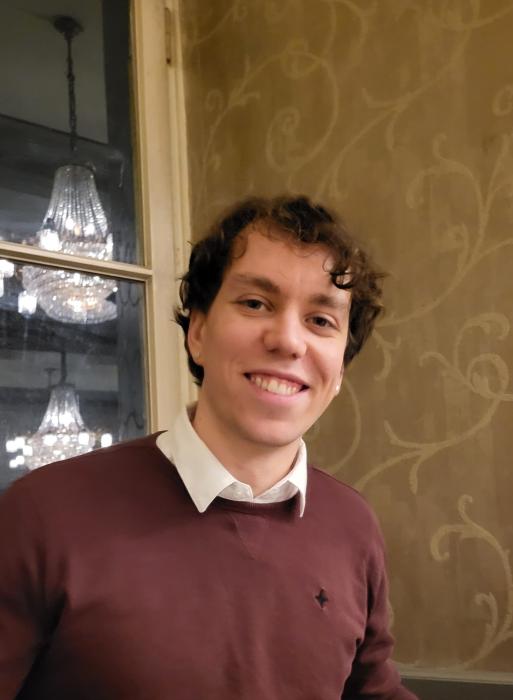
Microwave kinetic inductance detectors (MKIDs) are superconducting detectors that are excellent candidates for astronomy in the farinfrared (FIR), roughly 100 GHz to 10 THz. Radiation in this part of the electromagnetic spectrum is particularly hard to detect compared to optical or near-infrared radiation. Furthermore, some sources in the FIR are so faint that the detectors are required to detect single photons to determine the incident photon rate. Recent MKIDs are highly sensitve and are capable of detecting single photons in the FIR, although detection of lower energy photons remains a challenge. Photons produce pulses in the output signal of the detector. As the pulse height is dependent on the photon energy, low energy photons are hard to distinguish from the noise.
This thesis presents a system model that is used in estimating the photon rate. The system model describes signal relations and noise characteristics, so that it provides a foundation for developing statistical estimation and detection algorithms. Based on this model, various estimators are proposed, e.g. a generalized matched filter. This shows the utility of the system model in deriving solutions for estimation problems.
This thesis represents a first step in advancing signal processing techniques for single photon detection in MKIDs designed for FIR astronomy.
Additional information ...
EURASIP Webinar
- Wednesday, 12 March 2025
- 15:00-16:00
- (zoom link)
AI for applications in psychiatry
Justin Dauwels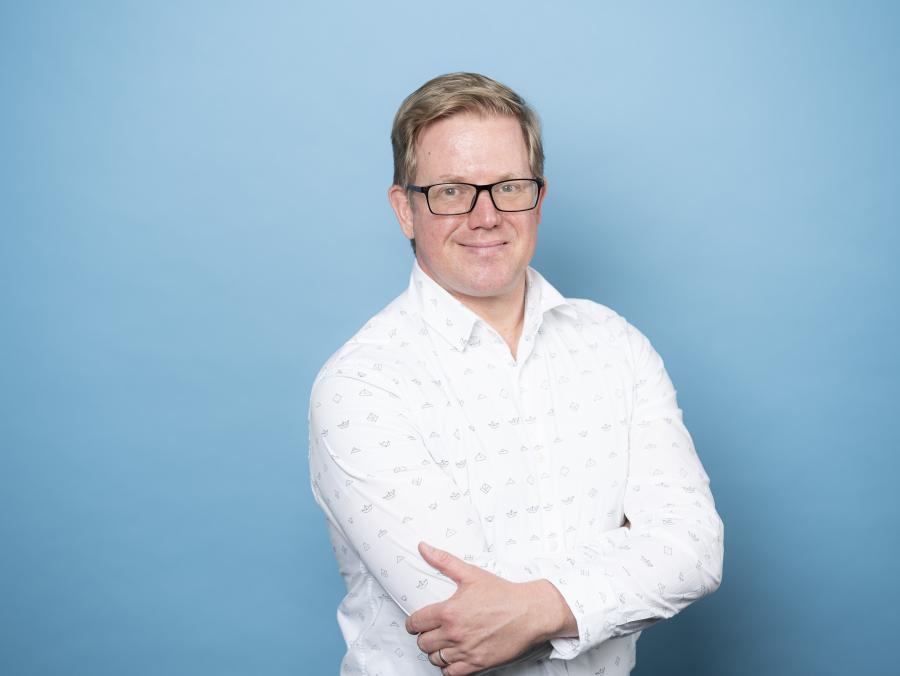
Abstract:
In this talk, we will consider applications of AI in the domain of psychiatry. Specifically, we will give an overview of our research towards automated behavioral analysis for assessing psychiatric symptoms. Schizophrenia (SCZ) and depression (MDD) are two chronic mental disorders that seriously affect the quality of life of millions of people worldwide. We aim to develop machine-learning methods with objective linguistic, speech, facial, and motor behavioral cues to reliably predict the severity of psychopathology or cognitive function, and distinguish diagnosis groups. We collected and analyzed the speech, facial expressions, and body movement recordings of 228 participants (103 SCZ, 50 MDD, and 75 healthy controls) from two separate studies. We created an ensemble machine-learning pipeline and achieved a balanced accuracy of 75.3% for classifying the total score of negative symptoms, 75.6% for the composite score of cognitive deficits, and 73.6% for the total score of general psychiatric symptoms in the mixed sample containing all three diagnostic groups. The proposed system is also able to differentiate between MDD and SCZ with a balanced accuracy of 84.7% and differentiate patients with SCZ or MDD from healthy controls with a balanced accuracy of 82.3%. These results suggest that machine-learning models leveraging audio-visual characteristics can help diagnose, assess, and monitor patients with schizophrenia and depression.
Speaker:
Dr. Justin Dauwels is an Associate Professor at the TU Delft (Signals and Systems, Department of Microelectronics), and serves as co-Director of the Safety and Security Institute at the TU Delft. He also is the scientific lead of the Model-Driven Decisions Lab (MoDDL), a first lab for the Knowledge Building program between the Netherlands police and the TU Delft.
His research interests are in data analytics with applications to intelligent transportation systems, autonomous systems, and analysis of human behavior and physiology. His academic lab has spawned four startups across a range of industries, ranging from AI for healthcare to autonomous vehicles.
Link to the webinar: https://us02web.zoom.us/j/87653221538?pwd=g8J1c2iwOKMrFZNsQT2d2PZ7R9W8HW.1
Additional information ...
MSc SPS Thesis presentation
- Monday, 10 March 2025
- 14:00
- HB17.140 (Seminar room)
Surgical Workflow Analysis, an Explainable Approach
Christos Spiliadis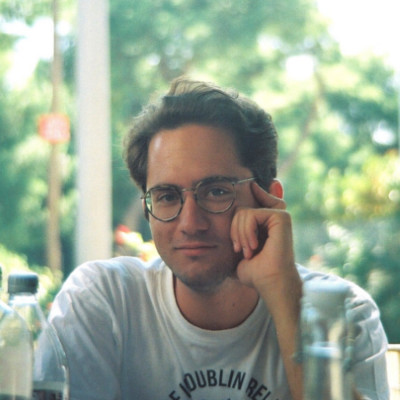
Surgical workflow analysis is crucial in optimizing procedural efficiency, resource utilization, and patient safety in catheterization laboratories. Traditional manual workflow analysis methods are labour-intensive and prone to inconsistencies, prompting the need for automated solutions that leverage machine learning and computer vision. This thesis presents an explainable two-stage model for surgical workflow analysis using ceiling-mounted cameras.
The proposed approach integrates a YOLOv8 object detection model with a Gaussian Mixture Model - Hidden Markov Model (GMM-HMM) framework. The first stage detects key objects that are input to the second stage. The GMM-HMM component then infers surgical workflow phases by modelling spatial and temporal dynamics, enabling real-time phase classification. The model is validated on two datasets from different hospitals, achieving a classification accuracy of 95.2% for the RDGG dataset and 95.4% for the Tampere dataset, ensuring its generalisability across diverse clinical environments. Experimental results demonstrate that the model is highly accurate in detecting workflow phases, emphasizing explainability and robustness. Combining YOLOv8’s efficient object detection with GMM-HMM’s structured temporal inference ensures that phase transitions are identified with minimal error. The model’s real-time feasibility and generalization across hospitals highlight its potential for clinical implementation.
This research advances automated surgical workflow analysis by addressing the dual challenges of interpretability and adaptability. Future work includes enhancing the model’s robustness to occlusions, integrating additional modalities such as audio data, and exploring its application in other surgical environments.
Additional information ...
Seminar Tadashi Ebihara (University of Tsukuba, Japan)
- Monday, 10 March 2025
- 15:30-16:30
- EEMCS, HB. 17.140
Underwater Acoustic Communication and Positioning in Shallow Waters
Tadashi Ebihara, Associate Professor, University of Tsukuba, Japan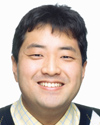
Underwater Acoustic Communication and Positioning in Shallow Waters
This study addresses the challenges of underwater acoustic (UWA) communication and positioning in shallow waters, which are characterized by significant delay and Doppler spreads. To provide a highly reliable communication, we propose Doppler-resilient orthogonal signal-division multiplexing (D-OSDM), which preserves orthogonality among data vectors even in doubly spread channels. Field tests, including MIMO configurations, demonstrate that D-OSDM enables reliable data transfer in mobile UWA environments with delay and Doppler spreads. Additionally, a new underwater positioning method is proposed for shallow waters, using direct wave arrival time groups and database matching. This method accurately measures baseline length from the impulse response of the UWA channel. Experimental trials in a harbor area showed positioning errors of about 0.2 meters and a near 0% missing rate, indicating the potential of this system for unmanned and remote underwater construction.
Microelectronics Research Day 2025
- Tuesday, 28 January 2025
- 10:00-18:00
- Aula
Microelectronics Research Day 2025
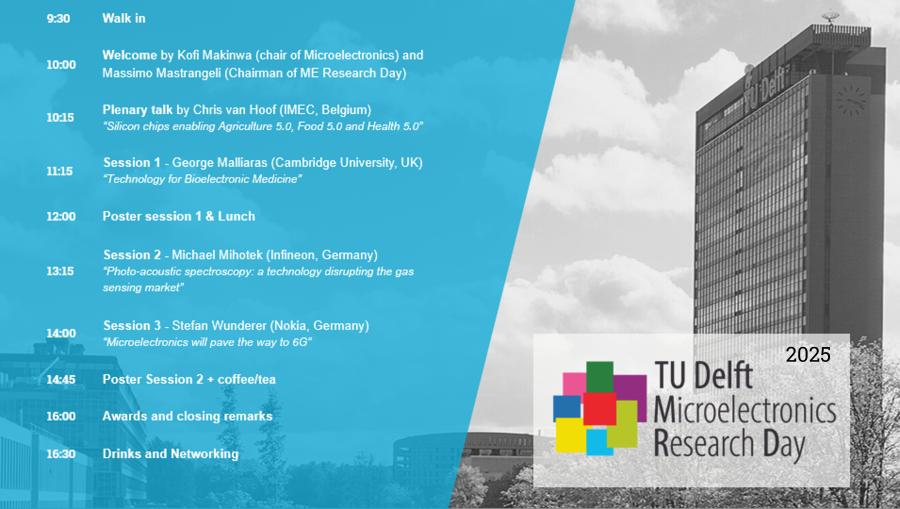
The second edition of TU Delft's Microelectronics Research Day will take place in the gorgeous and spacious setting of the Aula and its magnificent auditorium - come and join us to celebrate the department's great achievements and forward-looking community!
Program of the day
9:30 Walk-in
10:00 Welcome by Kofi Makinwa (chair of Microelectronics)
and Massimo Mastrangeli (Chairman of Research Day)
10:15 Plenary talk by Chris van Hoof (imec, Belgium)
11:15 Invited talk by George Malliaras (Cambridge University, UK)
12:00 Poster session 1 & lunch
13:15 Invited talk by Michael Mihotek (Infineon, Germany)
14:00 Invited talk by Stefan Wunderer (Nokia, Germany)
14:45 Poster session 2 & coffee/tea
16:00 Awards & closing remarks
16:30 Drinks & networking

MSc SPS Thesis presentation
- Thursday, 23 January 2025
- 09:30-11:30
- Lecture Hall D@ta (36.HB.01.630)
Distributed Affine Formation Control with Quadcopters
Shaan Hossain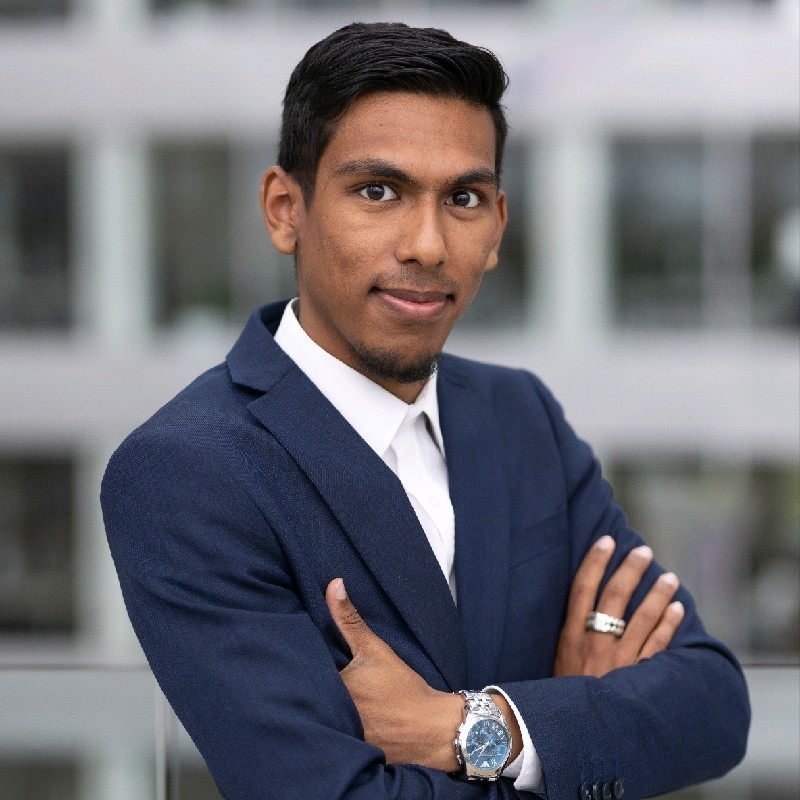
Microelectronics colloquium
- Thursday, 23 January 2025
- 16:00-17:00
- EEMCS, lecture hall Chip
Signal Processing for Intelligent Multi-Agent Swarms
Raj Thilak Rajan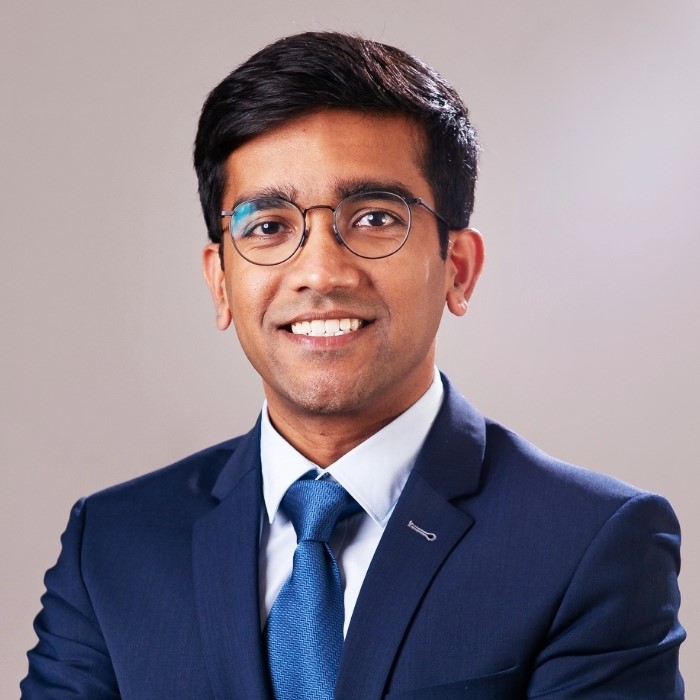
Multi-agent swarms or mobile networked cyber-physical systems are increasingly adopted in various applications, to enable robust and efficient operations in diverse and challenging environments. One of the key features of these multi-agent systems is edge-based autonomy and intelligence. Signal processing modelling plays a pivotal role in enabling intelligent multi-agent swarms — such as drones, autonomous vehicles, satellites, and space rovers — to achieve precise positioning, efficient navigation, optimal calibration, robust control, and adaptive learning in both terrestrial and non-terrestrial applications. Focusing on foundational Bayesian methods, we explore how sensor fusion and distributed optimisation empower agents to model complex behaviours, and enable them to make informed decisions in inaccessible and dynamic environments. In this talk, I aim to highlight key challenges and initial results from ongoing projects. I will also present the various interdisciplinary initiatives forged within TU Delft and beyond, that facilitate collaboration, validation and valorisation of signal processing across diverse multi-agent systems.
Additional information ...
MSc SPS Thesis presentation
- Thursday, 9 January 2025
- 14:00-16:00
- HB17.140 (Seminar room)
Dopple GroupChat (Dereverberation & Denoise in multi-channel full-duplex communication systems)
Long Xin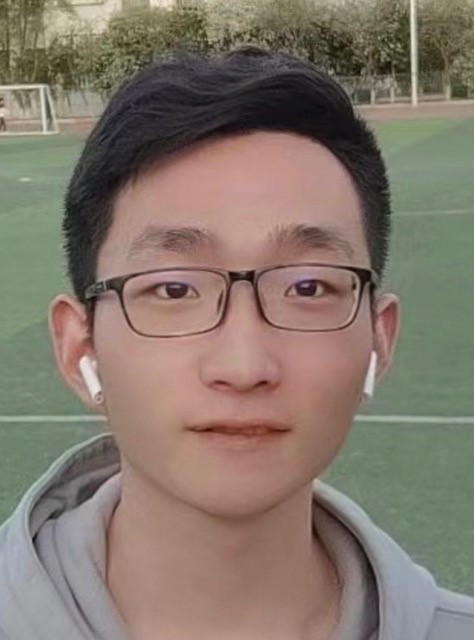
In response to the echo and noise interference problems faced in multi-user full-duplex voice communication scenarios, this work proposes a comprehensive solution encompassing signal modeling to beamforming optimization. First, by converting the Bluetooth channel into a virtual acoustic channel, a comprehensive acoustic transmission model covering both single-source and multi-source scenarios is established. Building on this foundation, various beamforming and filtering strategies are discussed, culminating in the proposal of the Multichannel Wiener Filter (MWF) beamforming scheme. Additionally, for the time-varying spatial domain, the update of the covariance matrix combined with a Voice Activity Detector (VAD) is addressed. Simulations demonstrate that the MWF scheme exhibits strong robustness and adaptability under different Bluetooth channel latencies and low Signal-to-Interference Ratio (SIR) environments. Furthermore, system performance is further enhanced when the user end is equipped with a sufficient number of microphones or in the presence of multiple bystanders.
PhD Thesis Defense
- Tuesday, 3 December 2024
- 10:00
- Aula Senaatszaal
Modelling Spatiotemporal Variability of Brain Responses in Functional Ultrasound
Aybüke Erol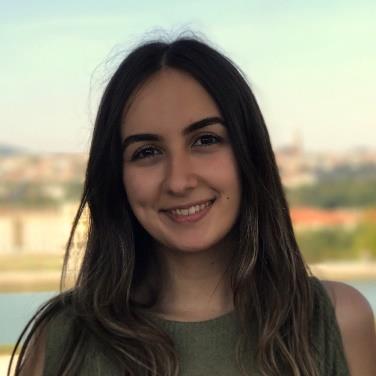
Decoding the Brain: Advances in Neuroimaging and Data Fusion
- Monday, 2 December 2024
- 13:30-17:00
- Aula, TU Delft (commissiekamer 3)
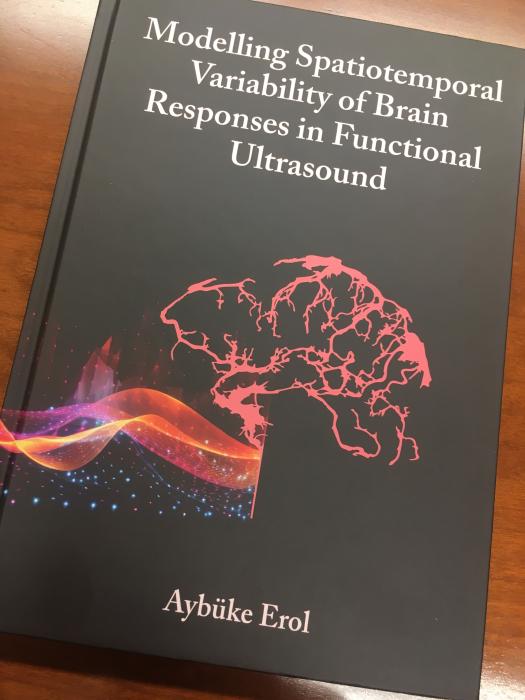
You are warmly invited to the symposium “Decoding the Brain: Advances in Neuroimaging and Data Fusion”, on the occasion of the PhD defense of Aybüke Erol (promotor: prof. Alle-Jan Van der Veen, co-promotors: dr. Borbala Hunyadi, dr. Pieter Kruizinga).
Aybüke’s PhD was supported by the Synergy Grant of the Department of Microelectronics, TU Delft. Her work combined functional ultrasound imaging with advanced signal processing to better understand task related brain hemodynamics.
This symposium will feature three talks. First, Prof. Matteo Carandini explains how neural recordings and imaging of blood flow can shed light on different brain states. Then, Aybüke Erol presents signal models for analysing such blood flow responses in functional ultrasound. Finally, Prof. Tülay Adalı highlights recent computational advances in large-scale neuroimaging data analysis and fusion. Detailed program and speaker bios are in the attached flyer.
Additional information ...
MSc SPS Thesis presentation
- Friday, 18 October 2024
- 11:00
- HB17.140 (Seminar room)
3D Microscopy Deconvolution of Very Large Images with an Adaptive Resolution Scheme
Chang GeEPFL
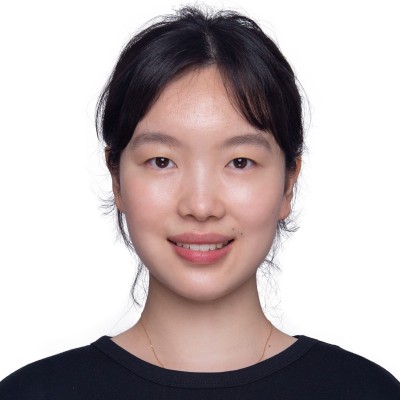
Microscopy is a crucial tool across various scientific domains. Due to light diffraction, the images acquired from optical microscopes are usually blurry and corrupted by noise. For an accurate quantitative analysis, the measured images need to be deconvolved to achieve higher resolution. Deconvolution processes are computationally expensive, due to the large data size. This leads to out-of-memory issues and extended computation time.
To address these problems, this project aims to develop a novel convolution scheme. It utilizes the special structure of the Point Spread Function, which in conventional microscopy techniques has most of its energy concentrated in the center. And implement multi-resolution signal processing methods. This approach enhances computational efficiency while retaining computational accuracy.
Additional information ...
PhD Thesis Defence
- Monday, 7 October 2024
- 15:00-17:00
- Aula Senaatszaal
Signal Processing and Optimization on Graphs: Learning Time-Varying Structures and Generalizing Convolution Principles
Alberto Natali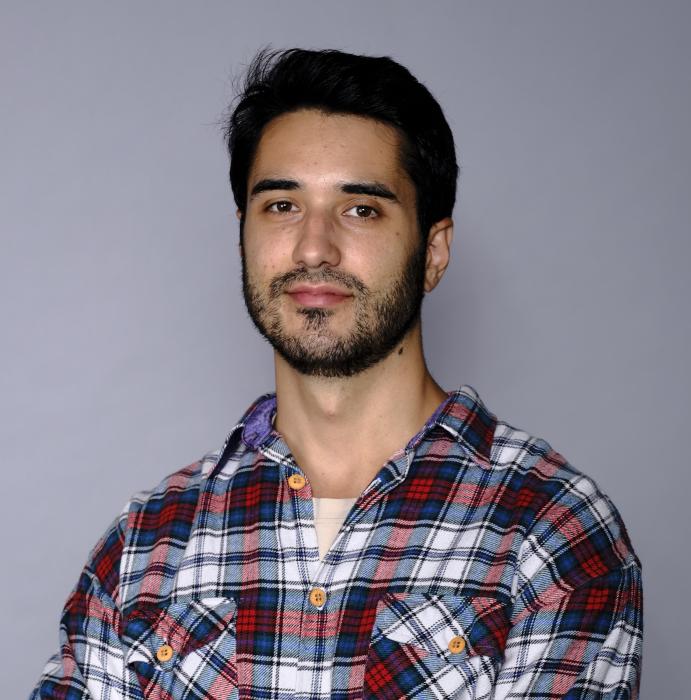
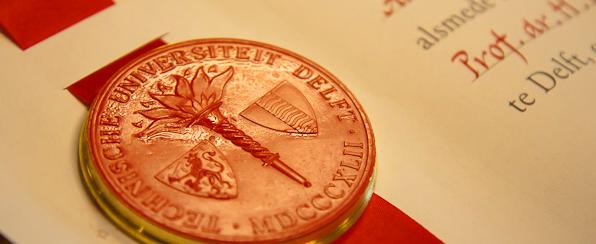
SPS Seminar | Paolo Banelli
- Monday, 7 October 2024
- 11:00-12:00
- EEMCS, Seminar room HB17.140
The Opportunistic Information Bottleneck in Goal Oriented Communications
Paolo BanelliUniversity of Perugia, Perugia, Italy
The Information Bottleneck (IB) represents a key-enabler for edge-assisted Goal-Oriented Communications ( GOC), since it allows to retrieve a compact representation of a data-source to be transmitted to an edge-server to feed learning tasks, taking into account specific requirements in terms of learning performance. Despite its theoretical value, this method is difficult to apply in practice, because it is based on a functional optimization problem that admits a closed-form solution only in specific cases (e.g, Multivariate Normal regression). To overcome this limitation, an Opportunistic Information Bottleneck (OIB) can be employed to adapt the optimal solution of the Multivariate Gaussian IB to general inference tasks that exploit Deep Neural Networks (DNNs).
Additional information ...
PhD Thesis Defence
- Wednesday, 2 October 2024
- 17:30-19:00
- Aula Senaatszaal
3D Motion-Based High-Resolution Imaging Techniques for Automotive Radar
Sen Yuan
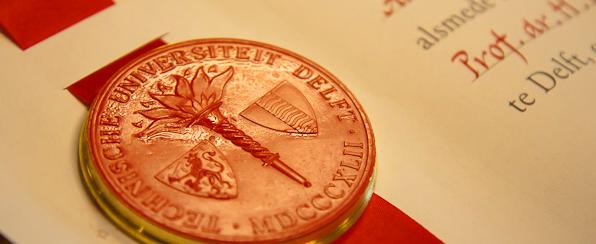
MSc SPS Thesis presentation
- Friday, 27 September 2024
- 14:00-16:30
- HB17.140 (Seminar room)
Graph Topology Identification Based on Covariance Matching
Yongsheng Han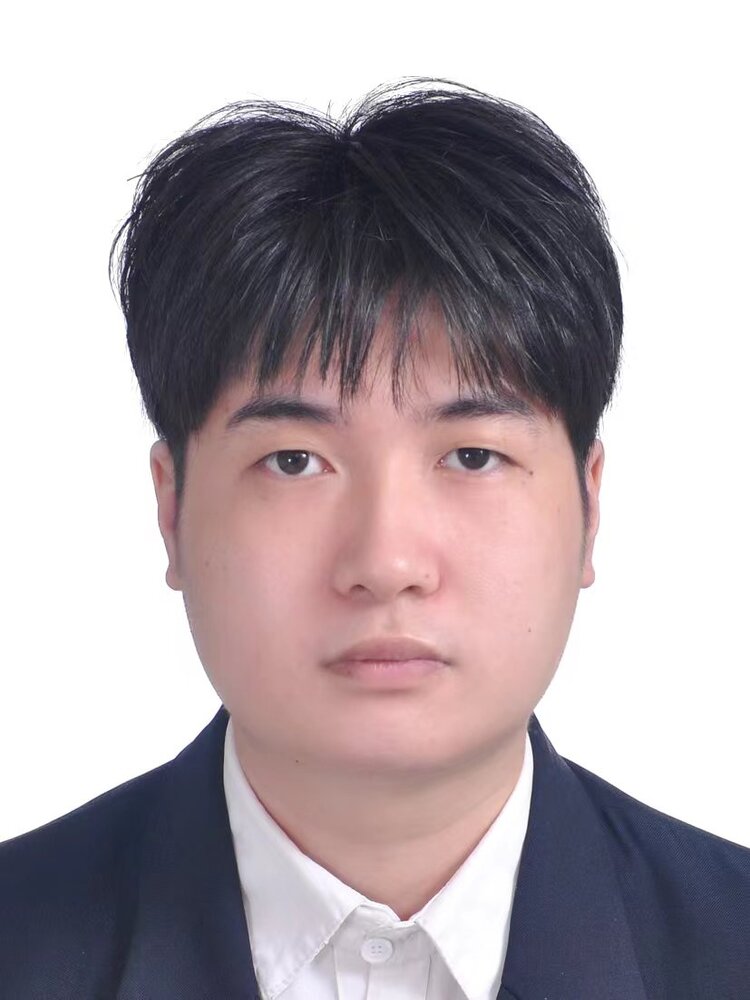
This project addresses graph topology identification for applications where the underlying structure of systems like brain and social networks is not directly observable. Traditional approaches based on signal matching and spectral templates have limitations, particularly in handling scale issues and sparsity assumptions. We introduce a novel covariance matching methodology that efficiently reconstructs the graph topology using observable data. For the structural equation model (SEM) using an undirected graph, we demonstrate that our method can converge to the correct result under relatively soft conditions. Furthermore, we extend our methodology to polynomial models, sparse directed graphs, and any known Gaussian distribution of latent variables, broadening its applicability and utility in diverse graph-based systems.
PhD Thesis Defence
- Wednesday, 18 September 2024
- 17:30-19:00
- Aula Senaatszaal
Automotive Polarimetric Radar for Enhanced Road Surface Classification & Vulnerable Road User Identification
Wietse Bouwmeester
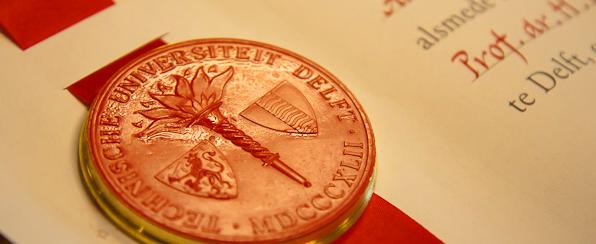
MSc SPS Thesis presentation
- Monday, 26 August 2024
- 10:00-11:30
- HB17.140 (Seminar room)
Automated Epilepsy Diagnosis beyond IEDs by Multimodal Features and Deep Learning
Yash Mirwani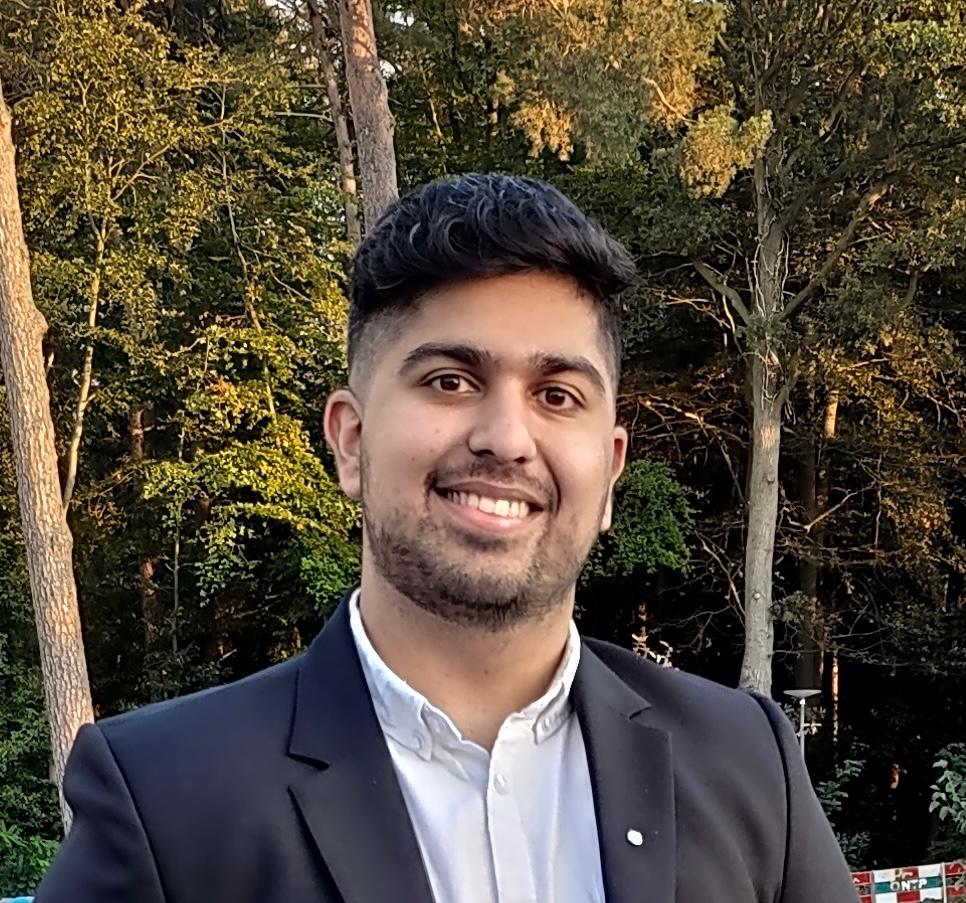
Automated diagnosis of epilepsy for differentiating epileptic EEGs without Interictal Epileptic Discharges (IEDs) from normal EEGs remains a critical challenge in clinical settings. Current state-of-the-art methods use algorithms that can effectively detect epilepsy seizures which improves the current treatment methods for people suffer from epilepsy. Electroencephalograms (EEGs) analyzed by neurologists which are not able to meet the criteria are further looked into to obtain an efficient classification. However, this manual process can be time-consuming and prone to errors.
The main objectives of this research include the development of a robust multi-processing feature extraction pipeline, the application of VGG16 model / XGBoost classifier, and the validation of the proposed methods on comprehensive EEG datasets. Specifically, the focus is on detecting epilepsy in EEG data without Interictal Epileptic Discharges (IEDs) which poses a significant challenge due to the complex nature of the EEG signals in such cases.
This thesis presents an automated epilepsy diagnosis approach using a multi-algorithmic feature extraction pipeline. The final models include the development of a robust multi-processing feature extraction pipeline, the application of advanced machine learning / deep learning algorithms, and the validation of the proposed methods on comprehensive EEG datasets. The results, achieved using an XGBoost classifier with leave-one-subject-out (LOSO) cross-validation, demonstrate comparable performance to state-of-the-art epilepsy detectors. The study emphasizes the detection of epilepsy without IEDs, optimizing models through nested cross-validation, and evaluates their performance on the Temple University Hospital (TUH) and Erasmus MC (EMC) Rotterdam datasets.
MSc SPS Thesis presentation
- Friday, 23 August 2024
- 14:30-16:00
- HB17.140 (Seminar room)
Performance assessment of IVA-based subgroup identification methods and their application in experimental fUS data
Christiaan Bot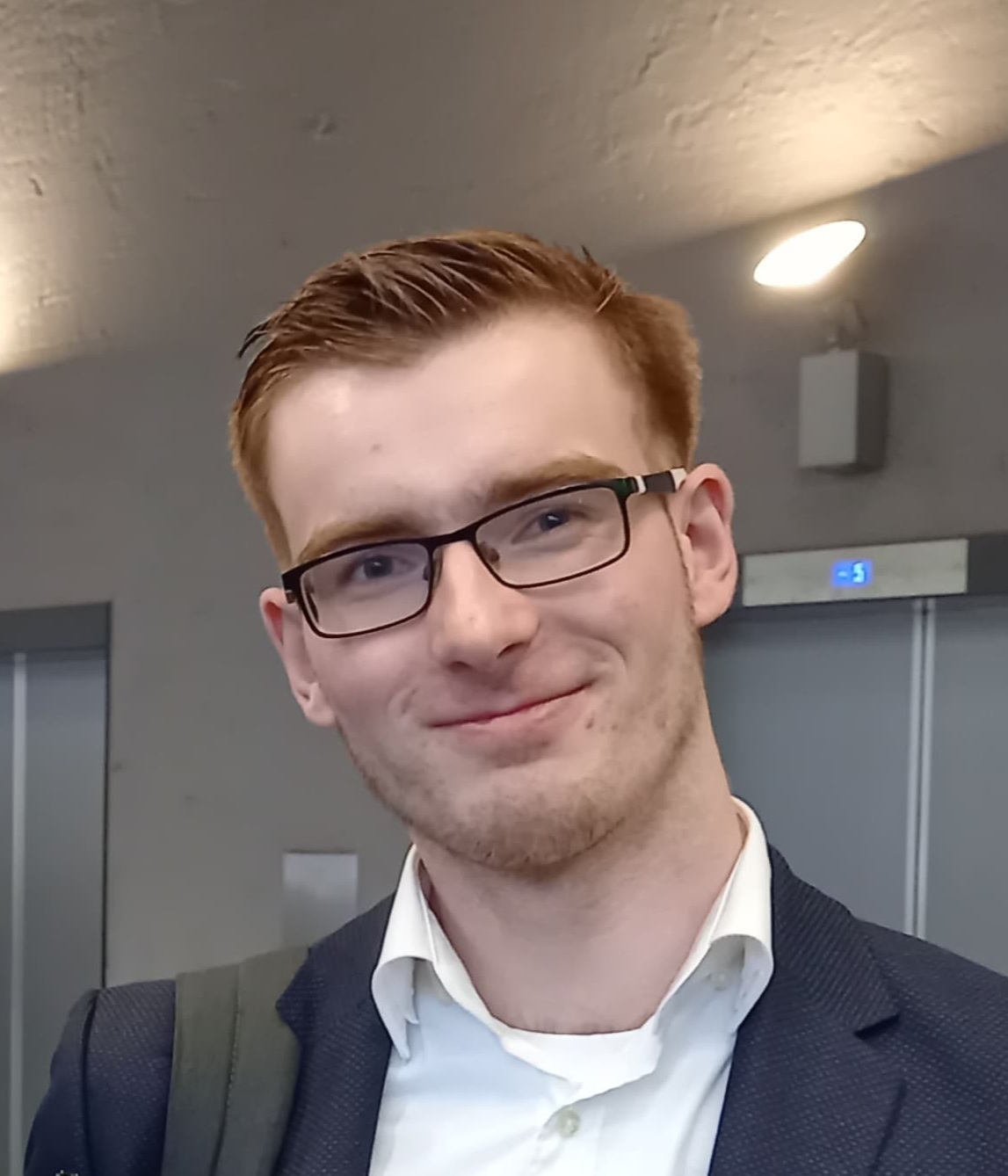
Using independent vector analysis (IVA) to analyze and find subgroups in functional magnetic resonance imaging (fMRI) and functional ultrasound (fUS) data requires a lot of manual labour. Recently methods like subgroup identification using IVA (SI-IVA) and IVA for common subspace identification (IVA-CS) have tried to reduce this labour through automation. However, both methods did not test for accuracy. This thesis shows through simulations that these proposed methods are not accurate or robust enough to be trusted and that spectral clustering is a better alternative for automatic subgroup identification. Spectral clustering is then incorporated into the analysis of experimental fUS data of two groups of mice to try and identify these automatically. In this analysis, adaptive constrained IVA (acIVA) was used to incorporate references, further improving the interpretability of the results as components are directly linked to prior constraints. However, applying subgroup analysis showed that the mice could not be clustered based on their response to the stimuli. Still, spectral clustering is more accurate in the simulations making it a promising alternative for automatic subgroup identification. Furthermore, combining spectral clustering with acIVA makes the results more interpretable due to constrained components not being subject to permutation ambiguity.
MSc SPS Thesis presentation
- Thursday, 22 August 2024
- 13:00-14:30
- HB17.140 (Seminar room)
Towards Optimizing PMCW Radar: Low Complexity Correlation and Enhanced Frame Design
Hanqing Wu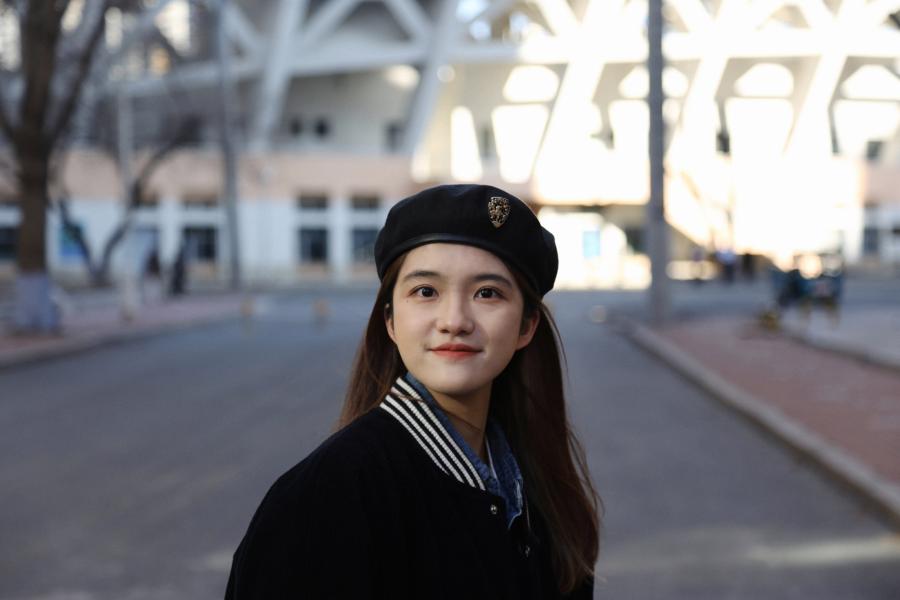
The rapid development of Advanced Driver Assistance Systems (ADAS) necessitates enhanced performance in automotive radar systems, with Phase Modulated Continuous Wave (PMCW) radar emerging as a key technology due to its high resolution, interference resistance, and robust performance. Despite these advantages, PMCW radar faces challenges such as high computational complexity and Doppler-induced range sidelobes. This thesis addresses these challenges by proposing a novel correlation method to reduce computational complexity and enhance processing efficiency, ensuring reliable target detection. Additionally, we tackle Doppler-induced range sidelobes by introducing code diversity and novel frame designs for MIMO systems, leveraging cyclic shifts and Hadamard matrices to balance sidelobe attenuation and sequence set size requirements. Through extensive analysis and simulations, the proposed methods demonstrate significant improvements in radar performance, especially in detecting weak targets behind strong reflectors. The findings contribute to developing more efficient, reliable, and scalable PMCW radar systems for advanced automotive applications.
Additional information ...
MSc SPS Thesis presentation
- Thursday, 25 July 2024
- 14:00-15:30
- HB17.140 (Seminar room)
Advanced Digital Signal Processing for Probabilistic Constellation Shaping and Partial Response Signaling
Chenrui XuETHz

Probabilistic constellation shaping (PCS) and partial response signaling (PRS) promise higher-speed communications but challenge the receiver-side digital signal processing (Rx DSP) developed for conventional QAM. This thesis focuses on the carrier recovery in Rx DSP, which is crucial for coherent optical communication, and proposes a carrier recovery scheme using generalized maximum likelihood estimation with negligible pilot overhead (approximately 0.2%). Through simulations and 100 GBaud experiments with PCS-64QAM, our scheme shows greater stability and accuracy, and doubles computational efficiency compared to others. Additionally, in a popular PRS scheme, Tomlinson-Harashima precoding combined with polybinary shaping (THP + Polybinary), we discuss the disputed issues regarding 2M modulo operations and investigate their impact on carrier recovery. Based on simulations and 96 GBaud experiments with THP + Polybinary-16QAM, our carrier recovery scheme enhances both accuracy and stability, effectively mitigating issues caused by zero symbols and residual inter-symbol interference. With the proposed scheme, gains from PCS and PRS can be further utilized in practical optical communication to achieve ultra-fast transmission. For the future, modifying the timing recovery and the blind equalization in Rx DSP to suit PCS/PRS systems is prioritized to further maximize PCS/PRS gains.
Conferences
- Monday, 24 June 2024
- Aula, TU Delft
7th Graph Signal Processing Workshop (GSP 2024)
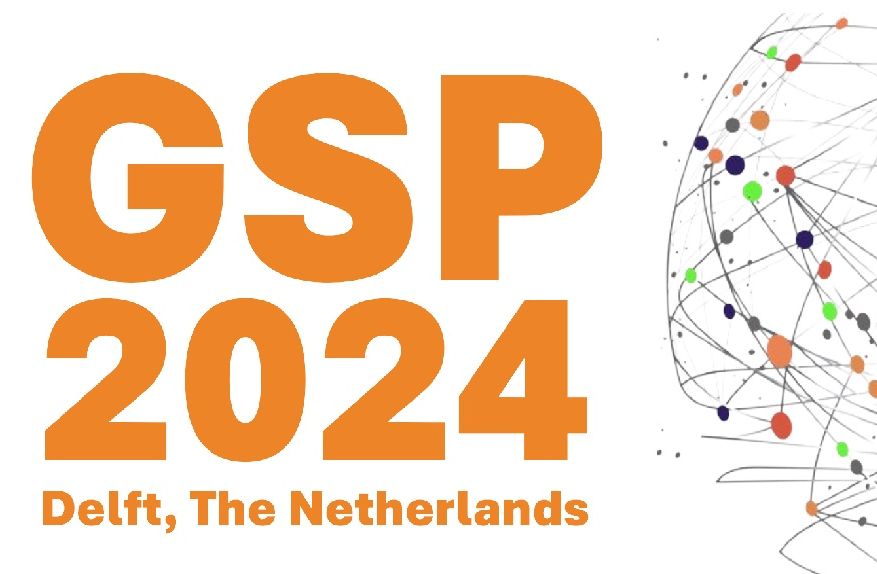
Following a series of successful workshops since 2016, we are pleased to announce that the 7th Edition of the Graph Signal Processing Workshop will be held June 24-26, 2024 in Delft, The Netherlands (campus TU Delft). The workshop will provide a warm welcome to experts and practitioners from academia and industry in the field of graph signal processing (GSP). The goal of GSP is to generalize classical signal processing and statistical learning tools to signals on graphs (functions defined on a graph).
Additional information ...
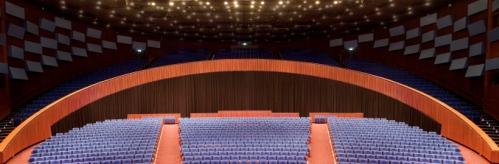
PhD Thesis Defence
- Tuesday, 18 June 2024
- 15:00-16:30
- Aula Senaatszaal
Model-based feature engineering of atrial fibrillation
Hanie Moghaddasi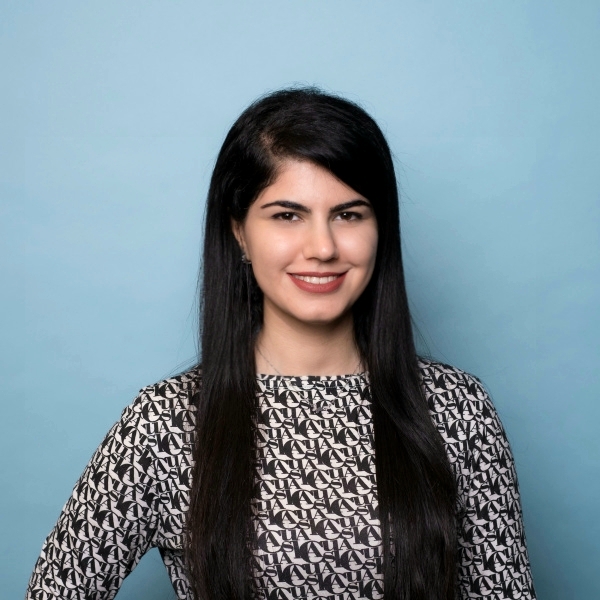
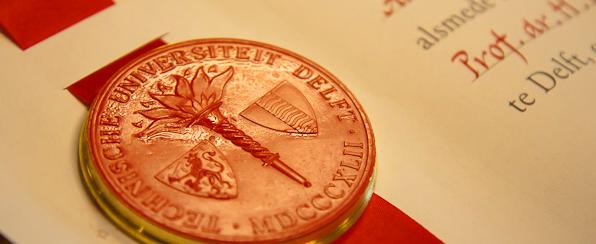
MSc SPS Thesis presentation
- Monday, 17 June 2024
- 16:00-18:00
- HB17.140 (Seminar room)
Precipitation Nowcasting using Deep Generative model
Zeineh Bou Cher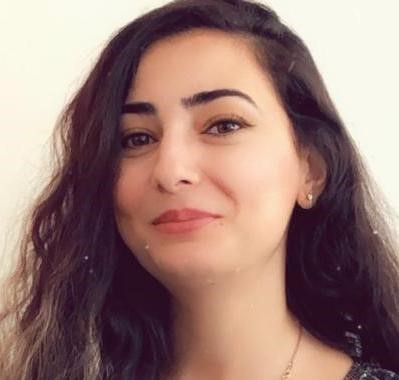
Abstract: Intense precipitation can have extensive economic outcomes, from disrupting outdoor activities to causing severe infrastructural damage, such as landslides, and endangering public safety. The urgency to mitigate these impacts underscores the need for improved early warning systems. Enhanced short-term weather prediction, or nowcasting, is critical for addressing these severe weather events effectively. Traditional meteorological forecasting methods, while foundational, are often constrained by simplistic physical assumptions and fail to capture the complex, nonlinear patterns of intense weather events. These methods also struggle with high computational demands and lack the resolution needed to detect crucial microscale atmospheric phenomena for accurate short-term forecasts.
To address these challenges, this research introduces a novel deep learning approach utilizing a streamlined architecture that combines a Vector Quantized Variational Autoencoder (VQVAE) and an Autoregressive (AR) Transformer. This model aims to predict weather conditions up to 180 minutes ahead, using data analyzed at 30-minute intervals. The proposed model displays comparable performance with the state-of-theart conventional methods and other deep learning nowcasting models in predicting precipitation and sometimes extreme events. This study seeks to enhance forecasting accuracy and efficiency, providing valuable contributions to the field of meteorological nowcasting.
MSc SPS Thesis presentation
- Monday, 10 June 2024
- 16:00-18:00
- HB.20.150
Precipitation Nowcasting using Deep Generative model
Zeineh Bou Cher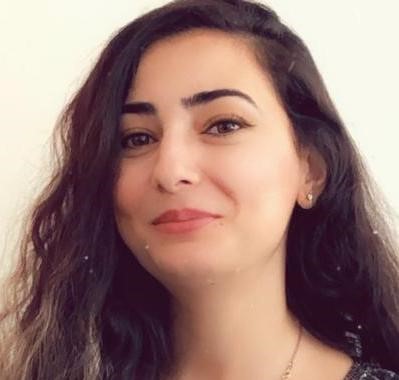
Abstract: Intense precipitation can have extensive economic outcomes, from disrupting outdoor activities to causing severe infrastructural damage, such as landslides, and endangering public safety. The urgency to mitigate these impacts underscores the need for improved early warning systems. Enhanced short-term weather prediction, or nowcasting, is critical for addressing these severe weather events effectively. Traditional meteorological forecasting methods, while foundational, are often constrained by simplistic physical assumptions and fail to capture the complex, nonlinear patterns of intense weather events. These methods also struggle with high computational demands and lack the resolution needed to detect crucial microscale atmospheric phenomena for accurate short-term forecasts. To address these challenges, this research introduces a novel deep learning approach utilizing a streamlined architecture that combines a Vector Quantized Variational Autoencoder (VQVAE) and an Autoregressive (AR) Transformer. This model aims to predict weather conditions up to 180 minutes ahead, using data analyzed at 30-minute intervals. The proposed model displays comparable performance with the state-of-theart conventional methods and other deep learning nowcasting models in predicting precipitation and sometimes extreme events. This study seeks to enhance forecasting accuracy and efficiency, providing valuable contributions to the field of meteorological nowcasting.
Additional information ...
Conferences
- Monday, 27 -- Tuesday, 28 May 2024
- Aula, TU Delft
44th Benelux Symposium on Information Theory and Signal Processing (SITB'24, Delft)
Additional information ...
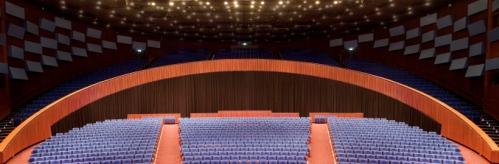
PhD Thesis Defence
- Tuesday, 21 May 2024
- 10:00-11:30
- Aula Senaatszaal
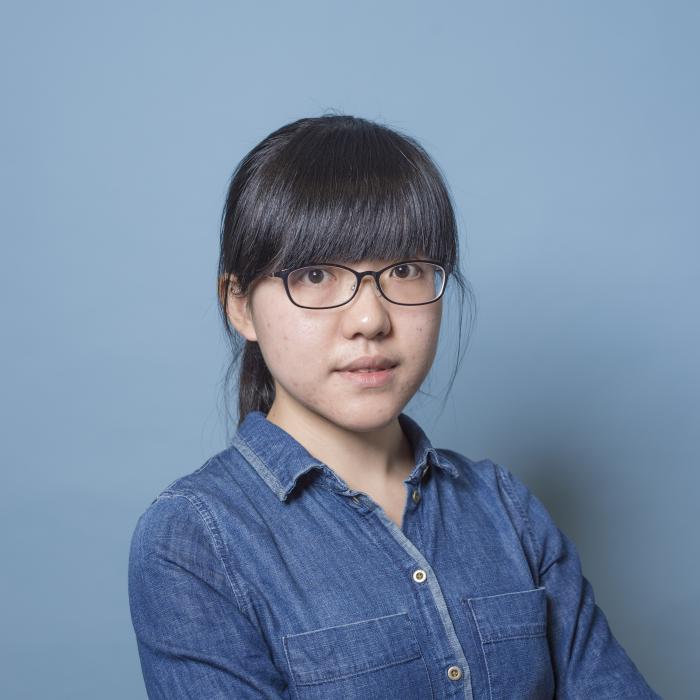
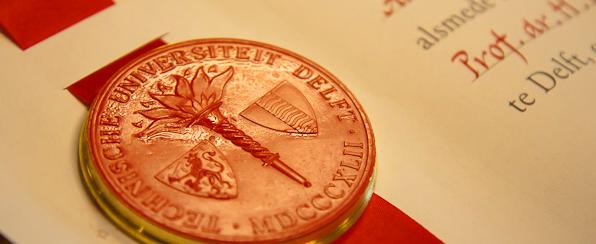
PhD Thesis Defence
- Monday, 6 May 2024
- 12:30-14:00
- Aula Senaatszaal
Multi-agent exploration under sparsity constraints
Christoph Manss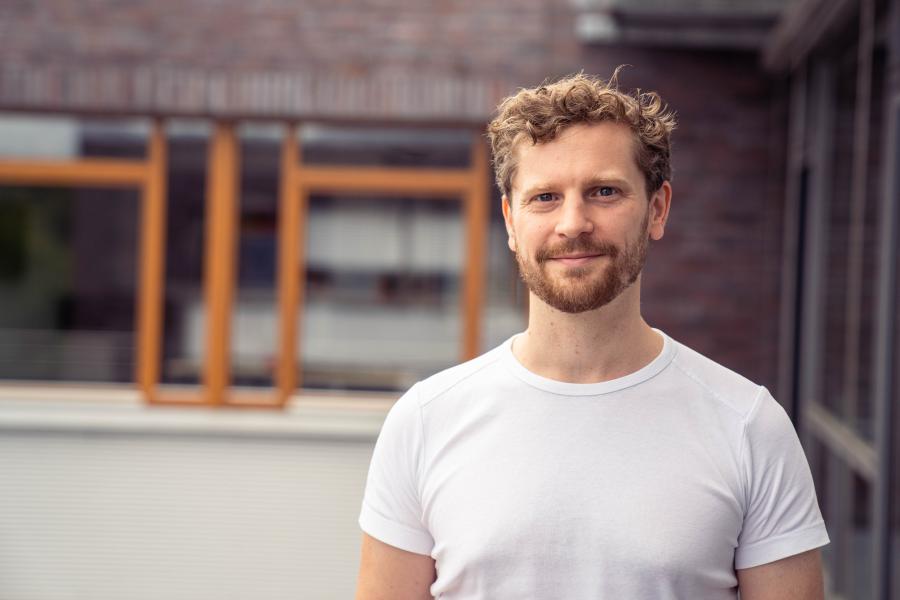
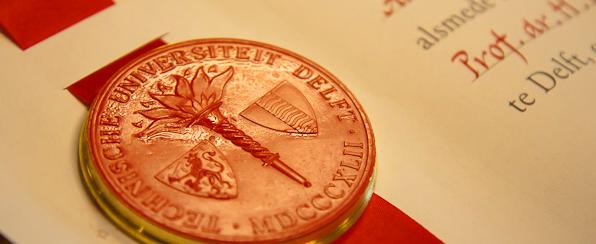
MSc SPS Thesis presentation
- Tuesday, 30 April 2024
- 10:00
- HB18.090
A SystemC SNN model for power trace generation
Wim KokPower analysis can be used to retrieve key information as secure systems leak data-dependent information over side channels. A proposed solution to break the correlation between side channel information and secret information was to replace a vulnerable part of the cryptography implementation with a neural network. This uses the inherent properties of a neural network to disrupt the correlation by breaking the linear power characteristics assumed by leakage models.
To test this neural network without physically creating a hardware implementation a simulation must be performed that provides both the data and the power information. Currently neural network simulators do not generate a power trace and analog circuit simulators generate more information traces than required increasing the simulation time.
This thesis describes the creation of a complete SystemC spiking neural network model that generates both data and power information. The information generated by this model was compared and verified with results acquired by the Cadence Spectre analog circuit simulation platform. The results indicate that the created SystemC SNN model works and generates comparable data and power traces as the Spectre simulator.
Signal Processing Seminar
- Monday, 29 April 2024
- 11:00-12:00
- HB 17.140
Harmonics to the Rescue: Why Voiced Speech Is Not a WSS Process
Giovanni Bologni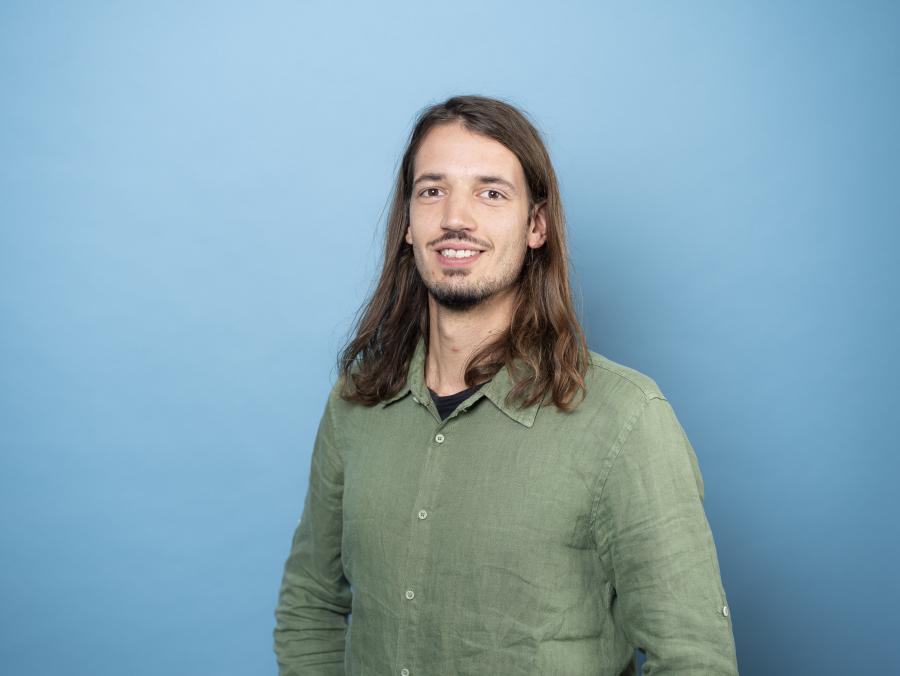
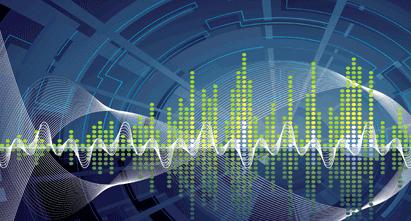
Signal Processing Seminar
- Thursday, 25 April 2024
- 11:00-12:00
- HB 17.140
Modelling Error Correction in Sparse Bayesian Learning via Grid Optimization
Yanbin He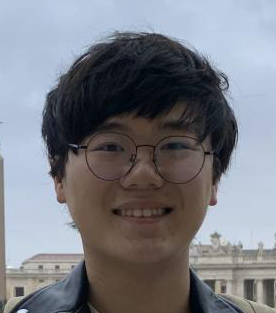
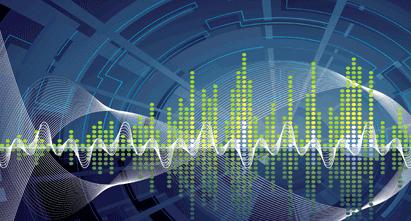
Signal Processing Seminar
- Thursday, 11 April 2024
- 11:00-12:00
- HB 17.140
Multiple Sensors Facilitated Automotive Perception System
Peiyuan Zhai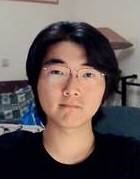
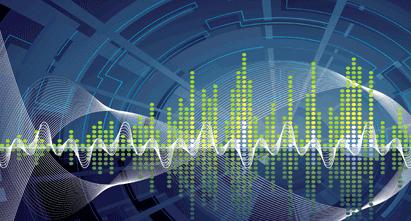
MSc SPS Thesis presentation
- Friday, 5 April 2024
- 14:30-16:31
- Snijderszaal (LB01.010)
Multiplicative Contrast Source Inversion method in Electrical Properties Tomography (CSI-EPT) based on Jacobi matrix inversion
Florens Helfferich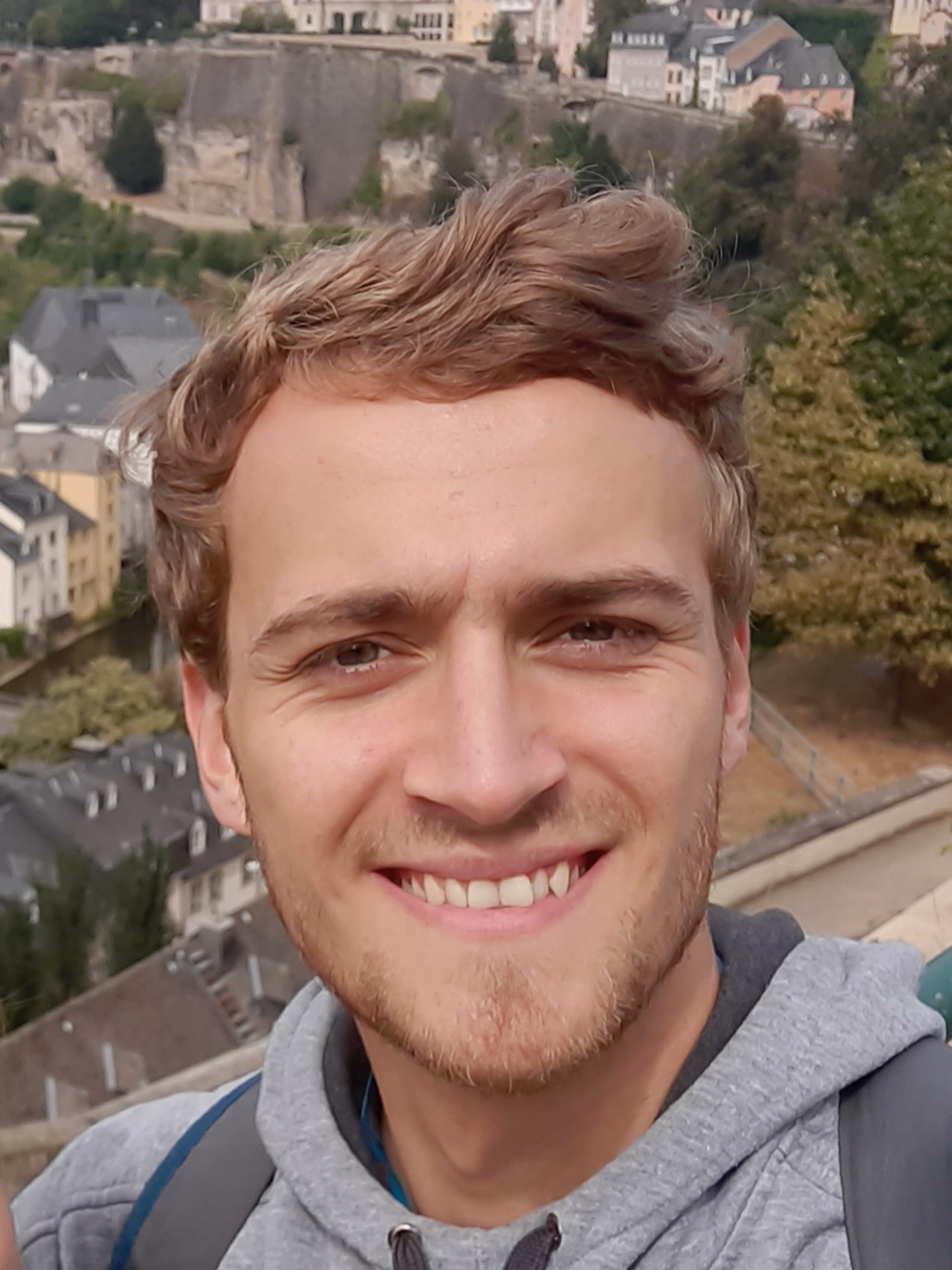
The biological tissue electrical properties of conductivity and permittivity affect the interactions of electromagnetic fields in the body. These properties vary throughout the different tissues as the tissue structure and composition varies. In this thesis, medical imaging and diagnosis is used as primary example to motivate exploration of a novel regularization approach to an MRI-based electrical properties tomography (EPT) method. Total variation (TV) regularization has been shown to perform noise reduction in the iterative Contrast Source Inversion EPT (CSI-EPT) method. The Jacobi matrix inversion regularization, an alternative to the known conjugate gradient formulation, is elaborated and applied to an E-polarized MRI fields scenario such that this thesis presents the Jacobi step regularized CSI-EPT. The alternative regularization method outperforms the known regularization method in the reconstruction qualities of noise-suppression and edge-preservation in the simulated MRI experiments using a virtual body model. Further advancements are also described, such as multiple inner-iterations Jacobi regularization and an anatomical prior initialization of the contrast function. Important future research topics are the incorporation and evaluation of the Jacobi step regularization into more advanced CSI-EPT versions, which are the three-dimensional and transceive phase based algorithms to correct realistic MRI data.
Signal Processing Seminar
- Thursday, 4 April 2024
- 11:00-12:00
- HB 17.140
Extracting Hemodynamic Activity With Low-Rank Spatial Signatures in Functional Ultrasound Using the Tensor Block Term Decomposition
Sofia Kotti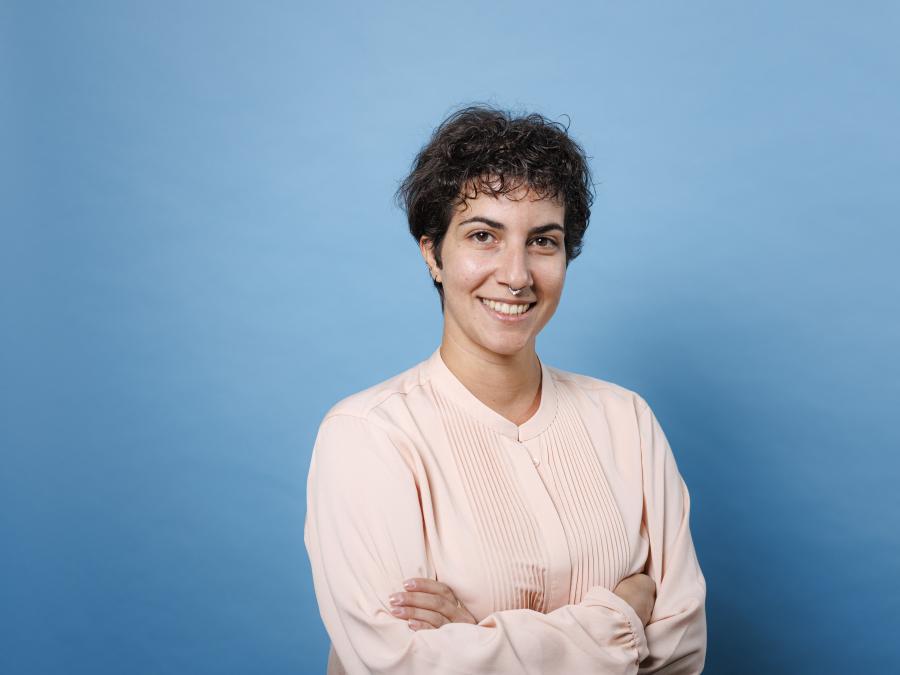
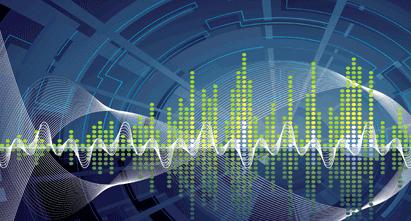
Signal Processing Seminar
- Thursday, 28 March 2024
- 11:00-12:00
- HB 17.140
Compositional Generative Modelling with Energy-based Models/Diffusion Models
Yanbo Wang, Srikar Chaganti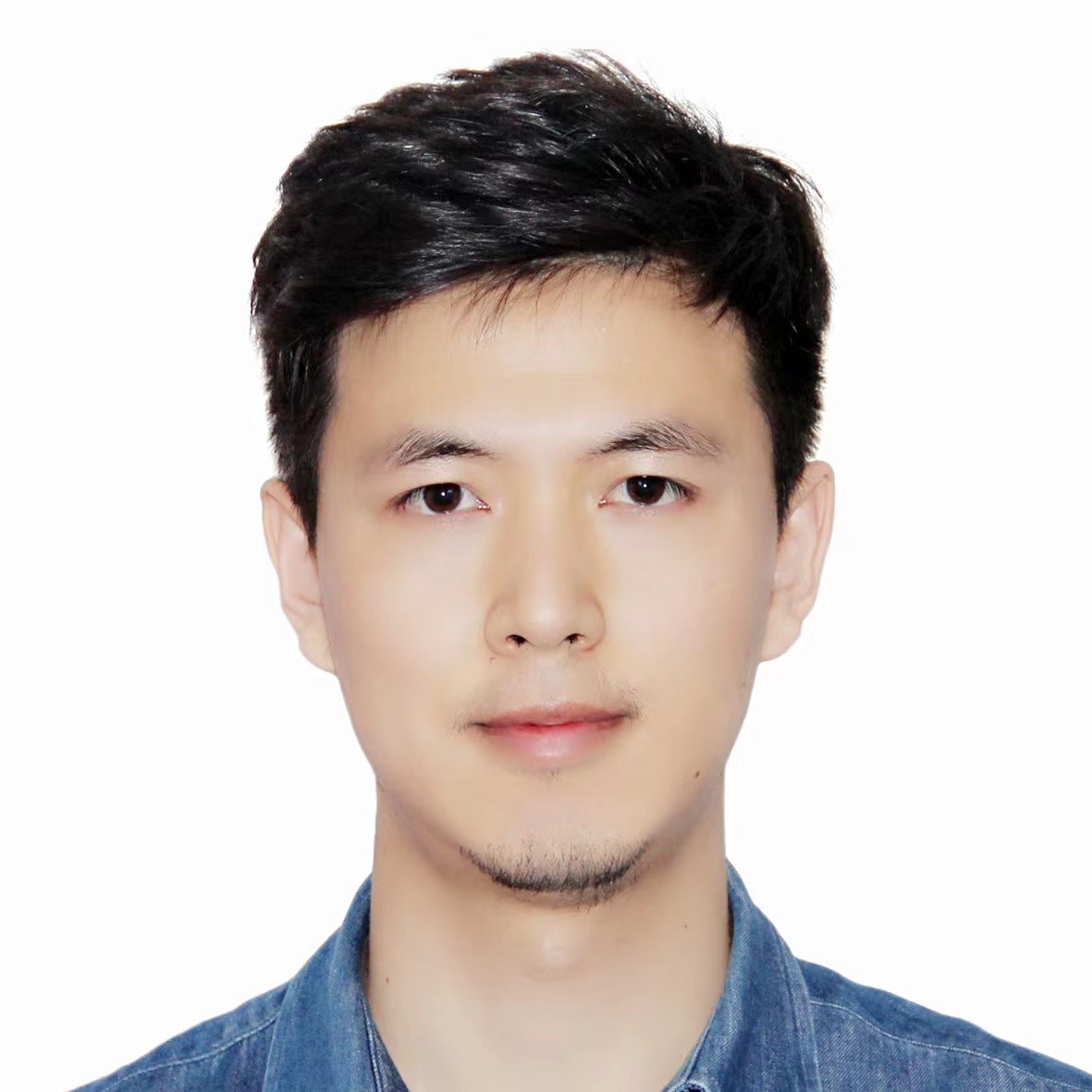
- Srikar Chaganti, “Target Localization using Distributed Radar under Communication Constraints” (MSc. Introduction)
- Yanbo Wang, “Compositional Generative Modelling with Energy-based Models/Diffusion Models”
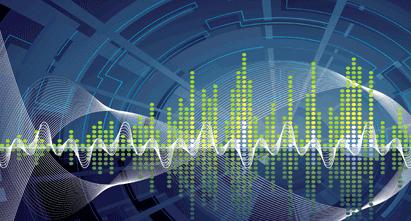
Signal Processing Seminar
- Thursday, 21 March 2024
- 11:00-12:00
- HB 17.140
Underwater Acoustic Communications: TNO-TUDelft Collaboration Opportunities
Costas PelekanakisTNO (Netherlands Organisation for Applied Scientific Research)
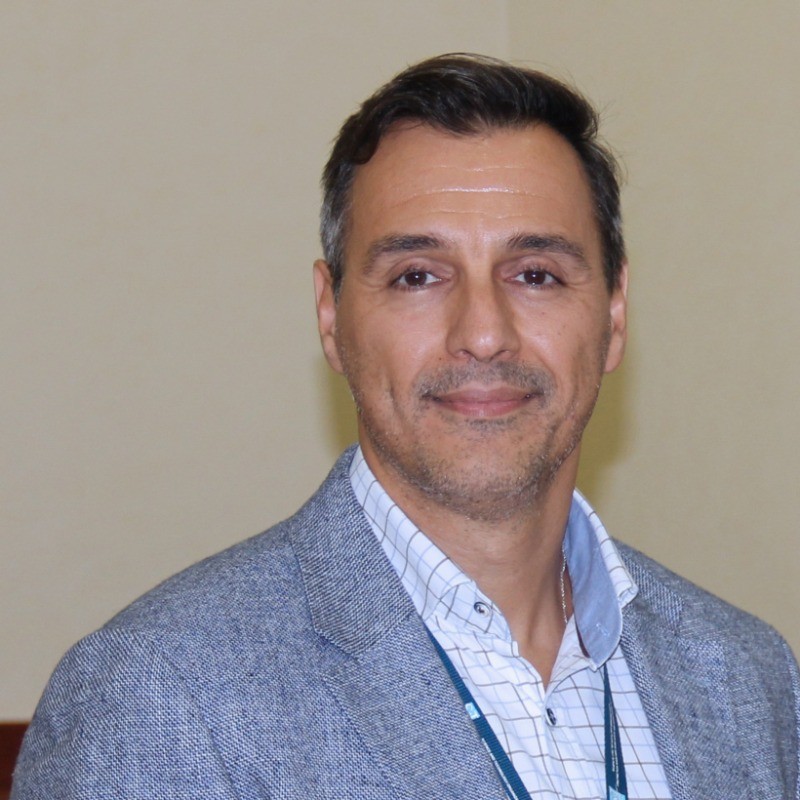
This talk/initiative aims to offer students the chance to collaborate with TNO, contributing to innovative research that shapes the field of underwater acoustic communications.
About Costas Pelekanakis
Costas received his Diploma from the Department of Electronic and Computer Engineering, Technical University of Crete, Greece, in 2001 and his M.Sc. and Ph.D. degrees in Ocean Engineering from MIT, USA, in 2004 and 2009, respectively. From 2009 to 2015, he worked with the Acoustic Research Laboratory at the National University of Singapore as a Research Fellow. In Singapore, he also worked as Lecturer for the Master of Defence Technology and Systems Program at the Temasek Defence Systems Institute from 2011 to 2014. From 2015 to 2023, he was a Senior Scientist at the NATO Centre for Maritime Research and Experimentation (CMRE) in La Spezia, Italy. He is currently a Senior Scientist at the Netherlands Organization for Applied Scientific Research (TNO) in the Hague, the Netherlands. In 2001, Costas was awarded the MIT Presidential Fellowship and in 2018, he was the co-recipient of the NATO Scientific Achievement Award. In 2019, he was the co-recipient of the IET Premium Award for Best Paper in Radar, Sonar & Navigation. His broad research area is underwater acoustic communications. Costas also serves as an Associate Editor for the IEEE Journal of Oceanic Engineering and is currently a member of the IEEE Oceanic Engineering Society (OES) Administrative Committee. He is a Senior member of the IEEE.
Additional information ...
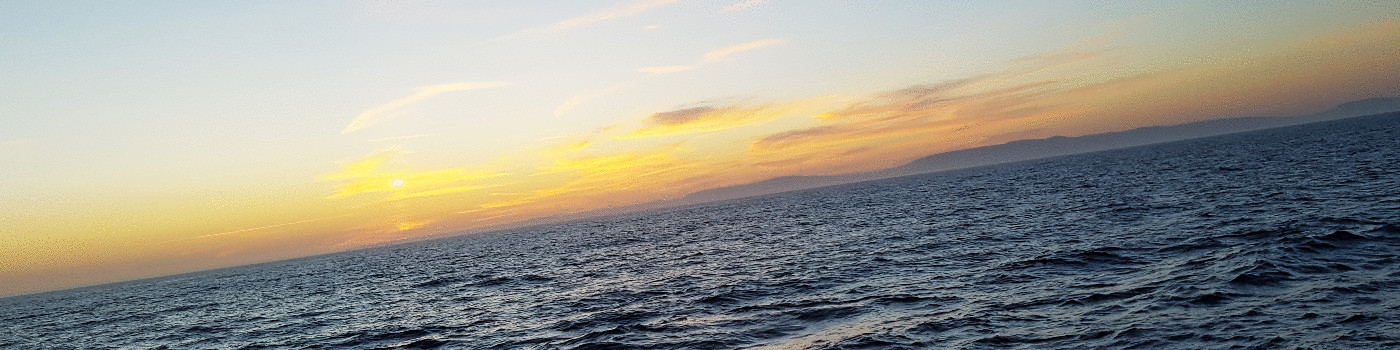
MSc SPS Thesis presentation
- Thursday, 21 March 2024
- 16:00-18:00
- HB17.140 (Seminar room)
Sparse Non-uniform Optical Phased Array Design
Kunlei Yu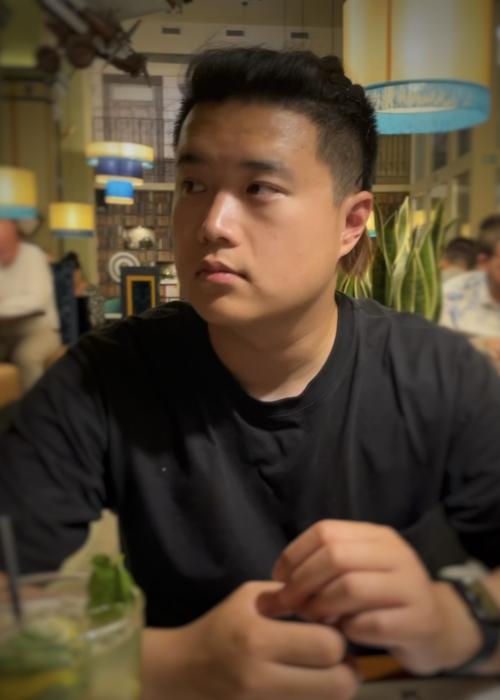
This thesis addresses the design and optimization of sparse non-uniform optical phased arrays (OPAs) for advanced automotive LiDAR systems. As autonomous driving technologies advance, the demand for high-resolution, reliable, and compact LiDAR systems has become increasingly critical. Traditional uniform OPAs, while effective, face limitations regarding power consumption. This work introduces an innovative approach to designing sparse non-uniform OPAs that achieve desired performance metrics essential for automotive applications, including beamwidth, field of view, and sidelobe levels, while minimizing element count and, consequently, energy consumption.
Through mathematical modelling and simulation, we formulate the problem of sparse OPA design as an optimization problem, leveraging techniques from compressive sensing to identify the most efficient element arrangements. We propose using the sparse array synthesis method to formulate the sparse OPA design problem, utilizing algorithms such as LASSO, thresholding, and iterative reweighted l1-norm minimization to achieve optimal sparse configurations. Our results demonstrate substantial improvements in effectiveness, offering a practical solution to the constraints posed by current LiDAR systems. This thesis contributes to the field by providing a comprehensive framework for the design of sparse non-uniform OPAs, highlighting the trade-offs and benefits of various design strategies. The findings advance our understanding of OPA design principles.
MSc SPS Thesis presentation
- Wednesday, 20 March 2024
- 13:30-15:30
- HB17.140 (Seminar room)
Sparse Non-uniform Optical Phased Array Design
Ankush Roy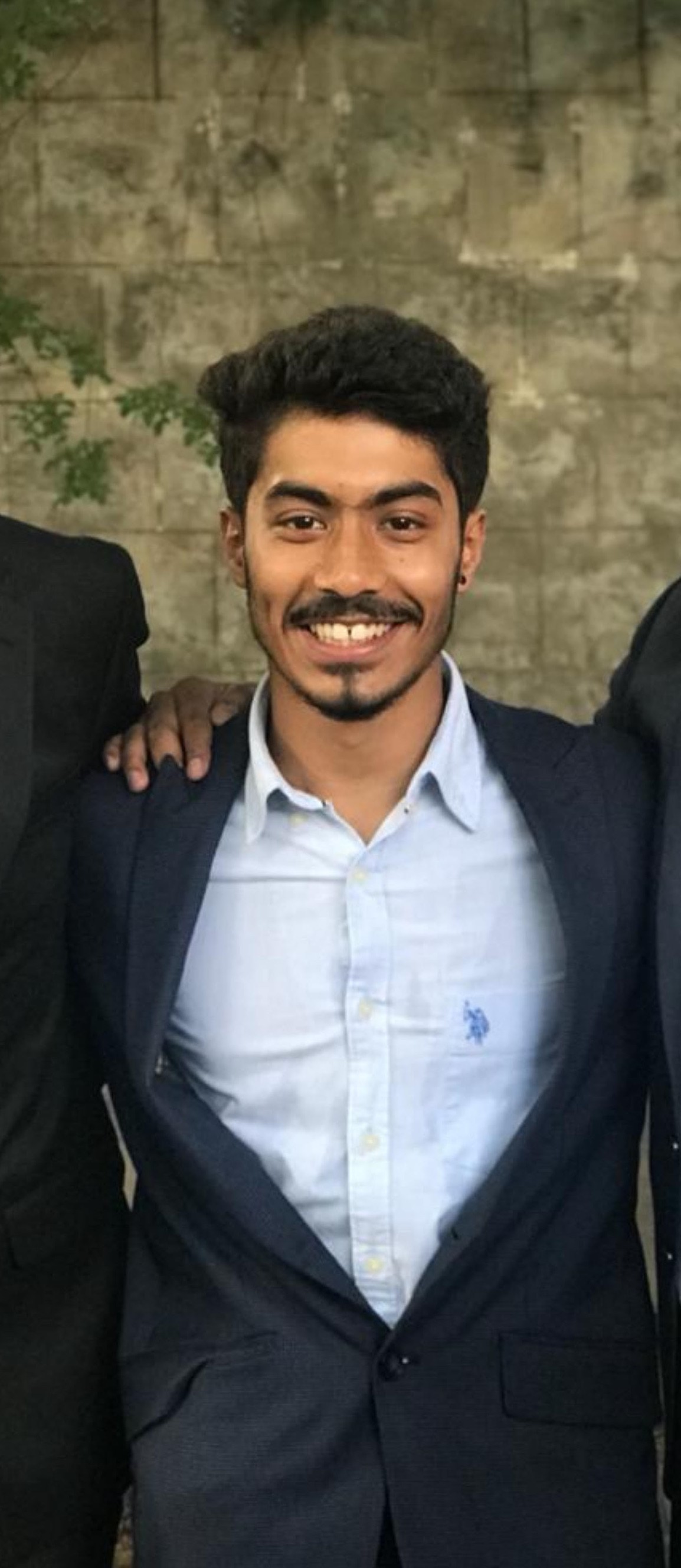
Extreme precipitation, like floods and landslides, poses major risks to safety and the economy, underscoring the need for sophisticated weather forecasting to predict these events accurately, enhancing readiness and resilience. Nowcasting, which uses real-time atmospheric data to predict short-term weather, is key in addressing this challenge. Traditional nowcasting systems, reliant on extrapolation from rainfall radar observations and constrained by simplistic physical assumptions, often struggle to detect complex, nonlinear weather patterns. This gap has opened the door for deep learning models, which have shown significant promise in improving the accuracy and reliability of short-term weather predictions, making them a focal point of recent research and the basis of this thesis's approach.
This thesis introduces a deep generative model designed for the nowcasting of extreme precipitation events up to 3 hours ahead, utilizing a Vector-Quantized Variational Autoencoder (VQ-VAE) to compress radar data into a low-dimensional latent representation, and an Autoregressive Transformer for predicting future radar images. Additionally, a binary classifier works in conjunction with the Autoregressive Transformer to identify extreme versus non-extreme weather events, using these classifications to inform an Extreme Value Loss (EVL) function. This loss function aims to improve the accuracy of predicting extreme weather events by addressing the data imbalance between normal and extreme precipitation occurrences. The proposed model displays comparable performance with the state-of-the-art conventional methods and other deep learning nowcasting models in predicting extreme events.
Signal Processing Seminar
- Thursday, 7 March 2024
- 11:00-12:00
- HB 17.140
Robust Tensor Decomposition Methods for Denoising Speckle Noise
Metin Çalış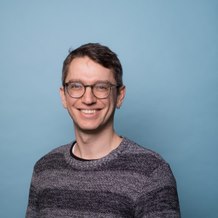
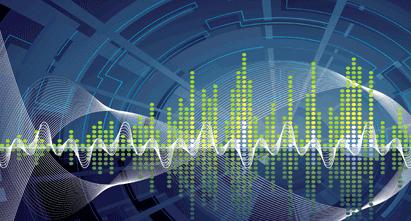
Signal Processing Seminar
- Thursday, 29 February 2024
- 11:00-12:00
- HB 17.140
Cauchy-Schwarz Divergence Information Bottleneck for Regression
Shujian YuDepartment of Artificial Intelligence, VU, Amsterdam
The information bottleneck (IB) approach is popular to improve the generalization and robustness of deep neural networks. Essentially, it aims to find a minimum sufficient representation t from input variable x that is relevant for predicting desirable response variable y, by striking a trade-off between I(x;t) and I(y;t), where I refers to the mutual information (MI). However, optimizing IB remains a difficult problem. In this talk, we study the IB principle for the regression problem and develop a new way to parameterize IB with deep neural networks, by leveraging the favorable properties of the Cauchy-Schwarz (CS) divergence. By doing so, we move away from the mean squared error (MSE) loss-based regression and ease estimation of MI terms by avoiding variational approximations or distributional assumptions. We investigate the improved generalization ability of our proposed CS-IB and demonstrate strong adversarial robustness guarantee. We observe that the solutions discovered by CS-IB always achieve the best trade-off between prediction accuracy and compression ratio. We additionally extend CS-IB to structured data such as graphs, and demonstrate its effectiveness to predict the age of patients based on their brain functional MRI (fMRI) data with a graph neural network.
Additional information ...
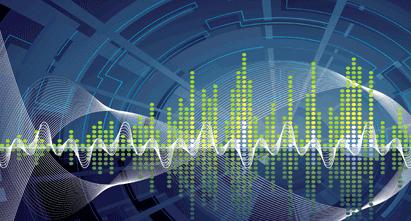
Signal Processing Seminar
- Thursday, 22 February 2024
- 11:00-12:00
- HB 17.140
Cooperative Collision Avoidance for Multi-agent Systems
Ellen Riemens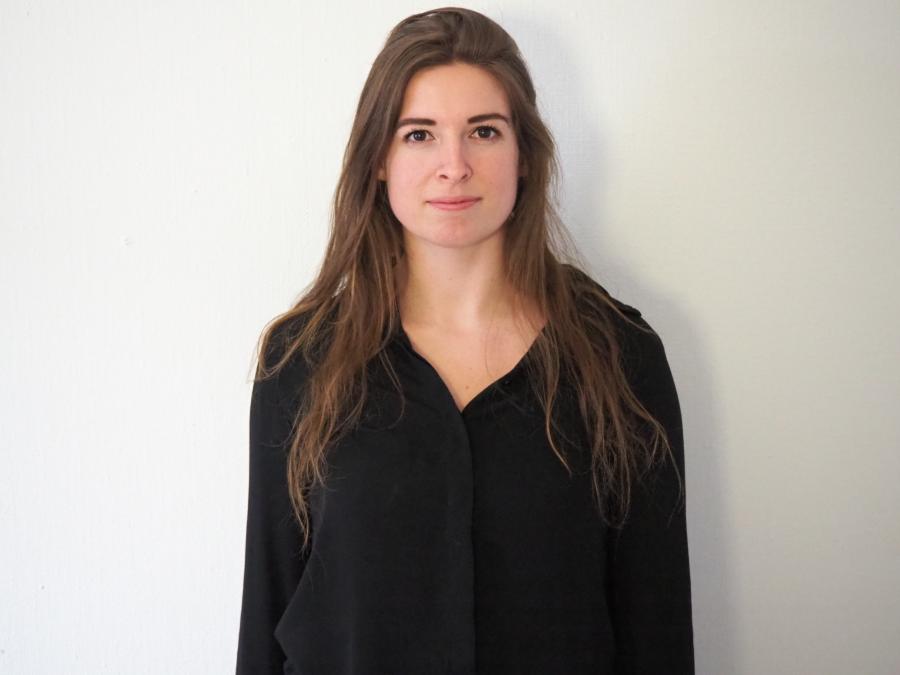
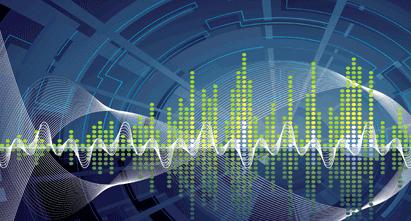
MSc SPS Thesis presentation
- Monday, 29 January 2024
- 13:31-15:30
- HB17.140 (Seminar room)
Towards Robust Object Detection in Unseen Catheterization Laboratories
Zipeng Wang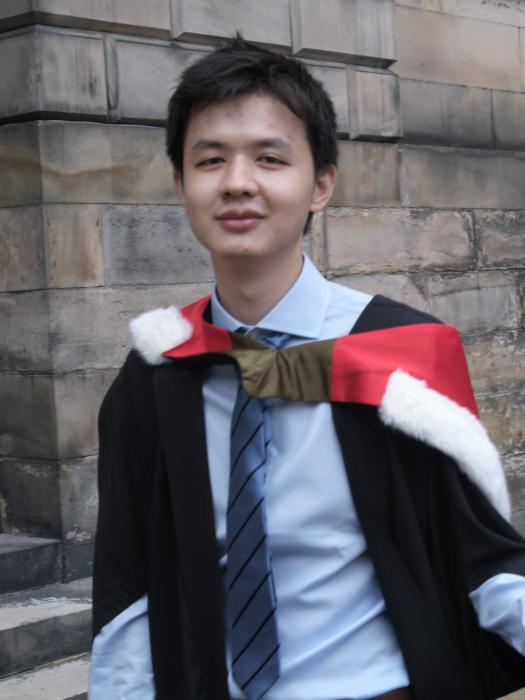
Deep-learning-based object detectors, while offering exceptional performance, are data-dependent and can suffer from generalization issues. In this thesis, we investigated deep neural networks for detecting people and medical instruments in the vision-based workflow analysis system inside Catheterization Laboratories (Cath Labs). The central problem explored in this thesis is the fact that the performance of the detector can degrade drastically if it is trained and tested on data from different Cath Labs.
Our research aimed to investigate the underlying causes of this specific performance degradation and find solutions to mitigate this issue. We employed the YOLOv8 object detector and created datasets from clinical procedures recorded at Reinier de Graaf Hospital (RdGG) and Philips Best Campus, supplemented with publicly accessible images. An aggregated version of object detection metrics was created for multi-camera system evaluation. Through a series of experiments complemented by data visualization, we discovered that the performance degradation primarily stems from data distribution shifts in the feature space. Notably, the object detector trained on non-sensitive online images can generalize to unseen Cath Labs, outperforming the model trained on a procedure recording from a different Cath Lab. The detector trained on the online images achieved an mAP@0.5 of 0.517 on the RdGG dataset. Furthermore, by switching to the most suitable camera for each object, the multi-camera system can further improve detection performance significantly. An aggregated 1-camera mAP@0.5 of 0.679 is achieved for single-object classes on the RdGG dataset.
THz Symposium
- Thursday, 25 -- Thursday, 25 January 2024
- 09:30-17:00
- Lecture Hall D@ta, 36.HB.01.630
Dutch Symposium on Terahertz Science and Technology - 3rd Edition

You are cordially invited to join us for the third edition of the Dutch Terahertz Symposium on January 25, 2024. It is a co-organized event between the Delft and Eindhoven University of Technology. With these series of symposiums, we aim to create an environment to exchange information and allow the Dutch Terahertz community to find collaborations and funding opportunities.
The 2024 Dutch Terahertz Symposium aims to bring together the Dutch research community and industry interested in the field of terahertz science and technology. There will be six featured talks from both academic and industrial institutions about the latest developments in the field. You can find the list of speakers and topics attached to this email. There will be plenty of opportunities for interaction with each other and inspired further community building.
Save the date to join a full day of interesting presentations, Q&As, and panel discussions!
Additional information ...
Signal Processing Seminar
- Thursday, 25 January 2024
- 11:00-12:00
- HB 17.140
The Virtual Array: A Possible Use Case in Array Design
Costas Kokke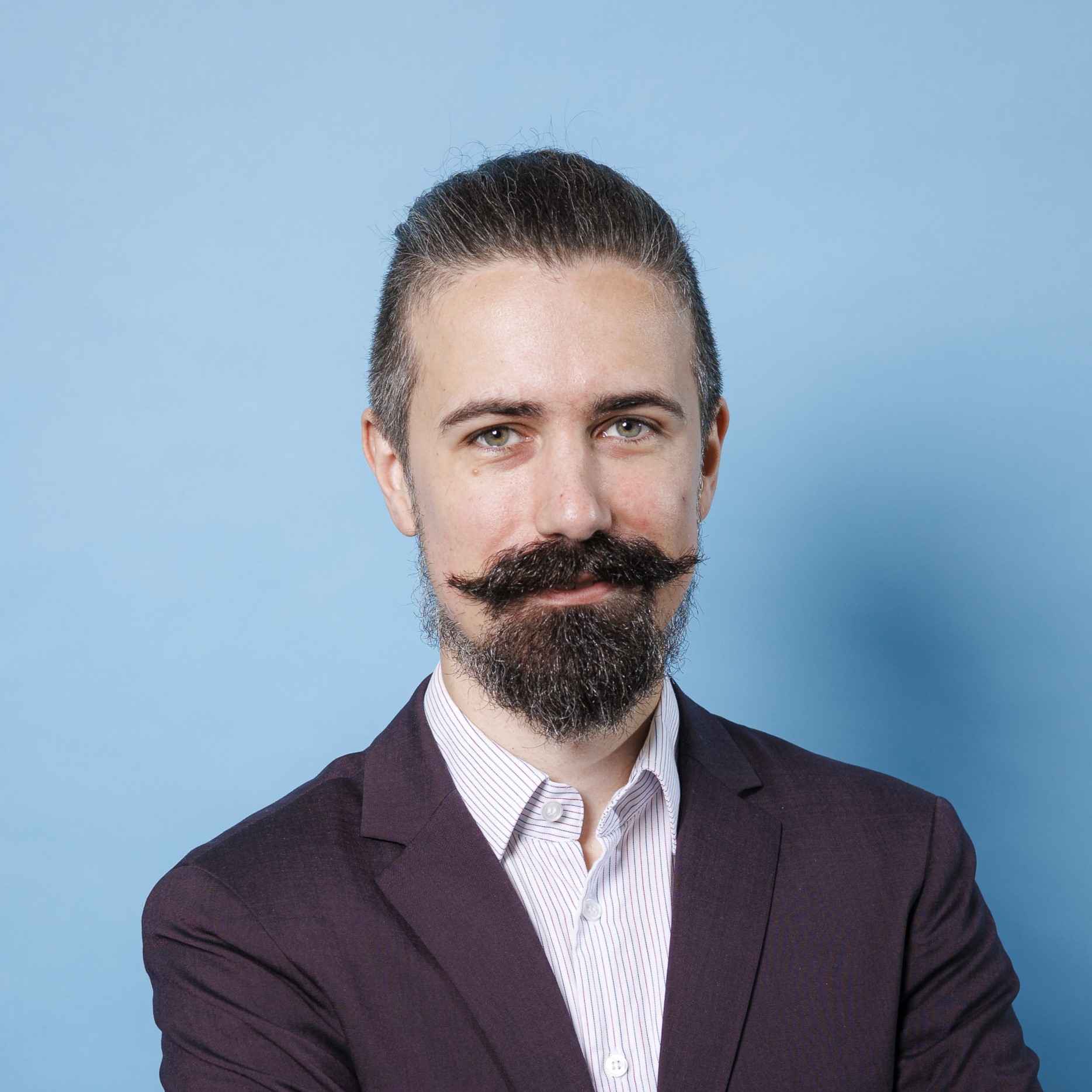
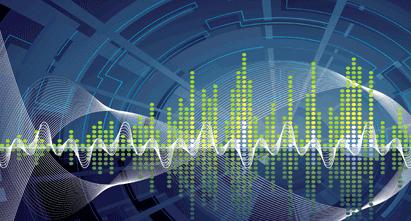
Physics-assisted multi-robot exploration of spatio-temporal dispersal phenomena
- Tuesday, 23 January 2024
- 17:00-18:00
Physics-assisted multi-robot exploration of spatio-temporal dispersal phenomena
Dmitry Shutin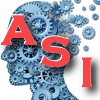
The problem of exploring a dispersal of a potentially hazardous or toxic material in air using robots has a number of applications for e.g., environmental monitoring, infrastructure inspection, or civil protection, to name only a few. Especially in situations when explored substances pose a health risk to human operators, autonomous solutions are of a great interest. However, the key challenge that arises on a path towards autonomy in this context is a rather complicated dynamics of the dispersed material, coupled with specifics of spatial aperture and low temporal resolution of olfactory (chemical) sensors used for perception. While the former precludes tele-operation (or makes it rather challenging), the latter requires perception and autonomy schemes that are able to cope with very low information rate acquired through olfactory sensing. To address these challenges the proposed solution incorporates two elements that will be discussed in this talk. First, a mobile swarm of robotic sensor carriers is used to increase spatial sampling, and thus capture spatial dynamics more efficiently. Second, a prior information about the dispersal process in terms of domain-specific knowledge is used to support data processing and autonomy. Specifically, the dispersal process is modeled with an advection-diffusion partial differential equation (PDE). The advection, or plainly speaking, the wind – a dominant transport mechanism in a majority of practically relevant applications – is modeled using Navier-Stockes equations and explored with a robotic swarm. Furthermore, using a probabilistic (Bayesian) formulation of the PDE models, the resulting representation can be relaxed to additionally allow for more control over model mismatches. Using data samples collected by multiple robots, the multi-robot exploration then includes two steps: (i) a cooperative solution to an inverse problem of identifying parameters of the PDEs given measurements, and (ii) exploration – the design of an optimal sampling scheme for multiple robotic platforms. This work will describe the used models, discuss the developed probabilistic inference schemes, their advantages and limitations, as well as demonstrate their performance in simulations and in experiments.
Multi-feature-based Automatic Targetless Camera-LiDAR Extrinsic Calibration
- Thursday, 18 January 2024
- 10:00
- HB20.150 (ME Meeting room)
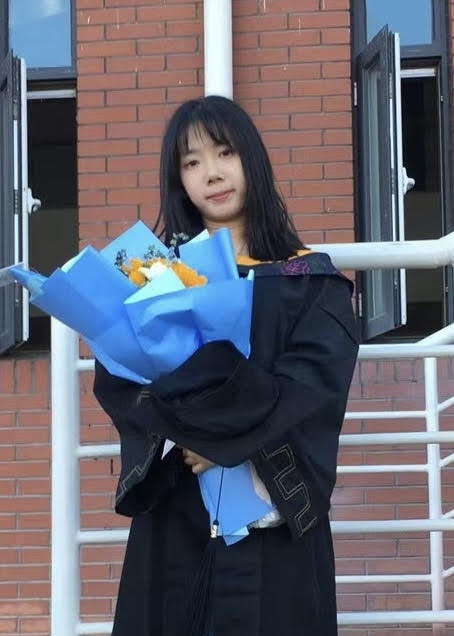
In autonomous driving, environmental perception, crucial for navigation and decision-making, depends on integrating data from multiple sensors like cameras and LiDAR. Camera-LiDAR fusion combines detailed imagery with precise depth, improving environmental awareness. Effective data fusion requires accurate extrinsic calibration to align camera and LiDAR data under one coordinate system. We aim to calibrate the camera and LiDAR extrinsic automatically and without specific targets. Targetless, non-automated calibration methods are time-consuming and labor-intensive. Existing advanced methods have proven that automatic calibration methods based on edge features are effective, and most focus on the extraction and matching of single features. The proposed method matches 2D edges from LiDAR's multi-attribute density map with image-derived intensity gradient and semantic edges, facilitating 2D-2D edge registration. We innovate by incorporating semantic feature and addressing random initial setting through the PnP problem of centroid pairs, enhancing the convergence of the objective function. We introduce a weighted multi-frame averaging technique, considering frame correlation and semantic importance, for smoother calibration. Tested on the KITTI dataset, it surpasses four current methods in single-frame tests and shows more robustness in multi-frame tests than MulFEAT.
Our algorithm leverages semantic information for extrinsic calibration, striking a balance between network complexity and robustness. Future enhancements may include using machine learning to convert sparse matrices to dense formats for improved optimization efficiency.
Signal Processing Seminar
- Thursday, 14 December 2023
- 11:00-12:00
- HB 17.140
Error-correcting codes and cryptography: from theory to practice
Xinmiao ZhangOhio State University
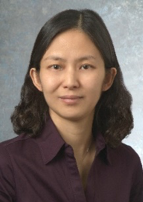
Error-correcting codes (ECCs) and cryptography schemes are indispensable to the reliability and security of numerous classic and emerging systems. Advanced ECCs play essential roles in the performance of 5G/6G wireless communications, hyper-scale distributed storage, in/near-memory computing, and quantum computing. They are also key enablers of high-density next-generation memories and wafer-scale integration, which are important pillars of the CHIPS Act. With the development of new technologies, such as quantum computers and cloud computing, traditional cryptography schemes are no longer secure and/or pose privacy concerns. There are imminent needs for post-quantum cryptography and homomorphic encryption for privacy-preserving cloud computing. Protecting the circuit chips implementing advanced functions from counterfeiting is also necessary to preserve the semiconductor supremacy achieved by the CHIPS Act.
This talk presents our recent contributions on related topics. Efficient and high-speed generalized integrated interleaved ECC decoders are developed to meet the tight latency and excellent error-correcting capability requirements of next-generation hyper-speed memories. Code construction and decoder designs are jointly optimized to enable low-latency failure recovery and continued scaling of distributed storage. Our generalized logic locking includes many previous designs as special cases and achieves better resilience towards various attacks. Logic locking that can effectively protect chips implementing fault-tolerant functions, such as machine learning, are also developed by exploiting algorithmic specifics. Last by not least, high-speed and low-complexity hardware accelerators are designed for homomorphic encryption and post-quantum cryptography.
Biography
Xinmiao Zhang received her Ph.D. degree in Electrical Engineering from the University of Minnesota. She is currently a Professor at the Ohio State University. She was a Senior Technologist at Western Digital/SanDisk 2013-2017. Prior to that, she was a Timothy E. and Allison L. Schroeder Associate Professor at Case Western Reserve University. Prof. Zhang’s research spans the areas of VLSI architecture design, digital storage and communications, cryptography, security, and signal processing.
Prof. Zhang is a recipient of the NSF CAREER Award 2009, the College of Engineering Lumley Research Award at The Ohio State University 2022, the Best Paper Award at ACM Great Lakes Symposium on VLSI 2004, and Best Paper Award at International SanDisk Technology Conference 2016. She authored “VLSI Architectures for Modern Error-Correcting Codes” (CRC Press, 2015), and co-edited “Wireless Security and Cryptography: Specifications and Implementations” (CRC Press, 2007). Prof. Zhang was elected the Vice President-Technical Activities of the IEEE Circuits and Systems Society (CASS) 2022-2023 and served on the Board of Governors of CASS 2019-2021. She was also the Chair (2021-2022) and a Vice-Chair (2017-2020) of the Data Storage Technical Committee (DSTC) of the IEEE Communications Society. She served on the technical program and organization committees of many conferences, including ISCAS, ICC, GLOBECOM, SiPS, GlobalSIP, MWSCAS, and GLSVLSI. She has been an Associate Editor for the IEEE Transactions on Circuits and Systems-I (TCAS-I) 2010-2019 and IEEE Open Journal of Circuits and Systems since 2019. She will be the Associate Editor-in-Chief of TCAS-I 2024-2025.
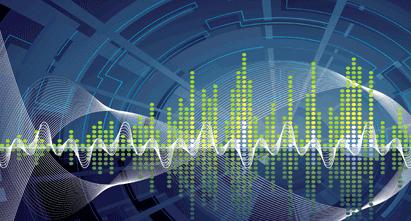
Signal Processing Seminar
- Friday, 8 December 2023
- 14:00-15:00
- HB 17.140
Future of Automotive Radar and Signal Processing Challanges
Tarik Kazaz, Juan Osorio, Karan JayachandraNXP Semiconductors, Eindhoven

The last decade has seen a rapid adoption of automotive radars to improve the safety of vehicles and to enable a higher level of driving autonomy. Even though major progress has been achieved in this effort, high-level automation has remained partially evasive due to the insufficient resolution of automotive radars.
In this presentation, we will be discussing several of the proposals to tackle those challenges, specifically the ones related to digitally modulated radars. The presentation will start with a brief discussion of the evolution of automotive radar and the alternatives to increase angular resolution, we will be focusing on the opportunities and challenges we envision for the adoption of imaging radars and specifically digitally modulated from the perspective of signal processing. Lastly, the presentation will finish with a live demonstration of one of the NXP corner radar sensors.
Presenters: Tarik Kazaz, Juan Osorio, Karan Jayachandra
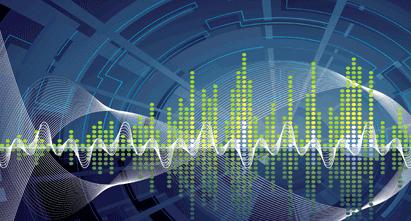
MSc SPS Thesis presentation
- Friday, 1 December 2023
- 14:00-16:00
- Lecture hall Boole
Compressed Sensing in Low-Field MRI: Using Multiplicative Regularization
Marien VerseputLUMC
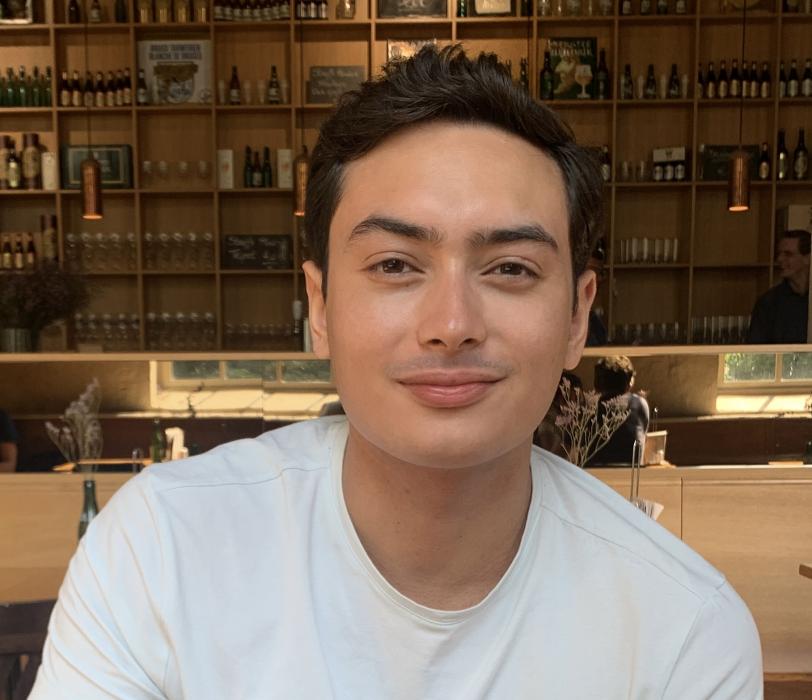
Magnetic resonance imaging (MRI) is a non-invasive tool to image the body’s anatomy and physiology, but suffers from long scan times. Compressed Sensing (CS) is used to accelerate MRI scans by incoherently taking fewer measurements and using a nonlinear optimization algorithm to image the undersampled data. Convex optimization techniques are generally used for image reconstruction. Minimizing a data fidelity term along with two regularization terms, which are a total variation (TV) based and a wavelet transform based function, is a standard procedure in CS-MRI. Regularization parameters are needed to balance the different terms, but it is impossible to know upfront what the optimal regularization parameters are to get the desired output. A consequence is that the algorithm of choice needs to be executed many times for many different values of the regularization parameters, which is a time-consuming process requiring knowledge of the algorithm.
In this work we rewrite and implement the regularization functions in a multiplicative manner by multiplying the data fidelity term with the regularization terms, thereby eliminating the need to tune the regularization parameters. Moreover, we include a region of support (ROS) mask to further accelerate reconstruction. The performance of different combinations of regularization functions and reconstruction algorithms are validated on a simulation study and various experiments on a low-field MRI scanner. This also shows the capability of CS applied to low-field MRI, which has lower signal-to-noise ratio compared to conventional MRI. Of all proposed methods, a nonlinear conjugate gradient method applied to the fully multiplicatively regularized objective function shows the most robust performance.
MSc SPS Thesis presentation
- Monday, 27 November 2023
- 14:00
- HB17.140 (Seminar room)
Sparse Millimeter Wave Channel Estimation From Partially Coherent Measurements
Weijia Yi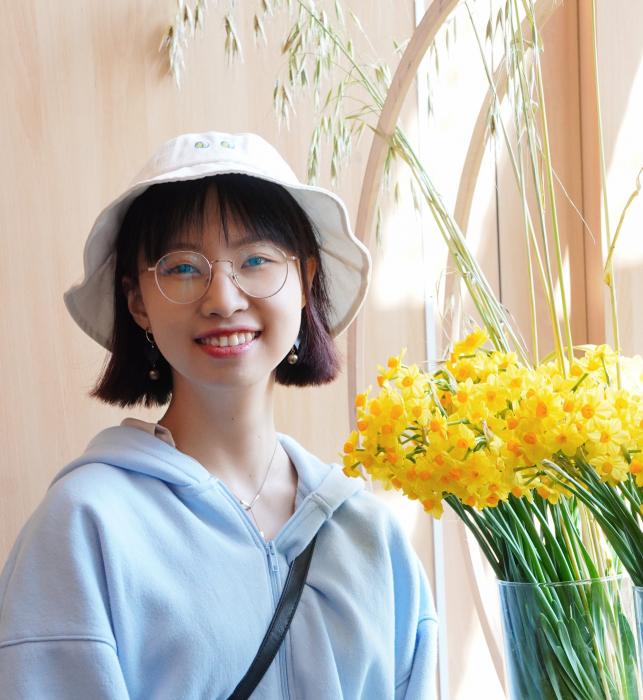
“This project develops a channel estimation technique for millimeter wave (mmWave) communication systems. Our method exploits the sparse structure in mmWave channels for low training overhead and accounts for the phase errors in the channel measurements due to phase noise at the oscillator. Specifically, in IEEE 802.11ad/ay-based mmWave systems, the phase errors within a beam refinement protocol packet are almost the same, while the errors across different packets are substantially different.
We show that standard compressed sensing algorithms that treat phase noise as a constant fail when channel measurements are acquired over multiple beam refinement protocol packets. Most of the methods that have addressed this problem treat phase noise as purely random, missing the inherent structure within the measurement packets. We present a novel algorithm called partially coherent matching pursuit for sparse channel estimation under practical phase noise perturbations. The proposed approach leverages this partially coherent structure in the phase errors across multiple packets. Our algorithm iteratively detects the support of sparse signal and employs alternating minimization to jointly estimate the signal and the phase errors.
We numerically show that our algorithm can reconstruct the channel accurately at a lower complexity than the benchmarks, and derive a preliminary support detection bound as a performance guarantee.”
Microelectronics Colloquium
- Thursday, 23 November 2023
- 15:30-16:30
- EWI Snijderszaal (LB 1.010)
Neuromorphic On-Device Intelligence for Energy-Efficient AIoT
Chang Gao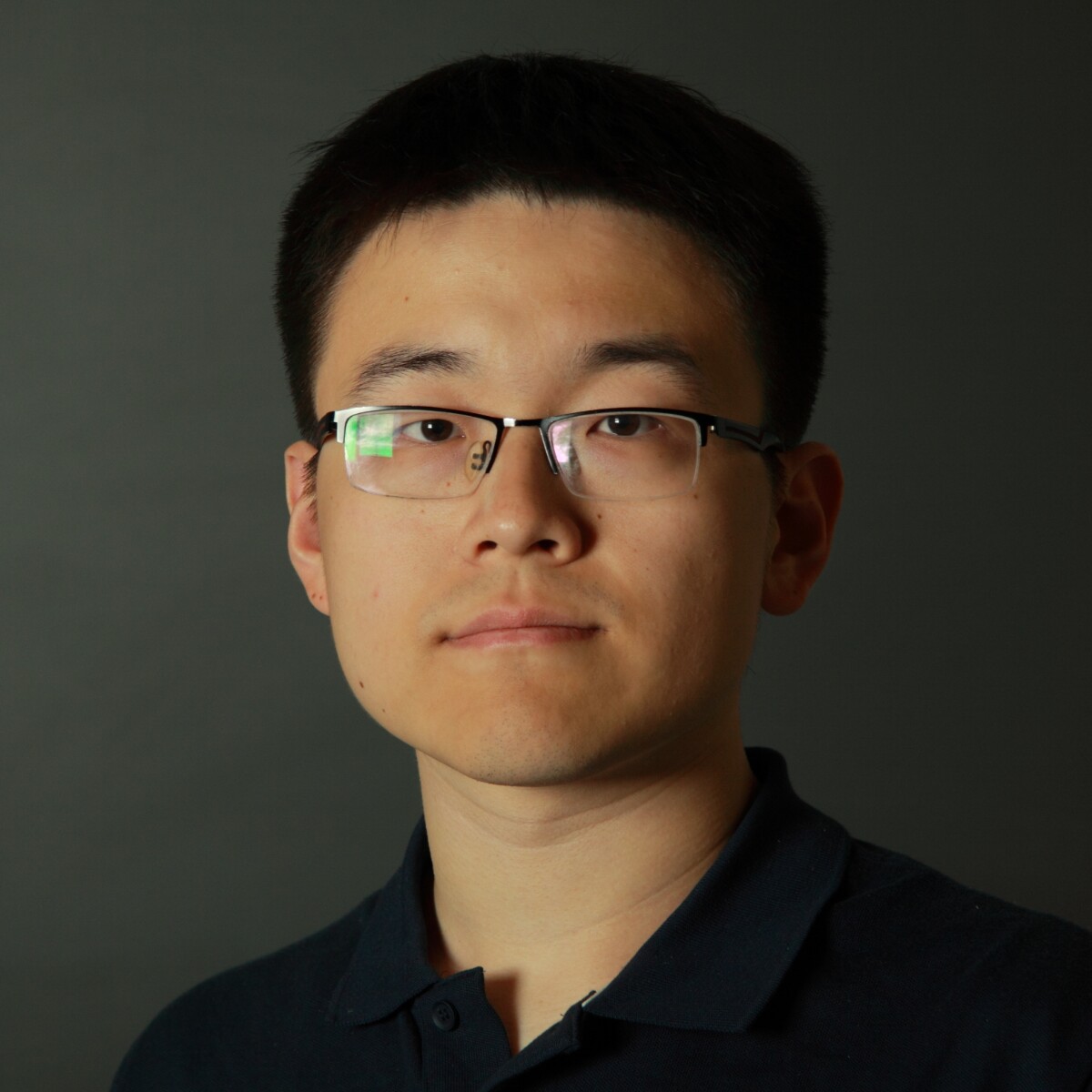
In the swiftly advancing realm of Artificial Intelligence of Things (AIoT), the integration of edge smart devices and communication networks is becoming increasingly central to our digital infrastructure. In this context, the need for energy-efficient computing—characterized by low latency and low power consumption—is paramount, not only to enhance user experience across various AIoT applications but also to contribute to carbon neutrality. Neuromorphic computing emerges as a promising, sustainable solution, offering both efficiency and effectiveness. This presentation will delve into our recent research in neuromorphic computing, focusing on its application in speech recognition, eye tracking, and robotic control. Our work underscores the potential of neuromorphic technology to substantially reduce latency and energy consumption while managing complex tasks with negligible accuracy loss. In addition, we will delve into the application of AI for the correction of non-linearity in wideband RF power amplifiers, a critical aspect of advanced RF signal processing for emerging 6G and WiFi 7 technologies vital for connecting data-intensive AIoT devices in the future. By integrating neuromorphic computing, we aim to make AIoT devices more accessible, thereby enhancing the quality of life and fostering a sustainable, environmentally friendly future.
Additional information ...
MSc SPS Thesis presentation
- Friday, 17 November 2023
- 14:00-16:30
- HB20.150 (ME Meeting room)
Subgraph Matching via Fused Gromov-Wasserstein Distance
Wenxin Pan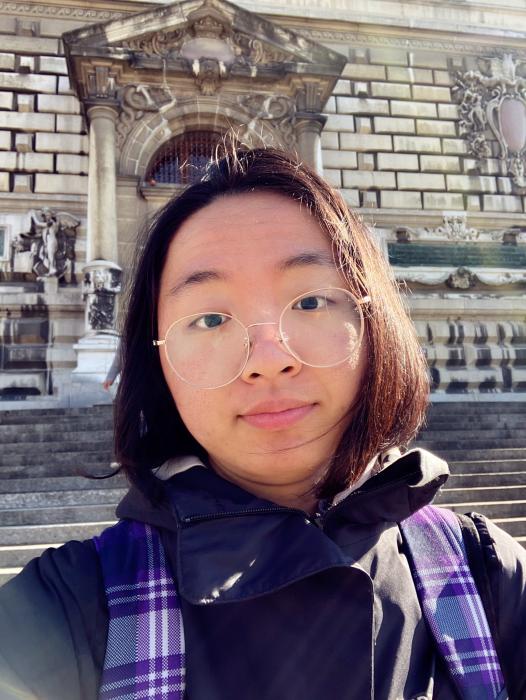
Subgraph matching is a fundamental problem in various fields such as machine learning, computer vision, image processing, and bioinformatics, where detecting specific substructures within an object is often crucial. In these domains, not only structure plays an essential role, but also the feature information on nodes should be incorporated, thus highlighting the necessity for comprehensive analytical approaches.
In this thesis, we propose two novel subgraph matching frameworks using the Fused Gromov-Wasserstein (FGW) distance, namely the Subgraph Optimal Transport (SOT) and the Sliding Subgraph Optimal Transport (SSOT). Both frameworks integrate a dummy node strategy to handle the discrepancy between two graphs of different sizes. The SSOT extends upon the SOT by incorporating a sliding window framework and Wasserstein pruning to enhance the performance, especially for sparse large graphs. Our frameworks can be easily implemented and are adaptable for problems of exact matching, top-k approximate matching, and inexact matching.
We further propose a normalized FGW distance to cater to the practical interests and enhance the performance evaluation. We adopt the Frank-Wolfe algorithm for optimization and develop computation-reducing techniques by isolating the dummy node.
By conducting experiments on both synthetic and real-world datasets, we demonstrate that the SOT method achieves excellent performance on small graphs, and the SSOT method improves the accuracy over the SOT on large graphs. Both these two methods show the ability to outperform the state-of-the-art methods in noisy environments in terms of accuracy and efficiency.
Microelectronics Research Day 2023
- Thursday, 9 November 2023
- 09:30-17:30
- EEMCS
Microelectronics Research Day 2023

TU Delft Microelectronics Research Day
Fully booked!
Registering 2023 not for possible anymore
MSc SPS Thesis presentation
- Tuesday, 7 November 2023
- 14:00-16:00
- HB17.140 (Seminar room)
Malleable Kernel Interpolation for Scalable Structured Gaussian Process
Hanyuan Ban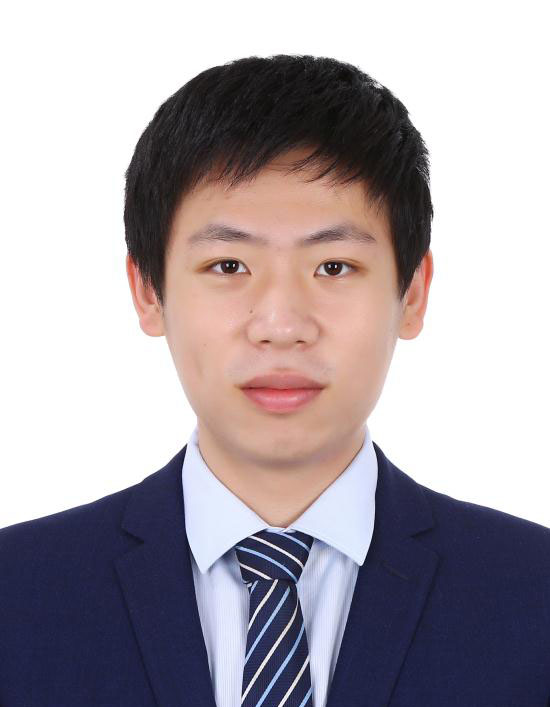
Gaussian process regression (GPR), a potent non-parametric data modeling tool, has gained attention but is hindered by its high com- putational load. State-of-the-art low-rank approximations like struc- tured kernel interpolation (SKI)-based methods offer efficiency, yet lack a strategy for determining the number of grid points, a pivotal factor impacting accuracy and efficiency. In this thesis, we tackle this challenge.
We explore existing low-rank approximations that facilitates the computation, dissecting their strengths and limitations, particularly SKI-based methods. Subsequently, we introduce a novel approxima- tion framework, MKISSGP, which dynamically adjusts grid points us- ing a new hyperparameter of the model: density, according to changes in the kernel hyperparameters in each training iteration.
MKISSGP exhibited consistent error levels in the reconstruction of the kernel matrix, irrespective of changes in hyperparameters. This robust performance forms the bedrock for achieving accurate approx- imations of kernel matrix-related terms. When employing our rec- ommended density value (i.e., 2.7), MKISSGP achieved a comparable level of precision to that of precise GPR, while requiring only 52% of the time compared to the current state-of-the-art method.
Additional information ...
MSc SPS Thesis presentation
- Friday, 27 October 2023
- 10:00
- HB17.140 (Seminar room)
ADS-B Based Trajectory Prediction for Aerial Vehicles
Haobo Wang
The evolution of aerial vehicle technology necessitates robust trajectory prediction models. These models are crucial for maintaining safe airspace and enabling autonomous operations. Automatic dependent surveillance–broadcast (ADS-B) is a surveillance system that enables aircraft to receive data from navigation satellites and periodically broadcasts it, enabling it to be tracked. Moreover, using ADS-B data for more general aerial vehicles has become a popular trend because it can provide real-time high-resolution aircraft state information and share this information with other vehicles in real-time for the aviation safety ecosystem.
In this project, we delve into ADS-B-based trajectory prediction for both aircraft and drone motion trajectories with the overarching goal of improving prediction accuracy. We initially implement several model-based
Kalman filters—including interactive multiple models (IMM)—to assess the accuracy of aircraft trajectory predictions across different model structures. The results reveal that the IMM filter outperforms the single model predictions in terms of root mean square error (RMSE).
Furthermore, we implement the Gaussian process (GP) with a sliding window scheme to predict online drone trajectories. Recognizing the high computational complexity of the GP, we also introduce a low-rank
approximation method, structured kernel interpolation (SKI) GP, aiming to conserve computational resources. Finally, we compare the prediction performances of the IMM filter, classical GP, and SKI GP on real drone
trajectories. The results highlight that the classical GP method enhanced prediction accuracy, achieving an RMSE of less than 1.7m, which is 50% lower compared to the model-based IMM filter. Additionally, the SKI GP
realizes a 25% reduction in computation time compared to the classical GP, despite a slight compromise in prediction accuracy.
ME colloquium
- Thursday, 26 October 2023
- 15:30-16:30
- EEMCS, Snijderszaal
Generative AI
Justin Dauwels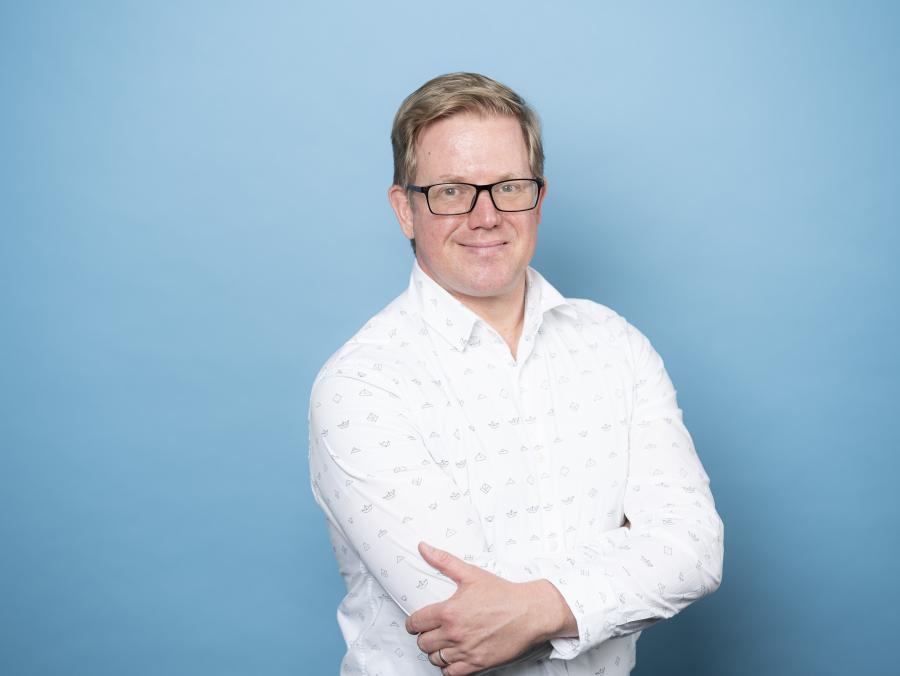
Generative AI
Generative AI refers to a category of artificial intelligence models that are designed to generate new content, such as text, images, audio, or other types of data. Probably the best known example of generative AI is ChatGPT, the fastest consumer application to hit 100 million monthly active users. Generative AI models use machine learning algorithms to learn patterns and structures from existing data and then produce new data that is similar in style or content to what they have been trained on.
In this presentation, I will talk about projects in our group on deep generative models. I will briefly present novel kinds of deep generative models that we are developing in our team. Next I will explain how we are designing such models for rainfall nowcasting, where we integrate physical laws into the deep generative models. At last, I will talk recent AI related initiatives that my team is involved in.
Additional information ...
MSc SS Thesis Presentation
- Thursday, 26 October 2023
- 10:00-10:45
- HB 20.150
Enhancing Fiber Direction Estimation from Electrograms
Elena van Breukelen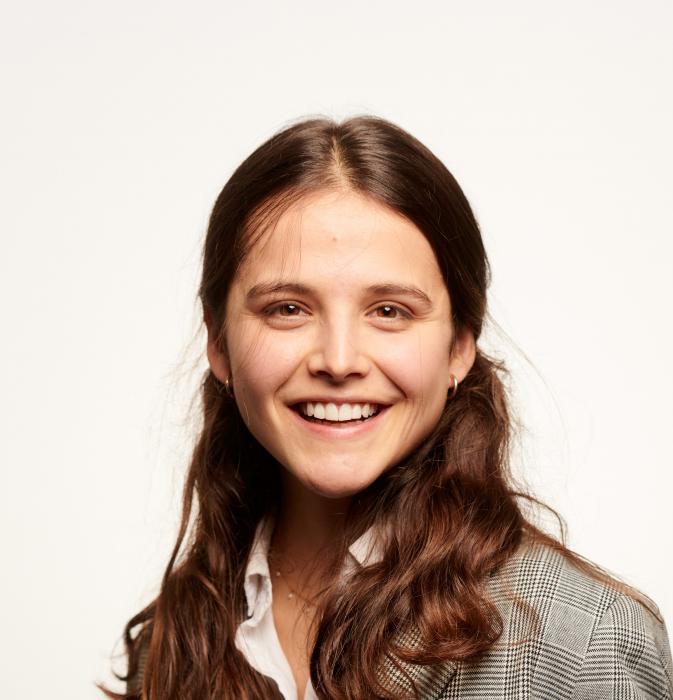
For the heart to pump blood throughout the body, electrical impulses that trigger the cellular contraction must be generated and spread through the myocardial tissue. These signals propagate faster along the longitudinal cardiac fiber direction than the transverse direction, conferring the heart with anisotropic conduction properties. Therefore, the arrangement of the fibers within the tissue governs the impulse propagation. Given the variability of the fiber direction across the heart and between patients, incorporating it into electrophysiological models would enhance our understanding of the mechanisms and progression of different heart conditions, such as atrial fibrillation (AF). The study of this common cardiac arrhythmia relies on analyzing electrical recordings of the heart, known as electrograms (EGMs), which, if integrated with the patient’s fiber architecture into cardiac models, can enable effective personalized treatment. Over the years, researchers have proposed different approaches to estimate the fiber direction from EGMs. However, these methods have been evaluated in different, usually simplistic, cardiac tissue models, making their comparison, and therefore selection of the most accurate approach for clinical and research applications, challenging.
The current study aims to identify the best fiber direction estimation method under consistent and realistic conditions. To achieve this goal, synthetic EGMs and local activation time (LAT) maps were generated from 2D and 3D monodomain models that mimicked the muscle bundle, atrial bilayer, and ventricular transmural fiber rotation structures. A comparison analysis of existing fiber direction estimation methods, first as described by their authors and then standardized to have the same spatial resolution, showed the superior performance of the techniques based on fitting an ellipse to local conduction velocity or conduction slowness vectors from a whole LAT map. The estimation accuracy of these methods can be further improved by increasing the number of vectors to which the ellipse is fitted. Nonetheless, given the influence of underlying layers in the epicardial recordings, the estimation error increases in the tissue models where fibers in the epicardial and endocardial layers run perpendicularly. The effect on the estimate of such architecture, characteristic of the inferior side of the right atria and the ventricles, can be accounted for by combining epicardial electrical recordings obtained after pacing either in the endocardium or the epicardium. Although a preliminary assessment of the estimation methods was carried out with human EGMs, future studies should focus on validating the methods in a controlled experimental framework and refining them for more localized fiber direction estimation. All in all, the automation of the techniques and their integration into electrophysiological models brings us a step closer to creating valuable clinical tools for diagnosing and treating electropathologies.
Additional information ...
PhD Thesis Defence
- Wednesday, 27 September 2023
- 12:00-14:00
- Aula Senaatszaal
Pitch-Matched Integrated Transceiver Circuits for High-Resolution 3-D Neonatal Brain Monitoring
Peng Guo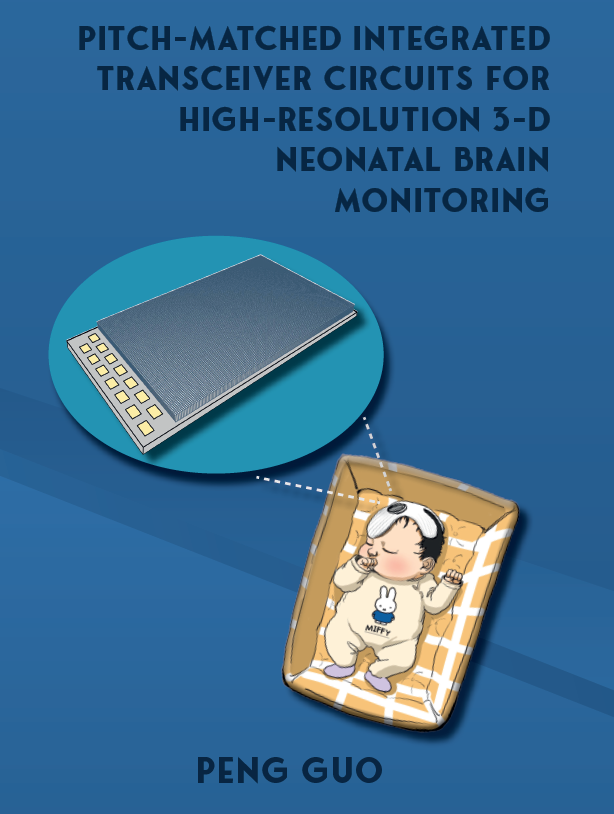
Peng Guo will defend his PhD thesis entitled
Pitch-Matched Integrated Transceiver Circuits for High-Resolution 3-D Neonatal Brain Monitoring
on Wednesday, Sept. 27th. The layman's talk will be at 12:00, the defence starts at 12:30.
The thesis can be downloaded from the TU Delft repository. Peng's work was part of the MIFFY project.
Livestream of the defence: link
Promotors: Michiel Pertijs and Nico de Jong
Abstract: This thesis presents the design and implementation of integrated ultrasound transceivers for use in transfontanelle ultrasonography (TFUS). Two generations of ultrasound transceiver ASICs integrated with PZT transducer arrays intended for TFUS are presented. In the first generation, a novel AFE design that combines an LNA with the continuous TGC function is realized in a bid to mitigate the gain-switching and T/R switching artifacts. Besides, a new current-mode micro-beamforming design based on boxcar integration (BI) is also implemented to reduce the channel count within a compact layout. In the second generation, the AFE is derived from the first version, while the design focuses on RX backend circuitry and channel-count reduction, including a passive BI-based µBF merged with a charge-sharing SAR ADC, which digitizes the delayed-and-summed signals, and a subsequent multi-level data link, which concatenates outputs of four ADCs. In total, a 128-fold reduction in channel count is finally achieved. The techniques we developed have established the groundwork and removed the initial barriers for an electronics architecture suitable for a wearable 3D TFUS device.
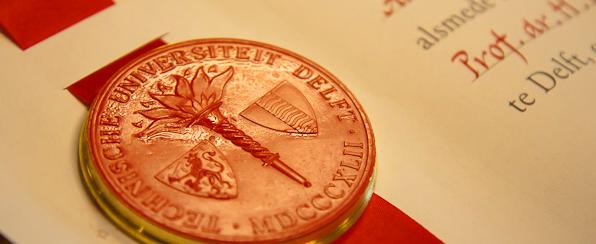
MSc SPS Thesis presentation
- Wednesday, 27 September 2023
- 10:00
- HB.20.150
Friction Identification on Gantry Stage
Lan Jia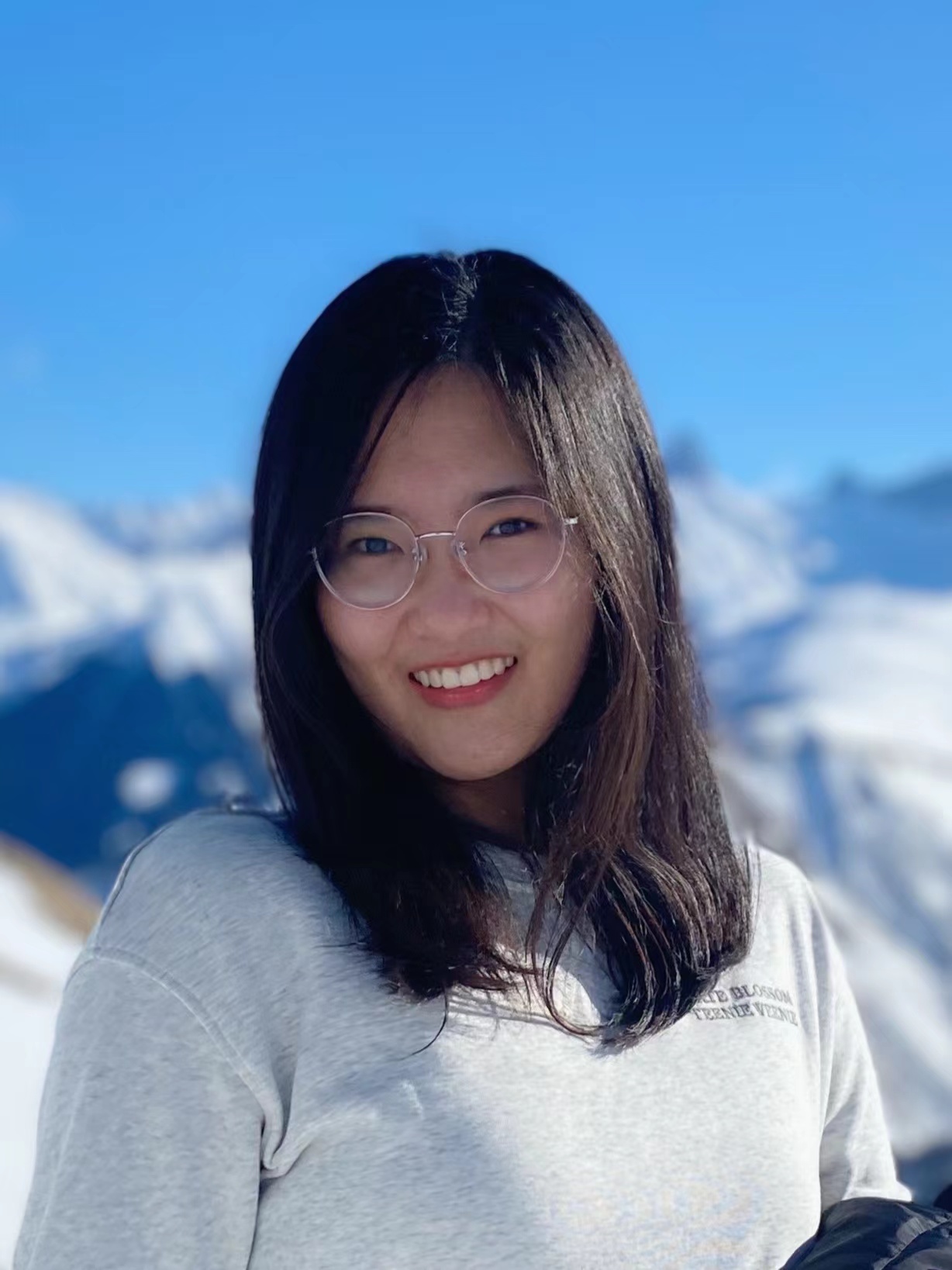
In an era marked by the demand for unprecedented levels of precision in engineering applications, the profound impact of friction forces on motion control systems cannot be underestimated. This thesis extensively investigates the frictional behavior of the Proton Motion Stage, an advanced high-precision motion control system developed by Prodrive Technologies. This research conducts both experimental investigations and computational simulations, offering valuable insights into its friction behavior across diverse conditions and scenarios.
The research begins with an analysis of existing models used to describe friction behavior in precision engineering systems. A critical evaluation of empirical models highlighting strengths and limitations is presented, and the LuGre friction model is selected for further research. Subsequently, the chosen model is used to simulate the behavior of the Proton Motion Stage. The simulation setup is described, including the incorporation of the LuGre friction model and the identification of system parameters. The accuracy of the identification is above 99%. The sensitivity analysis of the parameters is also conducted to enable a comprehensive exploration of friction dynamics. Finally, the research delves into static and dynamic parameter experiments, where cable slab forces' position-dependent impacts and velocity-friction maps that capture the intricate Stribeck effect are presented, and closed-loop and open-loop setups to dissect friction behavior during rapid motion changes are employed. Residual analysis of histogram and 90% confidence autocorrelation and cross-correlation is also presented to study the quality of identification. Overall, this thesis combines theory and practice to enhance our understanding of friction in precision engineering systems.
MSc SPS Thesis presentation
- Wednesday, 27 September 2023
- 10:00
- HB17.140 (Seminar room)
Small end-to-end OCR model
Jingwen Dun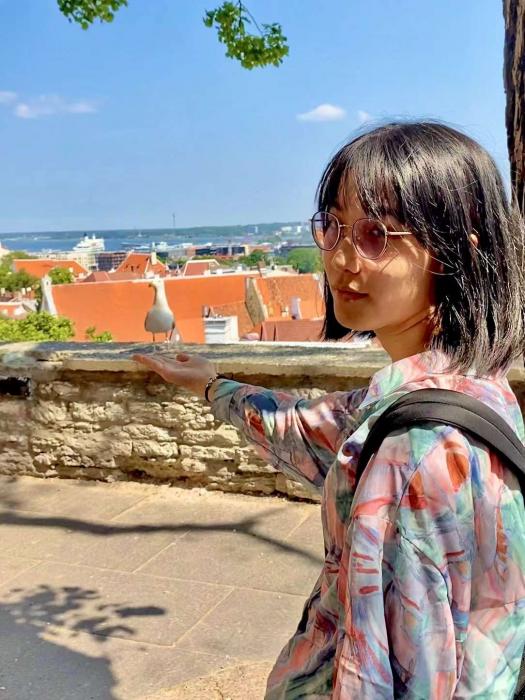
Optical Character Recognition (OCR) is a pivotal technology used to extract text information from images, finding wide-ranging applications in document digitization and medical records management. The integration of machine learning has ushered in an era of swift and precise OCR models. Broadly, OCR comprises two key components: detecting the bounding boxes around text instances and recognizing the characters within them. Presently, prevailing OCR models are primarily intricate two-stage systems necessitating real-time operation on remote servers. Nevertheless, end-to-end models exhibit superior performance from a data utilization perspective. There exist scenarios where offline models prove indispensable, such as in environments with restricted internet access or locales with stringent data privacy and security requirements.
This project delves into various end-to-end models, leveraging the PaddleOCR end-to-end model as a foundational reference to devise a compact OCR model tailored for edge devices. Through meticulous optimization of the backbone architecture and the introduction of diverse Feature Pyramid Network (FPN) structures within the stem network, we achieved a remarkable reduction in model size, down to 19MB. This represents a substantial advancement, constituting merely one-tenth of the original PaddleOCR end-to-end model's footprint.
By leveraging an extensive database and conducting a series of fine-tuning experiments specifically tailored for end-to-end OCR tasks involving curved text images, the model exhibits an impressive precision rate of 47.3% and an f-score of 45.3%. This achievement highlights the effectiveness of the customized loss function relative to the original model, despite its reduced size. Notably, this performance is comparable to certain end-to-end models with larger backbones. Furthermore, an Android demo has been carefully developed to demonstrate the model's capabilities on mobile devices, achieving an average processing time of 433 milliseconds per image.
MSc SPS Thesis presentation
- Wednesday, 20 September 2023
- 09:00-11:00
- HB.20.150
Efficient Content-Based Image Retrieval from Videos Using Compact Deep Learning Networks with Re-ranking
Doruk Barokas Profeta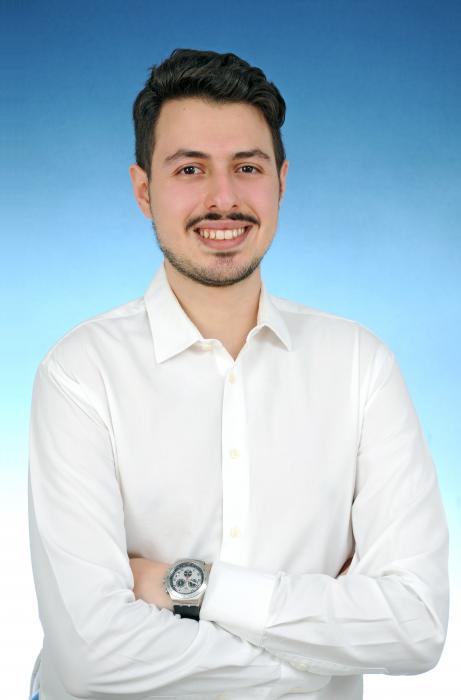
The rise of streaming and video technologies has underscored the significance of efficient access and navigation of digital content, particularly for scholars in fields like history and art. Scholars actively seek streamlined approaches to index, retrieve, and explore digital content, with a focus on locating specific instances. The process of searching for specific instances in video search is complex that requires the analysis of video sequences and the identification of relevant video segments. Advanced techniques and algorithms are necessary to ensure effective content-based retrieval of the required information.
In response to the escalating demand for accurate and swift access to relevant visual data within the vast spectrum of video resources, our research has been dedicated to the development of novel, efficient content-based image retrieval methods tailored for videos by integrating deep learning methodologies. Our comprehensive system contains two crucial components: keyframe extraction and content-based image retrieval. Keyframe extraction involves identifying significant frames within videos, while content-based image retrieval enables the retrieval of similar frames to a query image through feature extraction and ranking.
A unique aspect of our research lies in the exploration and analysis of a diverse range of feature extraction techniques derived from compact deep learning networks. We have compared our proposed method with state-of-the-art retrieval systems, evaluating performance metrics in terms of both accuracy and speed. Our method harnesses the power of compact deep learning network features in the initial ranking stage, effectively sublisting frames, and subsequently introduces re-ranking using a larger network. This innovative approach promises to deliver the best of both worlds: exceptional efficiency without compromising retrieval accuracy.
Repository link: http://resolver.tudelft.nl/uuid:751092b8-1b3d-4335-98bd-cc26e69d374c
Signal Processing Seminar
- Wednesday, 20 September 2023
- 10:30-11:30
- HB 13.140
Automotive Radar for Autonomous Driving: Signal Processing Meets Deep Learning
Sunqiao SunUniv. Alabama, USA
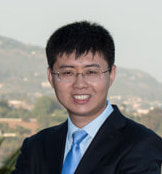
Millimeter-wave automotive radar emerges as one of key sensing modalities for autonomous driving, providing high resolution in four dimensions (4D), i.e., range, Doppler, and azimuth and elevation angles, yet remain a low cost for feasible mass production. In this talk, we will address the challenges in automotive radar for autonomous driving, examine how signal processing and deep learning can be combined to optimize the performance of automotive radar systems, and outline future research directions. Our focus will be on the generation of high-resolution radar imaging using multi-input multi-output (MIMO) radar and frequency-modulated continuous-wave (FMCW) technology. We will examine the challenges of waveform orthogonality, mutual interference, and sparse antenna array design and present our recent innovations in the field, including sparse array interpolation via forward-backward Hankel matrix completion, fast direction-of-arrival estimation via unrolling iterative adaptive approach, and adaptive beamforming via deep reinforcement learning, leading to the generation of high-resolution low-level automotive radar imaging, represented in bird's-eye view (BEV) format, providing rich shape information for object detection and recognition with deep neural networks. However, the radar BEVs are in general hardly shift-invariant over both angle and range since not every pixel is generated equally. The talk will highlight the importance of physics-aware machine learning in perception task on high-resolution radar imaging. We will show how incorporating radar domain knowledge and signal structure into deep neural network design can lead to more accurate and reliable object detection and recognition. Finally, we will discuss future research directions, including integrated sensing and communication, and collaborative radar imaging via an automotive radar network.
Bio:
Shunqiao Sun received the Ph.D. degree in Electrical and Computer Engineering from Rutgers, The State University of New Jersey under supervision of Prof. Athina Petropulu in Jan. 2016. He is currently an assistant professor at The University of Alabama, Tuscaloosa, AL, USA. From 2016-2019, he was with the radar core team of Aptiv, Technical Center Malibu, California, where he has worked on advanced radar signal processing and machine learning algorithms for self-driving vehicles and lead the development of DOA estimation techniques for next-generation short-range radar sensor which has been used in over 120-million automotive radar units. His research interests lie at the interface of statistical and sparse signal processing with mathematical optimizations, automotive radar, MIMO radar, machine learning, and smart sensing for autonomous vehicles. Dr. Sun has been awarded 2016 IEEE Aerospace and Electronic Systems Society Robert T. Hill Best Dissertation Award for his thesis “MIMO radar with Sparse Sensing”. He authored a paper that won the Best Student Paper Award at 2020 IEEE Sensor Array and Multichannel Signal Processing Workshop (SAM). He is Vice Chair of IEEE Signal Processing Society Autonomous Systems Initiative (ASI) (2023-2024). He is an associate editor of IEEE Signal Processing Letters and IEEE Open Journal of Signal Processing. He is a Senior Member of IEEE.
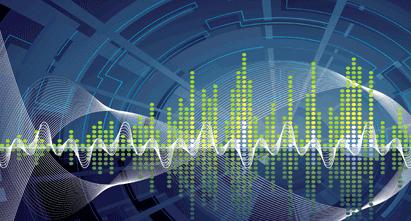
MSc SPS Thesis presentation
- Wednesday, 13 September 2023
- 10:00-12:00
- Pulse-Hall 9, 33.A2.300
Indoor in-network asset localization using Crownstone network
Vishakha K. Marathe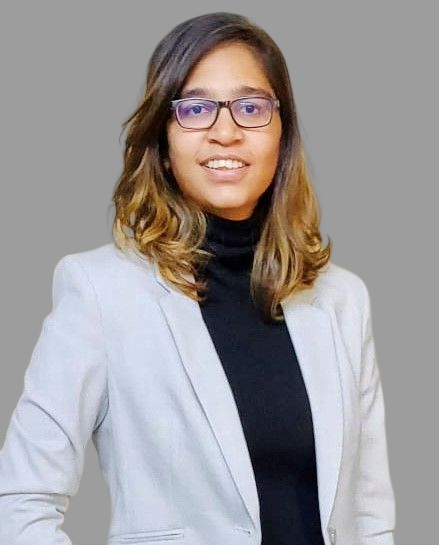
Indoor localization is the process of determining the location of an asset within an indoor environment. Crownstone, a subsidiary company of Almende, wants to fulfill specific tasks within the Crownstone network like developing indoor localization algorithms to get the room-level location of an asset for smart building, home automation and healthcare applications. Crownstones act as sensors that receive the Bluetooth messages transmitted by the asset and measure the strength of the received signal(RSSI).
There are two most widely used and researched asset localization methods, namely, model-based(MB) and data-driven(DD) methods have their drawbacks, especially because of the presence of obstacles, signal interference, reflections, noise that influence the RSSI signals and the dependency of the methods on the knowledge of sensor positions. In this thesis, a centralized multilateration(MB-C) algorithm as well as a simple averaging consensus based distributed(MB-D) algorithm is implemented. Algorithms are tested on real data collected at the Almende office(test environment) divided into a finite number of rooms.
To deal with the challenges posed by the MB algorithms like selecting a model, learning parameters and an additional step of mapping the position output to a room location, an Ensemble based centralized machine learning(DD-C) data driven algorithm is proposed that classifies the asset in one of the rooms with an accuracy of 65%. Algorithm is further improved by distributed data handling leading to a classification accuracy of 77%. To perform in-network localization, a consensus based distributed ML algorithm (DD-D) is proposed that performs local predictions within the Crownstone network using the same globally trained model giving a classification accuracy of 73%. The results show that the proposed DD algorithms perform better than the MB algorithms in terms of accuracy and are comparable in terms of prediction time. They also indicate that the proposed DD algorithms are more scalable, robust against noise but are computationally expensive.
Thesis link: http://resolver.tudelft.nl/uuid:7b0eb6bb-323f-44e8-b994-28dd8c9c9467
MSc SPS Thesis presentation
- Tuesday, 12 September 2023
- 13:00-15:00
- HB17.140 (Seminar room)
Direction Finding and Localization with Bluetooth 5.2
Xuchang Zhang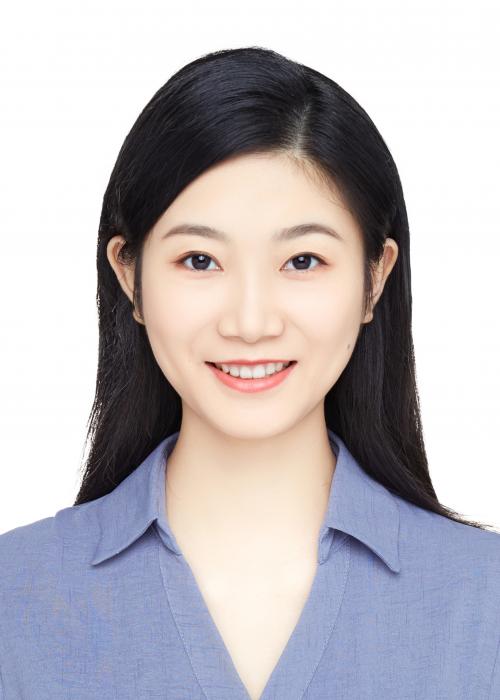
The potential of indoor localization using the Bluetooth Low Energy approach increases with the introduction of the Constant Tone Extension (CTE) feature in BLE 5.1. These small and energy-efficient beacons transmit signals that Bluetooth-enabled devices can detect, allowing for proximity and positioning calculations. This technology supports novel applications such as indoor wayfinding. By applying the new feature with an appropriate antenna array, it is possible to estimate both the Angle of Arrival (AoA).
Nevertheless, estimating AoA poses significant challenges, particularly in indoor scenarios. Throughout the span of this project, an in-depth analysis is being conducted on several elements, including multipath propagation and frequency offset. We first look into the I/Q data processing and then dive into the effect of frequency offset. Following that, several AoA estimation algorithms and multipath-mitigation strategies are discussed. Finally, we model the overall AOA estimation problem, followed by a positioning algorithm based on the estimated angles.
In this project, we propose an end-to-end indoor BLE positioning solution. Matlab simulation is performed to assess its performance. The simulation reveals that, with appropriate settings, the Toeplitz Reconstruction (TR) method is the best. In the setting of a 4-by-4 uniform rectangular array (URA), over 90% of the results show a position inaccuracy that is smaller than 0.14 meters.
After the simulation, we conduct a real-world experiment to assess the practicality and effectiveness of the solutions. The TR approach demonstrates a position error of less than 0.4 m, which is lower than previous BLE positioning research.
Finally, we suggest a few future research directions. This involves optimizing parameters, considering other antenna-affecting elements, etc.
Repository link: http://resolver.tudelft.nl/uuid:ff13dbcd-da99-4599-ad7f-f3b7282681bb
MSc SPS Thesis presentation
- Thursday, 31 August 2023
- HB17.140 (Seminar room)
Using Tensor Decompositions To Obtain Biomarkers From Auditory Event-Related Potentials
Kenneth Stunnenberg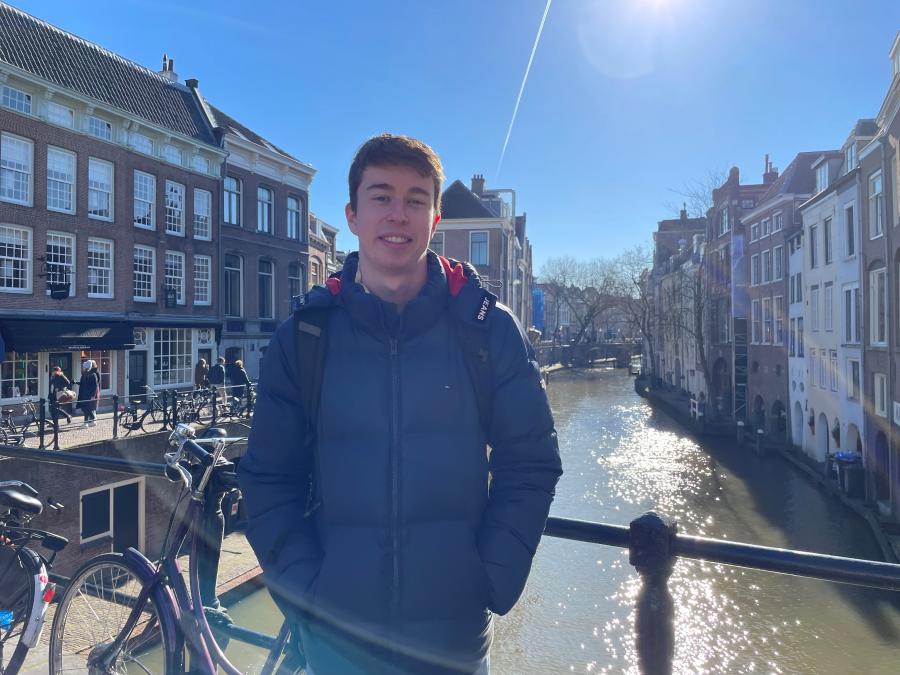
Brain disorders in children pose significant challenges to their development, impacting cognition, speech, movement, and behavior. The uncertainty surrounding prognostic information at the time of diagnosis leaves families with numerous questions about the future. The Child Brain Lab at Erasmus MC Sophia Children's Hospital conducts IQ, electroencephalogram (EEG), speech, and movement tests in playful environments, enhancing scientific research and healthcare practices for a better understanding of disease progression.
The Otolaryngology department at the Child Brain Lab focuses on auditory-related potentials (ERPs) obtained from EEG measurements to predict the future development of children with brain disorders. Analyzing ERP data from experiments like Mismatch Negativity (MMN) and Acoustic Change Complex (ACC) yields insights into developmental trajectories and connections between hearing, language, and brain development.
This thesis aims to explore alternative methodologies for extracting comprehensive information from ERPs, overcoming limitations of the commonly used peak amplitude and latency analysis. Tensor decompositions are employed to exploit structural information present in the data, using data fusion methods to combine multiple datasets for improved classification and deeper insights into group differences.
Simulations on artificial ERP data demonstrate that data fusion methods perform better on two ERP tensors compared to single tensor decomposition when group differences are shared between datasets. On a real dataset, tensor decompositions show promise for classifying subjects based on auditory event-related potentials while giving more insights into the neurological sources.
This report proposes an alternative method for analyzing ERP data, highlighting the potential of tensor decompositions and data fusion techniques.
MSc SPS Thesis presentation
- Monday, 28 August 2023
- 15:00
- Online
Path planning for Lunar rovers An Artificial Potential Field-based algorithm for the path planning of a walking Lunar rove
Thomas ManteauxEPFL
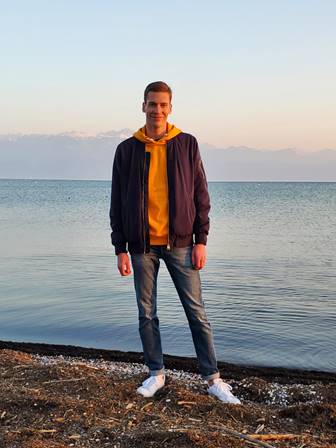
Abstract:
Abstract E↵ective path planning is a key challenge for Lunar rovers. This allows for safe autonomous navigation over complex and unknown areas. Lunar Zebro (LZ), a project of the Delft Univer[1]sity of Technology, is developing a robot to be the first European rover to walk on the Moon. This tiny rover, no bigger than an A4 sheet of paper, aims to explore a wide area to monitor solar radiations.
The thesis derives a path plannning algorithm for the local navigation of LZ. State-of-the[1]art path planning methods for terrestrial and non-terrestrial robots are studied. Metrics based on the needs of LZ are defined to compare the algorithms. Artificial Potential Field (APF)- based methods are identified as the most promising for LZ. APF methods are a category of path planning approaches in which robot motion is influenced by virtual forces generated by the destination point and obstacles. A Monte-Carlo simulation in a lunar environment is run to compare APF-based algorithms. The most relevant algorithm is picked up and improved (Bacteria-Aritificial Obstacle (B-AO) algorithm) with respect to success rate, path length and computing time. Finally it is implemented on a LZ prototype and tested in a challenging envi[1]ronment. Testing is conducted on a real lunar testbed made of sand, rocks and craters. A rock and crater abundance model is established considering rock and crater coverage of 2% and 15% respectively to represent the lunar surface as close as possible.
The B-AO algorithm shows 200% higher success rate and 50% lower computing time than the conventional APF algorithm, for only 5% longer path length than the optimal algorithm A*. It also outperforms state-of-the-art APF-based algorithms by more than 15% in reachabil[1]ity and 10% in path length for a similar or shorter planning time. Field testing results exhibit the robustness of the B-AO algorithm to real-world uncertainties in di↵erent scenarios. They also show that near-optimal paths are computed in real-time with limited available processing power. The bacterial approach of the B-AO algorithm makes it faster to execute and smaller to store than path planning algorithms used on existing or past non-terrestrial rovers.
Keywords: Path planning, Moon rover, Artificial Potential Field methods, Lunar Zebro
MSc SPS Thesis presentation
- Friday, 25 August 2023
- 10:30-13:00
- HB17.140 (Seminar room)
Coded Excitation for Doppler Ultrasound Imaging of The Brain
Lexi Zhu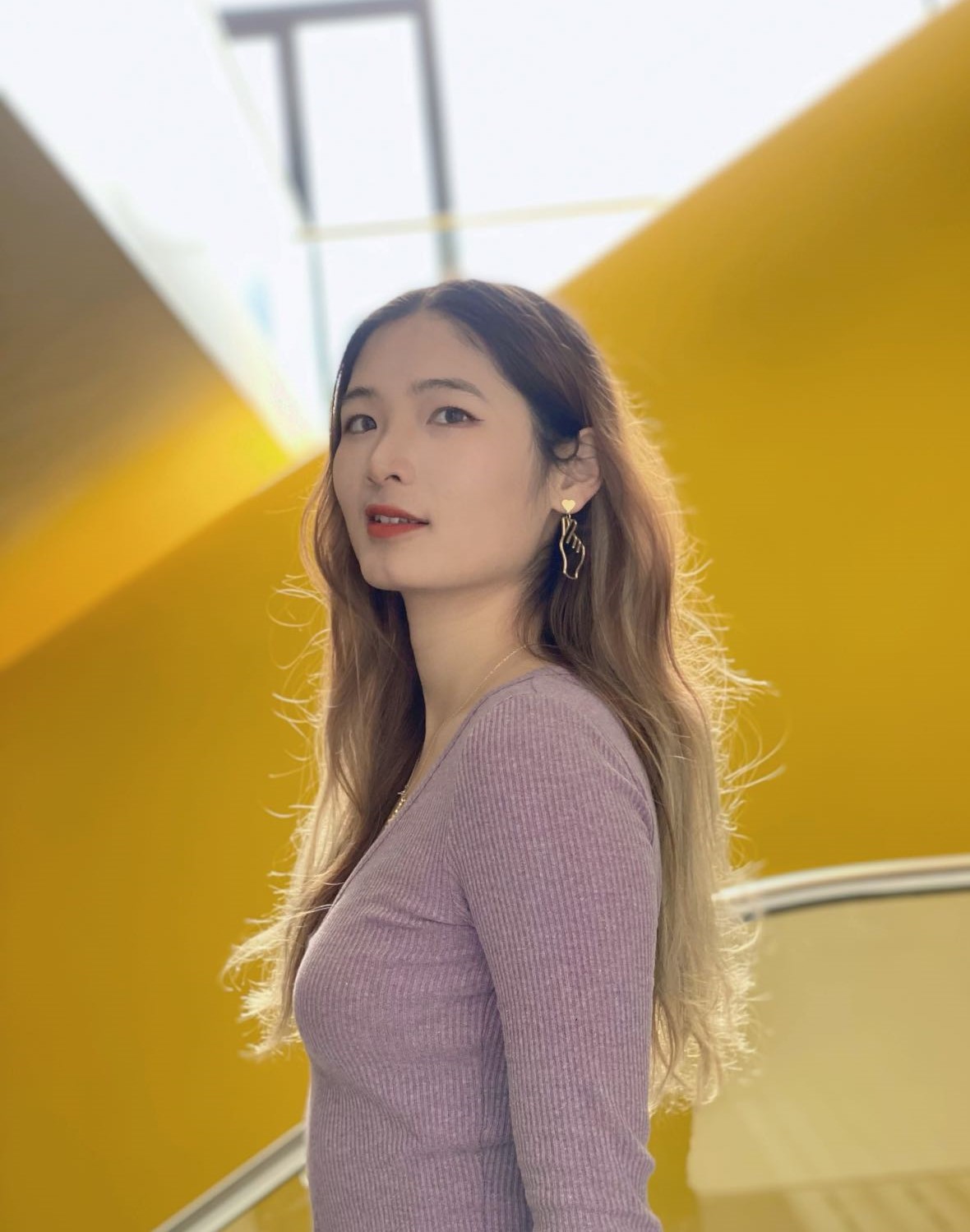
Doppler ultrasound imaging of cerebral blood flow faces challenges arising from a low signal-to-noise ratio (SNR) and a wide dynamic range. Echo signals received from blood cells are significantly weaker compared to surrounding tissues, such as the skull or brain soft tissue, resulting in inhibited visualization of small blood vessels and deep brain areas. To address this issue, this thesis explored the feasibility of employing and improving coded excitation techniques to enhance the SNR of Doppler ultrasound images. Furthermore, an optimized code for Doppler ultrasound imaging is designed, represented by a generalized encoding matrix.
The research begins with the definition of a linear signal model that incorporates the encoding matrix. Subsequently, a trace-constraint optimization problem is formulated based on maximizing the Fisher information matrix to find the optimized encoding matrix. The feasibility and performance of the optimized encoding matrix are assessed through simulations on both small and large array settings, which operate above Nyquist sampling frequency and under Nyquist sampling frequency respectively. The imaging results indicate that the optimized code exhibits higher SNR in deep image regions compared to existing coded excitation methods like Barker code while using the same number of transmissions, bit length, and same average transmit energy, albeit with a trade-off of decreased axial resolution. Nonetheless, this resolution degradation can be mitigated through the application of the iterative imaging technique LSQR. Finally, the optimized code is tested in a clinical transducer setting, and a blood flow simulation is conducted. The outcomes showcase the capacity of the proposed optimized code to enable higher SNR in Doppler ultrasound imaging and more accurate and informative clinical assessments.
MSc SPS Thesis presentation
- Thursday, 24 August 2023
- 10:00
- HB13.140, Boardroom EEMCS
Ultrasound Imaging through Aberrating Layers using a Virtual Array
Francesca De Carlo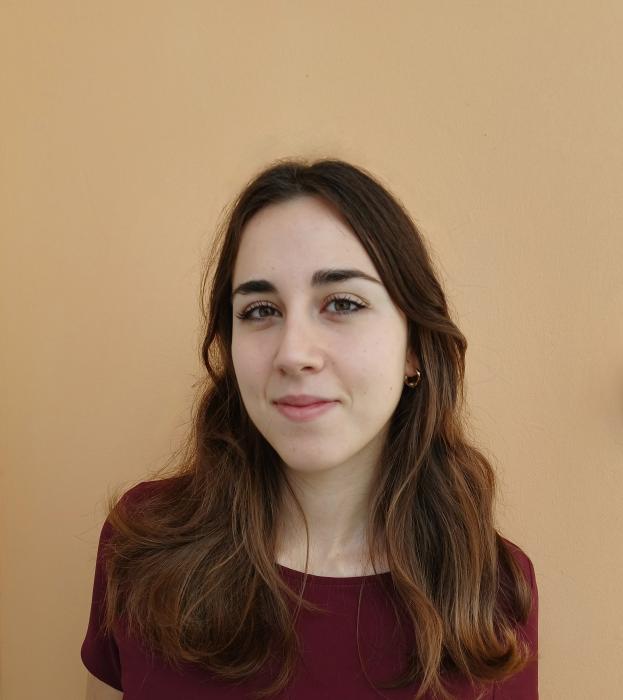
Ultrasound images are typically generated using the Delay-And-Sum (DAS) method, which assumes a homogeneous propagation medium. When an aberrating layer is situated between the sensor array and the imaging target, this assumption does not hold, and DAS is replaced with model-based methods. These methods are computationally expensive and require to accurately model the aberrations caused by the layer. This thesis investigates novel methods for image formation and aberration estimation. The effect of the layer is described using a set of transfer functions from the sensor array to a virtual array placed after the layer. In the first part, we assume the transfer functions are known, and we propose a new method for image formation. The transfer functions allow to map the signal from the sensor array to the virtual array, and the DAS method is used on the virtual array signal. This technique is equivalent to model-based matched filtering in terms of image quality, without requiring expensive matrix computations. In the second part, the transfer functions are unknown, and a novel technique is introduced for their estimation. Using pulse-echo data, a focus-quality metric is computed to quantify the accuracy of the transfer function estimate. The transfer functions are modeled using a dictionary and the dictionary coefficients are iteratively updated to increase the defined metric. The optimization leads to improved focus quality and sharper images. In the case the layer model requires a limited dictionary, the proposed algorithm generates an accurate estimate of the transfer functions.
MSc SPS Thesis presentation
- Wednesday, 23 August 2023
- 10:00
- Boardroom, HB13.140
Prediction of Post-induction Hypotension Using Machine Learning
Shuoyan Zhao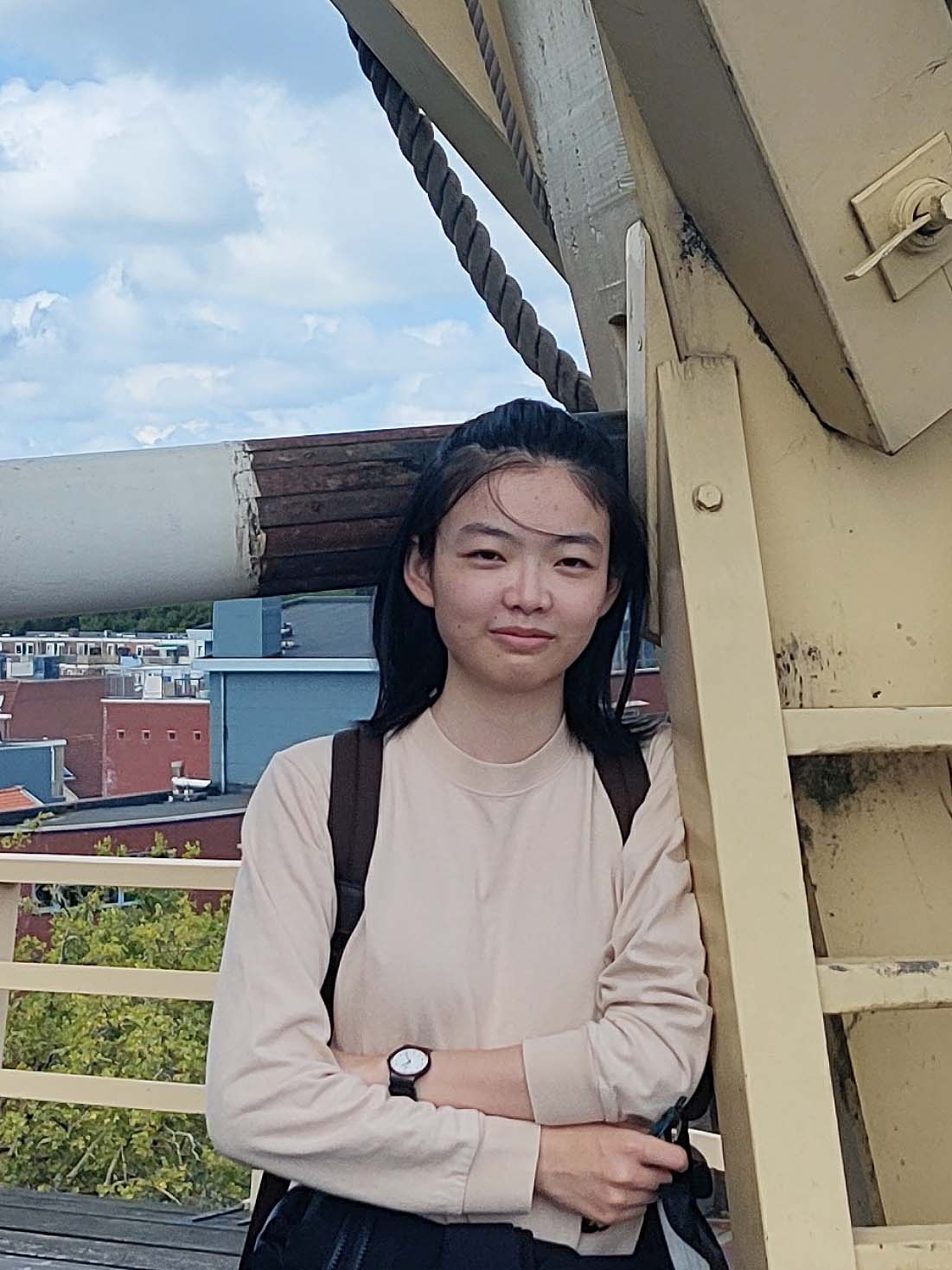
Abstract:
Anesthesia-related hypotension is a significant concern during surgery, occurring shortly after induction and potentially leading to severe complications. Since the anesthetic drug is believed to have an important role in the occurrence of post-induction hypotension (PIH), anesthesiologists now advocate for the appropriate selection of anesthetics dosage to avoid PIH.To facilitate such decision-making, an accurate prediction of PIH associated with a certain dosage of anesthetics is necessary.
This thesis presents a high-accuracy prediction model for PIH that supports anesthesia decision-making. The model is trained on data from the VitalDB database of 320 patients undergoing general anesthesia. The target output of this classification model is the occurrence of PIH, as defined through comprehensive analysis that incorporates clinical operations. Besides demographic data and vital signs, our model incorporates the dosage of propofol administered during the induction period as an input variable, mimicking real-world anesthetic plans. By employing the model in the target control infusion system of anesthesia, the anesthetics dosage can be varied as input, providing outcome predictions as security suggestions. An ensemble algorithm is employed to balance the prediction performance and the ability to elucidate the positive relationship between propofol and PIH risk, forming an anesthetics advice model. Compared to previous PIH prediction studies, our prediction model is validated in a more reliable nested cross-validation approach and achieves a higher performance (precision of 0.83 and recall of 0.84). We believe utilizing demographic and dynamic vital signs to predict HIP can be useful in determining the appropriate anesthetic dosage plan, offering potential improvements in patient care and safety.
MSc SPS Thesis presentation
- Tuesday, 22 August 2023
- 09:30
- HB17.140 (Seminar room)
Deep learning-empowered Content-based Video Image Retrieval (CBVIR)
Sinian Li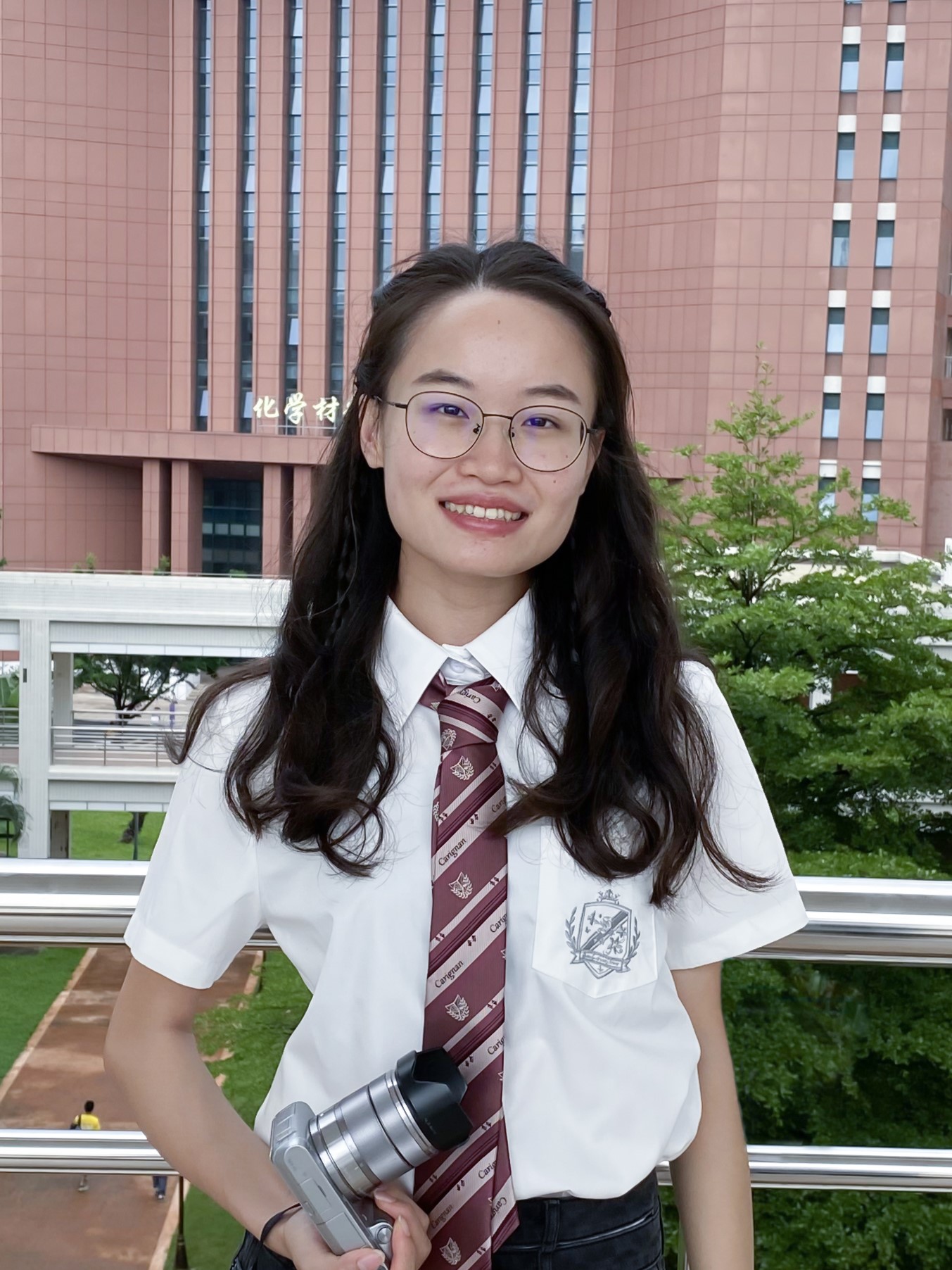
The advent of streaming and video has sparked a revolutionary shift in the presentation of materials across various fields, such as history, art, and media copyright protection. In this context, scholars and rights holders are seeking efficient solutions to index, retrieve, and browse through digital content searching for a specific instance. Unlike searching a specific instance in an image, searching in a video requires more than analyzing the visual features of an image and then comparing these features to a database, for it includes processing video sequences and retrieving video segments.
Motivated by the urgent need and promising applications across diverse disciplines, we present a novel deep-learning-empowered content-based video image retrieval (CBVIR) system with a strong emphasis on real-world applications. This system offers high efficiency and considerable accuracy, addressing the challenges associated with accessing and utilizing video materials effectively. Our initial approach revolves around the extraction of informative keyframes that effectively capture essential objects within the video. This process, known as Key Frame Extraction (KFE), enables us to distill the most crucial visual representations for further analysis. After the extraction of keyframes, the relatively smaller dataset allows for content-based image retrieval (CBIR) to be conducted, retrieving similar images from a database solely based on the content of the query image. In this project, a wide range of methods is investigated and analyzed, including traditional representation, handcrafted image feature extraction, and up-to-date machine learning-based image representations. Our contribution is striking a balance between high-level and low-level image representation for this task; targeting efficiency improvement, enhanced color-based KFE module is proposed and implemented, achieving high efficiency ratio and satisfactory accuracy and targeting accuracy, a traditional and deep learning-based hybrid feature is proposed, achieving valid efficiency ratio and highest accuracy. Overall, an automatic retrieving system requiring much less user engagement is provided together with a system GUI prototype.
MSc SPS Thesis presentation
- Tuesday, 22 August 2023
- 13:00
- HB17.140 (Seminar room)
LiDAR and Radar-Based Occupancy Grid Mapping for Autonomous Driving Exploiting Clustered Sparsity
Çağan Önen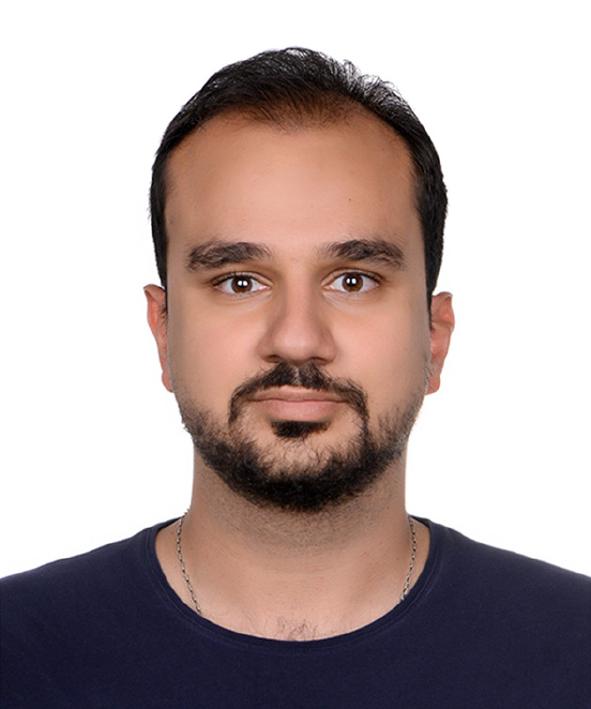
Occupancy grid maps are fundamental to autonomous driving algorithms, offering insights into obstacle distribution and free space within an environment. These maps are used for safe navigation and decision-making in self-driving applications, forming a crucial component of the automotive perception framework. An occupancy map is a discretized representation of a chosen environment that is constructed using point cloud information obtained from sensor modalities like LiDAR and radar. In this project, we formulate the problem of estimating the occupancy grid map using sensor point cloud data as a sparse binary occupancy value reconstruction problem. We utilize the inherent sparsity of occupancy grid maps commonly encountered in automotive scenarios. Besides, the spatial dependencies between the grid cells are exploited to provide a better reconstruction of the boundaries of the objects inside the range of the map and to suppress the false alarms emerging from the reflections coming from the road. To address sparsity and spatial correlation jointly, we propose an occupancy grid estimation method that is based on pattern-coupled sparse Bayesian learning. The proposed method shows enhanced detection capabilities compared to two benchmark methods, based on qualitative and quantitative performance evaluation with scenes from the automotive datasets nuScenes and RADIal.
MSc SPS Thesis presentation
- Monday, 31 July 2023
- 10:00-12:00
- HB17.140 (Seminar room)
Machine learning algorithm to estimate cardiac output based on arterial blood pressure measurements.
Alan Hamo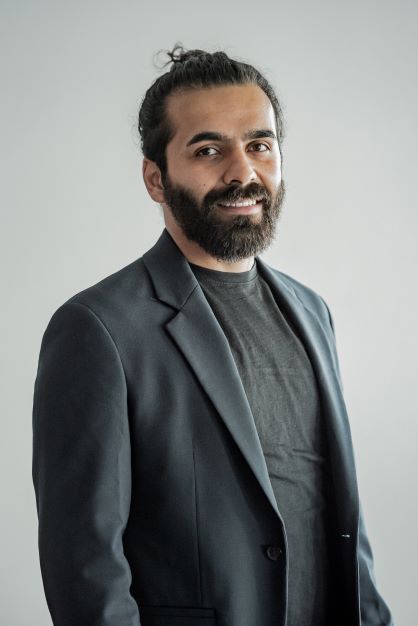
Cardiac output (CO), a vital hemodynamic parameter that reflects the blood volume pumped by the heart per minute, is crucial for determining tissue oxygen delivery and the heart's ability to meet the body's demands. Researchers developed various methods to measure cardiac output, including thermodilution using pulmonary artery catheters (PAC), also called Swan-Ganz catheters, the gold standard for cardiac output measurements. Such an approach involves an invasive procedure associated with complications, and it requires specialized equipment and expertise, limiting its use to critically ill patients undergoing operations in intensive care units (ICUs). An alternative, less invasive way to estimate CO is by analyzing arterial blood pressure (ABP) waveform. However, the relationship between cardiac output and blood pressure is unknown. This study uses machine learning and feature engineering techniques to discover the relationship between CO and ABP. We used the sparse identification non-linear dynamics (SINDy) algorithm to discover features that significantly contribute to the relationship between CO and ABP. Additionally, we investigated the optimum number of cardiac cycles needed to achieve the best performance providing insights into the temporal dynamics of CO estimation. The proposed approach achieved clinically acceptable performance regarding radial limits of agreement and bias. Further, the proposed approach was validated on an external dataset and achieved comparable performance. Finally, the learned model was interpreted as a differential equation describing the blood flow where CO acts as an external force to the system. All materials used in this study, including code, model, raw data, processed data, and extracted features, are available on GitHub to facilitate further development.
MSc SPS Thesis presentation
- Friday, 21 July 2023
- 10:00-12:30
- Timmanzaal (LB01.170)
Finding Representative Sampling Subsets on Graphs: leveraging submodularity
Tianyi Li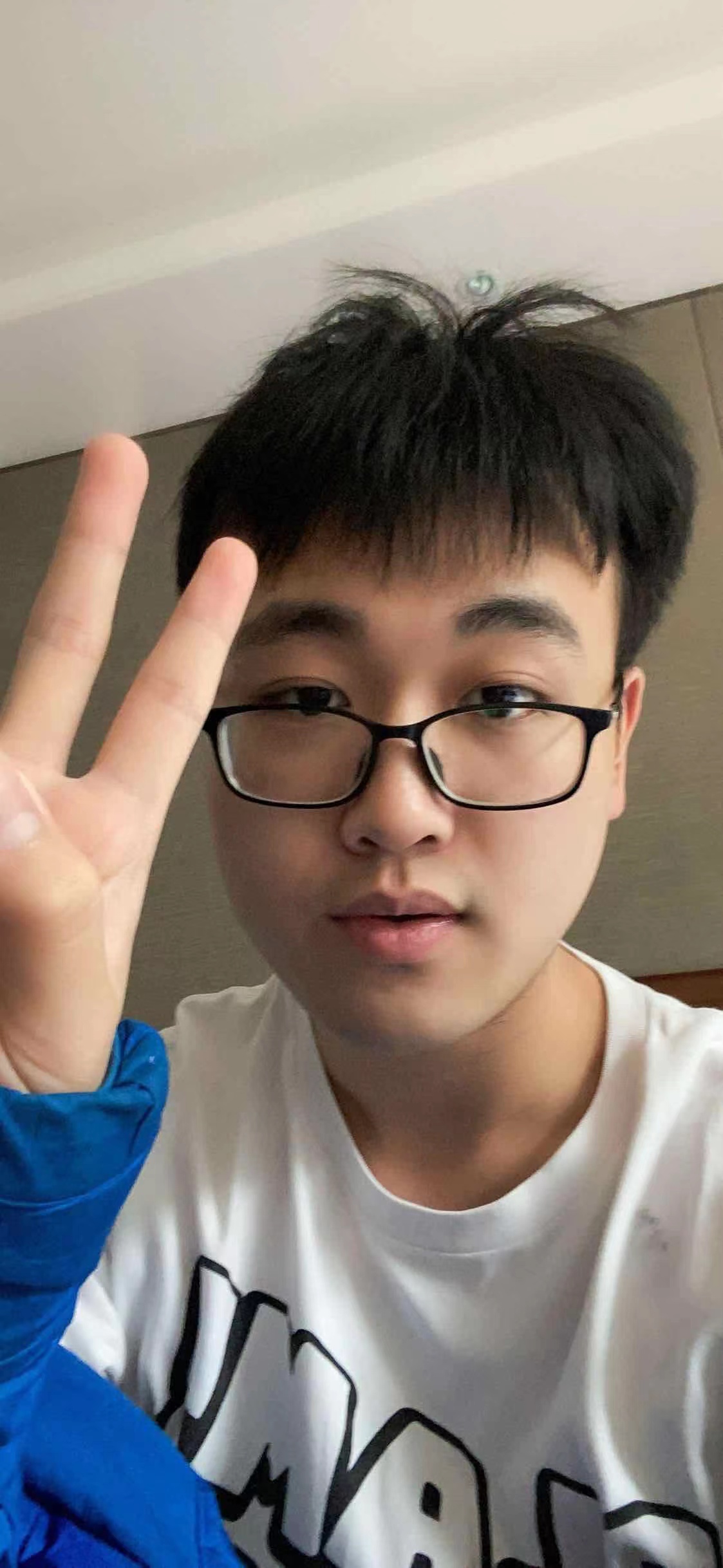
In this work, we deal with the problem of reconstructing a complete bandlimited graph signal from partially sampled noisy measurements. For a known graph structure, some efficient centralized algorithms are proposed to partition the graph nodes into disjoint subsets such that sampling the graph signal from any subsets leads to a sufficiently accurate reconstruction on average. Furthermore, we consider the situation when the graph is massive, where processing the data centrally is no longer impractical. To overcome this issue, a distributed framework is proposed that allows us to implement centralized algorithms in a parallelized fashion. Finally, we provide numerical simulation results on synthetic and real-world data to show that our proposals outperform state-of-the-art node partitioning techniques.
MSc SPS Thesis presentation
- Monday, 17 July 2023
- 13:00-14:30
- Lipkenszaal (LB01.150)
Privacy Analysis of Decentralized Federated Learning
Wenrui Yu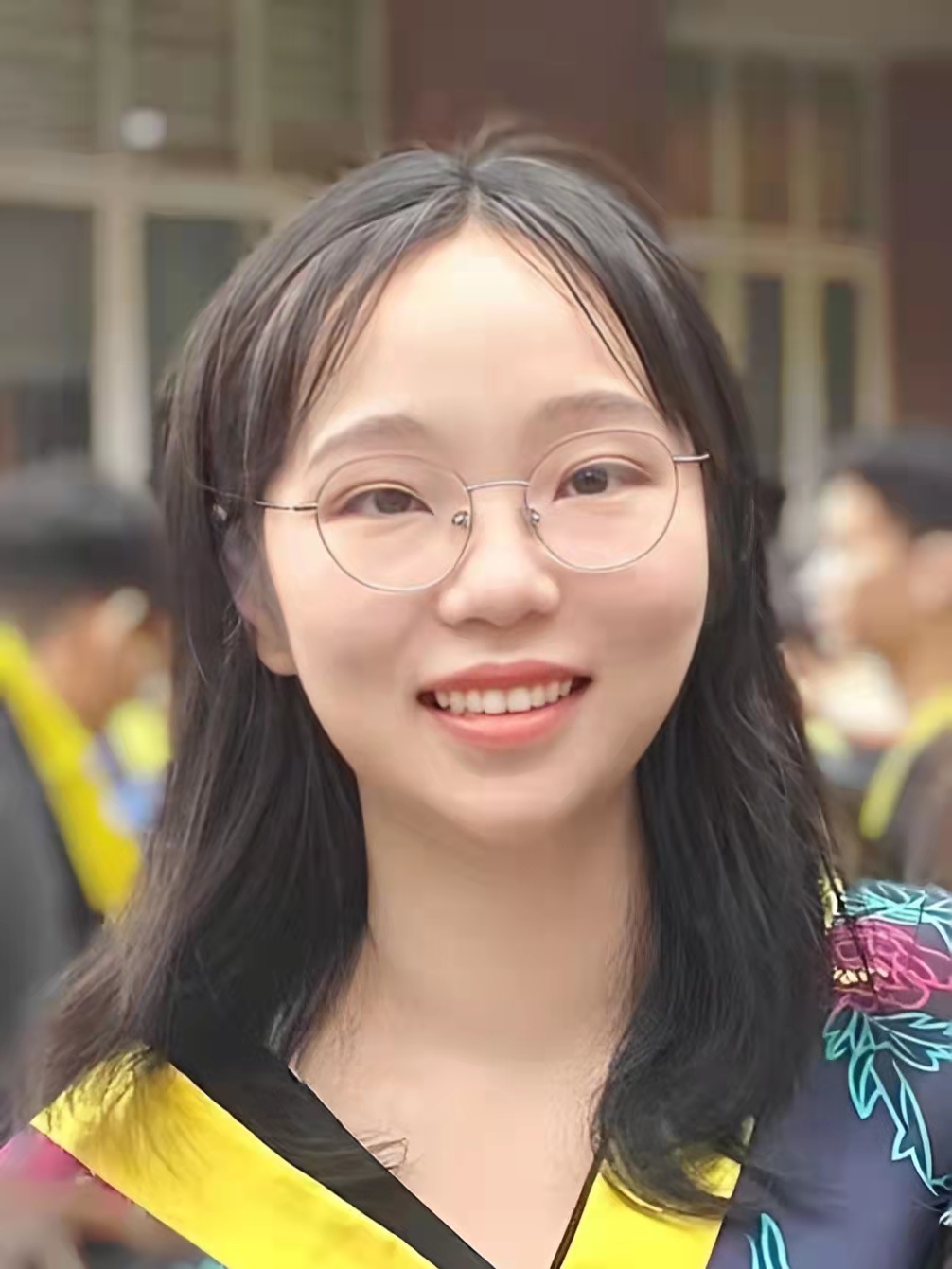
Privacy concerns in federated learning have attracted considerable attention recently. In centralized networks, it has been observed that even without directly exchanging raw training data, the exchange of other so-called intermediate parameters such as weights/gradients can still potentially reveal private information. However, there has been relatively less research conducted on privacy concerns in decentralized networks.
In this report, we analyze privacy leakage in optimization-based decentralized federated learning, which adopts generally distributed optimization schemes such as ADMM or PDMM in federated learning. By combining local updates with global aggregations, it was proved that optimization-based approaches are more advantageous compared to the traditional average consensus-based approaches, especially in scenarios where the data at the nodes are not independent and identically distributed (non-IID).
We further extend the privacy bound in distributed optimization to the decentralized learning framework. Different from the fact in the centralized learning framework the leaked information is the local gradients of each individual participant at all rounds, we find that in decentralized cases the leaked information is the difference of the local gradients within a certain time interval. Motivated by the gradient inversion in centralized networks, we then design a homogeneous attack to iteratively optimize dummy data whose gradient differences are close to the true revealed gradient differences. Though the gradient difference information still brings privacy concerns, we show that it is more challenging for adversaries to reconstruct private data using the difference of gradients than using the gradients themselves in the centralized case.
To deal with the privacy attack, we propose several potential defense strategies such as early stopping, inexact update and quantization etc. The main advantage of these approaches is that they introduce error/noise/distortion into decentralized federated learning for protecting private information from being revealed to others without affecting the training accuracy. In addition, we also show that the larger the batchsize is, the more difficult for the adversary to reconstruct the private information.
MSc SPS Thesis presentation
- Monday, 17 July 2023
- 09:00-11:30
- HB17.140 (Seminar room)
Simplicial Unrolling ElasticNet for Edge Flow Signal Reconstruction
Chengen Liu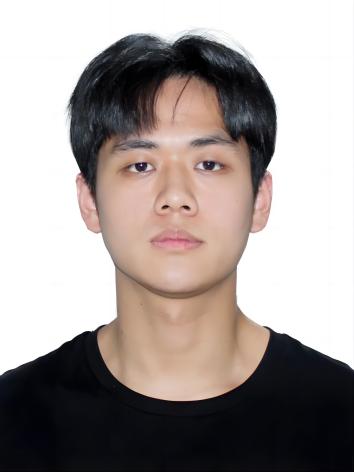
The edge flow reconstruction task improves the integrity and accuracy of edge flow data by recovering corrupted or incomplete signals. This can be solved by a regularized optimization problem, and the corresponding regularizers are chosen based on prior knowledge. However, obtaining prior information is challenging in some fields. Thus, we consider exploiting the learning ability of neural networks to acquire prior knowledge. In this thesis, we propose a new optimization problem for the simplicial edge flow reconstruction task, the simplicial ElasticNet, which is a regularized optimization problem that combines the advantages of the L1 and L2 norm. It is solved iteratively by the multi-block ADMM algorithm, and the convergence conditions are illustrated. By unrolling the simplicial ElasticNet's iterative steps, we propose a neural network with high interpretability and low requirement for the number of training data for the reconstruction task of simplicial edge flows. The unrolling network replaces the fixed parameters in the iterative algorithm with the learnable weights in the neural networks, thus exploiting the neural network's learning capability while preserving the iterative algorithm's interpretability. The core component of this unrolling network is simplicial convolutional filters with learnable weights to aggregate information from the edge flow neighbors, thus enhancing the learning and expressive ability of the network. We conduct numerical experiments on real-world and artificial datasets to validate the proposed approach. It is demonstrated that the simplicial unrolling network is significantly more advantageous than the traditional iterative algorithms and standard non-model-based neural networks in the case of limited training data.
MSc SPS Thesis presentation
- Tuesday, 11 July 2023
- 13:00
- HB13.140, Boardroom EEMCS
Estimating Transmembrane Currents and Local Activation Times from Atrial Epicardial Electrograms
Teodor Licurici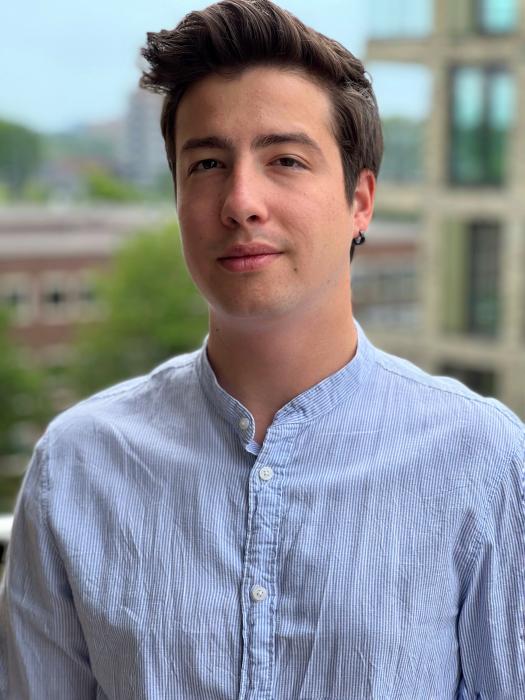
Estimating the transmembrane currents travelling through the epicardium and local activation times based on atrial epicardial electrograms can greatly help in the study of cardiac arrhythmias such as atrial fibrillation. This work focuses on the accurate estimation of the aforementioned signals and features. To do this, two least squares-based regression methods were used to estimate transmembrane currents from electrograms and then find their local activation times by searching for the maximum negative slope. The first least squares optimization method consists of using standard least squares, while the second consists of regularized least squares, by combining both lasso and ridge regression, to deal with signal sparsity and multicollinearity, respectively. Furthermore, to improve estimation results, multiresolution analyses based on wavelet decompositions and principal components analysis were used to filter out parasitic components that were present in the estimated transmembrane currents by separating them from the main activation complex of the decomposed signals.
Using these algorithms on simulated data, it was shown that promising results can be achieved for both transmembrane current estimations and LAT estimations. Several wavelet support sizes were tested on the simulated data to observe performance changes. These were compared to an already existing LAT estimation algorithm. The results mainly confirm the efficiency of the proposed methods on severely diseased tissue corrupted by conduction blocks and noise.
MSc SPS Thesis presentation
- Tuesday, 4 July 2023
- 10:30-12:00
- SPS Seminar room, HB17.140
Comparative analysis of clutter filtering techniques on freehand µDoppler ultrasound imaging
Xuan GaoErasmus MC
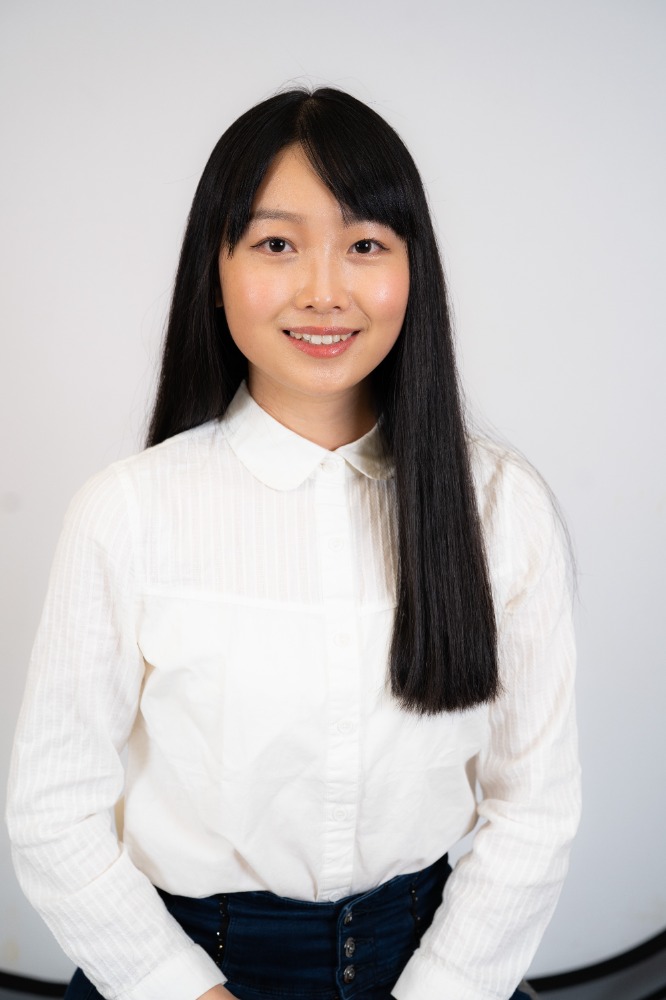
Micro-Doppler (µDoppler) ultrasound imaging is a high frame rate ultrasound imaging modality that provides high spatiotemporal resolution ultrasound images of blood flow. It is sensitive to slow blood flow and particularly suitable for capturing fast-changing phenomena like rapid blood flow. Clutter filtering is an essential step in µDoppler data processing to reject tissue clutter signals and keep blood flow information as much as possible. 3D freehand µDoppler imaging is an emerging ultrasound technique that can construct full spatial vasculature images with a panoramic view that conventional 2D ultrasound is not able to provide. As freehand implies the continuous and nonuniform movement of the probe, it becomes more challenging for clutter filtering to acquire high-quality images.
This thesis explores and compares different state-of-art clutter filtering techniques on freehand in-vivo µDoppler imaging of the human brain. Specifically, Singular Value Decomposition (SVD), Robust Principle Component Analysis (Robust PCA), and Independent Component Analysis (ICA) clutter filtering techniques have been investigated. The aim is to test and compare their performance on in-vivo µDoppler ultrasound data with freehand probe movement and understand how freehand motion affects the threshold selection criteria. Besides that, a newly proposed method that combines ICA clutter filtering and clustering is included in this thesis to bring another perspective for sorting independent components corresponding to blood flow and rejecting unwanted ones consisting mostly of tissue clutter signals.
MSc SS Thesis Presentation
- Thursday, 29 June 2023
- 09:00-09:45
- HB 17.140
Rank Detection Based on Generalized Eigenvalue Threshold in Arbitrary Noise
Bingxiang Zhong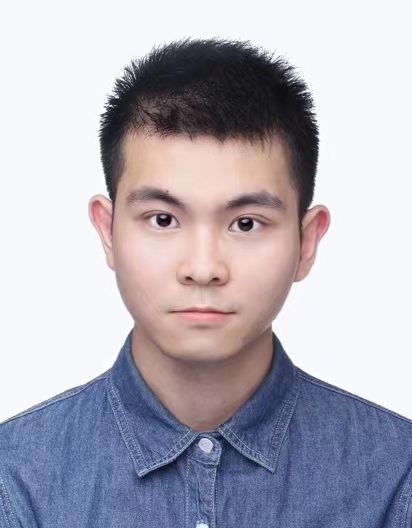
Rank detection is crucial in array processing applications, as many algorithms rely on accurately estimating the rank of the data matrix to ensure optimal performance. Under Gaussian white noise, rank can be detected through eigenvalue analysis. However, in arbitrary noise, prewhitening the data matrix with the noise covariance matrix is necessary, and rank detection is achieved by examining the generalized eigenvalues. Existing methods often assume the noise covariance structure or require a large number of noise samples. This thesis focuses on addressing the rank detection problem in scenarios with limited noise samples and arbitrary noise environments.
Firstly, we investigate the largest generalized eigenvalue threshold for the prewhitened data sample covariance matrix according to the random matrix theory. We develop a rank detection algorithm based on the threshold via a sequential test, and provide the performance analysis. A series of simulations demonstrate its superiority over conventional methods such as Minimum Description Length (MDL) and Akaike’s Information Criterion (AIC). Secondly, since the Short-time Fourier Transform (STFT) is commonly used for non-stationary signal analysis, we extend our rank detection method to the STFT domain. The correlations introduced by the STFT have a significant impact on the distribution of the noise. Therefore, we develop a technique to remove correlations among time-frequency bins based on exact expressions of these correlations.
After successfully eliminating these correlations, our proposed rank detection method achieves enhanced reliability and performance in the STFT domain. Lastly, we evaluate the effectiveness of our rank detection method in speech enhancement applications. Simulations confirm that utilizing the estimated rank improves speech quality compared to using the known number of sources.
Additional information ...
MSc SS Thesis Presentation
- Thursday, 29 June 2023
- 15:30-16:30
- Executive room YesDelft
Self-calibrated plant counting in early crop stand scenarios using deep clustering
Jonathan Dijkstra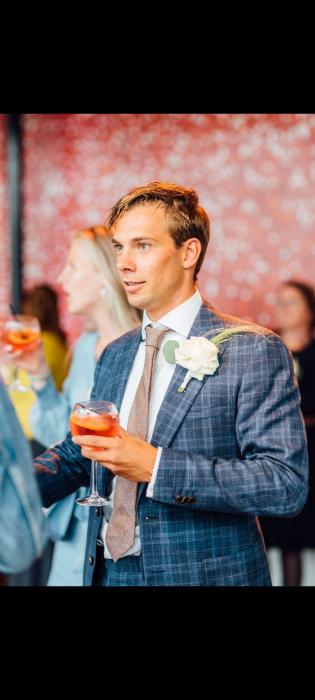
In recent years, the agricultural sector has seen significant techno- logical improvements under the flag of precision agriculture, assisting farmers in the manageability that coincides with large-scale farming. Moreover, precision agriculture aims to enable plant-specific farming on the macro scale that is demanded by the current global population growth. By more closely matching the individual needs of the plants, farmers are able to increase crop yield while reducing the environmen- tal footprint as well as the economic cost of farming due to savings in fertilizers and pesticides.
Visual inspection of arable land is a key factor in maintaining trace- ability of plant growth and health in precision farming. More specif- ically, plant counting, size measurement and plant localisation are of great use for farmers in yield prediction, growth tracking, and obtain- ing insight in the emergence ratio of the crop.
Most of the state-of-the-art plant counters, or object counters in gen- eral, rely on human annotations (labour intensive and error prone) as exemplars for the counting model. Self-supervised object counting, however, is a machine learning paradigm independent of human la- belled data, enabling an object counter to learn solely from raw photo- graphic data. Furthermore, the generative character of self-supervised learning models implies the potential to generalize well on unseen data to the model, such as new plant species or variations in plant size in the case of plant counting.
In this master’s thesis in cooperation with Tective Robotics, a study is performed towards the design of self-calibration based self-supervised object counting and localisation model for the scenario of early crop stand scenarios. More specifically, a novel self-calibrator has been developed to estimate the planting distance in between crops and a threshold for small object noise filtering (weeds, loose leaves ect). Im- plications of the self-calibrator are robustness to variations in plant size and allignement, accurate segmentation of occluded plant clusters and small-sized weed suppression.
Model testing on UAV orthomosaic arable land imagery collected by Tective Robotics B.V. has shown outstanding performance (R2 = 0.94) of the newly developed plant counting model, without the need of any labelled training data. The plant counter is comparable in performance to some of the commercially available plant counters. The plant counter, embedded in the entire data processing pipeline for Geotiff orthomosaics, has been made available on GitHub.
MSc SS Thesis Presentation
- Wednesday, 28 June 2023
- 10:00-10:45
- Snijderszaal (Room number LB01.010)
Coherent integration for imaging and detection using active sonar
Kaan Demir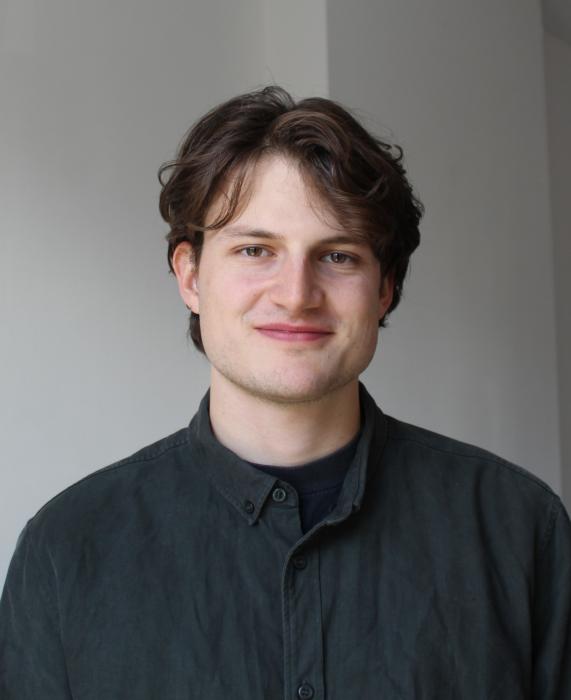
Existing sonar systems typically rely on a minimum signal strength of a single echo, which limits their performance in low signal-to-noise conditions. This thesis explores the concept of coherent integration for active sonar, with the aim of improving imaging and detection capabilities under low signal-to-noise conditions. The goal is to provide signal processing methods that achieve long-time coherent integration of the received echoes, thereby maximising the processing gain. Additionally, this research explores waveform design by comparing the performance of pseudo-random noise with chirps.
Two applications are seen in this thesis: moving target detection, which involves static sonar sensors, and synthetic aperture imaging, where the sensors move while the imaging scene remains static. For moving target detection, a processing methods is proposed which achieves coherent integration for constant velocity targets in a computationally efficient manner, and improves the detection performance by implementing a clutter filtering stage. For the second application, a processing method for imaging from a moving sensor pair is proposed. The resulting point-spread function for a circular sensor trajectory is investigated, from which a set of design rules are established. Additionally, a least squares algorithm is applied, which shows that the resulting image can be improved in terms of resolution and sidelobe interference.
Finally, the imaging and detection methods are tested and verified using an in-air demonstrator.
IEEE SPS Webinar
- Wednesday, 26 April 2023
- 17:00-18:00
- webex (see link)
Adaptive and Fast Combined Waveform-Beamforming Design for mmWave Automotive Joint Communication-Radar
Dr. Preeti Kumari, Dr. Nitin Jonathan Myers, Dr. Robert Heath W. Jr.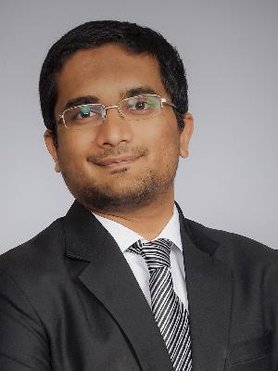
Millimeter-wave (mmWave) joint communication-radar (JCR) will enable high data rate communication and high-resolution radar sensing for applications such as autonomous driving. Existing mmWave JCR systems, however, suffer from a limited angular field-of-view and low estimation accuracy for radars due to the use of directional communication beams. In this presentation, we propose an adaptive beamforming design for mmWave JCR with a phased-array architecture that permits a trade-off between communication and radar performances.
To enable fast estimation of the mmWave radar channel in the Doppler-angle domain, we use a convolutional compressed sensing framework and optimize the radar waveforms within this framework. Our optimization accounts for the space-time sampling constraints that are specific to phased-array radars.
We evaluate the JCR performance trade-offs using a normalized mean square error (MSE) metric for radar estimation and a distortion MSE metric for data communication, which is analogous to the distortion metric in the rate-distortion theory. Numerical results demonstrate that our proposed JCR design enables the estimation of short- and medium-range radar channels in the Doppler-angle domain with a low normalized MSE, at the expense of a small degradation in the communication distortion MSE.
To attend, use the registration link provided below.
Additional information ...
Symposium
- Friday, 21 April 2023
- 09:00-18:00
- EEMCS - Lecture Hall Chip
Heterogeneous system integration - Driving the EU Chip Act ambitions
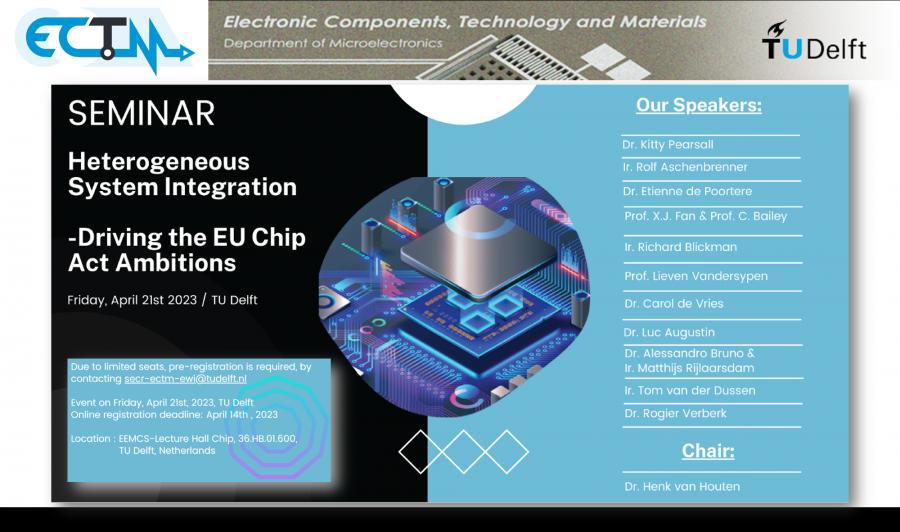
The Netherlands have a strong national ecosystem for quantum, photohics and semiconductor technologies, well connected to international key players. This symposium aims to build on this strength by intensifying collaboration among these domains.
Heterogeneous integration plays a crucial role in enabling future quantum, photonics and semiconductor technologies by creating new functionalities and business opportunities through the integration of different chips, technologies and materials into a single system.
This symposium will discuss the importance of heterogeneous integration and its potential for creating more industry and business value. It also aims to cultivate human resources for heterogeneous integration, further strengthening the Dutch ecosystem.
Join us to explore the exciting opportunities that heterogenous system integration can offer for the Dutch ecosystem and beyond, and to be part of the conversation on driving the EU Chip Act ambitions.
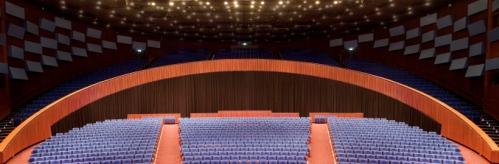
- Thursday, 30 March 2023
- 14:00
- Van der Poelzaal (LB01.220)
Array Processing in Atrial Fibrillation: Application of different signal models and LAT estimation techniques
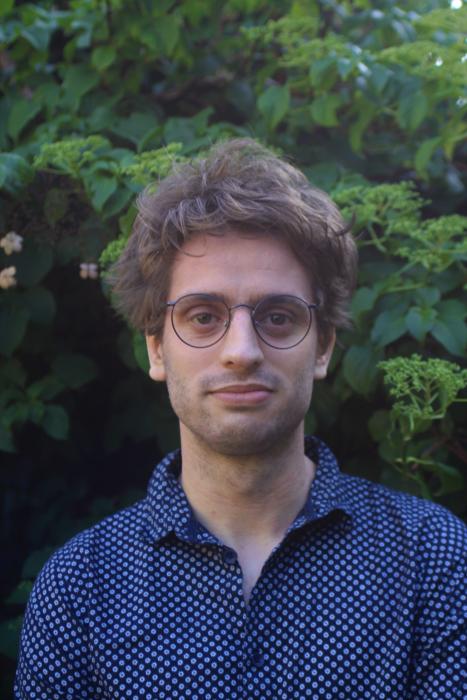
Thesis link : http://resolver.tudelft.nl/uuid:82dd26ec-d49b-4780-8b14-7ce2960c1b1b
Signal Processing Seminar
- Thursday, 16 March 2023
- 11:00-12:00
- HB 17.140
Robust Pareto-Optimal Radar Receive Filter Design for Noise and Sidelobe Suppression
Costas Kokke.jpg)
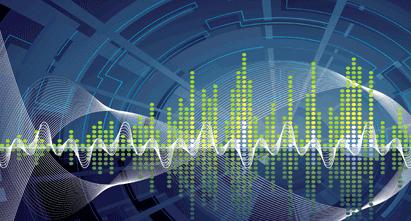
Signal Processing Systems Seminar
- Tuesday, 28 February 2023
- 11:00
- HB17.140
Community Detection in Multilayer Networks: Algorithms and Applications
Prof. Dr. Selin AviyenteDept of ECE, Michigan State University
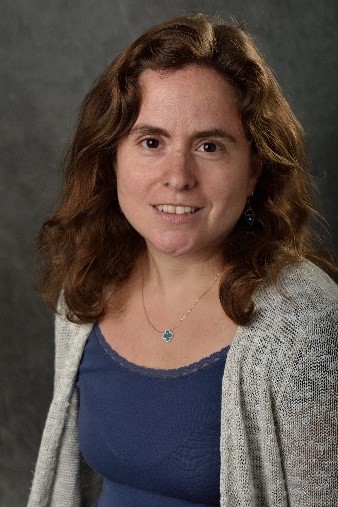
Abstract:
Modern data analysis and processing tasks typically involve large sets of structured data, where the structure carries critical information about the nature of the data. Typically, graphs are used as mathematical tools to describe the structure of such data. Traditional network models employ simple graphs where the nodes are connected to each other by a single, static edge. However, in many contemporary applications, this relatively simple structure cannot capture the diverse nature of the networks, e.g., multiple types of entities and interactions between them. Multilayer networks (MLNs) allow one to represent the interactions between a pair of nodes through multiple types of links. MLNs can further be categorized based on the homegeneity of the nodes and complexity of topological structure as: i) multiplex networks where each layer has the same set of entities of the same type and inter-layer edges are not shown as they are implicit; ii) heterogenous multilayer networks where the set and types of entities may be different for each layer and the relationships of entities across layers are shown using explicit inter-layer edges. A core task in the complexity reduction of these high-dimensional networks is community detection. In this talk, a joint nonnegative matrix factorization approach is proposed to detect the community structure in both multiplex and multilayer networks. The proposed approach considers the heterogeneity of layers and formulates community detection as a regularized optimization problem. The proposed approach is evaluated for both social networks and a fully connected multi-frequency brain network model.
Bio:
Selin Aviyente received her B.S. degree with high honors in Electrical and Electronics engineering from Bogazici University, Istanbul. She received her M.S. and Ph.D. degrees, both in Electrical Engineering: Systems, from the University of Michigan, Ann Arbor. She joined the Department of Electrical and Computer Engineering at Michigan State University in 2002, where she is currently a Professor and Associate Chair for Undergraduate Studies. Her research focuses on statistical and nonstationary signal processing, higher-order data representations and network science with applications to neuronal signals. She has authored more than 150 peer-reviewed journal and conference papers. She is the recipient of a 2005 Withrow Teaching Excellence Award, a 2008 NSF CAREER Award and 2021 Withrow Excellence in Diversity Award. She is currently serving as the chair of IEEE Signal Processing Society Bioimaging and Signal Processing Technical Committee, on the Steering Committees of IEEE SPS Data Science Initiative and IEEE BRAIN. She has served as an Associate Editor and Senior Area Editor for IEEE Transactions on Signal Processing, IEEE Transactions on Signal and Information Processing over Networks, IEEE Open Journal of Signal Processing and Digital Signal Processing.
SPS Seminar
- Friday, 24 February 2023
- 12:30-13:20
- Teams
Understanding the importance of AI quality management
Dr. Martin SaerbeckCTO Digital Service at TÜV SÜD (Singapore)
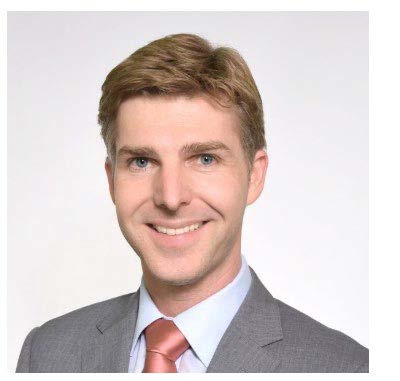
Organizations that want to benefit from AI while managing its risks need to overcome three challenges: adopting AI at scale, complying with regulations, and demonstrating the responsible use of AI. All these challenges can be addressed with appropriate AI quality management. Hence, organizations are looking for talent who understand both: AI technology and quality management. This lecture will introduce you to the core concepts of AI quality management. Awareness is the first step to update your skills and boost your AI careers. As a notified body, TÜV SÜD is a leading several initiatives around AI standardization and regulation. Join and gain an insight view in some of the latest developments and ongoing discussions.
Bio: In his role as CTO Digital Service at TÜV SÜD, Dr. Saerbeck oversees the technology roadmap and key implementation projects of digital testing services, including novel continuous testing services, targeting the demands of a connected smart industry. He has a long track record in academia and industry in the domains of smart sensor networks, robotics, and AI. After completing his PhD with Philips Research, Dr. Saerbeck started an interdisciplinary research team on human-machine interaction within the Institute of High Performance Computing, which developed novel technologies for several industries, including aerospace, manufacturing and retail. He is an awardee of the prestigious A*STAR Independent Investigatorship given by Agency for Science, Technology and Research, Singapore. Dr. Saerbeck has a passion for applied research, promoting translation of academic results in formal verification and artificial intelligence to make today’s connected smart systems safe, secure, and reliable.
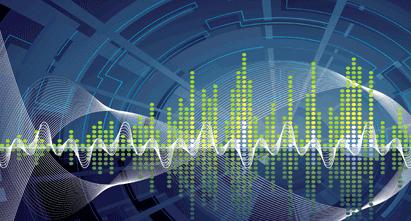
PhD Thesis Defence
- Tuesday, 31 January 2023
- 15:00-16:30
- Aula Senaatszaal
Ultrasound Imaging through Aberrating Layers
Pim van der Meulen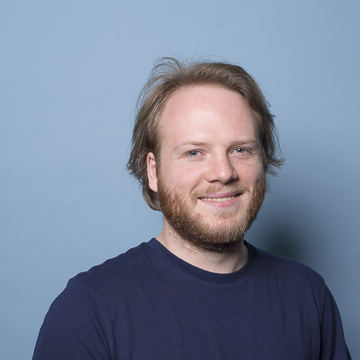
Whereas aberrating layers are typically viewed as forming an impediment to medical ultrasound imaging, they can surprisingly also be used to our benefit. As long as we can model the effect of an aberrating layer,we can utilize ‘model-based imaging’, the imaging technique explored throughout this thesis, to reconstruct ultrasound images where traditional beamforming methodswould fail, employing the ever increasing computational power available to us nowadays. Not only does this allow us to image through layers, but it also leads to interesting applications, such as 3D ultrasound imaging with spatially undersampled data, using an aberrating ‘coding mask’. The formulation of a measurement model, a fundamental part of model-based imaging, also gives insight into the imaging problem mathematically, and allows us to investigate methods for estimating the effect of an aberrating layer ‘blindly’, i.e., without explicitly measuring it.
In this thesis, we thus investigate (a), imaging through a layer when the layer’s aberration effect is known, and how it can be applied to imaging with spatially undersampled data, and (b), methods and algorithms for estimating the effect of the aberrating layer without knowing it a priori.
In the first part of this thesis, we illustrate how using model-based imaging can be utilized for 3D ultrasound imaging using a single ultrasound transducer, and equipping it with a plastic coding mask. The plastic mask acts as an analog coder, that scrambles the transmitted and received waves in a manner that is location dependent. As a result, the temporal shape of an ultrasound echo can be used instead of the traditional method of using phase differences between sensors in a sensor array. Imaging is instead accomplished using model-based imaging. By measuring the pulse-echo response of each pixel, we can form an image by solving a regularized linear least squares problem, which takes into account the measured pixel-specific pulse-echo signals. The proposed device and imaging method is then verified experimentally.
In the following chapter, a coding mask design method is proposed for the aforementioned imaging device. A measurement model is formulatedwhere themask geometry is an explicit parameter to be optimized. After forming this model, a numerical optimization method is proposed and numerically tested. Our numerical experiments show that optimized mask geometries exhibit an energy focusing effect on the region-of-interest, whilst simultaneously decorrelating echo signals between pixels.
In the second part of this thesis, in contrast, we consider methods for calibrating propagation models when the pulse-echo response per pixel is not known. The most important calibration challenge we consider is that of imaging through an aberrating layer in front of an ultrasound array. This could be subcutaneous fat or the human skull, for example. In this thesiswe formulate ameasurement model consisting of a partwhere wave propagation is known (i.e., the assumed homogeneous region behind the aberrating layer, where the contrast image of interest is located), and an unknown propagation part, consisting of the Green’s functions from an array sensor to any point on the the interface of the aberrating layer and the imaging medium. We then investigate methods for finding this set of Green’s functions without explicitly measuring them (so called ‘blind’ calibration).
The first proposed method exploits the singular value decomposition of the measurement data in combination with the assumed Toeplitz structure of the matrices representing the aberrating layer’s Green’s functions. However, the method is lacking in practicality since an additional set ofmeasurements is required with a phase screen mounted on the interface of the aberration layer and the imaging medium. The second method resolves these practical issues by utilizing a covariance matching technique. A sufficiently large set of measurements is obtained where each measurement is different due to e.g. moving particles such as blood flow or micro-bubbles. Using the covariance of the data, algorithms are then defined that can estimate the transfer functions of the aberrating layer from the measurement covariance data.
Finally, we propose a method for estimating the electro-mechanical impulse response of an ultrasound sensor, by simply measuring its pulse-echo response from a flat plate reflector in front of the sensor. Estimating the one-way (electro-mechanical) impulse response then becomes a de-autoconvolution problem, for which we propose a method by solving a semi-definite relaxation of the de-autoconvolution problem.
Additional information ...
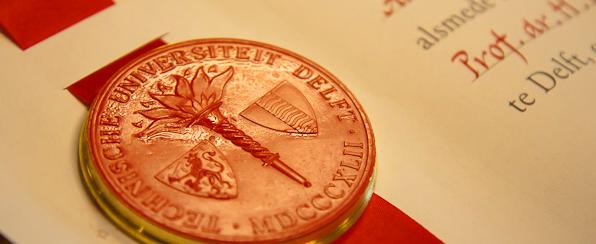
Signal Processing Seminar
- Tuesday, 31 January 2023
- 11:00-12:00
- HB 17.140
A framework for augmented reality visualization of simulated and experimental ultrasound images
Adrian BasarabUniversity of Lyon
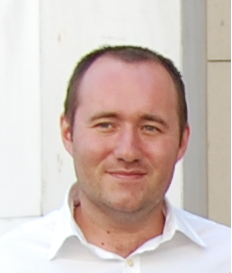
In a number of clinical applications, such as breast cancer surgery, ultrasound medical images are acquired and analyzed prior to or during the intervention. However, these images are no longer available during the intervention, or are only visible on a computer screen, thus imposing important constraints to practitioners. Moreover, 2D ultrasound images are still the clinical standard, while the tissues of interest are naturally 3D.
The main idea of this work is to construct a framework whose objective is to fill the gap between 2D multimodal acquisitions and real-time visualization in augmented reality of 3D reconstructed volumes, at the time of intervention. In particular, the talk will focus on: i) an experimental platform able to visualize ultrasound images in augmented reality in real time, and ii) an efficient ultrasound image simulation method mimicking freehand scanning of a virtual 3D volume, allowing real-time visualization of the simulated images in augmented reality.
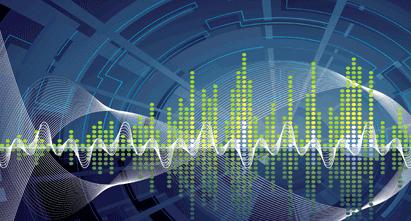
MSc SPS Thesis presentation
- Tuesday, 31 January 2023
- 15:45
- HB17.140
Digital self-timed neuron design for Spiking Neuron Networks
Tianyu DuInnatera
Spiking Neural Networks(SNN) have been widely leveraged by neuromorphic systems due to their ability to closely mimic biological neural behavior, where information is exchanged and received between neurons in the form of sparse events(spikes). Such neuromorphic systems are highly energy-efficient because the use of a global clock can be avoided by asynchronous event-driven operations. Neurons, as the basic processing units of neuromorphic systems, are required to be lowpower and high-speed for the implementation of complex networks. In this work, two fully event-driven digital Integrate-and-Fire(IF) neuron design is presented. Both design exploits the hierarchical structure, which allows the synaptic weights can be accumulated by local compute units in parallel. Instead of using handshake protocols, the proposed design generates on-demand event pulses to drive the weight accumulation, so we call it self-timed. Both neurons are designed by SystemVerilog and synthesized in TSMC 28nm technology. According to the synthesis results, both designs can finish the accumulation of 1024 6-bit weights within 100ns, with a power consumption of 0.055pJ per spike and 0.23pJ per spike respectively.
MSc SPS Thesis presentation
- Monday, 30 January 2023
- 15:45
- HB17.140
On-chip Self Timed SNN Custom Digital Interconnect System
Jiongyu HuangInnatera
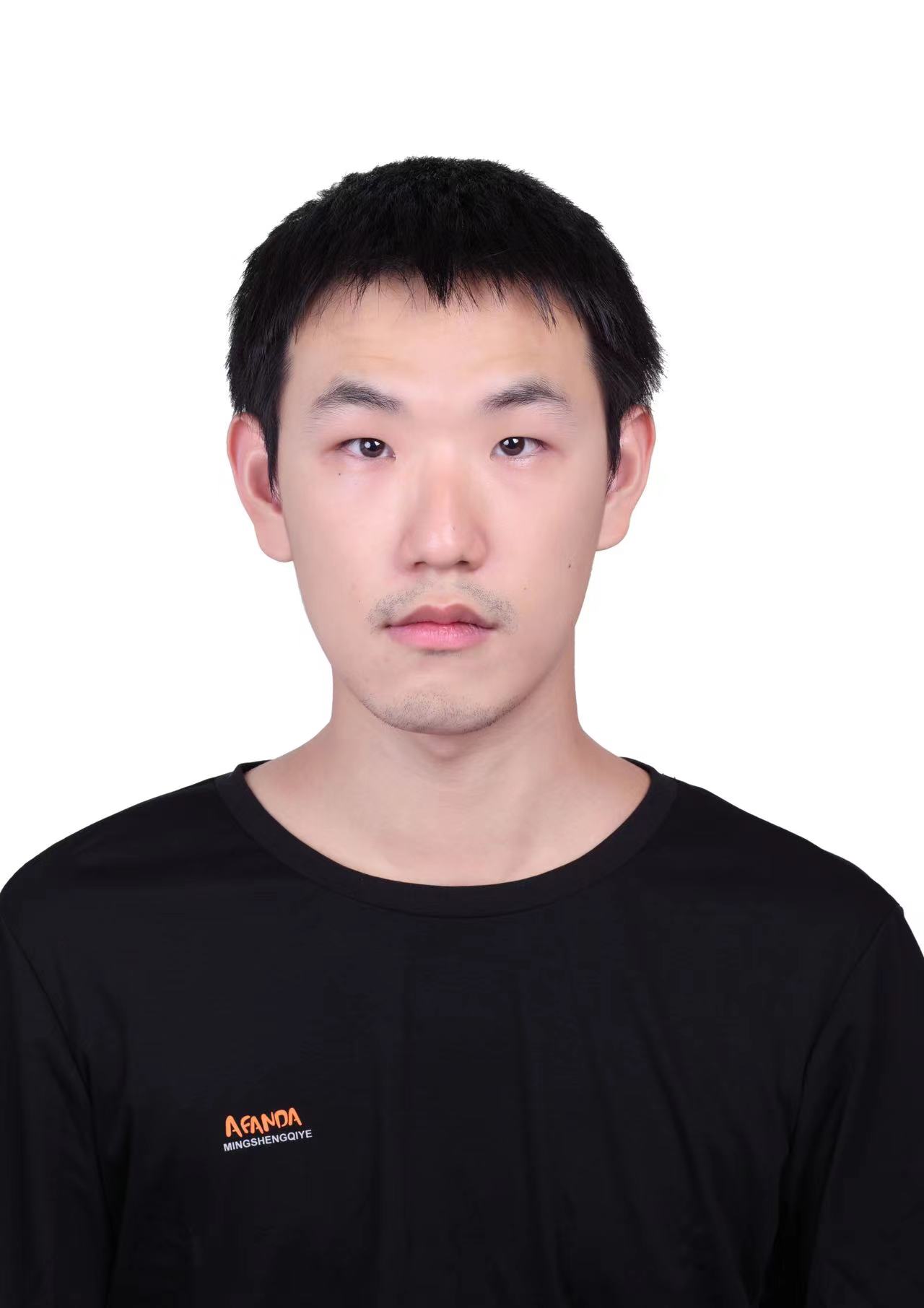
A Spiking neural network (SNN) is a type of artificial neural network which encodes information using spike timing, network structure, and synaptic weights to emulate the information processing function of the human brain. Within an SNN, it is always required to support the spike transmission that travels between neurons(array). This thesis aims to design a customized high-speed interconnect system which supports multi-point communication in a neuromorphic computing system. The burst-mode two-wire protocol in point-to-point communication is applied in this interconnect system, which is designed in high-level modelling with SystemC. In order to improve the utilization of hardware resources, a virtual channel system is involved.
Furthermore, this system could be extended to a variable number of neuron arrays to support different types of spiking neural networks. Also, optimization methods are adopted to increase the transmission rate of the system and save unnecessary energy consumption. The interconnect system could achieve a throughput of 3.802 Gbits/s with the given MNIST use case, based on the evaluation of simulation results.
MSc ME Thesis presentation
- Tuesday, 24 January 2023
- 11:00
- HB13.140, EWI
Physics-Informed Data Augmentation for Human Radar Signatures
Edoardo FocanteTNO
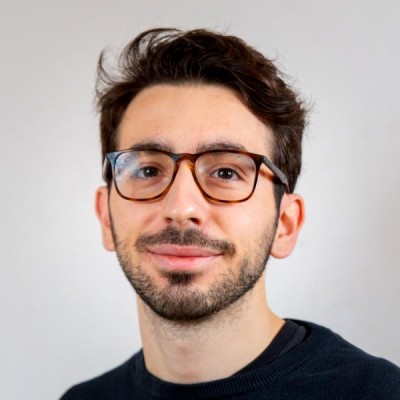
In recent years, neural networks (NNs) have seen a surge in popularity due to their ability to model complex patterns and relationships in data. One of the challenges of using NNs is the requirement for large amounts of labelled data to train the model effectively. In many real-world applications such as radar, labelled data may be scarce due to the high cost of acquiring measurements together with privacy and security concerns.
To overcome the lack of data, researchers have resorted to data augmentation (DA), a technique that aims to solve the problem at the root by generating new training samples by leveraging the available ones. In computer vision, image transformations and generative networks are used to perform DA. These techniques, however, may lead to the production of physically unfeasible samples that may hinder the generalization capabilities of classifiers in domains where the data has an underlying physical meaning.
Physics-informed machine learning aims to incorporate physical prior knowledge and governing equations of the target domain into the machine learning pipeline to improve the performance of NNs in fields with limited available data but with well-defined physical models. In this thesis, physics-informed DA in the radar domain is addressed to improve the task of classifying armed and unarmed walking individuals through micro-Doppler spectrograms.
To begin with, the usage of model-driven micro-Doppler radar simulations to improve the existing generative augmentations is investigated. After introducing several generative NN architectures, the quality and diversity of the produced synthetic spectrograms are evaluated together with their effect on the downstream classification task.
Next, the impact of visual transformations on micro-Doppler spectrograms is studied. Their effect on the underlying physics of the micro-Doppler spectrograms and their impact on feature extraction is assessed. Based on the results, a new DA technique is devised to improve the feature extraction process by informed segmentation of the input spectrogram, halving the size of the NN model and reducing the risk of overfitting.
IEEE Autonomous Systems Initiative Webinar
- Wednesday, 21 December 2022
- 17:00-18:00
- zoom
Active inference in cognitive neuroscience
Prof. Karl FristonUniversity College London
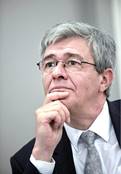
In the cognitive neurosciences and machine learning, we have formal ways of understanding and characterising perception and decision-making; however, the approaches appear very different: current formulations of perceptual synthesis call on theories like predictive coding and Bayesian brain hypothesis. Conversely, formulations of decision-making and choice behaviour often appeal to reinforcement learning and the Bellman optimality principle. On the one hand, the brain seems to be in the game of optimising beliefs about how its sensations are caused; while, on the other hand, our choices and decisions appear to be governed by value functions and reward. Are these formulations irreconcilable, or is there some underlying imperative that renders perceptual inference and decision-making two sides of the same coin.
Speaker biography
Karl Friston is a theoretical neuroscientist and authority on brain imaging. He invented statistical parametric mapping (SPM), voxel-based morphometry (VBM) and dynamic causal modelling (DCM). These contributions were motivated by schizophrenia research and theoretical studies of value-learning, formulated as the dysconnection hypothesis of schizophrenia. Mathematical contributions include variational Laplacian procedures and generalized filtering for hierarchical Bayesian model inversion. Friston currently works on models of functional integration in the human brain and the principles that underlie neuronal interactions. His main contribution to theoretical neurobiology is a free-energy principle for action and perception (active inference).
Friston received the first Young Investigators Award in Human Brain Mapping (1996) and was elected a Fellow of the Academy of Medical Sciences (1999). In 2000 he was President of the international Organization of Human Brain Mapping. In 2003 he was awarded the Minerva Golden Brain Award and was elected a Fellow of the Royal Society in 2006. In 2008 he received a Medal, College de France and an Honorary Doctorate from the University of York in 2011. He became of Fellow of the Royal Society of Biology in 2012, received the Weldon Memorial prize and Medal in 2013 for contributions to mathematical biology and was elected as a member of EMBO (excellence in the life sciences) in 2014 and the Academia Europaea in (2015). He was the 2016 recipient of the Charles Branch Award for unparalleled breakthroughs in Brain Research and the Glass Brain Award, a lifetime achievement award in the field of human brain mapping. He holds Honorary Doctorates from the University of Zurich and Radboud University.
Zoom link
Please register for the webinar, here.
IEEE SPS BISP-TC Webinar
- Tuesday, 6 December 2022
- 15:00-16:00
- webex
Tensor Decompositions in Functional Neuroimaging
Borbála Hunyadi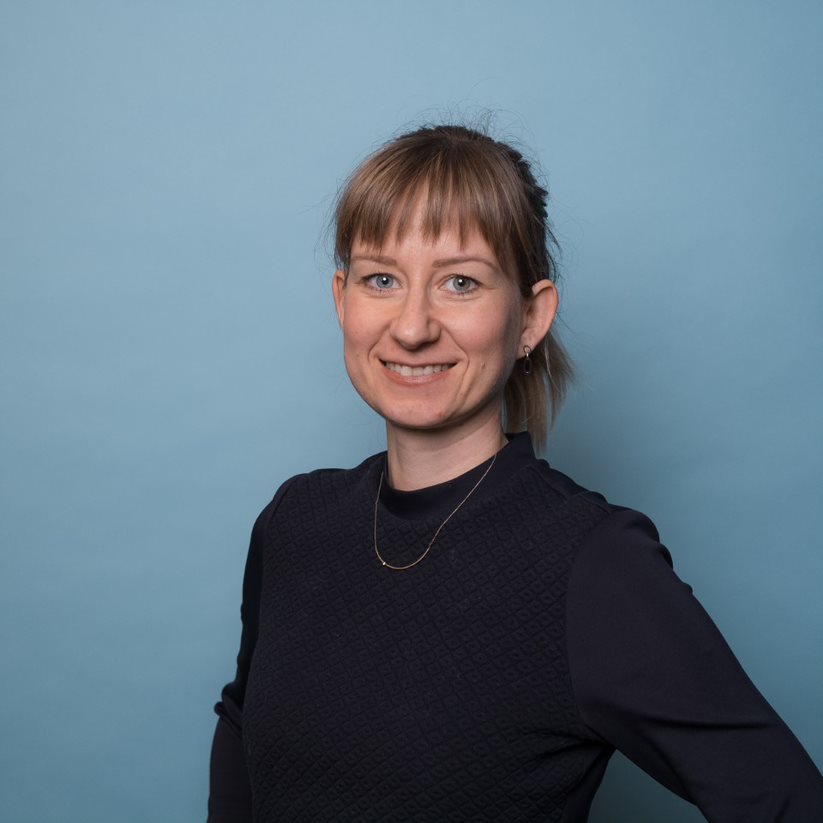
Brain data are inherently large scale, multidimensional, and noisy. Indeed, advances in imaging and sensor technology allow recordings of ever-increasing spatio-temporal resolution. Multidimensional, as time series data are recorded at multiple locations (electrodes, voxels), from multiple subjects, under various conditions. Finally, the data are noisy: the recorded observations are a mixture of ongoing brain activity, physiological, and non-physiological noise sources. Tensors (higher order arrays) are the natural representations of such multidimensional data. Tensor decompositions, in general, aim to write a large and high-order tensor in terms of the product and summation of several smaller and low-rank tensors (including vectors and matrices). A tensor decomposition with a well-chosen number of terms and ranks can approximate the original data tensor using much fewer entries; even to capture the underlying sources separately in its individual components. This talk will first give an introduction to multilinear algebra and tensor decompositions, discuss current challenges in large-scale brain data analysis, and finally highlight some successful applications of tensor decompositions in EEG and functional ultrasound (fUS) data processing.
Registration
Follow this link.
Additional information ...
IEEE SPS Autonomous Systems Initiative
- Wednesday, 30 November 2022
- 17:00-18:00
- zoom
Computational Self-awareness and Self-organization: A Paradigm for Building Adaptive, Resilient Computing Platforms
Dr. Nikil DuttUniversity of California, Irvine
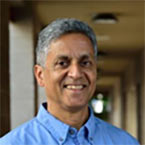
Self-awareness and self-organization have a long history in biology, psychology, medicine, engineering and (more recently) computing. In the past decade this has inspired new self-aware/self-organizing strategies for building resilient computing platforms that can adapt to the (often conflicting) challenges of resiliency, energy, heat, cost, performance, security, etc. in the face of highly dynamic operational behaviors and environmental conditions. I will begin by outlining a computational self-awareness paradigm that enables adaptivity and which supports system resilience. Computational self-awareness is achieved through introspection (i.e., modeling and observing its own internal and external behaviors) combined with both reflexive and reflective adaptations via cross-layer physical and virtual sensing and actuations applied across multiple layers of the hardware/software system stack.
Next I will outline strategies for combining computational self-awareness with self-organization for life-cycle management of dependable distributed computing platforms. Our ongoing NSF/DFG Information Processing Factory (IPF) project applies principles inspired by factory management that combine self-awareness and self-organization for continuous operation and optimization of highly-integrated-but-distributed embedded computing platforms. While each IPF computational component exhibits autonomy through self-awareness, collections of IPF entities can self-organize; the resulting emergent behavior must be controlled to ensure guaranteed service even under strict safety and availability requirements. I will outline two use cases: i) End-to-end computational pipelines for a single autonomous IPF component, and ii) Truck platooning as an exemplar for distributed-but-coupled IPF autonomous systems. The talk will conclude with the opportunities and challenges arising from adopting computational self-awareness and self-organization for making complex computational systems more resilient and self-adaptive.
Zoom link: see below.
Additional information ...
MSc ME Thesis presentation
- Wednesday, 30 November 2022
- 14:00-16:00
- HB17.140
Self-timed interconnect for SNN - From Point to Point Communication to Multi-array Segmented-bus solution
Jinyao Zhang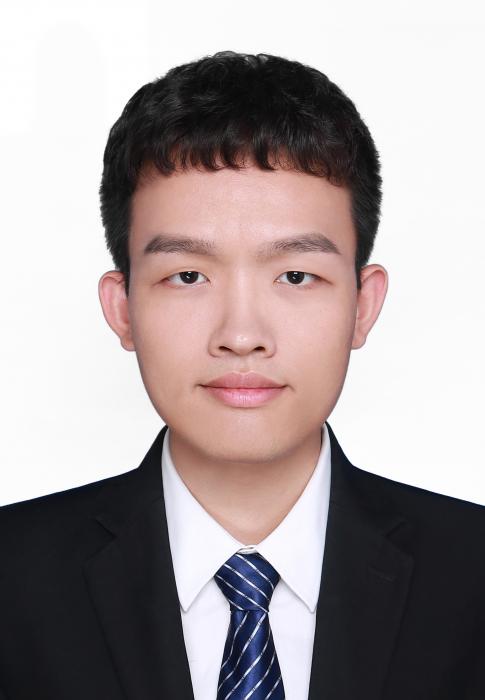
Spiking Neural Networks use Address Event Representation to communicate among different Neuron Arrays. To mimic the behavior of the human neural system and meets the requirement for large Neuron Array communication, the AER interconnect should be area-saving, have low power, and operates at high speed.
This thesis aims to build self-timed interconnects for point-to point and multi-array communication. The whole system is designed at the RTL level using SystemVerilog. For point-to-point communication, two transmitters are implemented and compared according to their synthesis results. In the multi-array communication structure, we develop a generalized segmented-bus topology and the element fence, to control its segments. Different timing problems in the design are analyzed and corresponding solutions are proposed. The whole system can operate at around 1Gbps in a self-timed manner without any timing problems.
MSc ME Thesis Presentation
- Tuesday, 29 November 2022
- 15:00-15:40
- HB17.140
A New Logarithmic Quantization Technique and Corresponding Processing Element Design for CNN Accelerators
Longxing Jiang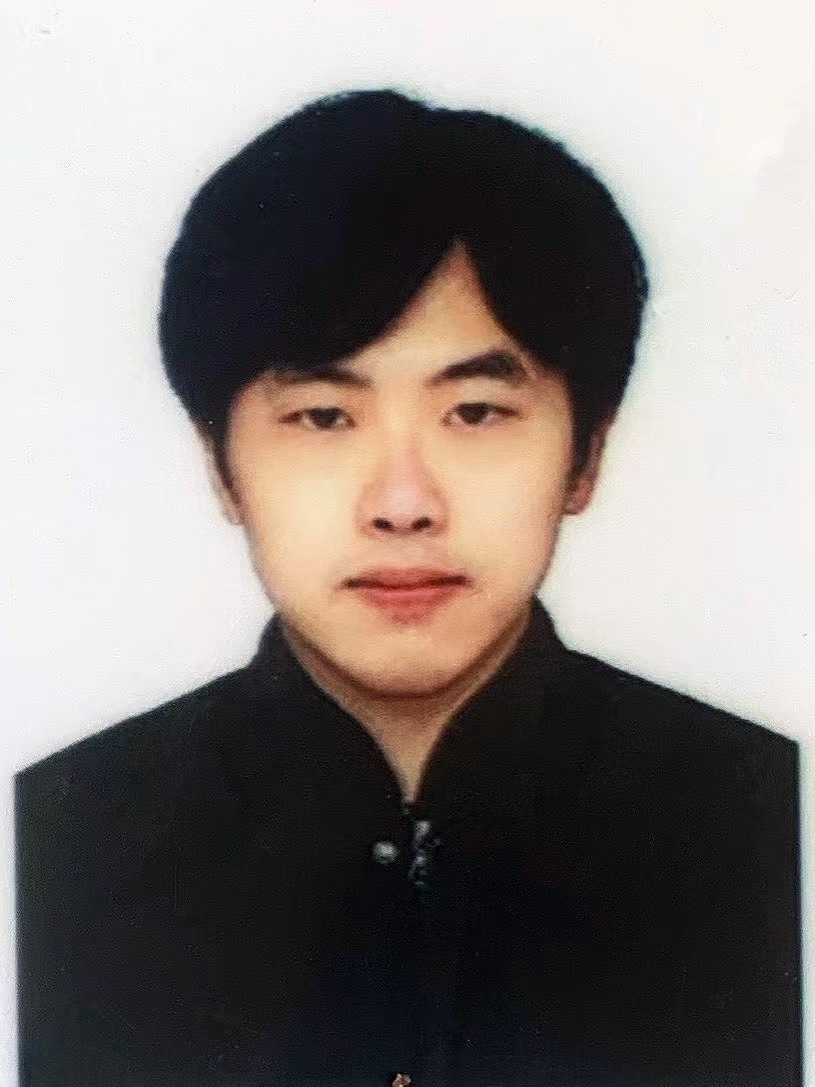
Convolutional Neural Networks (CNN) have become a popular solution for computer vision problems. However, due to the high data volumes and intensive computation involved in CNNs, deploying CNNs on low-power hardware systems is still challenging. The power consumption of CNNs can be prohibitive in the most common implementation platforms: CPUs and GPUs. Therefore, hardware accelerators that can exploit CNN parallelism and methods to reduce the computation burden or memory requirements are still hot research topics. Quantization is one of these methods.
One suitable quantization strategy for low-power deployments is logarithmic quantization.
Logarithmic quantization for Convolutional Neural Networks (CNN): a) fits well typical weights and activation distributions, and b) allows the replacement of the multiplication operation by a shift operation that can be implemented with fewer hardware resources. In this thesis, a new quantization method named Jumping Log Quantization (JLQ) is proposed. The key idea of JLQ is to extend the quantization range, by adding a coefficient parameter ”s” in the power of two exponents (2sx+i ).
This quantization strategy skips some values from the standard logarithmic quantization. In addition, a small hardware-friendly optimization called weight de-zeroing is proposed in this work. Zero-valued weights that cannot be performed by a single shift operation are all replaced with logarithmic weights to reduce hardware resources with little accuracy loss.
To implement the Multiply-And-Accumulate (MAC) operation (needed to compute convolutions) when the weights are JLQ-ed and dezeroed, a new Processing Element (PE) have been developed. This new PE uses a modified barrel shifter that can efficiently avoid the skipped values.
Resource utilization, area, and power consumption of the new PE standing alone and in a systolic array prototype are reported. The results show that JLQ performs better than other state-of-the-art logarithmic quantization methods when the bit width of the operands becomes very small.
MSc ME Thesis Presentation
- Monday, 28 November 2022
- 15:00-15:40
- HB17.140
Off-chip Self Timed SNN Custom Digital Interconnect System
Yichen Yang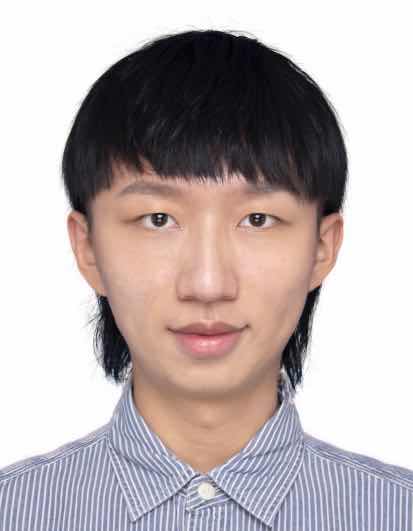
To support the spike propagates between neurons, neuromorphic computing systems always require a high-speed communication link.
Meanwhile, spiking neural networks are event-driven so that the communication links normally exclude the clock signal and related blocks.
This thesis aims to develop a self-timed off-chip interconnect system with ring topology that supports multi-point communication in neuromorphic computing systems. This interconnect system is implemented in high-level modeling with SystemC and involves the burstmode two-wire protocol in point-to-point communication. In order to ensure the flexibility of the system, the distributed control system is involved. Further, the system can be configured with different numbers of chiplet to fulfill various spiking neural network structures.
We also explore optimization methods, which is a bi-directional ring topology achieving the growth of throughput. Based on evaluation and simulation results, the interconnect system can achieve 4.57Gbps with the specific application scenario.
Additional information ...
MSc CE Thesis Presentation
- Monday, 28 November 2022
- HB17.140
Mapping of Spiking Neural Network Architecture using VPR
Jinyun Long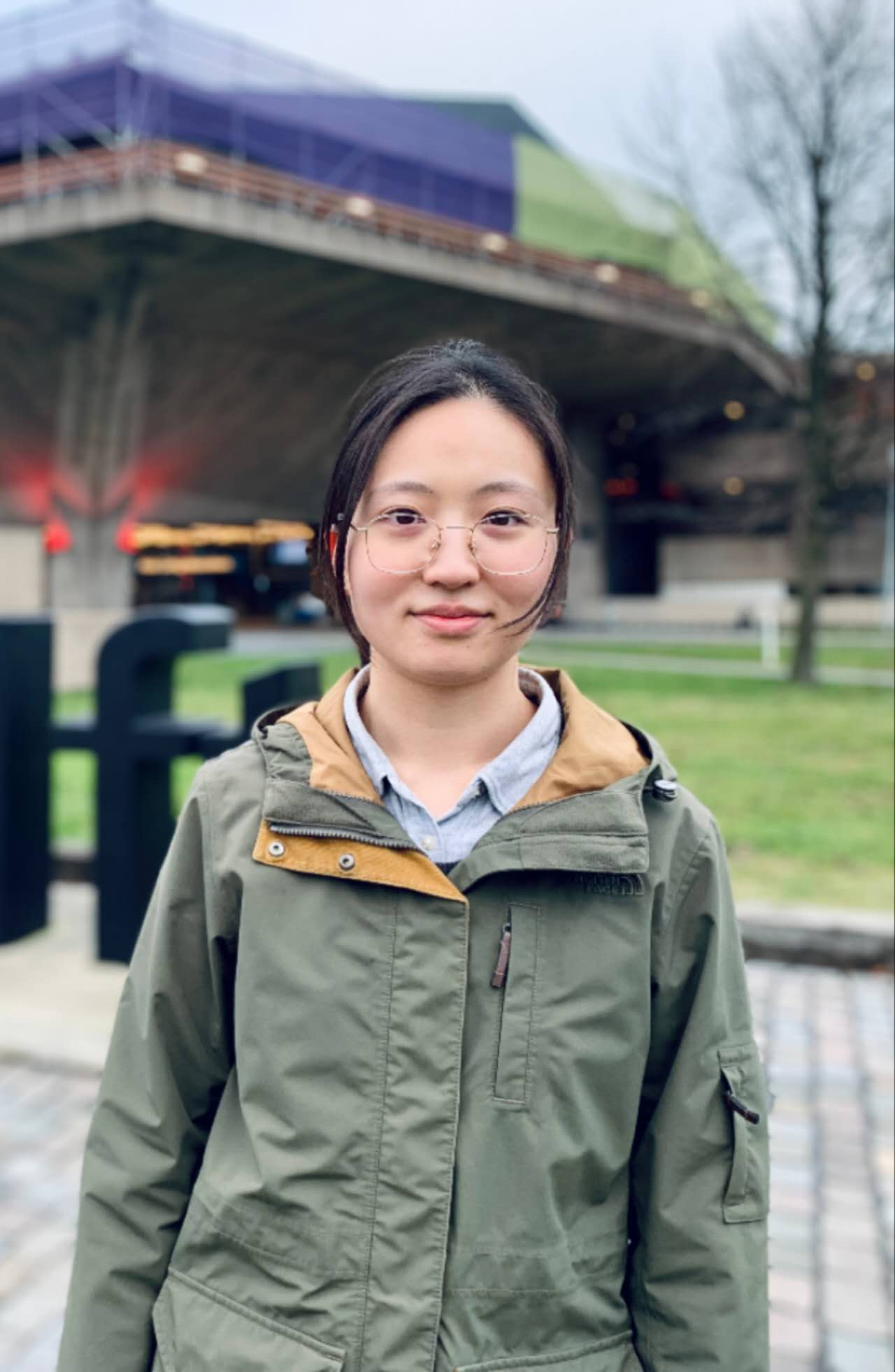
As the new generation of neural networks, Spiking Neural Network architectures executes on specialized Neuromorphic devices. The mapping of Spiking Neural Network architectures affects the power consumption and performance of the system. The target platform of the thesis is a hardware platform with Neuromorphic Arrays with columns for neural signal processing.
The explorations for the mapping methods are based on VPR, an open-source academic CAD tool for FPGA architecture exploration.
The packing of VPR is used for mapping neurons to Neuromorphic Arrays. VPR includes two levels of mapping: pins and neurons.
An evaluation of the mapping methods is established. Based on the evaluation, the optimized mapping solution is generated. Modifications are made in VPR to adapt to SNN architectures. An ActivityCriticality input file is added to the VPR flow for the optimized mapping solution.
Additional information ...
MSc ME Thesis Presentation
- Monday, 31 October 2022
- 10:00-10:45
- EWI - Meeting room 15.1
A Low-Noise Transimpedance Amplifier for Ultrasound Imaging with 40dB Continuous-Time Gain Compensation
Qian Wang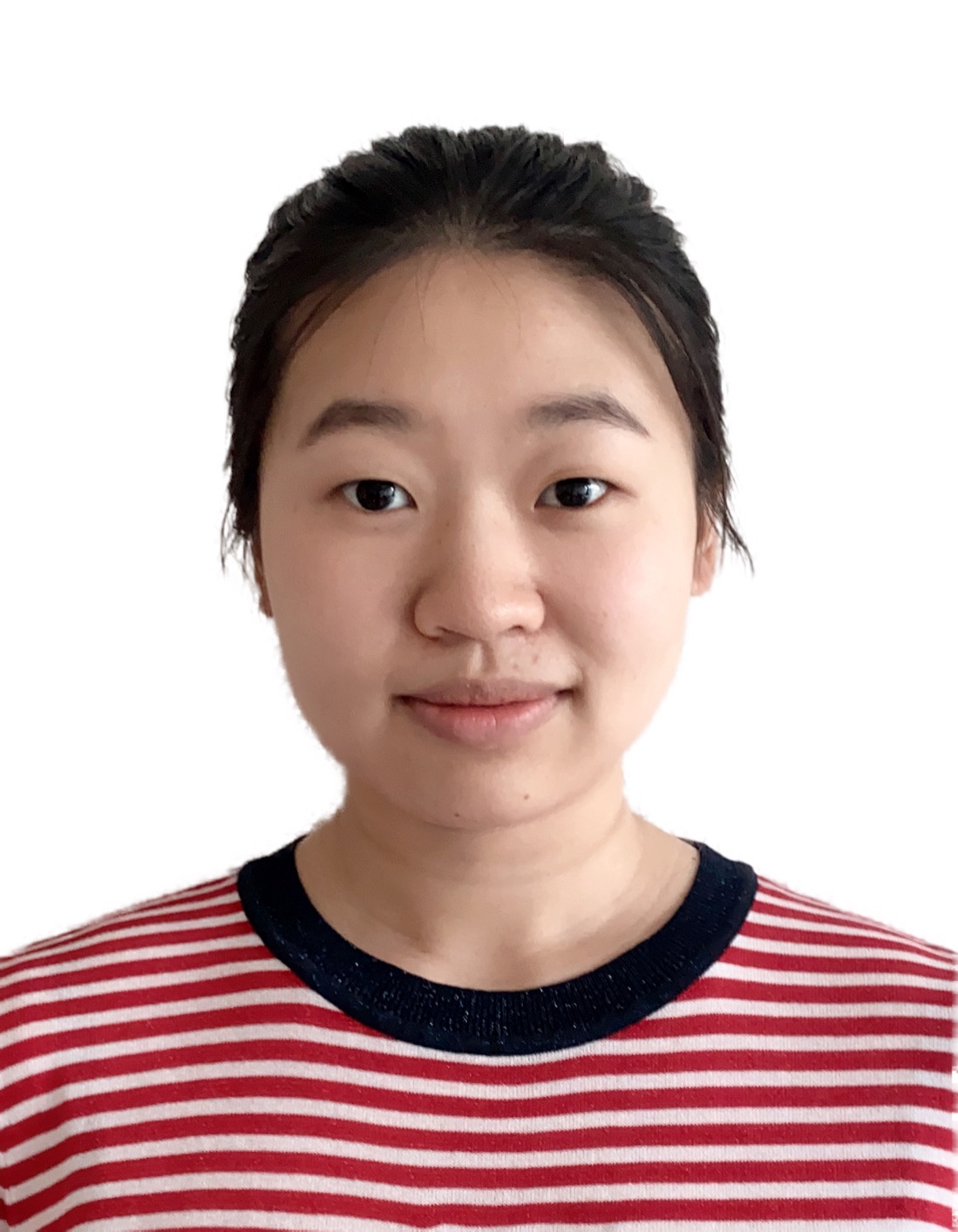
This work presents a low-noise amplifier (LNA) for miniature 3D ultrasound probes. Time gain compensation (TGC) is required to provide continuously variable gain and compensate for the attenuated echo signal, resulting in decreased output dynamic range (DR). As TGC is embedded in the LNA, a power-hungry LNA is no longer needed to handle the full dynamic range of attenuated echo signal. Compared to prior art where TGC is applied after the LNA, this structure reduce die area and power consumption greatly.
The LNA with built-in TGC functionality is comprised of a transimpedance amplifier (TIA) with exponentially increasing feedback resistive network. Since a transducer with a relatively high impedance is targeted, a TIA is utilized to interface with the tranducer and sense the signal current. TGC is implemented in a continuous fashion by tunable resistors so as to alleviate imaging artifacts associated with gain switching moments. The resistive feedback network is achieved by triode transistors with exponentially decreasing gate voltages. Three parallel branches of triode transistors are varied simultaneously to obtain 40dB gain range. Each branch consists of two back-to-back triodes to mitigate non-linearity related to the body effect.
The variable-gain loop amplifier employing a current-reuse topology enables constant closed-loop bandwidth in an energy-efficient way. The first stage is a fixed-gain stage with dynamic biasing to save power at the lowest gain setting. The next two stages are variable-gain stages with variable resistive loads. The load resistor is implemented in the same fashion as the TIA’s feedback resistor to achieve intrinsic gain matching. The last stage is a buffer to provide low output impedance for stability.
The LNA has been designed in 0.18 μm CMOS technology and occupies an estimated die area of 0.0339 mm2. The effective gain range is 40 dB with ±1 dB gain error. The LNA’s noise floor at the highest gain is below 1.15 pA/rt-Hz and its harmonic distortion is better than -40 dB. During 100 μs receive period, the total power consumption is 6mW from a ±0.9 V supply. The LNA featuring small area and high power efficiency is a promising circuit for miniature 3D ultrasound probes.
MSc ME Thesis presentation
- Friday, 28 October 2022
- 09:30
- LB01.010, Snijderszaal
Autonomous Landing of an Unmanned Aerial Vehicle
Siddhy Ganesh Shetty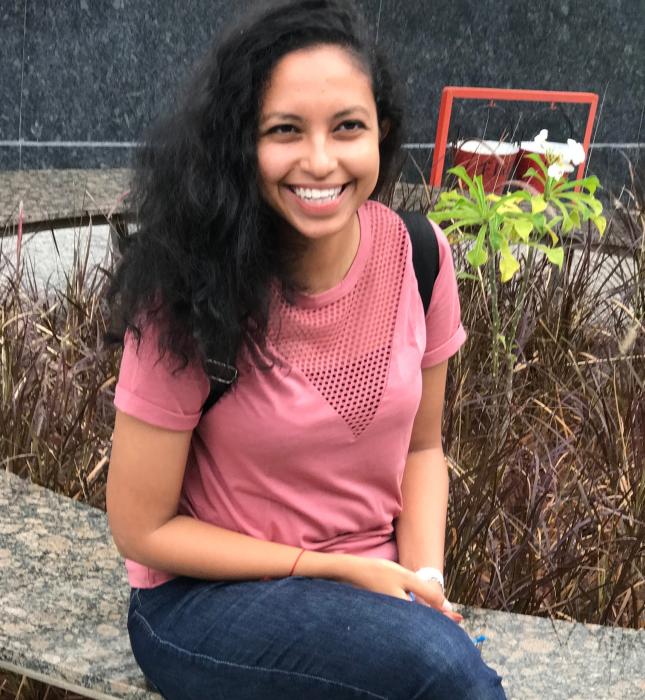
Abstract:
With the advancement in technology, Unmanned Aerial Vehicles (UAVs) have been able to safely maneuver in risky environments. During landing, the UAV should slow down while not affecting its physical design. Currently, multiple sensors are being used to increase the accuracy while landing which might weigh down some of the smaller UAVs. The usage of a single sensor to guarantee safe landing is yet on its early stages of development. It was observed that insects use Optical Flow to maneuver, and this has been used as a method to design UAV's journey and land safely. By dividing each frame, an Optical Flow Difference is calculated which is used as a metric for the UAV to move towards the landing platform.
Once the UAV has positioned itself on top of the elevated landing platform, image dilation method is used to safely land the UAV. One of the methods tested used Image Dilation Method using IMU. Using the calculated image dilation, the control input to the UAV is generated and the UAV lands. This method failed in providing safe landing and it also did not take the vision of the UAV into consideration. Then, Image Dilation Method using Features from Accelerated Segment Test (IDMF-AST) was tested. This method tracks features observed by the UAV and calculated an estimate of the image dilation. The estimate of image landing is used to control the UAV. This showed dependency on the landing design platform and the hyperparameters that are used for implementing IDMF for the type of landing.
Different landing designs were tested for different elevated landing platforms. It was observed that concentric circles on a textured landing marker provided the highest probability of safe landing. To tackle the dependency of the hyperparameters, a Classification Model was proposed to find an optimal set of hyperparameters for each possible height of the platform. This trained model was incorporated with the IDMF, thereby designing the proposed Adaptive IDMF algorithm. The Adaptive IDMF was tested against the original IDMF on the metrics of safe landing probability, time taken to safely land and simulation time. Adaptive IDMF performed better compared to IDMF providing an 190% increase in probability of safe landing, faster safe landing and lesser simulation and compilation time.
Additional information ...
Microelectronics Colloquium
- Thursday, 27 October 2022
- 15:30-16:30
- LB 1.010 Snijderszaal
Advances in Low-Field MRI Hardware Design and Data Processing
Rob Remis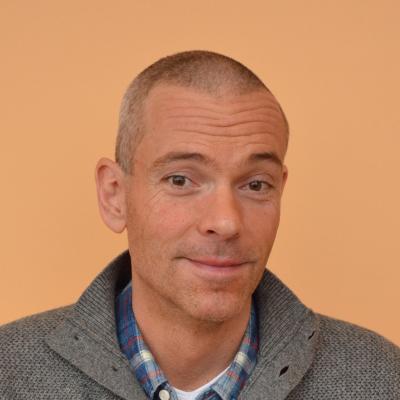
In this talk we discuss several recent advances in low-field Magnetic Resonance Imaging (MRI). We focus on magnet and gradient coil design for a low-field MR scanner in which the strong background field is generated by permanent magnets (Halbach systems). These design problems are treated as inverse source problems, which are severely ill-posed in general. How to obtain approximate (regularized) solutions to these problems is discussed and the practical implementation of these solutions is addressed as well. Several processing algorithms that can handle compressed noisy MR input data are also presented and we illustrate the performance of these algorithms on simulated and measured low-field MR data.
Additional information ...
MSc ME Thesis presentation
- Friday, 21 October 2022
- 13:30-16:30
- HB17.150/140 - CAS Seminar room
Energy-efficient seizure detection for wearable EEG
Xiaoning Shi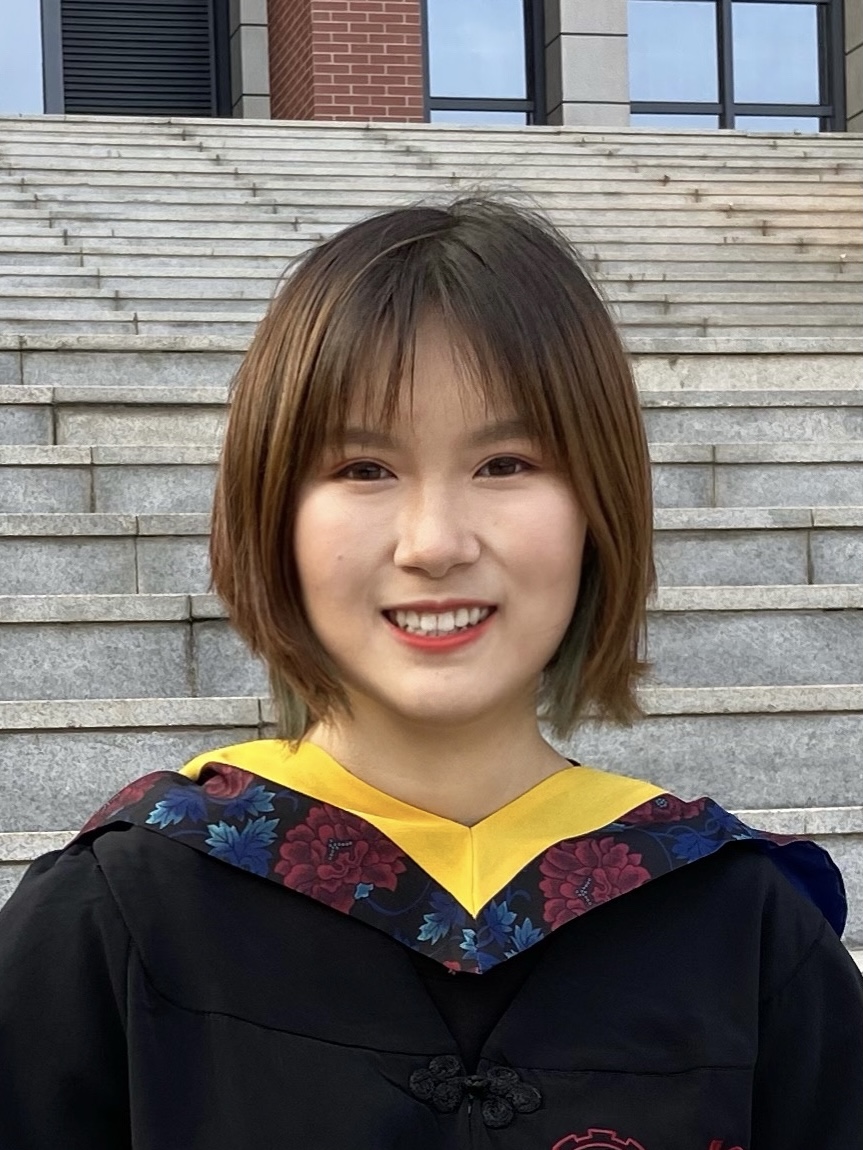
MSc ME Thesis presentation
- Monday, 17 October 2022
- 09:30-11:30
- HB17.140/150
A trainable activation scheme for applying Neural Radiance Fields in novel view synthesis tasks
Chuhan Wang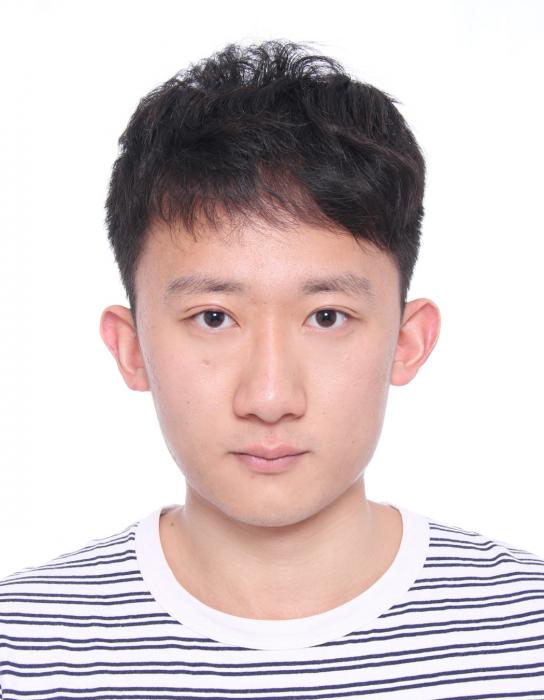
3D scene reconstruction is a common computer vision task with many applications. The synthesized virtual environments are beneficial for many downstream applications such as 3D modeling, building inspection, virtual reality, etc. As conventional scene reconstruction methods often require expensive data collections and prior information on the geometry, a learning-based method named Neural Radiance Fields (NeRF) has gained a surge of interest within the computer vision community recently for its capacity to achieve state-of-the-art view synthesis performance and achieve photorealistic rendering from only a sequence of RGB images as the input. However, NeRF has a strict requirement for input images with accurate camera poses, which is often not available in real-life applications. To this end, we provide an end-to-end guideline for 3D scene reconstruction using NeRF under a real-world scenario the objective is to reconstruct the HDB facade in Singapore. This guideline requires only RGB images as the input and can achieve photorealistic rendering results which are competitive with the results from the conventional point cloud-based method. Besides, we build our own models upon NeRF and also improve its performance in representing fine details. We first research that the ability to represent high-frequency contents in the signal is limited by its ReLU activations in the Multi-layer Perceptron (MLP) network, and demonstrate that mapping the input to the MLPs from low dimensional space to high dimensional space significantly improves the reconstruction and view synthesis quality. Afterward, We make several attempts to perform input mapping. We first use Gaussian-distributed Fourier features to replace the original positional encoding used in NeRF. Then, we research the activations in coordinate MLP and propose an embedding-less NeRF model equipped with parameterized sine activations called SIRENeRF. Next, we extend the use of parameterized activations from sine activations to a class of non-periodic activations and propose a trainable activation scheme that not only achieves higher scene reconstruction results but also enjoys better flexibility to different datasets. Experiments show that all of the above attempts outperform the positional encoding in terms of scene reconstruction and view synthesis results.
MSc ME Thesis presentation
- Friday, 14 October 2022
- 14:30
- Online
Nowcasting of extreme precipitation using deep generative model
Haoran Bi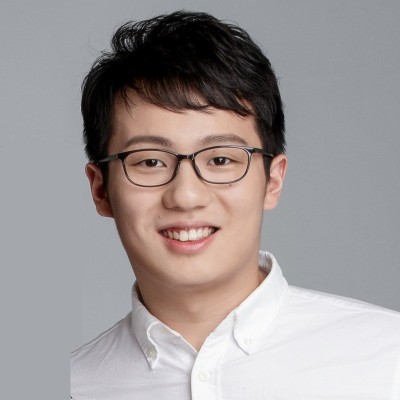
Extreme precipitation can often cause serious hazards such as flooding and landslide. Both pose a threat to human lives and lead to substantial economic loss. It is crucial to develop a reliable weather forecasting system that can predict such extreme events to mitigate the effect of heavy precipitation and increase resilience to these hazards.
Numerical Weather Prediction (NWP) models play the dominant role in the field of weather forecasting. However, due to their long computational time, these models had limited utility in predicting weather conditions in the following several hours. This gap is filled by nowcasting, an observation-based method that uses the current state of the atmosphere to forecast future weather conditions for several hours. Operational nowcasting systems typically apply extrapolation algorithms to rainfall radar observations based on simple physics assumptions. However, the physics constraints also limit the performance, and the methods can hardly capture non-linear patterns in the radar observations. Besides the conventional methods, deep learning models have started to play an essential role in this field. Recent works have shown the promising potential of using deep learning models to tackle the nowcasting task, which is also this thesis's focus.
The thesis work mainly studied in two directions: the development of novel deep generative models for precipitation nowcasting and the application of statistical approaches for better modeling and prediction of extreme events. For the first direction, our proposed model is inspired by recently developed deep learning models from the field of visual synthesis. The model makes use of a two-stage structure: the first stage is a Vector Quantization Variational Autoencoder (VQ-VAE) which compresses the original high-resolution radar observations into a low-dimensional latent space. The second stage works in this latent space. It contains an autoregressive Transformer that models the probabilistic distribution of latent space data. The trained Transformer can predict the latent space representation of future frames. ForT better modeling and prediction of extreme events, Extreme Value Loss (EVL) is proposed and incorporated with the autoregressive Transformer. The loss function aims at penalizing predicting extreme cases as non-extreme and predicting non-extreme cases as extreme in order to solve the high imbalance between extreme and normal precipitation data. Our results show that the proposed model shows comparable performance with the state-of-the-art conventional method and other deep learning nowcasting models. The proposed EVL has also been shown to improve the overall performance and accuracy in predicting extreme events.
Additional information ...
MSc ME Thesis presentation
- Friday, 14 October 2022
- 10:45-12:46
- Hall Chip, HB01.600
IMU-Based Adaptive Filtering for Movement Artifact Removal from ECG Recorded With a Single Lead Wearable Device
Cesar Eduardo Cornejo Ramirez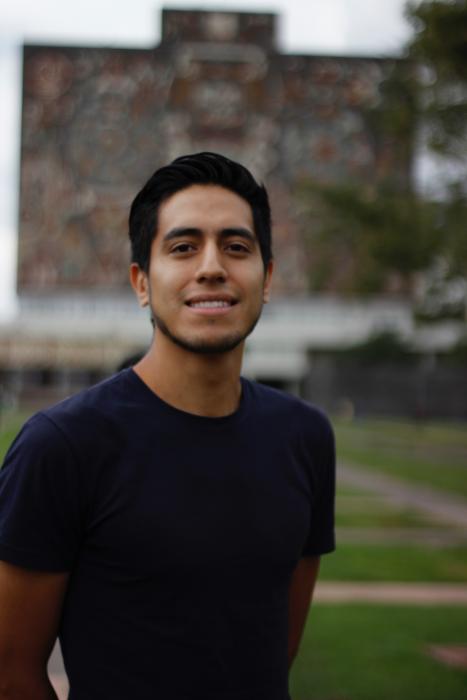
Background and Objectives: Wearable devices (WDs) capable of recording electrocardiograms (ECGs) for prolonged periods in ambulatory settings offer the possibility of detecting non-predictable events such as epileptic seizures and atrial fibrillation. Nevertheless, these systems suffer from additional noise sources, such as movement artifacts (MA).
Several adaptive (AF) algorithms have been proposed in the literature to suppress movement artifacts from ECG without consistent results. Adaptive algorithms for signal enhancement require a reference signal correlated with the noise and not correlated with the sign of interest. This correlation can change significantly depending on the absolute and relative location of the electrodes and the location of the reference sensor (i.e., accelerometer, gyroscope); objectively measuring the correlation is not a trivial problem.
For this reason, first, we used an algorithm to obtain a rough estimate of the movement artifacts from the recorded ECG to calculate the correlation between them and the available reference signals (three-axis accelerometer and three-axis gyroscope) and selected the one with the highest correlation. Then, we compare three adaptive filter algorithms using the signal-to-noise ratio (SNR) coefficient as the evaluation parameter.
Methods: For testing the implemented adaptive filters, first, we used a simulated signal, then data from an open-online database, and last, a single lead ECG wearable device called AFi® (Praxa Sense™, The Netherlands) with an embedded IMU. To induce the movement artifacts in a controlled setting, participants performed a set of predefined movements within three intensities; high (running, jumping), moderate (torso rotations, pushups), and low (walking). Then we analyzed the recorded data offline as follows:
- Test the correlation between noise and the IMU components, and select the component with the highest correlation to be used as a reference input for the adaptive filters.
- Compare three adaptive filters in terms of SNR improvement; the Least means squares (LMS), the Normalized least means squares (NLMS), and the Recursive least squares RLS.
- Filter the selected reference input with wavelet decomposition, and test if there is a filter performance improvement in SNR.
Results: The implemented adaptive filters performed as expected with the simulated signals, but they showed very poor results once we used them on real data.
The RLS filter showed superior performance than the least mean squares-based filters in terms of convergence speed and the root mean squared error minimization. Nevertheless, it requires a high correlation (ρ) above ρ>0.8 between the reference input and the undesired signal or noise to provide a proper signal enhancement and morphology recovery.
The low correlation between the movement artifacts and the components of the IMU used as a reference input for the adaptive filters affected the filter performance heavily. Filtering the reference input with the wavelet decomposition did not improve the correlation or the filter performance.
Conclusions The correlation between ECG motion artifacts and movement recorded with inertial sensors appeared to be low and inconsistent. Given this, adaptive filters using inertial sensors as reference input are unsuitable for removing ECG movement artifacts.
MSc ME Thesis presentation
- Monday, 10 October 2022
- 09:30-10:31
- LB 1.150 Lipkenszaal
Predicting noise attenuation level in the earplugs using Gaussian Process Regression
Stephy Annie Curie Rakesh Arya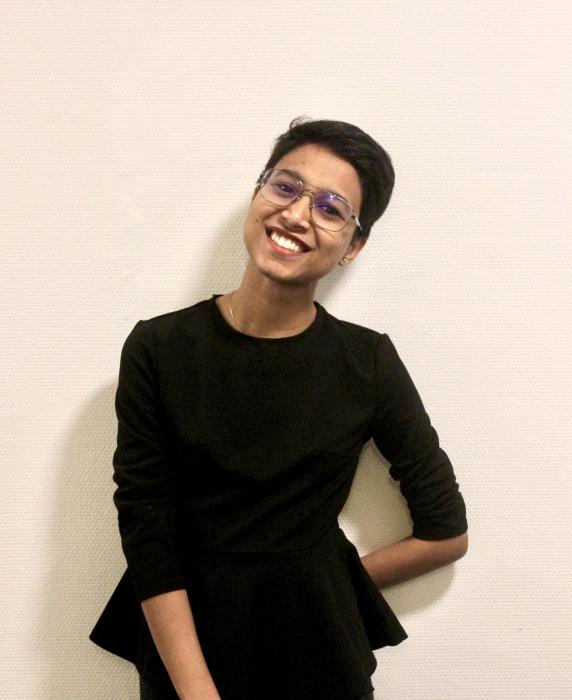
The earplug development followed by ALPINE, a hearing protection company is a trial and error method. This method leads to high material wastage. It is also a time-consuming and expensive development process. So, the objective of this thesis is to ease the error earplug development process by implementing a prediction model. The research was steered towards understanding the factors that influence the sound dampening properties in an earplug, building the dataset, and analysing the data and regression models. The regression model must be able to predict the noise attenuation provided by an earplug based on the material and design specifications for which Gaussian Process Regression (GPR) model was used. With RBF kernel function and through hyperparameter optimization, the GPR model was trained and tested on datasets.
The main finding of this thesis is that noise attenuation provided by an earplug is highly subjective. Sound perception is crucial in earplug design. Even though the most ideal sound attenuation prediction model for earplugs can be developed, in the end, everything relies on individual sound perception. So, the prediction of noise attenuation in earplugs has a higher level of uncertainty. However, with accurate age, gender, and ethnicity information, the earplugs can be modelled and designed for each group and the sound attenuation prediction model can be developed to make predictions with higher certainty.
MSc ME Thesis presentation
- Friday, 30 September 2022
- 10:00-11:00
- Timmanzaal (LB01.170)
Detecting Medical Equipment in the Catheterization Laboratory using Computer Vision
Renjie Dai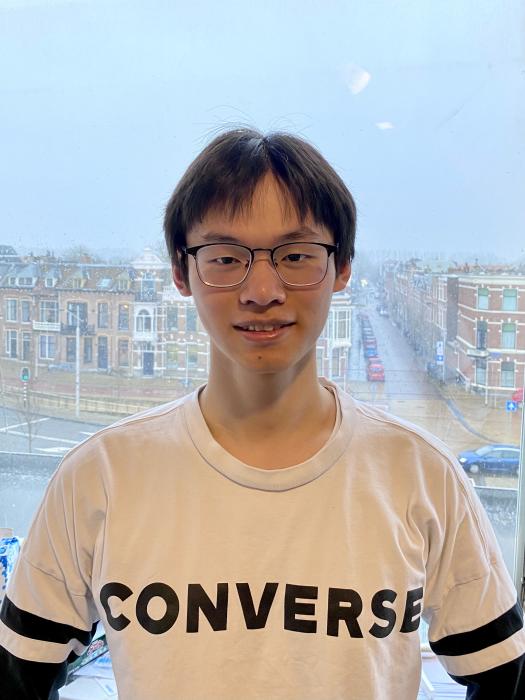
Workflow analysis aims to improve the efficiency and safety in operating rooms by analyzing surgical processes and providing feedback or support, where observations can be made and evaluated by algorithms rather than human experts. For our study, we mount five calibrated cameras from different angles in a Catheterization Laboratory (Cath Lab) to observe and analyze Cardiac Angiogram procedures. To automate the classification of workflow and personnel activities, we propose an object detection algorithm based on Scaled-YOLOv4 with a filter to improve bounding box prediction. Scaled-YOLOv4, as a state-of-the-art technique, is featured with extremely fast processing speed and decent precision. However, we find that Scaled-YOLOv4 still suffers when detecting objects with flexible appearance due to fixed anchors. This can result in the prediction of bounding boxes missing parts of the object or containing a blank environment. In this work, we design a filter following Scaled-YOLOv4 to improve the prediction of the bounding box by matching the features detected from different cameras. With the keypoints detected by SuperPoint and matched by SuperGLue, the filter adjusts the boundaries of the bounding box to include all the matched keypoints. The proposed algorithm achieves 95.1% mAP in detecting medical equipment in the Catheterization Laboratory and a real-time speed of 58 FPS on RTX 3090.
MSc ME Thesis presentation
- Tuesday, 27 September 2022
- 10:00-11:00
- LB01.190
Reconstruction and Rendering of Buildings as Radiance Fields for View Synthesis
Enpu Chen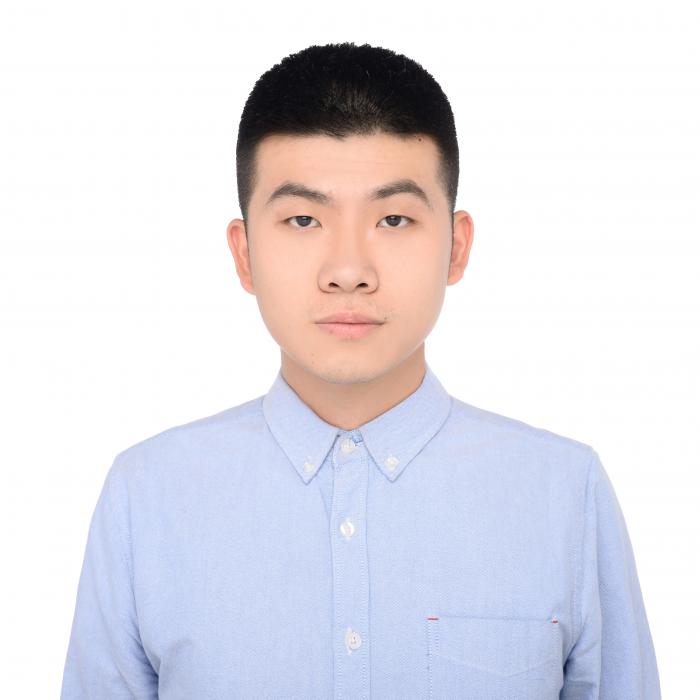
In inspection and display scenarios, reconstructing and rendering the entire surface of a building is a critical step in presenting the overall condition of the building. In building reconstruction, most works are based on point clouds because of their enhanced availability. In recent years, neural radiance fields (NeRF) have become a common function for implementing novel view synthesis. Compared to other traditional 3D graphic methods, NeRF-based models have a solid ability to produce photorealistic images with rich details that point clouds based methods cannot offer. As a result, we decided to investigate the performance of this technique in architectural scenes and look for ways to improve it for more significant scenes.
This thesis explores the ability to reconstruct large-field scenes with NeRF-based models. NeRF introduced a fully-connected network to predict the volume density and view-dependent emitted radiance at the special location, which will be projected into an image through classic volume rendering techniques. Due to the limitation of near-field ambiguity and parameterization of unbounded scenes, the original NeRF does not perform well on 360° input view, especially when the inputs are sparse. An inverted sphere parameterization that facilitates free view synthesis is introduced to address this limitation so that the foreground and background views can be trained separately. Besides that, we also compare the performance of tracing different light geometries, ray and cone, respectively. Meanwhile, to generate the reconstructed scene precisely, raw RGB images should be pre-processed to estimate the corresponding camera parameters. Finally, customized camera paths should be prepared to generate the final rendered video.
According to our experiments, training foreground and background separately is a promising method to solve practical large-scale scene reconstruction problems. A complete wrap-around view of the target building can be obtained using adjusted camera path parameters. Furthermore, introducing conical frustum casting into the original model also provides an alternative method to implement reconstruction. We named this method mip-NeRF++, which can contribute to the final results to some extent.
MSc ME Thesis presentation
- Monday, 26 September 2022
- 09:00-11:00
- Online via Teams
Automatic Camera Extrinsics Estimation in the Catherization Laboratory
Jinchen ZengSurgical workflow analysis has gained more importance in operating rooms, which could take responsibility for the working condition, the safety of both patients and surgical personnel, as well as the working efficiency. Focusing on the optimization of the workflow, a set of cameras is installed in the Catherization Laboratory in Reinier de Graaf Gasthuis for multiple computer vision related researches. However, our cameras are calibrated only once after installation. The orientation and position of the cameras could be changed after days or months, which could lead to a wrong localization.
Compared with the traditional calibration method (calibration patterns or markers), we propose a new image-based camera pose estimation pipeline tested in the Catheterization Laboratory. Our proposed pipeline exploits object detection model (Scaled-YOLOv4) to detect fixed objects. The mean average precision with 50% IoU threshold (mAP@.5) achieves more than 0.99 for all detected objects. Then use a self-supervised interest point detector and descriptor (SuperPoint). With the detected feature points, a feature matching technique based on graph neural networks (SuperGlue) is adopted to match the interest points detected in the target image with reference points annotated in the image databases (image DBs). The point-correspondences between the image coordinates and the 3D coordinates are applied to solve Perspective-n-Point (PnP) problem to compute the orientation and position of each camera (Camera pose). The final camera pose estimation achieves a 5.79-pixel reprojection error with a 4.97-cm Euclidean distance error. Compared with other image-based camera pose estimation techniques, our pipeline requires no 3D reconstruction or 3D point cloud in the scene model. Using the video from real procedures, we show that the pipeline is able to estimate the camera pose with high accuracy.
Microelectronics Colloquium
- Thursday, 22 September 2022
- 15:30-16:30
- TBD
Sparsity-constrained Linear Dynamical Systems
Geethu Joseph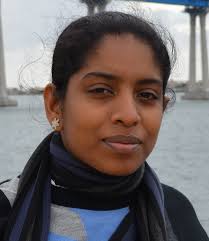
Abstract: At the intersection of control engineering and signal processing sits the upcoming field of sparse control and state estimation of linear dynamical systems. It deals with linear dynamical systems with control inputs having a few nonzero entries compared to their dimensions. Constraining the inputs to be sparse is often necessary to select a small subset of the available sensors or actuators at each time instant due to energy, bandwidth, or physical network constraints. Bringing together research from classical control theory and compressed sensing, the talk presents a comprehensive overview and critical insights into the conceptual foundations of sparsity-constrained systems, including the formulation, theory, and algorithms. We look at the concrete example of a budget-constrained external agent controlling the opinion of a social network.
Additional information ...
MSc ME Thesis presentation
- Thursday, 22 September 2022
- 13:30-16:00
- van Katwijkzaal (HB08.150)
Frequency Domain Joint Estimation of HRF and Stimulus from fUS Data
Yitong Tao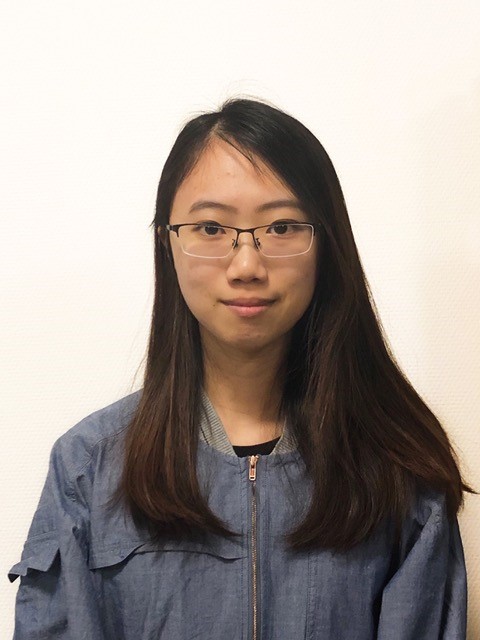
To better understand how brain signals are processed and even how the human mind works, analyzing the hemodynamic signal model is one of the most essential steps. In the CUBE group of Erasmus MC, functional ultrasound (fUS) data of a mouse’s brain is recorded. By using this fUS dataset, this thesis will solve the problem regarding the joint estimation of hemodynamic response function (HRF) and the underlying stimulus. Usually, hemodynamic responses are investigated in the time domain, while this thesis provides another perspective
from frequency domain signal processing.
We consider the hemodynamic response as a convolutive signal mixture, then try to transform it into an instantaneous mixing model by converting the context into the frequency domain. By applying independent vector analysis (IVA), this estimation problem can be solved without facing permutation ambiguity which is a well-unknown problem regarding independent component analysis (ICA). Additional steps before and after IVA are also discussed so that a whole estimation road map is formed.
Both simulation and experimental analysis are provided to validate this estimation algorithm. Results show that by using this method, both stimulus and HRF estimation can be achieved satisfyingly in a suitable experimental setting. This thesis provides insights and future potentials for IVA to be further investigated in neural signal processing problems.
Additional information ...
PhD Thesis Defence
- Thursday, 15 September 2022
- 12:00-13:30
- Aula Senaatszaal
Advanced Measurement Techniques and Circuits for Array-Based Transit-Time Ultrasonic Flow Meters
Douwe van Willigen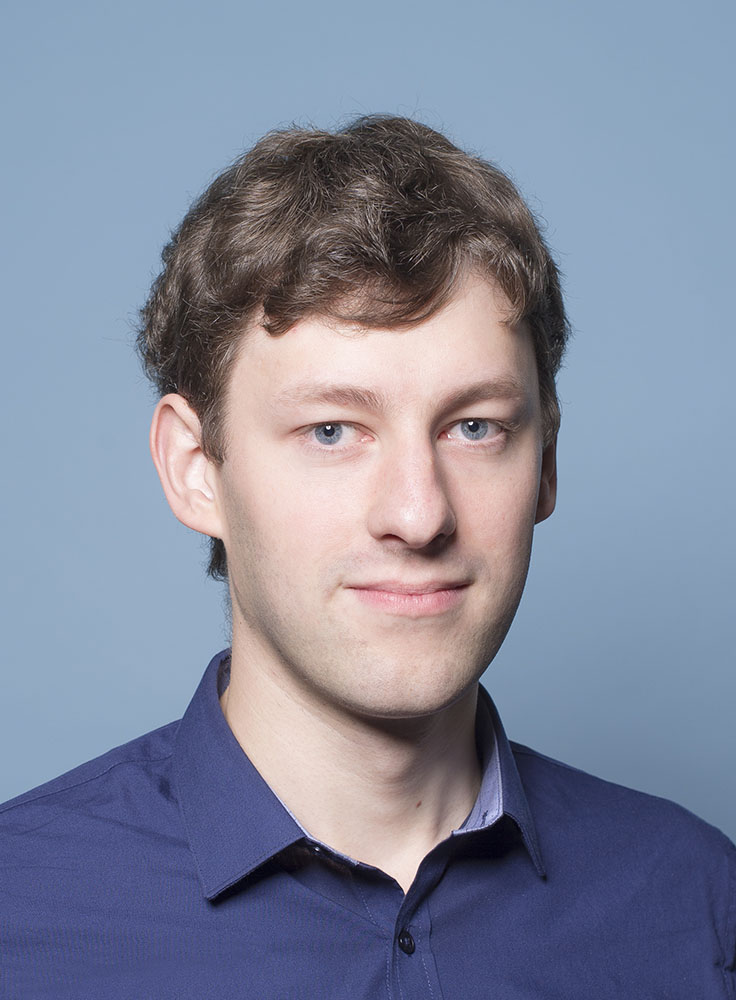
This thesis describes the design, prototyping and evaluation of matrix-based clamp-on ultrasonic flow meters. Several new measurement techniques are presented as well as an Application-Specific Integrated Circuit (ASIC) designed for accurate measurement of flow velocity with matrix transducers.
The influence of circuit topologies on the zero-flow performance of ultrasonic flow meters has been analyzed and an algorithm is presented to reduce the offset. With a linear transducer array, flow measurements have been performed via two different acoustic paths, demonstrating the ability to accurately measure flow with array transducers through a stainless-steel pipe wall. In order to improve signal quality, an ASIC has been designed that is able to drive and read-out 96 piezo transducer elements. The ASIC has been characterized electrically and flow measurements have been performed in combination with the linear transducer arrays.
Several new techniques, enabled using transducer arrays, have also been explored. By tapering the amplitude of the transmit signals, spurious waves can be suppressed. An auto-calibration technique has been developed that uses additional acoustic measurements to estimate the diameter of the pipe and the speed of sound in the pipe wall and liquid. Finally, a simulation study has been performed to explore the possibility of exploiting the beam-steering capabilities of transducer arrays to measure flow velocity profiles by using measurements obtained via multiple acoustic paths.
Thesis:
https://doi.org/10.4233/uuid:e2f4b411-3d8e-4b93-b037-096009c59f61
Collegerama (live stream of the defence):
https://collegerama.tudelft.nl/mediasite/play/571eb84f3e724d47b46df7e8d0eb3a7a1d
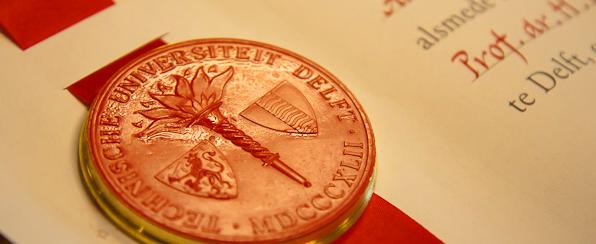
PhD Thesis Defence
- Tuesday, 13 September 2022
- 12:00-13:30
- Aula Senaatszaal
Integrated Transceiver Circuits for Catheter-based Ultrasound Probes and Wearable Ultrasound Patches
Mingliang Tan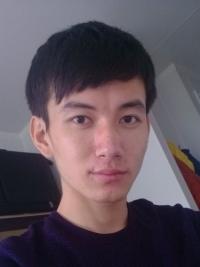
Promotors: Michiel Pertijs and Ronald Dekker
Thesis: link
Collegerama link (live stream of the defence): link
Abstract: This thesis describes the design, prototyping, and experimental evaluation of transceiver ASICs (application-specific integrated circuits) for catheter-based ultrasound probes and wearable ultrasound patches. Various circuit techniques are proposed to address requirements and implementation bottlenecks in these applications. Prototype chips are presented to demonstrate the effectiveness of these techniques. To reduce the loading effect of micro-coaxial cables in an ICE probe based on capacitive micro-machined ultrasound transducers (CMUTs), an ASIC prototype including element-level high-voltage pulses and low-noise trans-impedance amplifiers has been implemented. Besides reducing the loading effect from micro-coaxial cables, ASICs play an important role in achieving cable-count reduction, which is crucial for 3-D imaging catheters, such as forward-looking IVUS probes. Circuit techniques are proposed to implement a prototype ASIC which only requires 4 cables to interface with a 2D piezoelectric transducer array. Additionally, to address the challenges in interface electronics for wearable ultrasound patches, a prototype ASIC is presented that contains 64 reconfigurable transceiver channels that can interface with different transducer elements by employing channel-parallelizing techniques.
Additional information ...
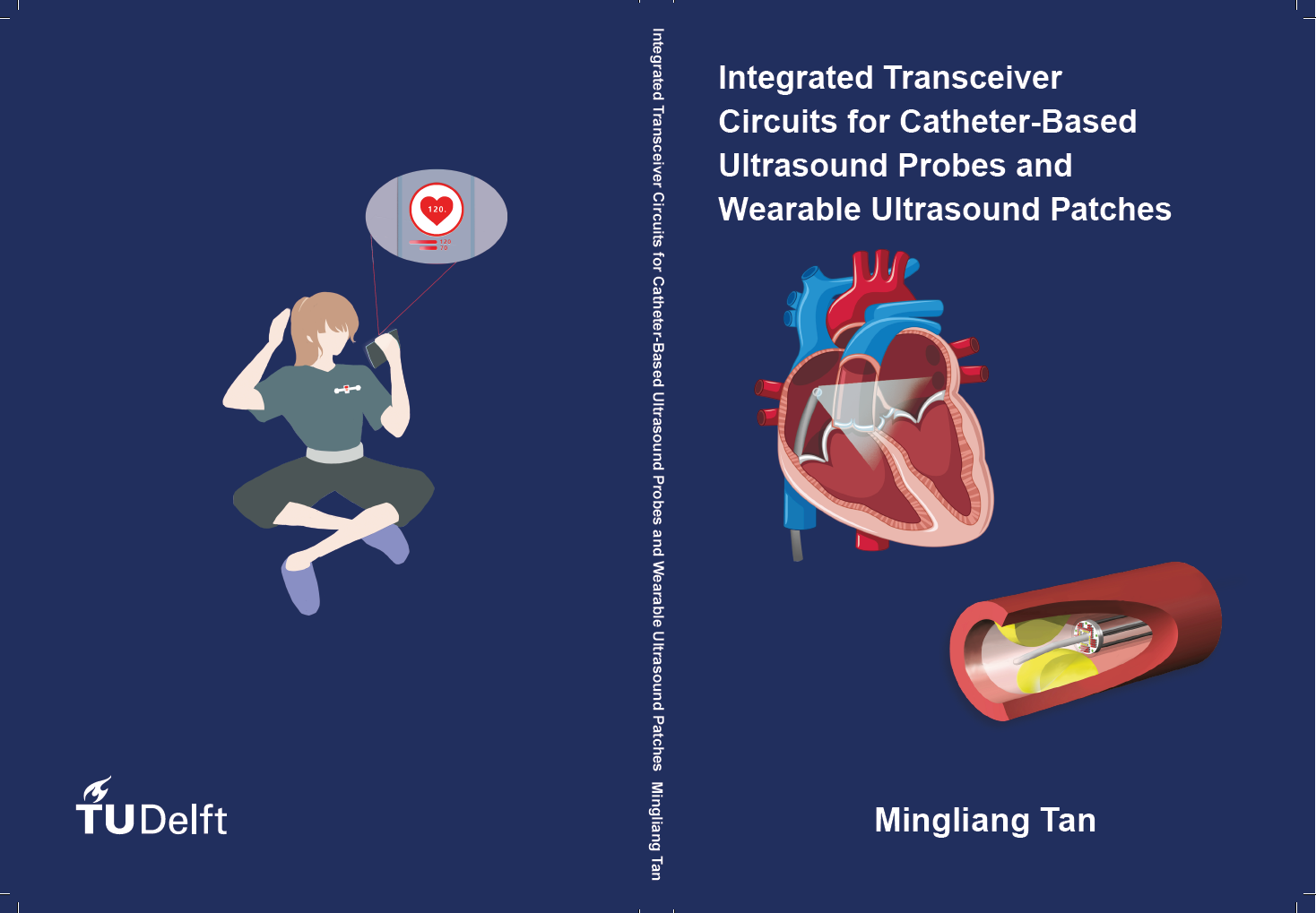
Special EI Colloquium
- Tuesday, 13 September 2022
- 10:00-11:00
- EWI - Lecture Hall F
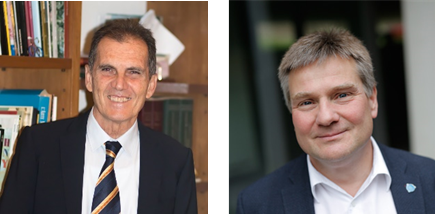
Real-time High-Frame Rate imaging: Novel Methods and Applications
Prof. Piero Tortoli
Microelectronics Systems Design Laboratory
University of Florence, Italy
Medical imaging is increasingly based on High-Frame-Rate (HFR) methods, which are in principle capable of producing one frame (or even one data volume) per transmission event. However, achieving such a goal in real-time implicitly involves the transfer and processing of huge amount of data at high rates, and this can be done only through an appropriate experimental setup.
In this talk, the main characteristics of the hardware-based open scanner ULA-OP 256 are briefly reviewed, and its recent advancements, such as the data transfer acceleration obtained through an architectural change, and the possible expansion toward the control of an unlimited number of probe elements, are reported in detail. The “virtual real-time” modality will also be described as ideal to obtain the best performance from specific HFR imaging modalities. Finally, the combination of ULA-OP 256 with properly designed sparse 2-D arrays will be shown suitable for the investigation of full volumes. The talk will be concluded with the presentation of experimental results in a few sample applications, including multi-plane imaging, HFR CFM and HFR vector Doppler.
Micro- and Nanosystems at ESAT, KU Leuven
Prof. Michael Kraft
ESAT, Micro- and Nano-Systems
KU Leuven, Belgium
This seminar will give an brief overview of the activities in micro- and nanosystems at the Electrical Engineering Department (ESAT) of KU Leuven. It will describe the available infrastructure and give a short overview of current research activities in the division Micro- and Nanosystems (MNS), which currently comprises 24 PhD students, 4 postdoctoral researchers and 2 technicians.
A selection of current active projects and recent highlights will be presented, including work on:
- Coupled resonators for mass sensing applications
- Piezoelectric ultrasound technology arrays for medical imaging and underwater communication
- Micromachined probes for neuro recording and stimulation
- Multi-parameter sensing chip for bioreactor condition monitoring
- Genetic Algorithm for the design of MEMS devices (accelerometers and microgrippers)
Finally, the newly founded Leuven Institute for Micro- and Nano Integration (LIMNI) will be briefly introduced.
Note: This Colloquium precedes the PhD defence of Mingliang Tan, which will take place in the Aula on the same day at 12:00 (layman’s talk), 12:30-13:30 (defence). More information can be found here.
MSc SS Thesis Presentation
- Monday, 29 August 2022
- 09:30-10:15
- Lecture Hall F
Improving the Estimation of Epicardial Activation Times Using Spatial Information
William Hunter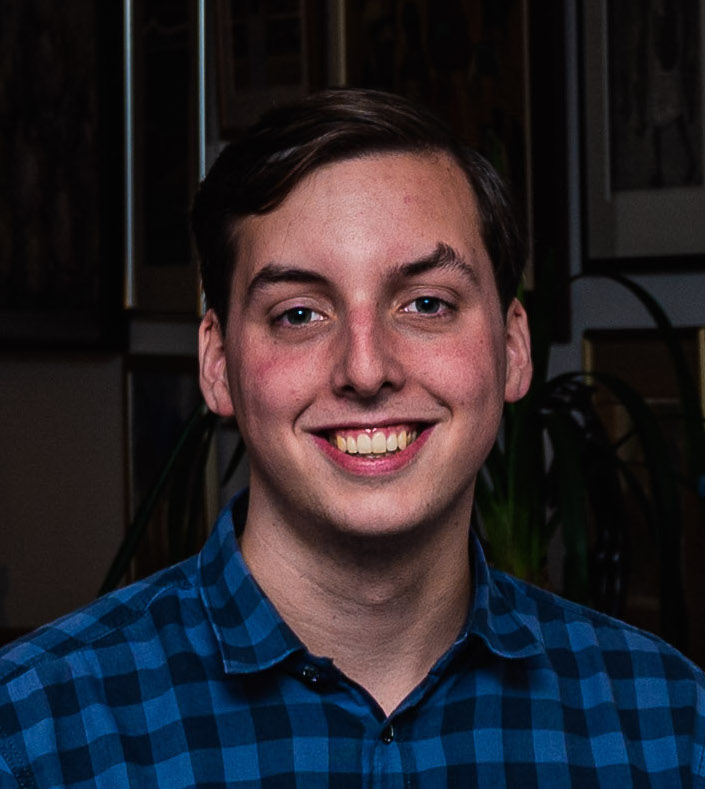
Atrial fibrillation is a common cardiovascular disease, affecting the regular beating of the heart through chaotic contraction of the heart's upper chambers. On its own, the condition—increasingly prevalent among the elderly—is not life threatening, but it leads to an increased risk of stroke and heart failure. As of yet, there is no consensus on the physiological mechanisms responsible for initiating and sustaining atrial fibrillation. A more detailed view of cardiac activity would improve understanding of the disease, making earlier diagnosis possible and improving options for treatment.
The contraction of the cardiac muscles is governed by electrical signals propagating through the tissue. Cardiac activity can be monitored with a high spatial resolution by measuring the electrical potential directly on the epicardium of the heart during open-heart surgery, using an array of closely spaced electrodes. From these electrograms, estimating the time of local activation of the cardiac tissue underneath each electrode provides a quantitative way of mapping the mechanisms of atrial fibrillation. Various methods exist to estimate the activation times, but the complex signals that are typical of atrial fibrillation make it difficult to obtain accurate results. This thesis proposes combining two existing methods for estimating the local activation times. Based on a model of the electrogram as a spatial convolution of local transmembrane currents, an inverse problem is formulated and solved, resulting in a less opaque view of the cardiac activity at the electrode locations by attenuating distant disturbances and emphasizing local activity. The deconvolution output is fed to the second step, where cross-correlating certain pairs of signals gives an estimate for the mutual time delay in local activation. A graph representation of the electrode array is used to define neighbor order and decide which signal pairs are correlated. The set of pairwise time delays this produces is then converted to an estimate for the local activation times, using a least-squares estimator.
The proposed method is evaluated using different simulated cardiac settings. In a setting with one stimulation source, earlier results of the deconvolution and cross-correlation methods are confirmed, and the proposed method is seen to produce a slightly lower mean error than reference methods. In the higher-complexity triple-source setting, the latter effect is again visible. Reinforced by the performance of the different methods in increasingly noisy settings, the main merits of the proposed method for the estimation of local activation times can be said to be found in the form of increased consistency, not significantly improving on the accuracy of existing methods.
Additional information ...
MSc SS Thesis Presentation
- Friday, 26 August 2022
- 10:00-10:45
- Van Katwijkzaal (HB 08.150)
Cooperative Localization of Unmanned Aerial Vehicles using ADS-B
Xuzhou YANG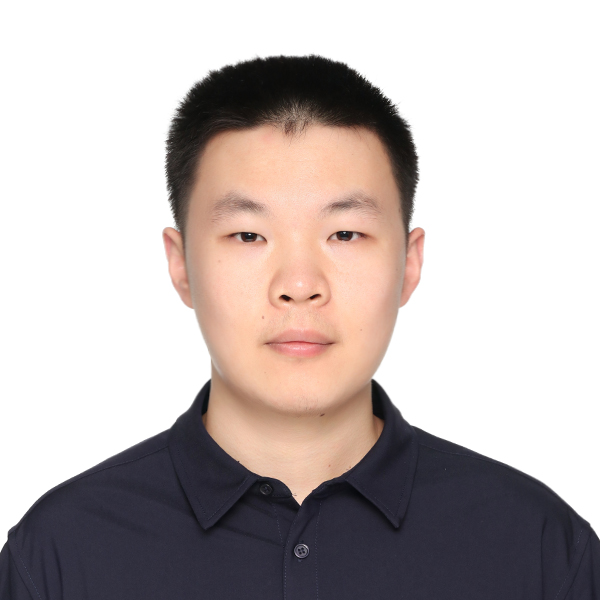
As unmanned aerial systems (UAS) turn into a full-fledged industry, the sky will be much more crowded in the future. Large-scale UAV applications make reliable UAV navigation a pressing need. Traditionally, global navigation satellite system (GNSS) is extensively used as the primary positioning, navigation, and timing (PNT) service. However, GNSS is vulnerable to intentional radio interference such as spoofing, jamming, and repeating. Hence, alternative PNT (APNT) attracted many researchers' attention.
In this thesis, instead of GNSS signals, ADS-B signals from piloted aircraft are leveraged for UAV navigation. We propose a cooperative navigation strategy for multiple UAVs in GNSS-denied environments. It consists of: 1) a system-level, leader-follower cooperative strategy; 2) a sensor fusion algorithm for individual UAV navigation based on the extended Kalman filter. Furthermore, the effects of asynchronous clocks are studied and a joint relative positioning and synchronization algorithm is applied to tackle this problem.
Finally, Monte Carlo experiments in a multi-UAV scene are performed to verify the proposed algorithms. The results show that the proposed algorithms achieve a performance comparable to civilian GNSS on the selected data set and under the system assumptions we made. Moreover, the proposed cooperative navigation framework only needs one ground station of limited service capacity as external aid. Compared with large-scale, specialized terrestrial APNT service networks, our proposed framework is more flexible and the system can be deployed in areas without infrastructure.
Additional information ...
MSc SS Thesis Presentation
- Thursday, 25 August 2022
- 13:00-13:45
- Room G
Robust Formation Control against Observation Losses
Zhonggang Li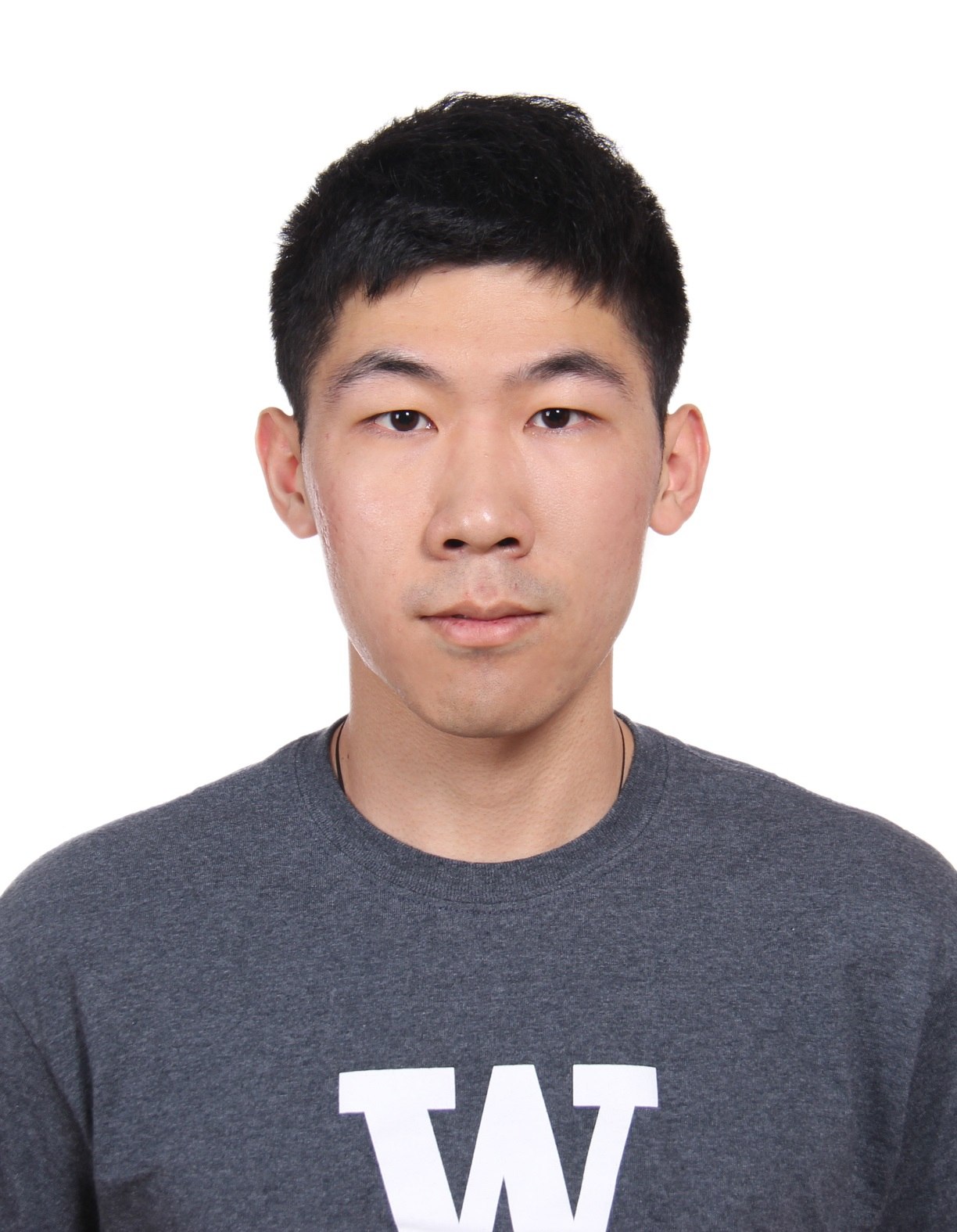
Distributed formation control has received increasing attention in multiagent systems. Maintaining certain geometry in space is advantageous in many applications such as space interferometry and underwater sensing. At present, there is a variety of distributed solutions for agents to converge to desired formations and track a series of prescribed maneuvers. They typically rely on the relative kinematics e.g., relative positions of the neighboring agents as state observations for the local controller. In harsh working environments, the acquisition of the relative kinematics is challenged and observation losses might occur, which can be detrimental to the optimality of formation.
In this work, observation losses in noisy environments are addressed under a distributed formation control framework. Three types of solutions are proposed to enhance the robustness which is evaluated through the improvements of tracking error, convergence speed, and smoothness of trajectories in both random and permanent loss settings. Firstly, a relative localization technique is proposed using formation itself as a spatial constraint. Secondly, a dynamic model is established for the agents entailed by a Kalman filter-based solution. Finally, a fusion of the previous two types is inspired and it exhibits superior performance than both aforementioned types individually.
This work not only provides means of relative localization without additional sensor data but also shares insights into coping with random or permanent graph changes for stress-based formation control systems. This could potentially lead to the exploration of formation control with subgraphs or energy-efficient sensing as future directions.
Additional information ...
MSc SS Thesis Presentation
- Thursday, 25 August 2022
- 10:00-10:45
- LB01.040
Distributed Gaussian Process for Multi-agent Systems
Peiyuan Zhai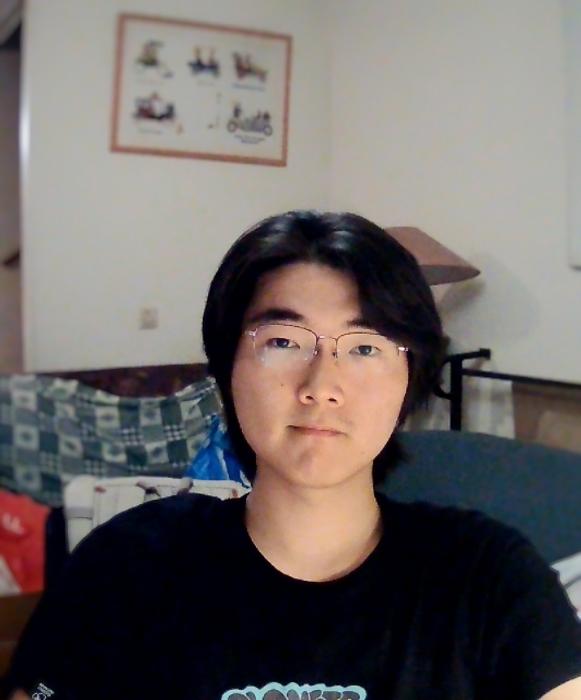
This work is focused on environmental monitoring and learning of unknown field by Gaussian Process (GP) in Multi-agent Systems (MAS). The two main problems are how to develop fully-distributed and robust algorithm to (1). optimize GP hyperparameters, and (2). aggregate GP predictions from agents.
The state-of-the-art distributed GP hyperparameter optimization algorithm is proximal alternated direction method of multipliers (pxADMM), which requires a center station in MAS. Based on pxADMM, two fully-distributed algorithms are proposed so that the center station is no longer needed. Asynchronous behavior is also introduced into the proposed algorithms to deal with heterogeneous processing time of agents.
Current aggregation methods are classified based on whether datasets are independent. Under independence assumption, PoE and BCM families of methods are distributed by applying discrete time consensus filter (DTCF), which is proposed to be replaced by primal-dual method of multiplier (PDMM) for faster convergence. Without independence assumption, the Nested Pointwise Aggregation of Experts (NPAE) can be distributed by NPAE-JOR in complete graph with high flooding overhead. We propose fully-distributed CON-NPAE in connected graph to eliminate flooding overhead.
Simulation results show that the proposed hyperparameter optimization algorithms are fully-distributed at a cost of 2 to 4.5 times more iterations compared to pxADMM. The fully-dsitributed PoE and BCM based methods are accelerated, and the fully-distributed CON-NPAE makes comparable aggregations as NPAE without flooding overhead. Future work will be focused on the theoretical convergence analysis of fully-distributed pxADMM, the effect of network structure on CON-NPAE and new type of distributed NPAE based on inducing points.
Additional information ...
MSc SS Thesis Presentation
- Wednesday, 24 August 2022
- 12:00-12:45
- Lecture Hall Ampere
Detect and Avoid for Autonomous Agents in Cluttered Environments
Mosab DiabAutonomous agents are the future of many services and industries such as delivery systems, surveillance and monitoring, and search and rescue missions. An important aspect in an autonomous agent is the navigation system it uses to traverse the environment. Not much emphasis has been paid in the past on autonomous agent navigation in cluttered environments. Cluttered and unknown environments such as forests and subaquatic environments need to have autonomous navigation systems developed just for them due to their uncertain and changing nature.
Path planning algorithms are used for the navigation of an autonomous agent in an environment. The agent needs to reach a target location while avoiding the obstacles it detects along the path. Such a system is called a Detect and Avoid (DAA) system and there are different implementations for it of which some are explored in this thesis.
The Artificial Potential Fields method or APF for short is a method for mobile agent navigation which is based on generating an attractive force on the agent from the target and a repulsive force from the obstacles. This leads to the agent reaching the target while avoiding the obstacles along the way. The Classical APF (CAPF) method works for structured environments well but not for cluttered environments. The CAPF method can be replaced with a modified version where the agent is surrounded by a set of points (called bacteria points) around its current location and the agent moves by selecting a bacteria point as a future location. This method is named the Bacteria APF (BAPF) method. This selection happens through combinatorial optimization based on the potential value of each bacteria point.
In this thesis, we propose two distinct contributions to the BAPF method. The first one being the use of an adaptive parameter in the repulsive cost function which is determined through a brute-force search. The second addition is a branching cost function that changes the value of the repulsive potential based on predefined perimeters around each obstacle. We show through simulations on densely and lightly cluttered environments that this Improved BAPF (IBAPF) method significantly improves the performance of the system in terms of the convergence to the target by almost 200% and reduced the time it takes to converge by around 25% as well as maintain the safety of the navigation route by keeping the average distance from obstacles around the same value.
Additional information ...
MSc SS Thesis Presentation
- Monday, 22 August 2022
- 14:00-15:00
- EEMCS Lecture room F
Search by Image: Deep Learning Based Image Visual Feature Extraction
Yanan Hu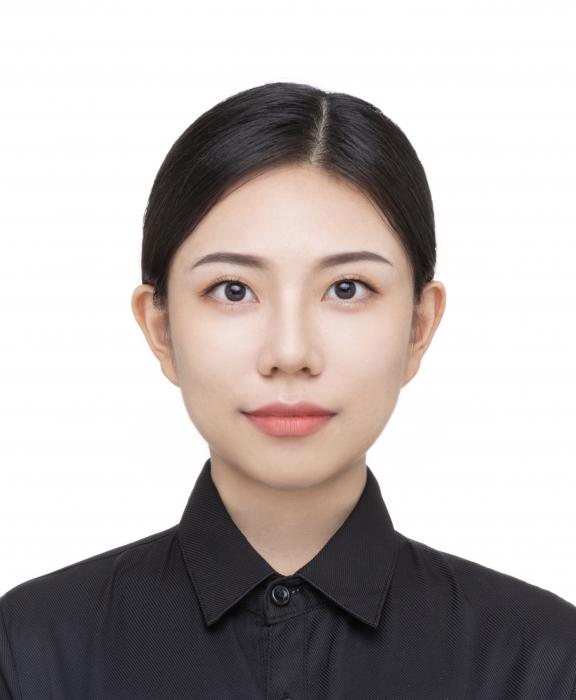
In recent years, the expansion of the Internet has brought an explosion of visual information, including social media, medical photographs, and digital history. This massive amount of visual content generation and sharing presents new challenges, especially when searching for similar information in databases —— Content-Based Image Retrieval (CBIR). Feature extraction is the foundation of image retrieval, making research into obtaining concrete features and representations of image content a vital concern. In the feature extraction module, We first pre-process the target image and input it into a CNN to obtain feature maps for different channels. These feature maps can be aggregated into compact and global uniform descriptors by pooling. Then these global descriptors are further dimensionalised and normalized by whitening methods to obtain image feature vectors that are easy to compute and compare. In this process, the accuracy of the retrieval depends on how accurately the final feature vectors represent the meaning expressed by the target image. Therefore, various CNN network structures, pooling and whitening methods are proposed to get more concrete feature vectors.
In this thesis, our study (1) fine tunes the pre-trained CNNs, (2) optimizes the application of second-order attention information in feature map, (3) applies and compares popular feature enhancement methods in both aggregating and whitening, (4) explores how to combine all strengths, and (5) propose a new model \textit{ResNet-SOI}, which achieves 53.4(M) and 59.2(M) mAP on the challenging benchmark \textit{ROxford5k+1M} and\textit{ RParis6k+1M}, and outperforms the state-of-art methods.
MSc SS Thesis Presentation
- Friday, 22 July 2022
- 10:00-10:45
- LB 01.010 Snijderszaal; Teams
Image-Based Query Search Engine via Deep Learning
Yuanyuan YaoTypically, people search images by text: users enter keywords and a search engine returns relevant results. However, this pattern has limitations. An obvious drawback is that when searching in one language, users may miss results labelled in other languages. Moreover, sometimes people know little about the object in the image and thus would not know what keywords could be used to search for more information. Driven by this use case with many applications, content-based image retrieval (CBIR) has recently been put under the spotlight, which aims to retrieve similar images in the database solely by the content of the query image without relying on textual information.
To achieve this objective, an essential part is that the search engine should be able to interpret images at a higher level instead of treating them simply as arrays of pixel values. In practice, this is done by extracting distinguishable features. Many effective algorithms have been proposed, from traditional handcrafted features to more recent deep learning methods. Good features may lead to good retrieval performance, but the problem is still not fully solved. To make the engine useful in real-world applications, retrieval efficiency is also an important factor to consider while has not received as much attention as feature extraction.
In this work, we focus on retrieval efficiency and provide a solution for real-time CBIR in million-scale databases. The feature vectors of database images are extracted and stored offline. During the online procedure, such feature vectors of query images are also extracted and then compared with database vectors, finding the nearest neighbours and returning the corresponding images as results. Since feature extraction only performs once for each query, the main limiting factor of retrieval efficiency in large-scale database is the time of finding nearest neighbours. Exact search has been shown to be far from adequate, and thus approximate nearest neighbour (ANN) search methods have been proposed, which mainly fall into two categories: compression-based and tree/graph-based. However, these two types of approaches are usually not discussed and compared together. Also, the possibility of combining them has not been fully studied. Our study (1) applies and compares methods in both categories, (2) reveals the gap betweentoy examples and real applications, and (3) explores how to get the best of both worlds. Moreover, a prototype of our image search engine with GUI is available on https://github.com/YYao-42/ImgSearch
Additional information ...
MSc SS Thesis Presentation
- Wednesday, 20 July 2022
- 13:00-13:45
- zoom
Gaussian Process enhanced Distributed Particle filtering
Rui Tang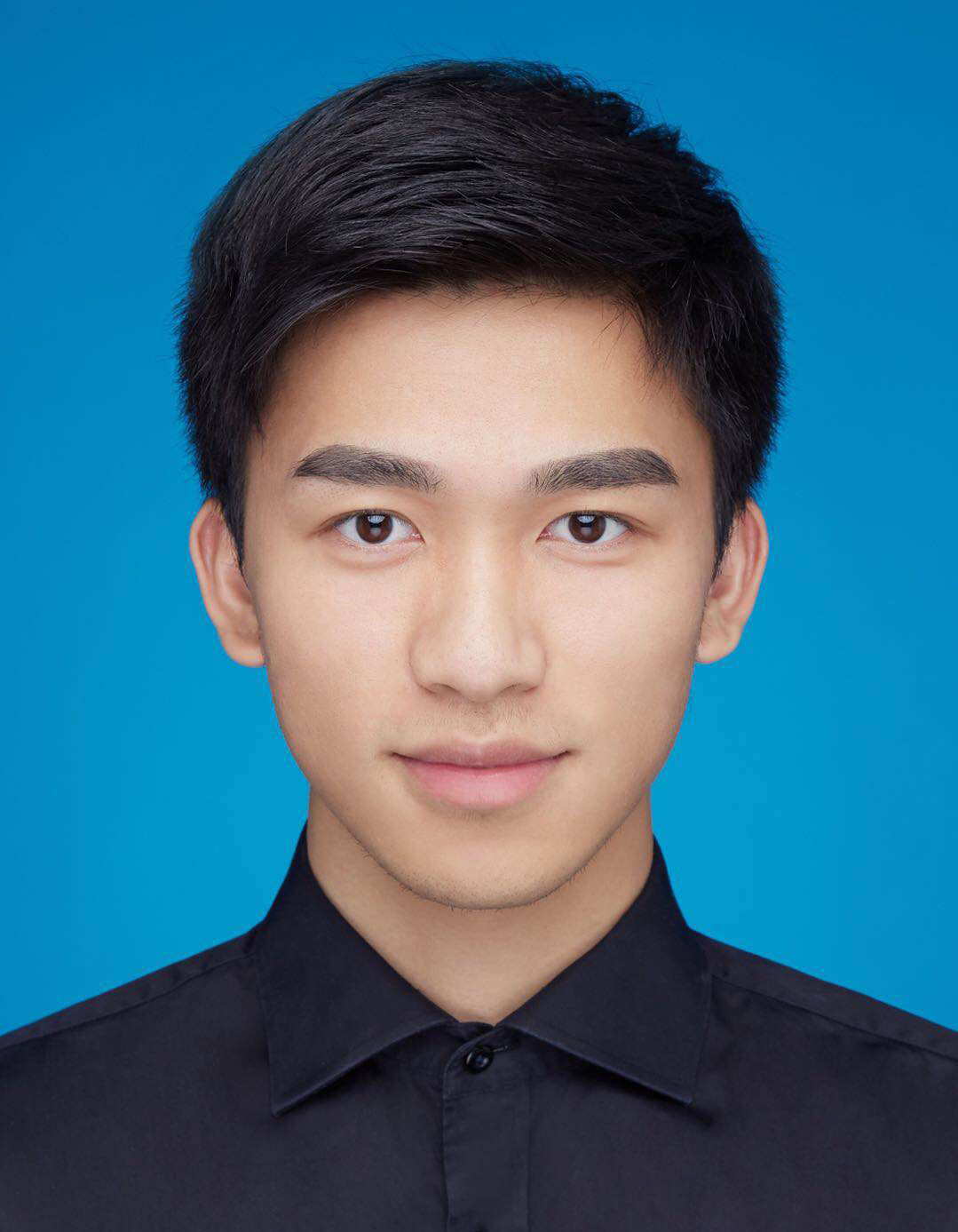
In many applications of multi-agent networks, the physical systems consist of massive nonlinear and non-Gaussian elements. Hence, in the first decade of this century, intensive research on distributed particle filters (DPFs) has been conducted to address the distributed estimation problems. For distributed algorithms, communication overhead is an important metric in terms of engineering feasibility. In previous work, the approach to distributed particle filtering relies on a parameterization of the posterior proba-bility or likelihood function, to reduce communication requirements. However, as more and more effective resampling algorithms are proposed, the dependence of particle filter performance on particle set size is greatly reduced, so this thesis attempts to explore the possi-bility of DPFs based on direct particle exchange. In this thesis, the Gaussian process enhanced resampling algorithm is used. Meanwhile, several metaheuristic optimization algorithms (i.e., genetic algorithm and firefly algorithm) are further adapted to seek the global optimal particle set to improve the estimation performance. Furthermore, all algorithms are simulated in target tracking scenarios and are evaluated from three aspects: time, space, and communication complexity.
Additional information ...
MSc SS Thesis Presentation
- Thursday, 30 June 2022
- 09:30-10:15
- EWI-Lecture Hall G
Sensor-to-Cell Height Estimation for Conductivity Estimation in Cardiac Cells
Cees Kos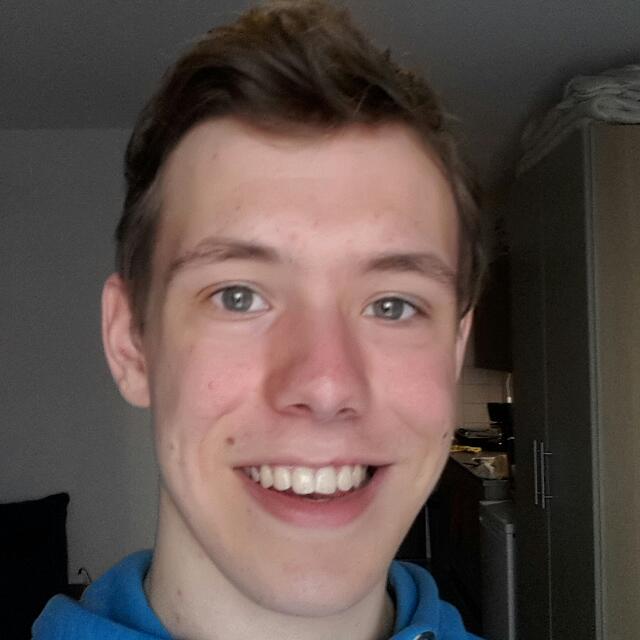
The heart is one of our vital organs. It functions by periodically contracting in a rhythmic way. Sometimes, this rhythmic behaviour is affected by abnormalities in the tissue. These conditions are referred to as cardiac arrhythmias. One kind is of specific interest, called atrial fibrillation (AF). To further study AF, methods have been developed to estimate the conductivity of cardiac cells based on the measured electrical signals. In addition, other parameters of interest besides the conductivity are involved. The goal of this project was to consider one of the parameters in the electrogram (EGM) model: the sensor-to-cell height.
First, we studied the effect of the height as a parameter in the model when used for conductivity estimation. To that end, a detector was built with which we can explain the effect of all involved parameters on the ability to accurately estimate any parameters of interest.
In addition, we considered the case where the height is unknown and is estimated, thus possibly including estimation errors. The focus was on the consequences of making errors in the estimation of the height with respect to conduction block detection and conductivity estimation.
Lastly, the effort was made to estimate the height. Here, the optimisation problem of height estimation was formalised and derived as its implementable form. At first, a simplified EGM model was assumed in order to mimic and estimate cell-specific effects, i.e. the cell conductivities. Then, the height was estimated in various situations to study its behaviour and performance under different conditions. Then, also the standard EGM model was used in the same way, after which we also tested the performance of the designed algorithm in combination with existing conductivity estimation methods.
MSc SS Thesis Presentation
- Thursday, 30 June 2022
- 10:00-10:45
- Lecture hall Data
Analyzing dynamic functional connectivity using state-space models on mice fUS data
Ruben Wijnands
In recent years, the increase in brain research led to the development of large-scale brain imaging techniques. With large-scale brain imaging techniques, such as functional magnetic resonance imaging (fMRI), functional connectivity analyses have shown altered connectivity patterns in humans and mice with neurobiological disorders, such as autism spectrum disorder (ASD). To further investigate different mutations that contribute to ASD, a behavioral neuroscientific experiment has been performed at the neuroscientific department of Erasmus MC. During the trial, brain activity in groups of wild-type and homozygous mice is measured using functional ultrasound (fUS) while allowing for spontaneous behaviors of mice. The homozygous mice lack the SHANK2 protein, resulting in hyperactivity and autistic-like behavioral alterations associated with ASD in humans. Understanding the origin of ASD is key to providing effective treatment. However, with the introduction of novel large-scale brain imaging techniques such as fUS, new methods have to be developed that enable functional connectivity analyses. Furthermore, new insights might also be acquired from dynamic functional connectivity analyses, in which changes in functional connectivity over time are evaluated. Subsequently, the question remains if it is possible to unravel differences in brain dynamics between wild-type and homozygous mice using a dynamic functional connectivity analysis.
First, an fUS data model is developed to model how fUS signals arise from a generative perspective. This model comprises a combination of a convolutive and a state-space model. Subsequently, inference of functional networks and their temporal dynamics can be performed. Also, a pre-processing pipeline for experimental fUS data is designed to reduce problem complexity and data cleaning. The performance of the developed methods is evaluated on the experimental data set, where a difference in brain dynamics between wild-type and homozygous mice is investigated.
It is found that a deconvolution procedure using the non-negative least absolute shrinkage and selection operator (NNLASSO) is necessary to reconstruct the underlying activity of neural populations. After that, using the hidden Markov model (HMM) as a state-space model, it is found that functional networks and their temporal dynamics can be learned from fUS data using expectation maximization (EM). It has been discovered that the developed methods consistently decompose reconstructed neural activity into biologically plausible functional networks from experimental fUS data. Also, with 96% certainty, a difference in brain dynamics between wild-type and homozygous mice is found using this method.
In summary, in this thesis, novel methods are developed to perform a dynamic functional connectivity analysis on experimental fUS data. Also, by performing such dynamic functional connectivity analysis for the first time on fUS data, a consistent decomposition of reconstructed neural activity into biologically plausible functional networks and a possible difference in brain dynamics between wild-type and homozygous mice are found. This research highlights the potential of fUS as a large-scale brain imaging technique in the quest to understand the origin of ASD and other neurobiological disorders.
IEEE SPS IFS-TC Webinar Series
- Wednesday, 29 June 2022
- 16:00-17:00
- zoom (need to register)
Communication Efficient Privacy-Preserving Distributed Optimization
Richard Heusdens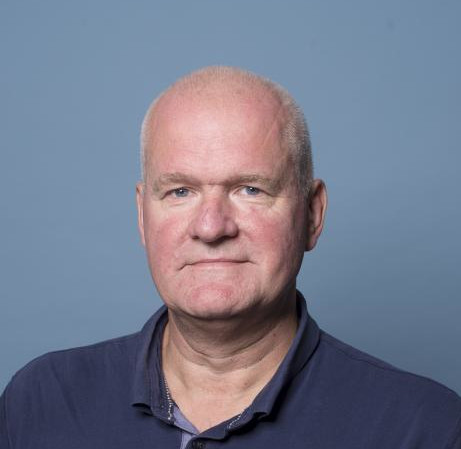
Privacy issues and communication costs are both major concerns in distributed optimization in networks. There is often a tradeoff between them because encryption methods used for privacy-preservation often introduce significant communication overhead. In this talk, we discuss a quantization-based approach to achieve both communication efficiency and privacy-preserving in the context of distributed optimization. By deploying an adaptive differential quantization scheme, we allow each node in the network to achieve the optimum solution with low communication costs while keeping its private data unrevealed. The proposed approach is general and can be applied in various distributed optimization methods, such as dual ascent and methods based on operator splitting (PDMM and ADMM). We consider two widely used adversary models, passive and eavesdropping, and investigate the properties of the proposed approach using different applications and demonstrate its superior performance compared to existing privacy-preserving approaches in terms of privacy, accuracy, and communication cost.
This webinar is organized by the IEEE Signal Processing Society Information Forensics and Security Technical Committee. See under "additional information" for a registration link.
Additional information ...
MSc SS Thesis Presentation
- Monday, 27 June 2022
- 09:30-10:15
- EWI-Lecture Hall Chip
Estimation of Atrial Fibre Directions Based on Epicardial Electrograms
Jordi de Vries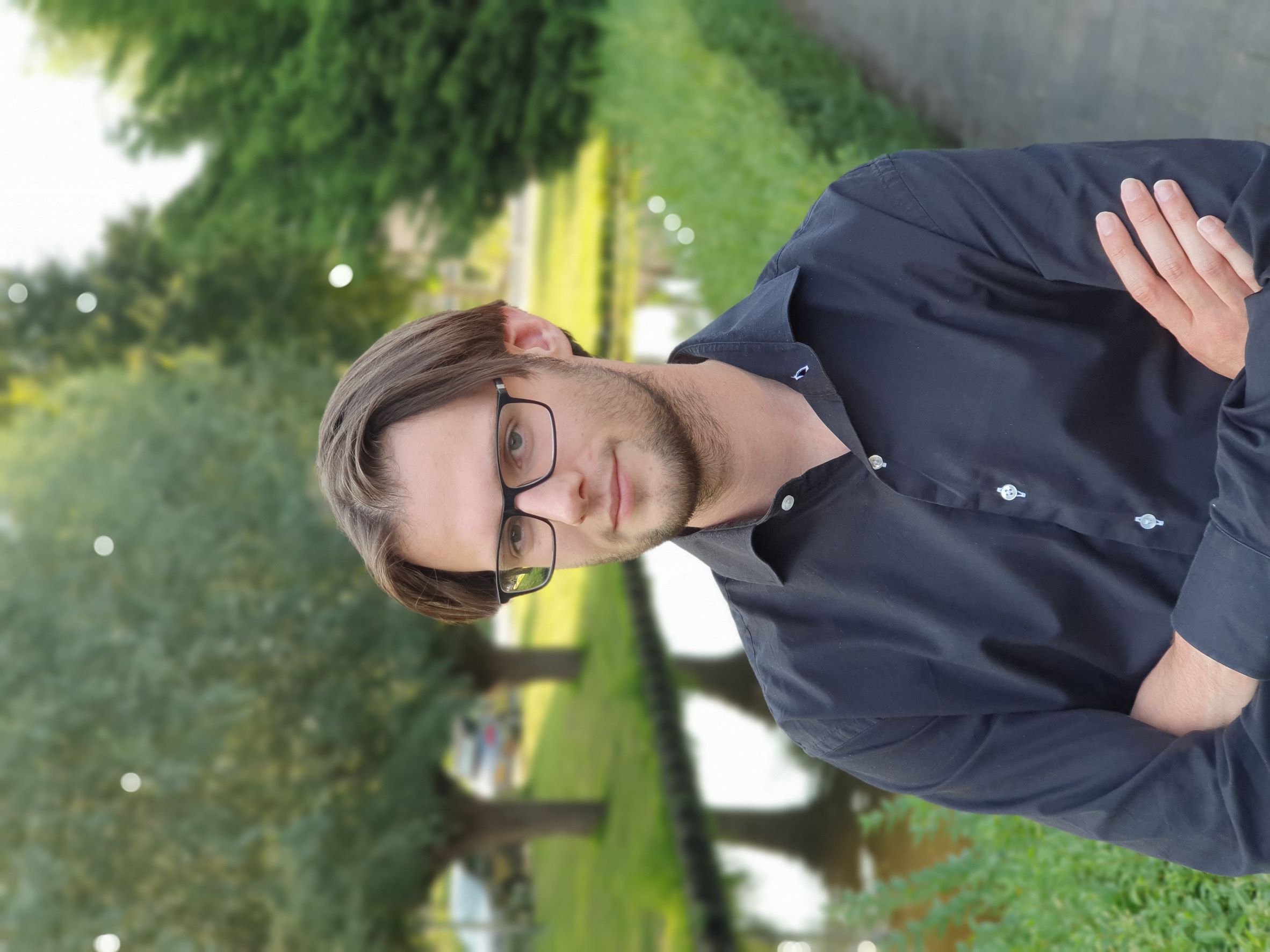
Being able to estimate atrial tissue conductivity parameters from epicardial electrograms is an important tool in diagnosing and treating heart rhythm disorders such as atrial fibrillation. One of these parameters is the atrial fibre direction, which is often assumed to be known in conductivity estimation methods.
In this thesis, a novel method to estimate the fibre direction from epicardial electrograms is presented. This method is based on local conduction slowness vectors of a propagating activation wave, which can be calculated from a corresponding activation map of the atrial tissue. These conduction slowness vectors follow an elliptical pattern that strongly depends on the underlying conductivity parameters. The fibre direction and conductivity anisotropy ratio can therefore be estimated by fitting an ellipse to the conduction slowness vectors.
Applying the presented method on simulated data shows that it can accurately estimate the fibre direction, and that the performance of the method depends mostly on the range of wavefront directions present in the measurement area. The main advantage of the presented method over existing methods is that it still functions in the presence of conduction blocks, as long as the surrounding tissue is approximately homogeneous.
Additional information ...
TU Delft Women+ In Engineering
- Friday, 24 June 2022
- 16:00-18:00
- Pulse Breakout
End of year event

W+IE (Women+ in Engineering) invites you to this end of year meeting where we can enjoy drinks and snacks together and learn from our guest speakers from Qorvo WiT and TU Delft Integrity Office. We'll also learn more about gender issues by playing the Dilemma game.
Additional information ...
PhD Thesis Defence
- Thursday, 23 June 2022
- 15:00-16:30
- Aula Senaatszaal
Multiband channel estimation for precise localization in wireless networks
Tarik Kazaz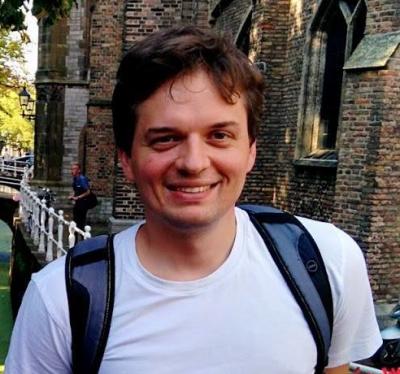
Can we reach decimeter accuracy on wireless localization?
Additional information ...
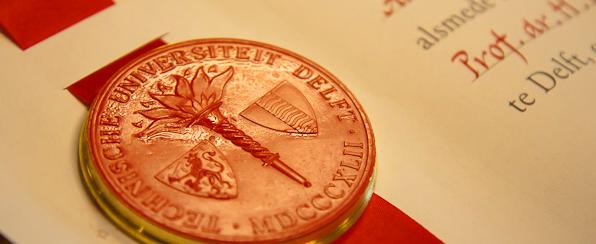
MSc SS Thesis Presentation
- Friday, 17 June 2022
- 14:00-15:00
- Van der Poelzaal (LB01.220)
Link adaptation and equalization for underwater acoustic communication using machine learning
Mauries van Heteren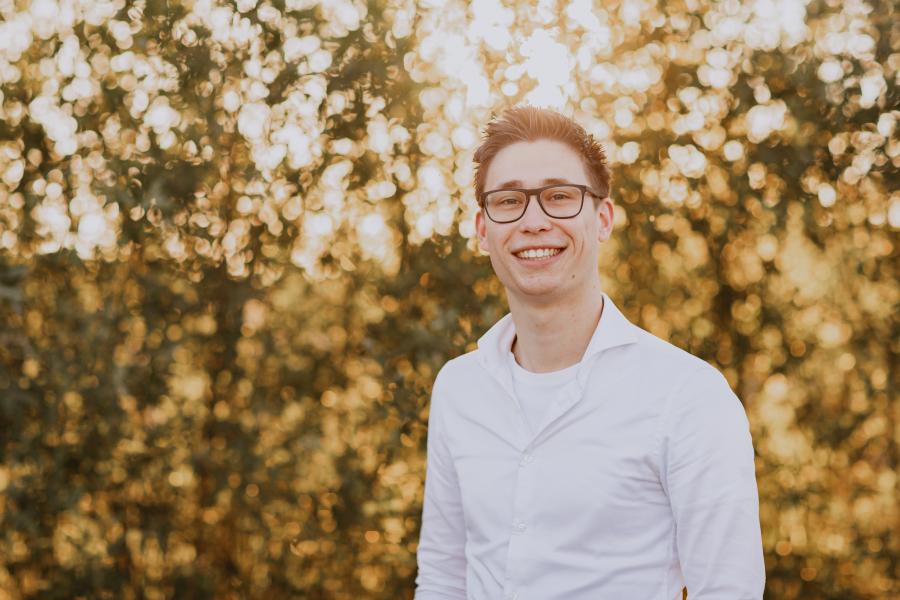
The underwater acoustic environment is amongst the most challenging mediums for wireless communications. The three distinct challenges of underwater acoustic communication are the low and nonuniform propagation speed, frequency-dependent attenuation and time-varying multipath propagation. To cope with these challenges, physical layer communication systems allow the selection of communication parameters based on environmental conditions and constraints. This is also known as link adaptation.
In this thesis, the frequency-repetition spread-spectrum (FRSS) physical layer is studied. Various channel parameters are used to classify the optimal FRSS format. Furthermore, different machine learning classifiers are implemented to solve the classification problem. It is determined that the output signal-to-noise ratio provides enough information to switch effectively between transmission formats. Among the implemented machine learning classifiers, the decision tree strikes a good balance between performance and computational complexity. It is shown that a small performance gain can be achieved when custom channel parameters are extracted from the estimated impulse response and the equalizer error sequence using a deep neural network.
Optimizing the equalization process is another method to better cope with difficult environmental conditions. Various adaptive filter algorithms are implemented for the decision feedback equalizer used in the FRSS receiver. The optimal algorithm parameters are found by means of algorithm unrolling. It is shown that the standard least mean squares algorithm cannot be outperformed by various other optimization algorithms that use linear or non-linear filters.
The Watermark channel simulator is used to study the performance of the link adaptation and equalization optimization solutions for a wide range of underwater channels.
Additional information ...
CAS MSc Midterm Presentations
- Thursday, 16 June 2022
- 13:30-14:30
- Online [zoom]
IMU-based Adaptive Motion Artifact Reduction in Wearable ECG
Cesar Eduardo Cornejo Ramirez
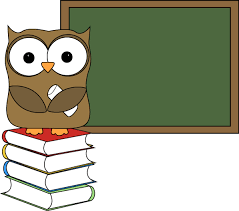
CAS MSc Midterm Presentations
- Thursday, 16 June 2022
- 13:00-14:30
- Online [zoom]
Novel View Synthesis of HDB Façade in Singapore using Neural Radiance Fields
Chuhan WANG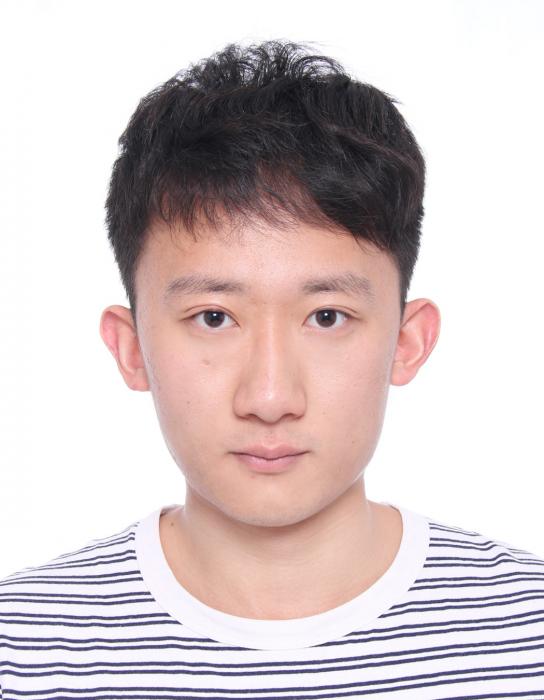
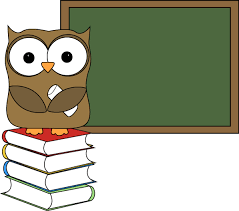
CAS MSc Midterm Presentations
- Thursday, 16 June 2022
- 13:30-14:30
- Online [zoom]
Extreme-value Neural Networks for Weather Forcasting
Zhiyi WANG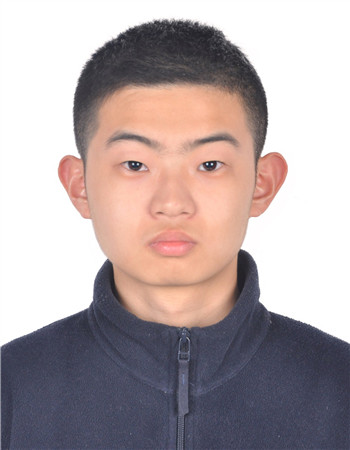
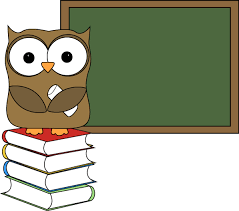
PhD Thesis Defence
- Wednesday, 15 June 2022
- 15:00-16:30
- Aula Senaatszaal
Modelling and analysis of atrial epicardial electrograms
Miao Sun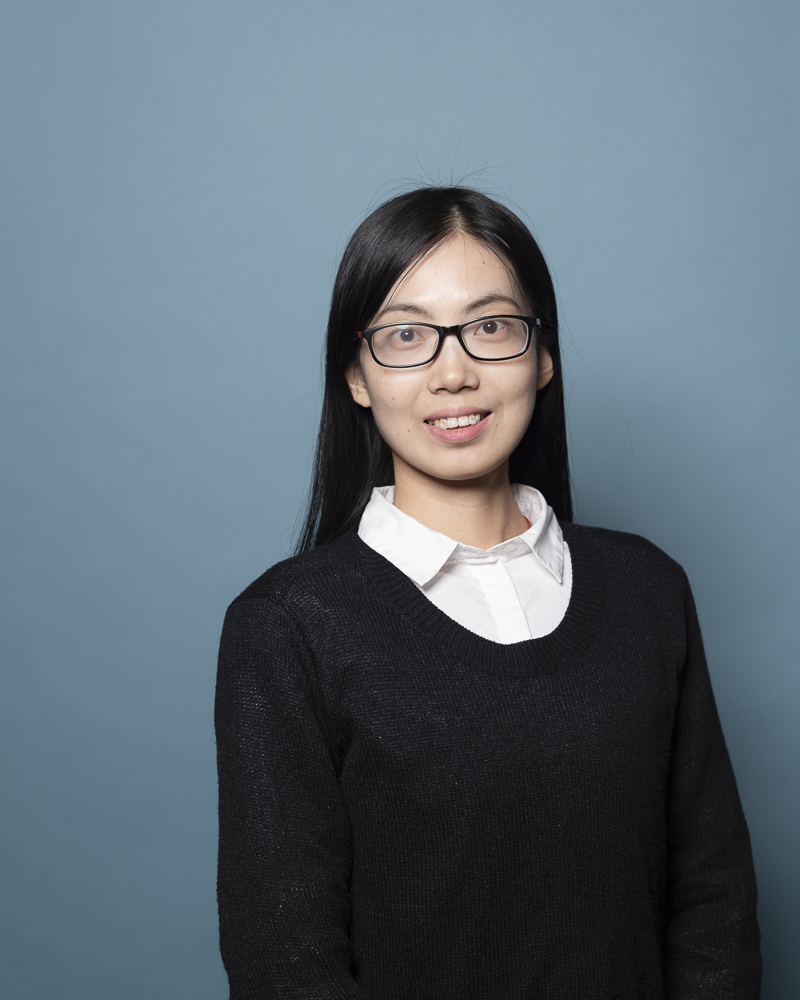
Atrial fibrillation (AF) is a frequently encountered cardiac arrhythmia characterized by rapid and irregular atrial activity, which increases the risk of strokes, heart failure and other heart-related complications. The mechanisms of AF are complicated. Although various mechanisms were proposed in previous research, the precise mechanisms of AF are not clear yet and the optimal therapy for AF patients are still under debated. A higher success rate of AF treatments requires a deeper understanding of the problemof AF and potentially a better screening of the patients.
In order to study AF, instead of using human body surface ECGs, we use the epicardial electrograms (EGMs) obtained directly from the epicardial sites of the human atria during open heart surgery. This data is measured using a high-resolution mapping array and exhibits irregular properties during AF. Although different studies have analyzed electrograms in time and frequency domain, there remain many open questions that require alternative and novel tools to investigate AF.
Experience in signal processing suggests that incorporating the spatial dimension into the time-frequency analysis on the multi-electrode electrograms may provide improved insights on the atrial activity. However, the electrophysiologcial models for describing spatial propagation are relatively complex and non-linear such that conventional signal processing methods are less suitable for a joint space, time, and frequency domain analysis. It is also difficult to use very detailed electrophysiologcial models to extract tissue parameters related to AF fromthe high-dimensional data.
In this dissertation, we propose a radically different approach to study and analyze the EGMs from a higher abstraction level and from different perspectives to get more understanding of the characteristics of AF. We also develop a simplified electrophysiological model that can capture the spatial structure of the data and propose an efficient method to estimate the tissue parameters, which are helpful to analyze the electropathology of the tissue, e.g., cell activation time or conductivity.
Additional information ...
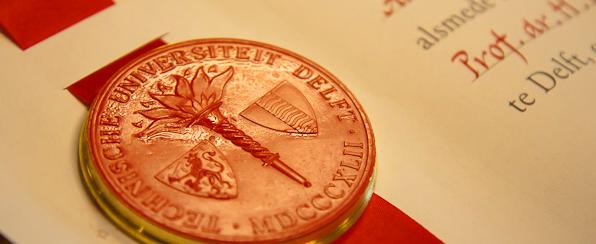
CAS MSc Midterm Presentations
- Thursday, 9 June 2022
- 13:30-14:30
- Online [zoom]
Reconstruction and Rendering of Buildings as Radiance Fields for View Synthesis
Enpu CHEN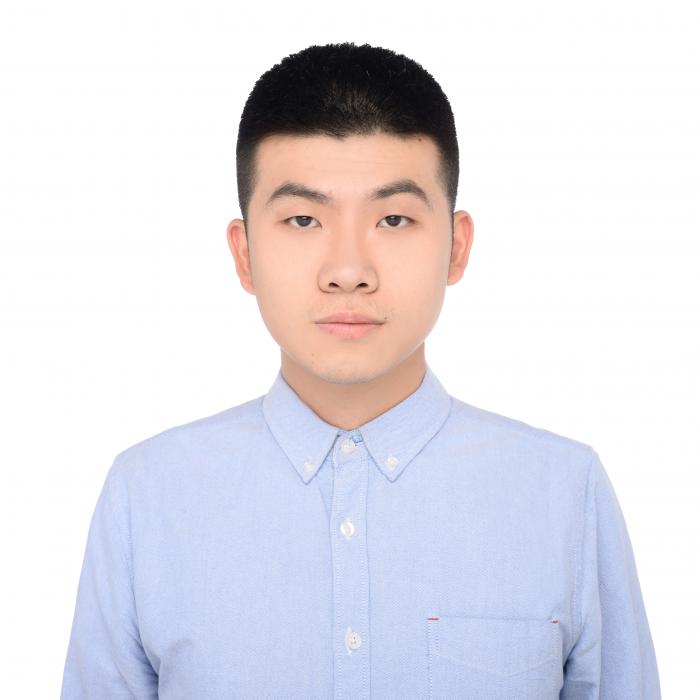
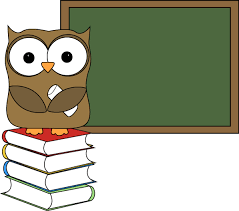
Signal Processing Seminar
- Thursday, 9 June 2022
- 13:30-14:30
- Online [zoom]
Data Processing on Expanding Graphs with Graph Filters
Bishwadeep Das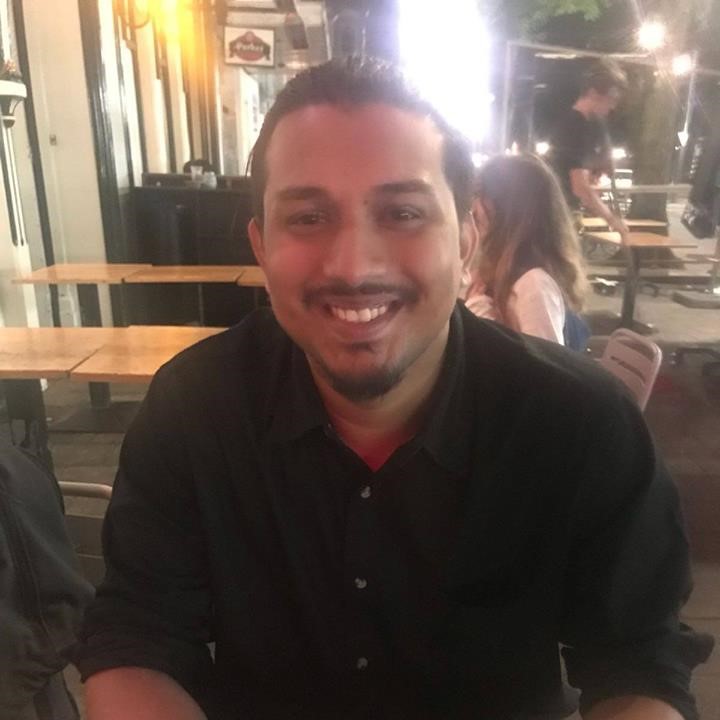
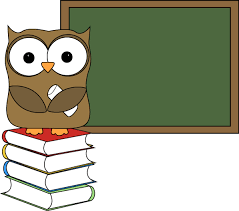
PhD Thesis Defence
- Wednesday, 8 June 2022
- 09:30-11:30
- Aula Senaatszaal
Direction of Arrival estimation and Self-Calibration techniques using an array of Acoustic Vector Sensors
Krishnaprasad Nambur Ramamohan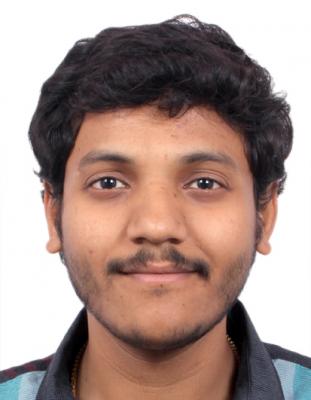
The localization and characterization of sound have played a vital role in various applications, ranging from noise control of machinery to battlefield awareness. Microphone arrays are commonly used to find sound sources, which implicitly inherits a series of limitations. Alternatively, acoustic vector sensors (AVSs) have shown promising results in overcoming most of those limitations, specifically having a larger operation frequency while requiring a smaller number of sensor nodes. However, literature about this topic is still evolving and mainly focused on the theoretical aspects, disregarding most real-world limitations. This thesis extends the AVS arrays' theoretical framework for direction-of-arrival (DOA) estimation of far-field sources while considering practical constraints. Specifically, the study considers the DOA estimation problem using AVS arrays in three main scenarios: spatially under-sampled configurations, the presence of calibration errors, and sensors with a reduced number of channels.
The idea of spatial sampling by AVS arrays has a different interpretation compared to the equivalent acoustic pressure sensor (APS) arrays. Notably, it is possible to carry out unambiguous DOA estimation using a spatially under-sampled AVS array, which is the main topic of interest in the first part of this work. Here we study the effects of the grating lobes or spatial aliasing on the performance of DOA estimation. We will observe that this idea can also be extended to beamforming applications.
Subsequently, in the second part of this work, we consider the DOA estimation problem using AVS arrays in the presence of calibration errors. First, identifiability conditions are derived for the solution to exist. Then two main classes of self-calibration approaches are proposed. The first calibration approach is array geometry independent and is based on sparse recovery techniques that lead to a one-step solver to estimate both the source DOAs and the calibration parameters jointly. Further, the extension of the proposed selfcalibration approach in the presence of wide-band sources is also presented. The second calibration approach applies only to a uniform linear array (ULA) of AVSs, where the Toeplitz block structure of its covariance matrix is exploited to estimate the calibration errors followed by the estimation of the source DOAs.
In the last part of the thesis, an alternate configuration of an AVS is considered for DOA estimation with a reduced channel count. We refer to such an AVS as a uniaxial AVS (U-AVS). The DOA estimation performance using a U-AVS array is analyzed, and specifically, the impact of the extra degree-of-freedom originating from the fact that each U-AVS in the array can have arbitrary orientation is studied comprehensively. Furthermore, all the analyses and proposed algorithms in this thesis are supported by real experimental results performed with AVS arrays in an anechoic chamber.
To conclude, this research on AVS arrays paves the way to achieve an increased situational awareness across our society; this could be either by detecting and localizing problems or threats occurring in an urban environment or assisting soldiers on the battlefield to make a timely decision to achieve peace.
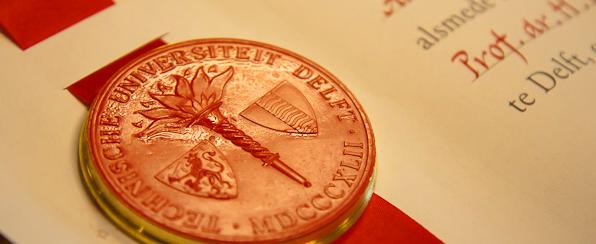
CAS MSc Midterm Presentations
- Thursday, 19 May 2022
- 13:30-14:30
- Online [zoom]
Link Adaptation and Equalization for Underwater Acoustic Communication Using Machine Learning
Mauries van Heteren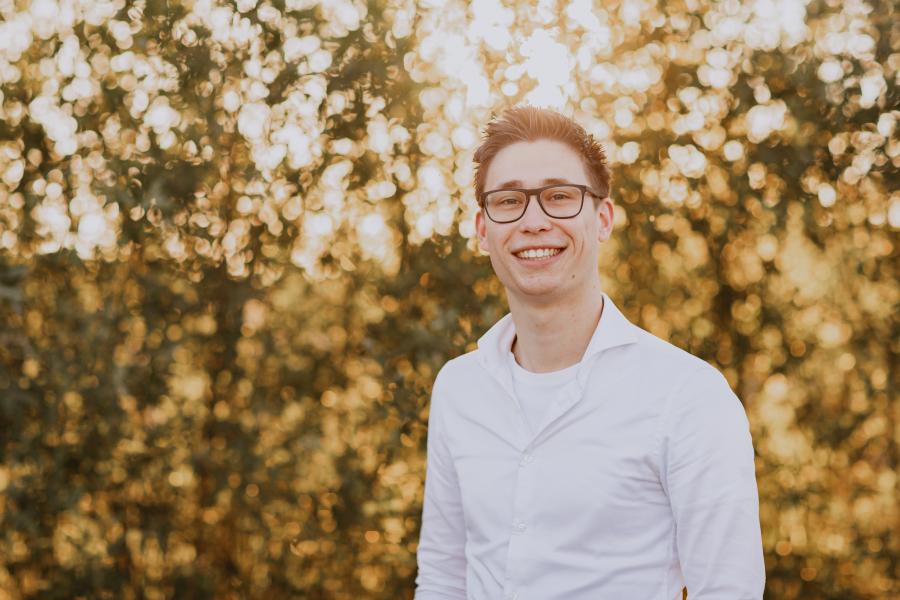
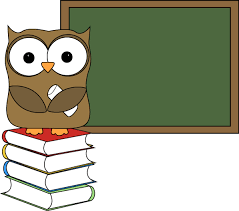
CAS MSc Midterm Presentations
- Thursday, 19 May 2022
- 13:30-14:30
- Online [zoom]
IMU-based Adaptive Motion Artefact Reduction in Wearable ECG
Cesar Eduardo Cornejo Ramirez
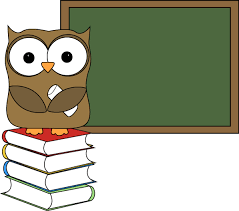
CAS MSc Midterm Presentations
- Thursday, 19 May 2022
- 13:30-14:30
- Online [zoom]
Finding Camera Position and Orientation using Marker-Less Computer Vision in the Catheterization Laboratory
Jinchen ZENG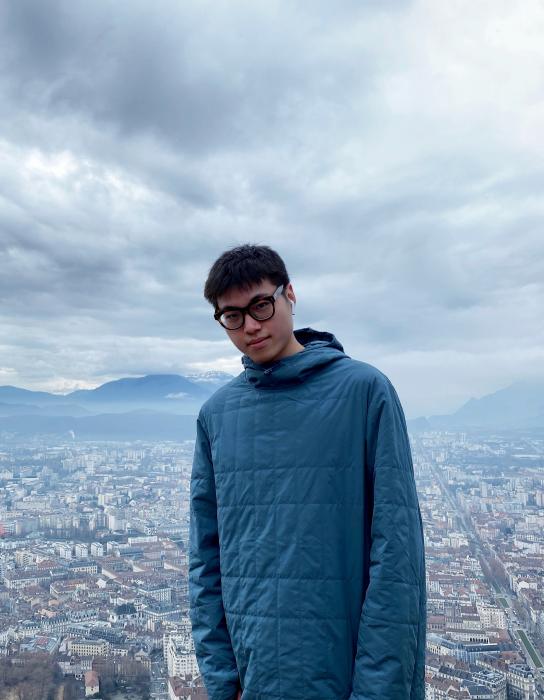
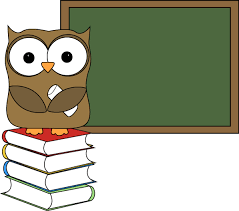
MSc SS Thesis Presentation
- Monday, 16 May 2022
- 09:30-10:15
- Timmanzaal (LB01.170)
Multiple Subbands Ranging Signals Design and Investigation on Frequency Dependence of the Subband Channel Impulse Responses within an Ultra-wideband Channel
Xiaoyao Luo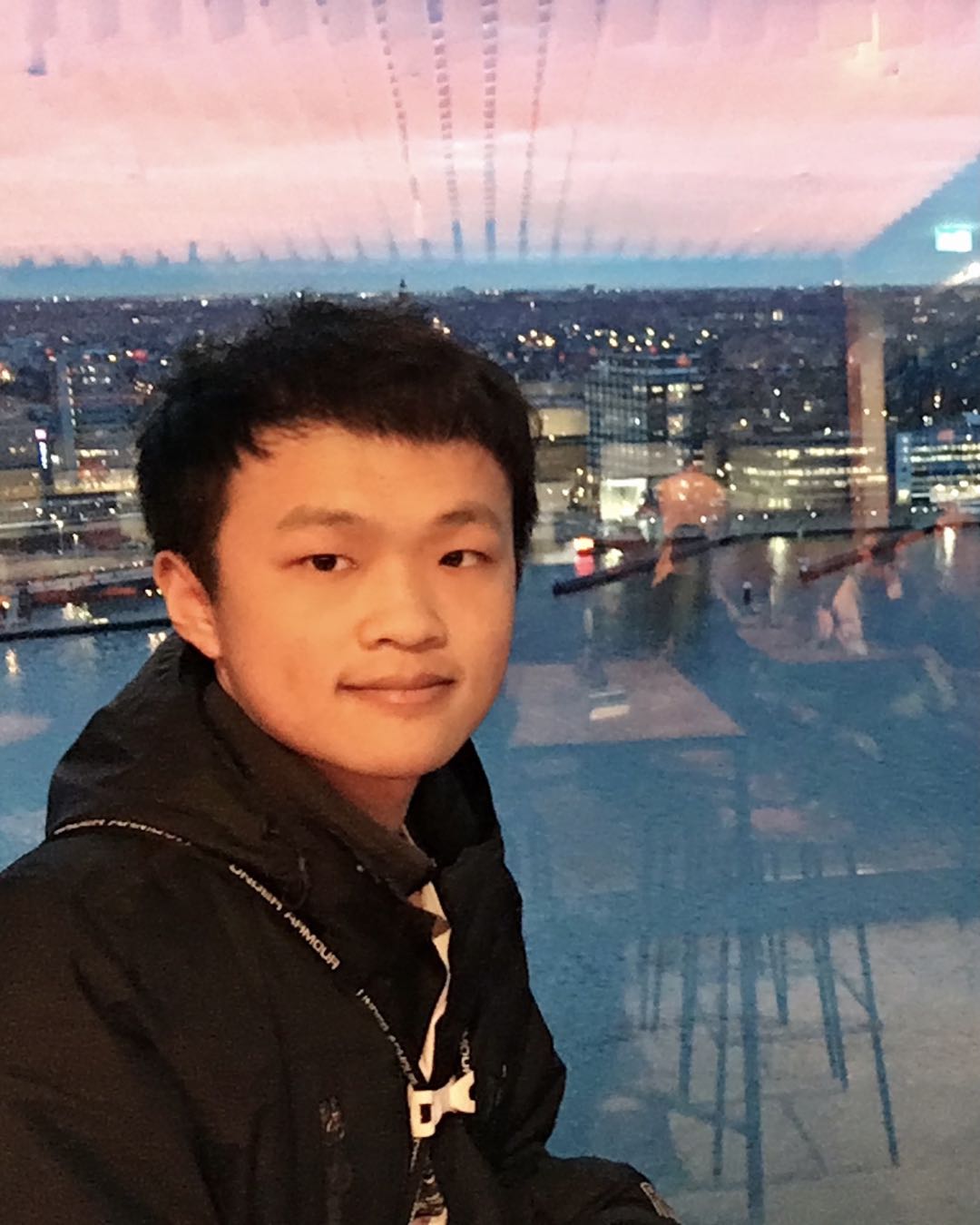
This thesis covers two topics. The first one is signal design for accurate Time-of-Arrival estimation using a number of frequency separated signals. Rather than use a full UWB band, we will using sparse subband signals spanning the full band to construct a new virtual UWB signal. To evaluate the performance of the constructed signal, Cramerrao lower bound and auto-correlation are used. And given a given fixed bandwidth the number of subbands within a 1 GHz UWB channel, optimal subbands’ allocation will be found based on the evaluation results. Our results show that when three 50 MHz subbands are used to construct a virtual 1GHz UWB signal, a lower CRLB and better auto-correlation performance can be reached when subband are close to the edges of the virtual band. However, the autocorrelation still has multiple peaks, which poses a serious challenge for accurate time estimation.
The second topic is to investigate the frequency dependence of the channel impulse response of subbands with different frequency separations. We propose a covariance calculation method to determine the frequency dependence which changes with frequency separation. To validate the method, different artificial UWB channels with distinct paths are given. The results show that covariances between the subband CIRs stay at high level when measured at the direct path and the majority interference caused by other paths can be eliminated by wider bandwidth subband. Given UWB channels measured from 5 to 10 GHz with a link-budget of 120dB, the frequency dependence of the direct path and reflections are determined, different bandwidths and frequency separations are used, the results show that the channel impulse response of the subbands will become different when measured at different center frequencies, where the difference increases with increased frequency separation of the subbands. The correlation of the direct path is maintained over larger frequency distances than that of reflected paths.
Additional information ...
CAS MSc Midterm Presentations
- Thursday, 12 May 2022
- 13:30-14:30
- Online [zoom]
Re-ranking for Improved Image Query-Based Search
Qi ZhangAdditional information ...
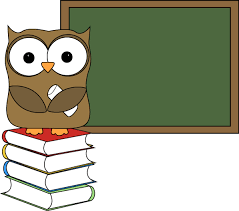
CAS MSc Midterm Presentations
- Thursday, 12 May 2022
- 13:30-14:30
- Online [zoom]
Detecting Medical Equipment in the Catheterization Laboratory using Computer Vision
Renjie Dai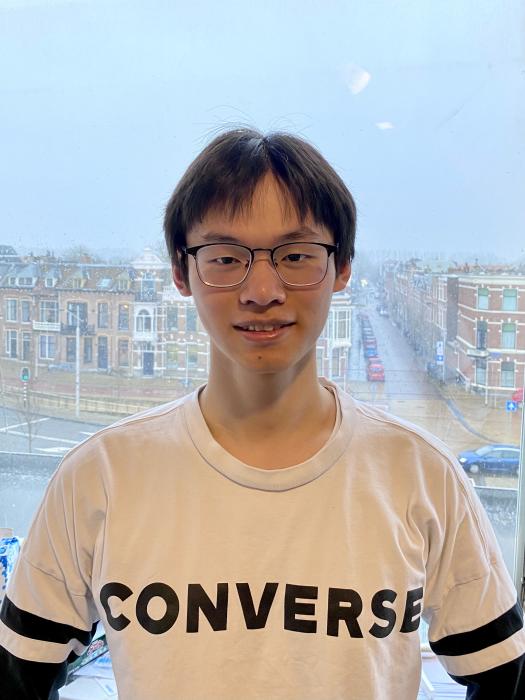
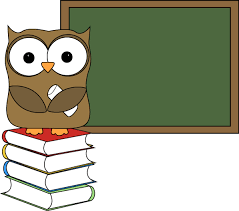
CAS MSc Midterm Presentations
- Thursday, 12 May 2022
- 13:30-14:30
- Online [zoom]
Distributed Gaussian Process on Multi-Agents Network for Environmental Monitoring
Peiyuan Zhai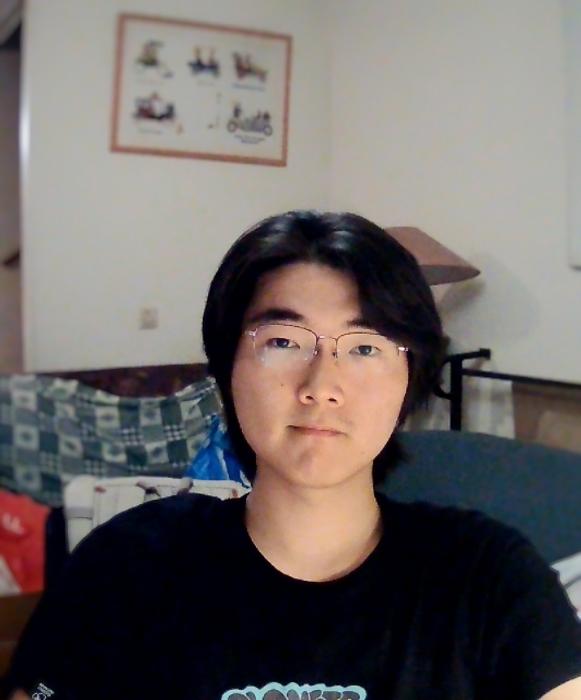
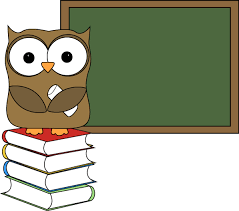
PhD Thesis Defence
- Monday, 2 May 2022
- 10:00-11:30
- Aula Senaatszaal
Image Reconstruction for Low-Field MRI
Merel de~Leeuw den Bouter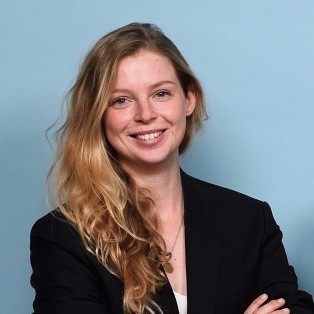
This thesis presents imaging algorithms for a prototype low-field MRI system, in particular regularization, handling missing data, and deep learning approaches.
Additional information ...
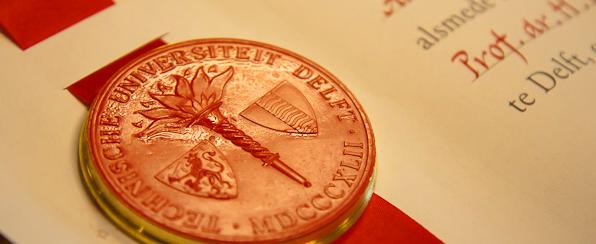
CAS MSc Midterm Presentations
- Thursday, 28 April 2022
- 13:30-14:30
- Online [zoom]
Frequency Domain Joint Estimation of HRF and Stimulus from Functional Ultrasound Data
Yitong Tao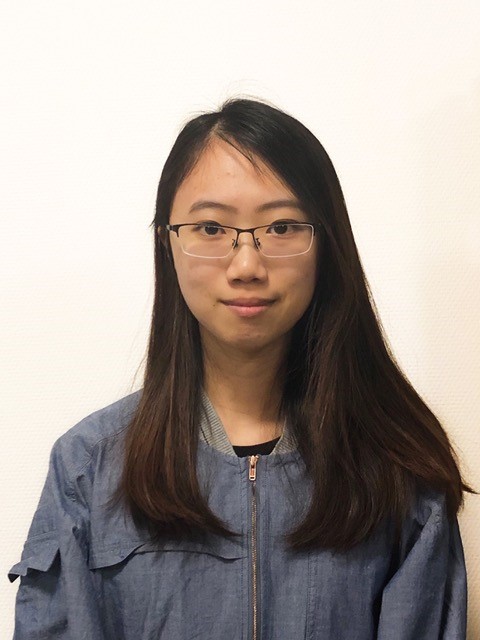
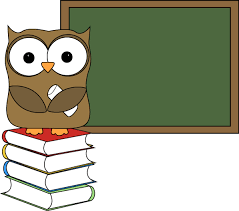
CAS MSc Midterm Presentations
- Thursday, 28 April 2022
- 13:30-14:30
- Online [zoom]
Cooperative Localization using ADS-B for Unmanned Aerial Vehicles
Xuzhou Yang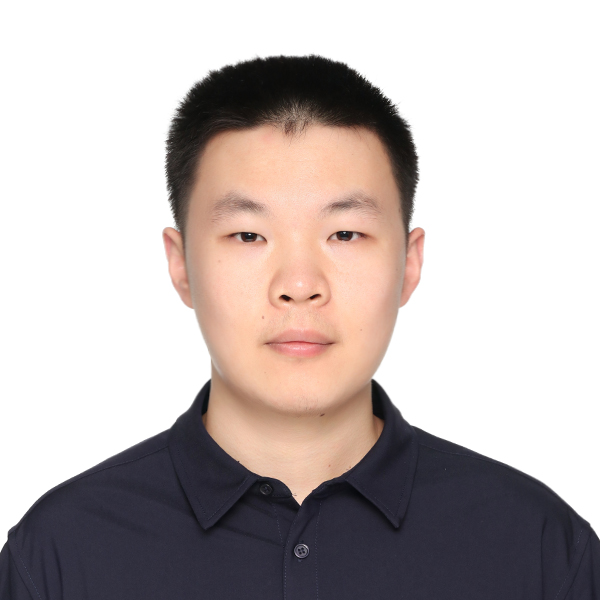
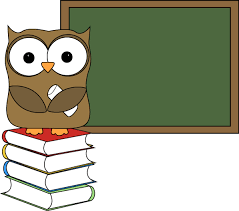
CAS MSc Midterm Presentations
- Thursday, 28 April 2022
- 13:30-14:30
- Online [zoom]
Development of a Detect and Avoid Control System for Autonomous Agents in Cluttered Environments using the Artificial Potential Field Method
Mosab Diab
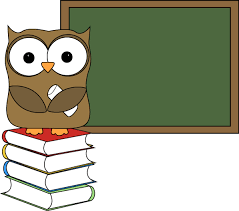
MSc SS Thesis Presentation
- Tuesday, 26 April 2022
- 10:15-11:00
- EWI Snijderszaal
Estimating atrial activity in epicardial electrograms: a beamforming perspective
Tijs Moree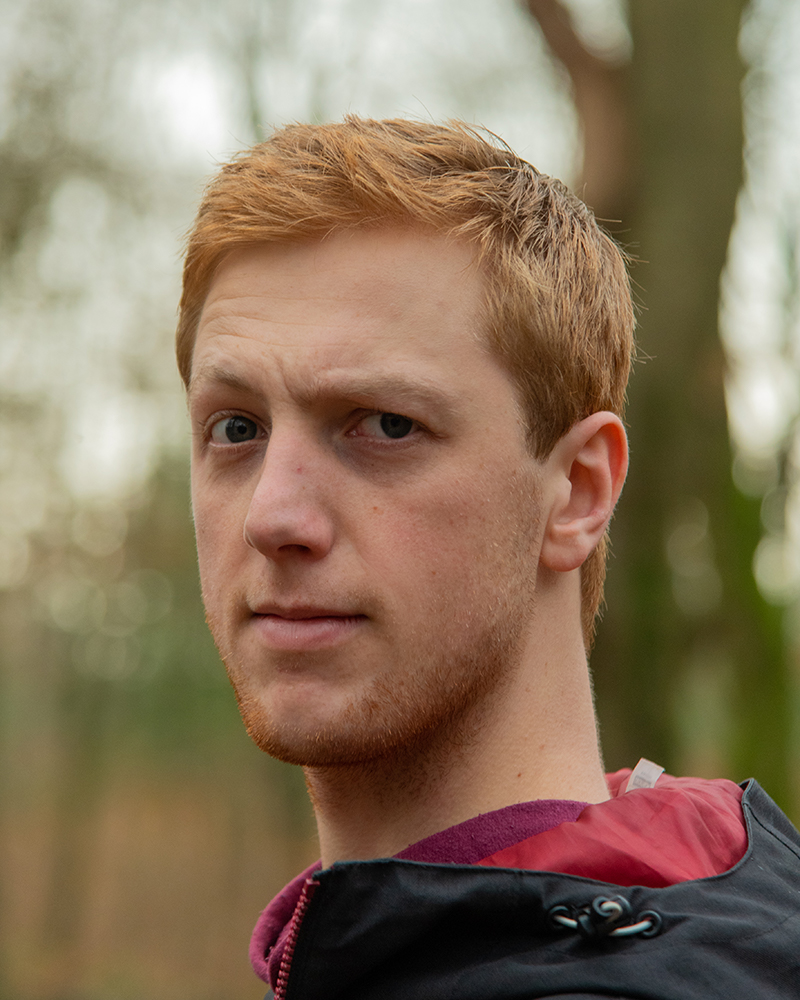
The most common serious heart rhythm disease is atrial fibrillation. It is not fatal on its own but does increase the risk of heart failures and strokes. There is little understanding about the mechanisms behind the disease, so more insight is desired. Using an array of electrodes, measurements are being performed of the electrical atrial activity directly on the heart tissue. These signals are, however, not clean and suffer from far-field interference coming from the ventricles.
During normal sinus rhythm the atrial and ventricular activities are separated in time and easy to distinguish. In case of atrial fibrillation this is not always the case. Luckily, there is a major difference between both signals: the ventricular signal comes from far away and arrives therefore approximately simultaneously at all electrodes. A simple, but effective way to remove this ventricular activity is to use a bipolar electrode. It produces the difference between two normal unipolar electrodes, thus removing the common ventricular signal component.
The bipolar electrode, however, distorts the atrial signal component, which in some orientations can even lead to removing it altogether. This bipolar electrode is known as a differential beamformer from the field of array signal processing. There are more complex beamformers that can keep the atrial component undistorted and therefore produce better results than the bipolar electrode.
This thesis proposes a Fourier-domain signal model for all available electrodes relying on an atrial and ventricular transfer function. It is possible to estimate these transfer functions from the data blindly. Three beamformers are derived utilizing the signal model and the transfer functions. The bipolar electrode is extended to multiple electrodes like the other beamformers as well.
This thesis proposes a Fourier-domain signal model for all available electrodes relying on an atrial and ventricular transfer function. It is possible to estimate these transfer functions from the data blindly. Three beamformers are derived utilizing the signal model and the transfer functions. The bipolar electrode is extended to multiple electrodes like the other beamformers as well.
Experiments with simulated data show that the complex beamformers indeed keep the atrial activity undistorted and are still able to remove the ventricular activity effectively when using multiple electrodes, except for very complex data where the signal model is not valid. For low numbers of electrodes the beamformers are not useful, they hardly remove the ventricular activity while keeping the atrial component undistorted, where the bipolar electrode does the opposite.
The electrograms are also used to estimate local activation times of the cells underneath the electrodes which says something about the health of the cardiac tissue. Besides the mentioned filtering, this thesis proposes a method to estimate those moments in time by looking at the time-domain version of the atrial transfer function, called the atrial impulse response. For simple data, it performs well compared to state-of-the-art methods, but for more complicated data, it does not.
MSc SS Thesis Presentation
- Monday, 25 April 2022
- 14:00-14:45
- (online)
Towards Gridless Sound Field Reconstruction
Ids van der Werf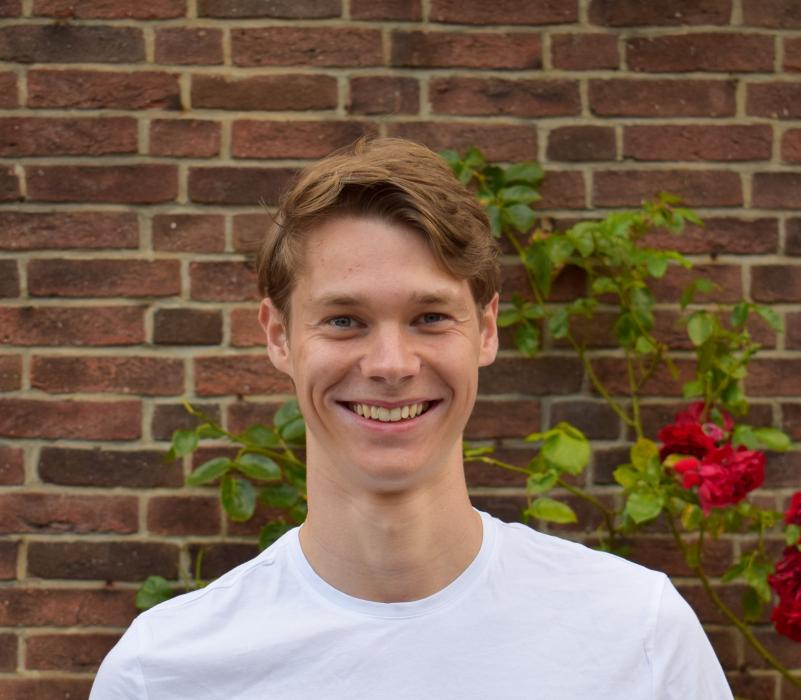
Sound pressure varies over space and time. Knowledge about this exact behavior has many applications, e.g., room compensation, dereverberation and sound field reconstruction. Inside enclosures, the sound field is influenced by the surroundings, such as the geometry of the enclosure and the materials used. Reconstructing a satisfying sound field in the whole enclosure by extrapolating from few measurements is thus not an obvious task.
The sound field in a room can be represented by a weighted sum of room modes. Thus, we can estimate the room modes and compute the sound field from it. To estimate the room modes, compressive sensing literature uses on-the-grid, sparse reconstruction methods. However, these on-the-grid methods are known to suffer from basis mismatch.
In this work, we investigate the use of a gridless framework for estimating room modes using atomic norm minimization, a gridless method. The advantage of this approach is that it does not suffer from this basis mismatch problem. We derive a bound for the sound field reconstruction problem in a one-dimensional room with rigid walls and relate this to the frequency separation that is required by the atomic norm. We conclude that for perfect reconstruction of the room modes based on the investigated gridless approach, additional prior knowledge about the signal model is required. For example, knowledge about the shape of the room modes can be used. We show how recovery is possible in a one-dimensional setting by exploiting both the structure of the sound field and the acquisition method.
Additional information ...
CAS MSc Midterm Presentations
- Thursday, 21 April 2022
- 13:30-14:30
- Online [zoom]
Personnel Activities Observation in the Catheterization Laboratory
Yingfeng Jiang
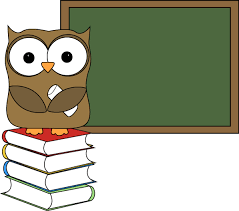
CAS MSc Midterm Presentations
- Thursday, 21 April 2022
- 13:30-14:30
- Online [zoom]
Autonomous Landing of an UAV
Siddhy Ganesh Shetty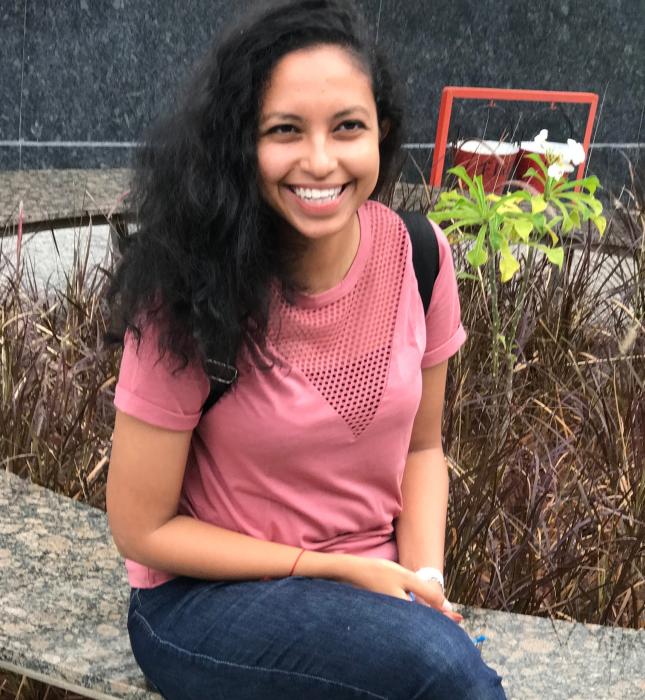
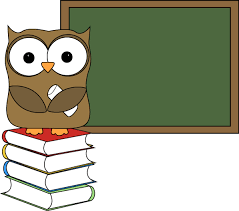
CAS MSc Kick-off Presentations
- Thursday, 21 April 2022
- 13:30-14:30
- Online [zoom]
Machine Learning of Ultrasound Data: Cardiovascular Parameters Detection Using Carotid Artery Ultrasound Measurements
Zhuangzhuang Yu
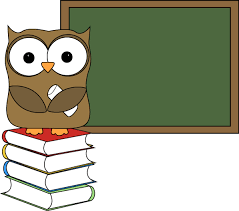
CAS MSc Midterm Presentations
- Thursday, 14 April 2022
- 13:30-14:30
- Online [zoom]
The Impact of Jamming and Spoofing on GNSS Signals
Pim Jansen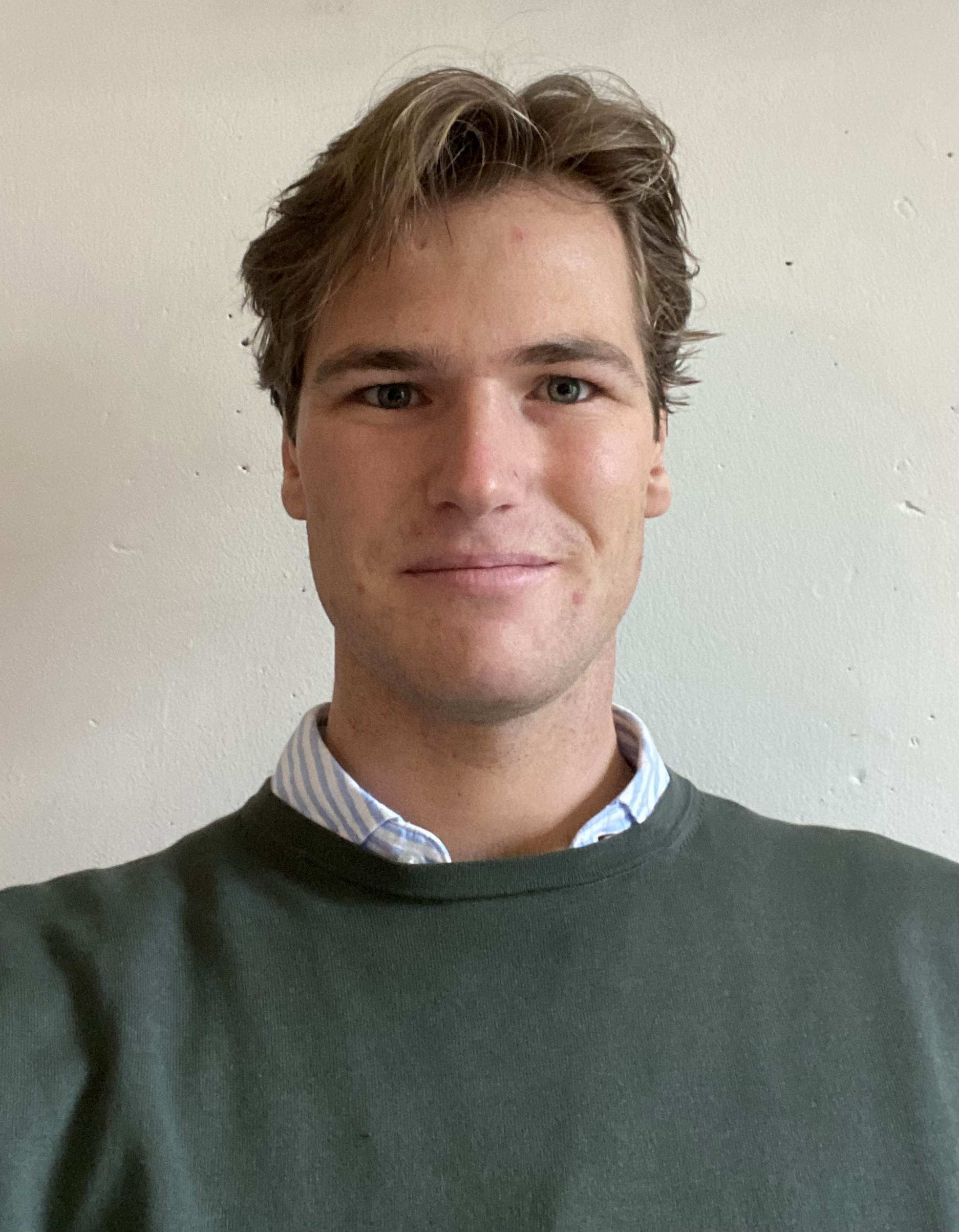
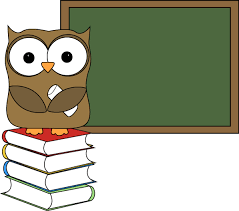
CAS MSc Midterm Presentations
- Thursday, 14 April 2022
- 13:30-14:30
- Online [zoom]
Energy-Efficient Seizure Detection for Wearable EEG
Xiaoning Shi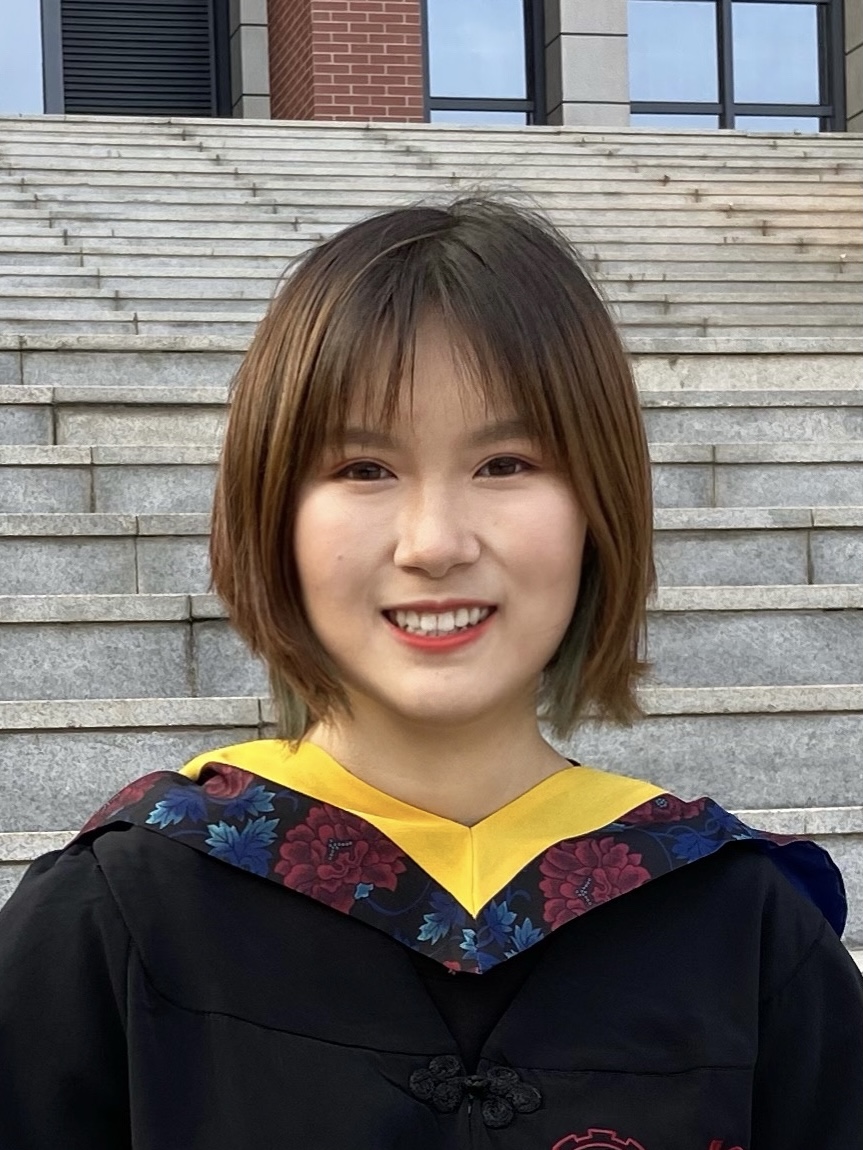
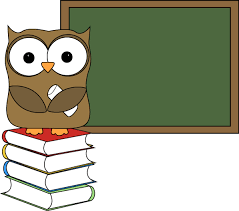
CAS MSc Midterm Presentations
- Thursday, 14 April 2022
- 13:30-14:30
- Online [zoom]
Distributed Particle Filtering
Rui TANG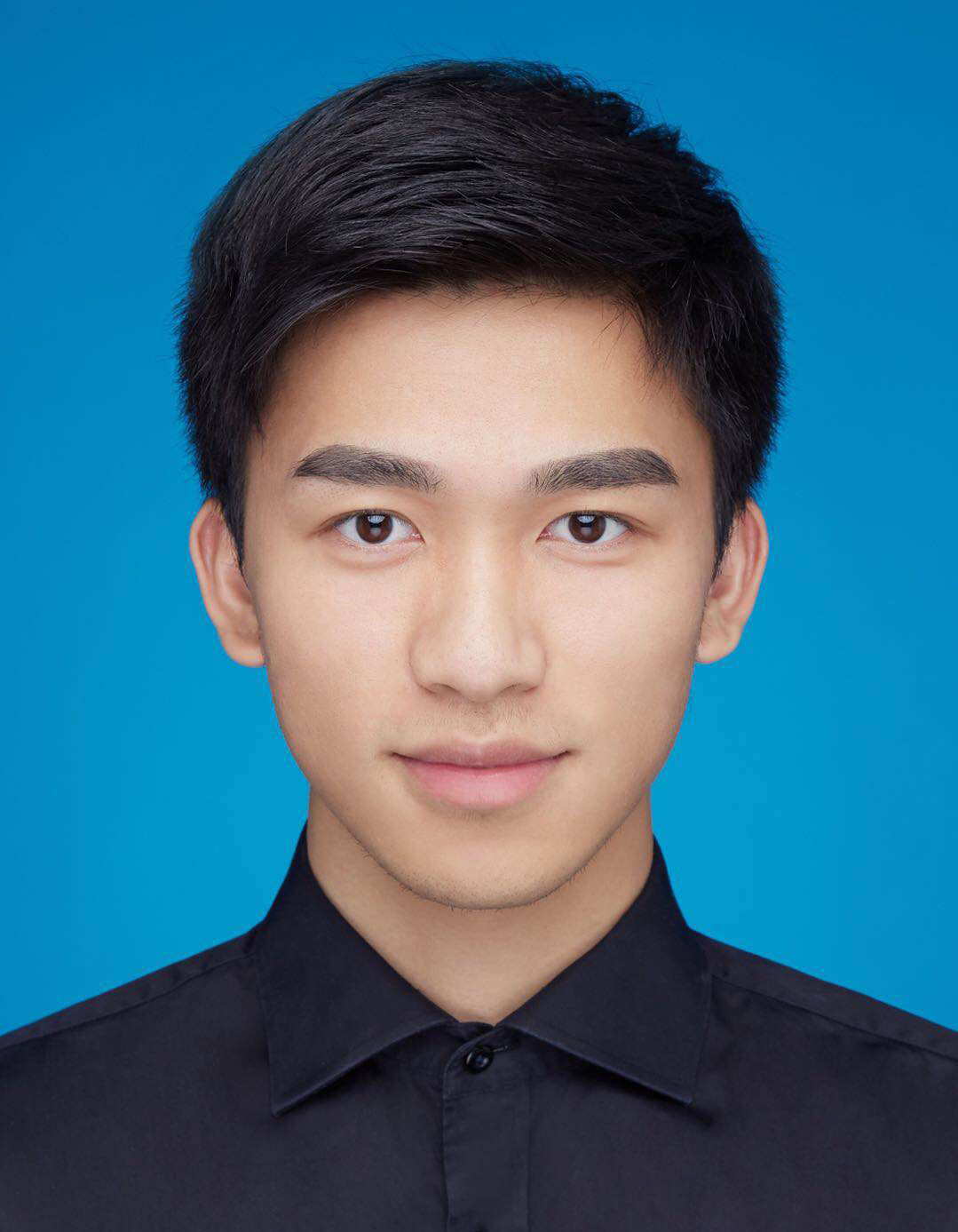
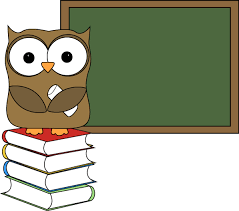
CAS MSc Midterm Presentations
- Thursday, 7 April 2022
- 13:30-14:30
- Online [zoom]
Modular Inductive Biases: To What Extent Can They Improve Neural Reasoning and Generalization?
Nan Lin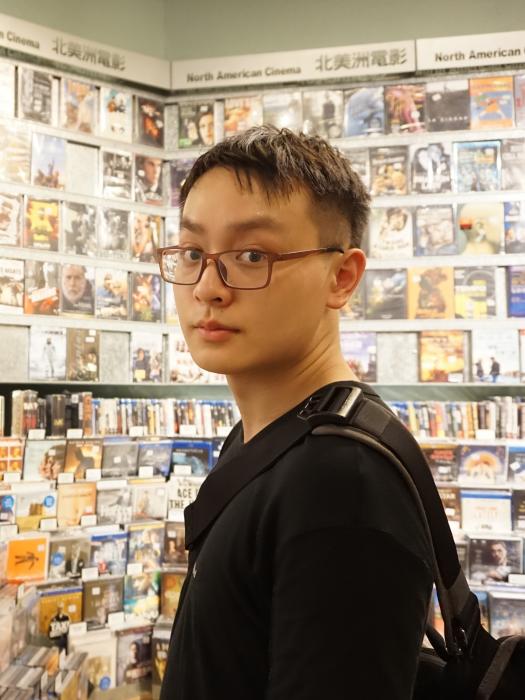
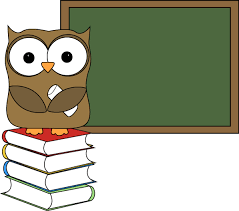
CAS MSc Midterm Presentations
- Thursday, 7 April 2022
- 13:30-14:30
- Online [zoom]
Distributed System Design for Space-based Correlators
Brenda Hernandez Perez
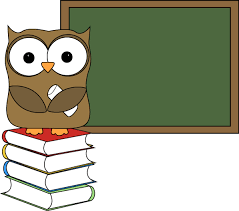
Signal Processing Seminar
- Thursday, 7 April 2022
- 13:30-14:30
- Online [zoom]
Single-Pulse Estimation of Target Velocity on Planar Arrays
Costas Kokke.jpg)
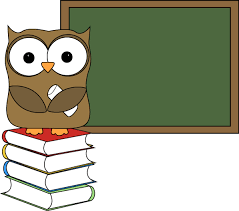
CAS MSc Midterm Presentations
- Thursday, 31 March 2022
- 13:30-14:30
- Online [zoom]
Relative Affine Localization for Robust Formation Control
Zhonggang LI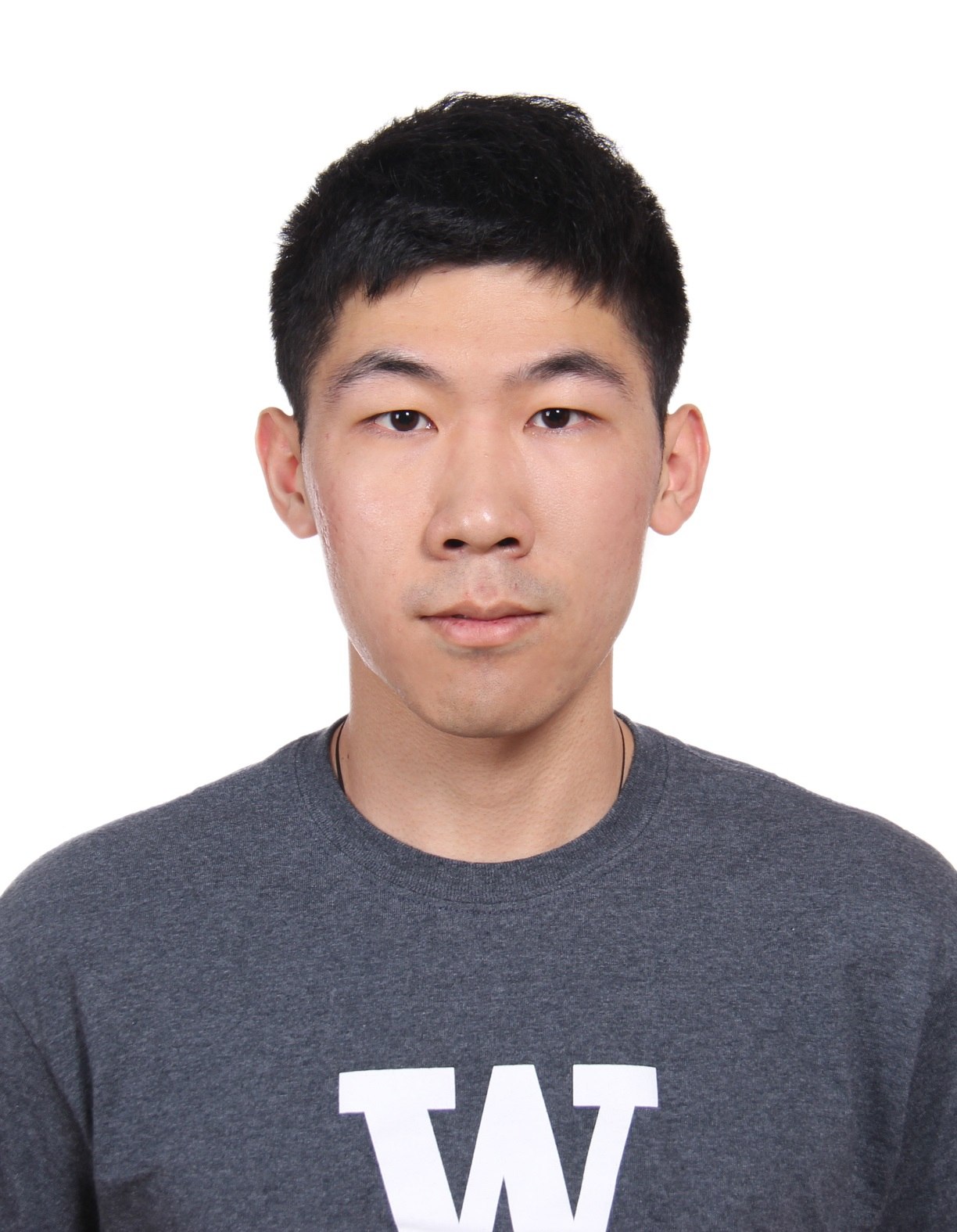
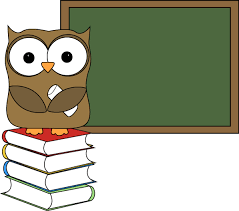
CAS MSc Midterm Presentations
- Thursday, 31 March 2022
- 13:30-14:30
- Online [zoom]
Search by Image: A Digital History Search Engine in Online Image Repositories
Yanan HU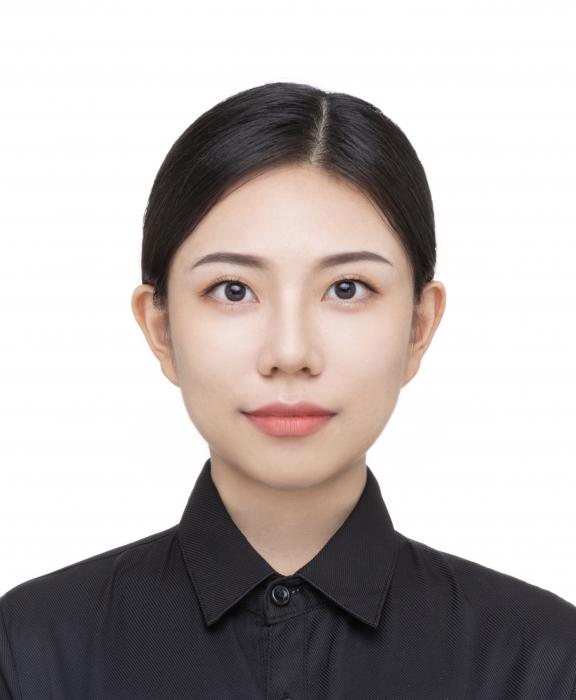
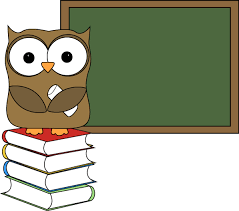
MSc SS Thesis Presentation
- Tuesday, 29 March 2022
- 13:00-13:45
- zoom (and HB17.090)
Few-shot emotion recognition using intelligent voice assistants and wearables
Mihir Kapadia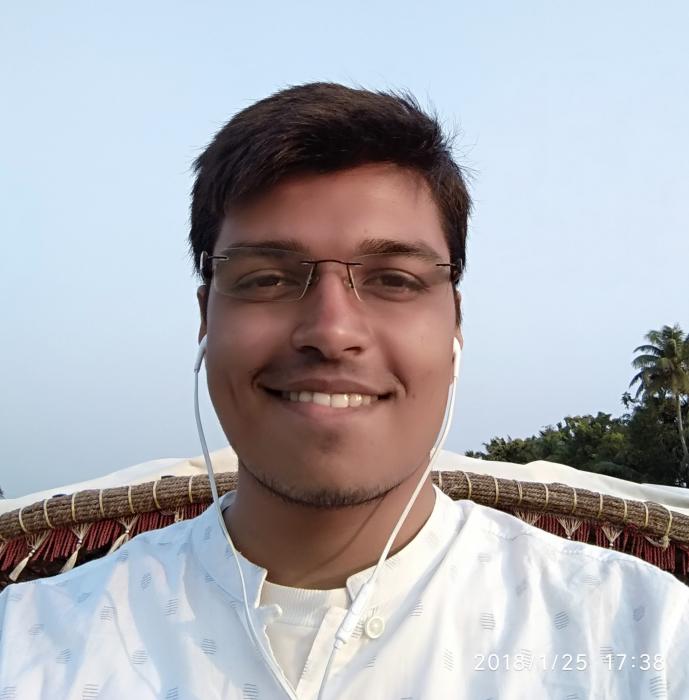
Emotion Recognition is one of the vastly studied areas of affective computing. Attempts have been made to design emotion recognition systems for everyday settings. The ubiquitous nature of Intelligent voice assistants (IVAs) in households, make them a great anchor for the introduction of emotion recognition technology to consumers. The existing systems lack such pipelines and rely on dictionary-based architectures in their design. Further, these systems lack conversational properties and are merely an extension of information retrieval engines.
In this setting, we propose to introduce and develop emotion recognition pipelines that are suited to the interactions, common with these IVAs. To augment the existing emotion recognition pipelines which rely on audio information, we look at physiological information derived from wearables. Our proposed model uses multimodal embeddings with a Siamese Network to achieve the task of emotion recognition from a few samples. Physiological signals of blood volume pulse (BVP) and electrodermal activity (EDA) are used as additional input embeddings to two audio embeddings arising from the speech samples. We employ the state-of-the-art training schedules for Siamese Networks, which use a very limited amount of training on support datasets via sample pair comparisons. The performance of the model is evaluated using weighted binary accuracy and f1 scores.
The proposed model is applied on two datasets that denote two unique experimental settings - the K-EmoCon dataset and RECOLA dataset. We demonstrate an improvement in the state-of-the-art accuracy with the K-EmoCon dataset with accuracies of 63.97% and 66.91% on arousal and valence dimensions respectively. Further, on the RECOLA dataset, the model performs moderately well with 53.81% and 53.87% respectively for arousal and valence dimensions. In addition to this, we present a study of the effects of variation of available support set for training from the dataset. We make some salient observations for these experiments across individual participants and also identify how the label distributions affect the performance of the model. Further, we investigate the impact of real-world noise samples from the DEMAND dataset on the two datasets. We observe that the proposed model is robust and performs sustainingly well even in the presence of imputed noise.
Additional information ...
CAS MSc Midterm Presentations
- Thursday, 24 March 2022
- 14:00-15:00
- Online [zoom]
Distributed Optimization using Stochastic PDMM: Convergence, Transmission Losses and Privacy
Sebastian Jordan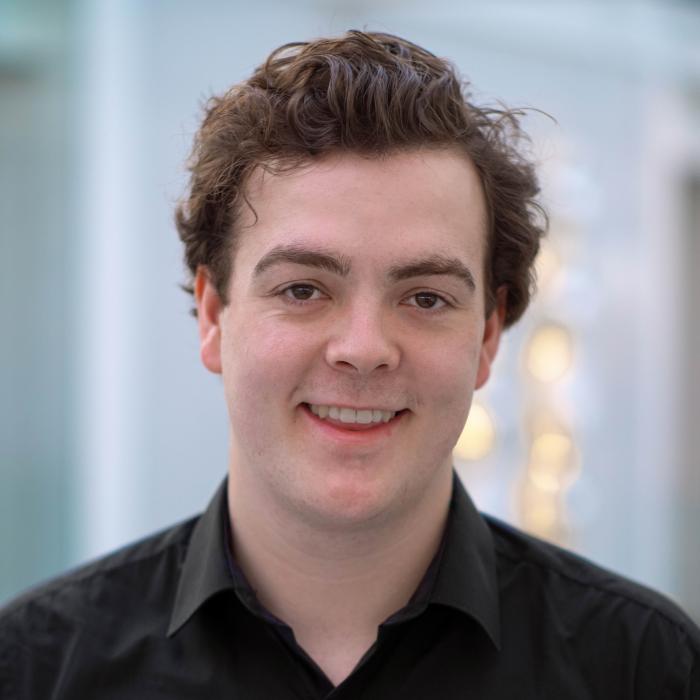
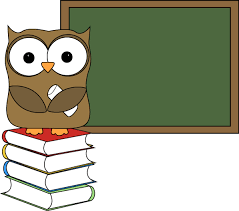
CAS MSc Kick-off Presentations
- Thursday, 24 March 2022
- 14:00-15:00
- Online [zoom]
IMU-based Adaptive Motion Artifact Reduction in Wearable ECG
Cesar Eduardo Cornejo Ramirez
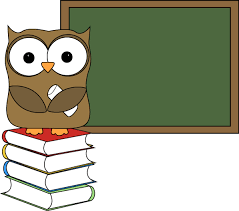
Signal Processing Seminar
- Thursday, 24 March 2022
- 14:00-15:00
- Online [zoom]
High Performance Control System Architecture with an Output Regulation Theory-based Controller and Two-Stage Optimal Observer for the Fine Pointing of Large Scientific Satellites
Valerio FoglianoThis study describes the functional architecture and the control law of an attitude control system designed for large scientific satellites with high accuracy and stability pointing requirements, such as those necessary during the fine pointing operations. The satellite dynamical motion has been reproduced by an accurate software simulator implementing the error models of the sensors and actuators currently being part of the on-board system configuration of state-of-the-art space missions. Moreover the main disturbances affecting the satellite motion during its mission are considered. The control system architecture includes an optimal estimator, the well-known Multiplicative Extended Kalman Filter, modified for the time delay correction of the star tracker measurements by means of a forward state propagation for a correct implementation of measurement update. This estimator is cascaded with a second one specifically used for disturbance acceleration estimation. The estimation task is then coupled with a linear optimal control algorithm combined into an error feedback output regulator, based on a space system design involving the exosystems of the disturbances and reference profiles. The reported control system has analytically been proved to be globally stable and the reported simulations highlight the high performances of the algorithm, capable of fully satisfying a stringent pointing error requirement. Lastly, the proposed attitude control system is shown to be capable of tracking not only step reference trajectories but also sinusoidal time varying signals within a disturbed environment and promises robust performances also in case of possible actuators faults.
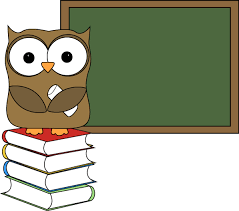
CAS MSc Information Market
- Thursday, 24 March 2022
- 12:30-13:30
- EWI HB 17.110
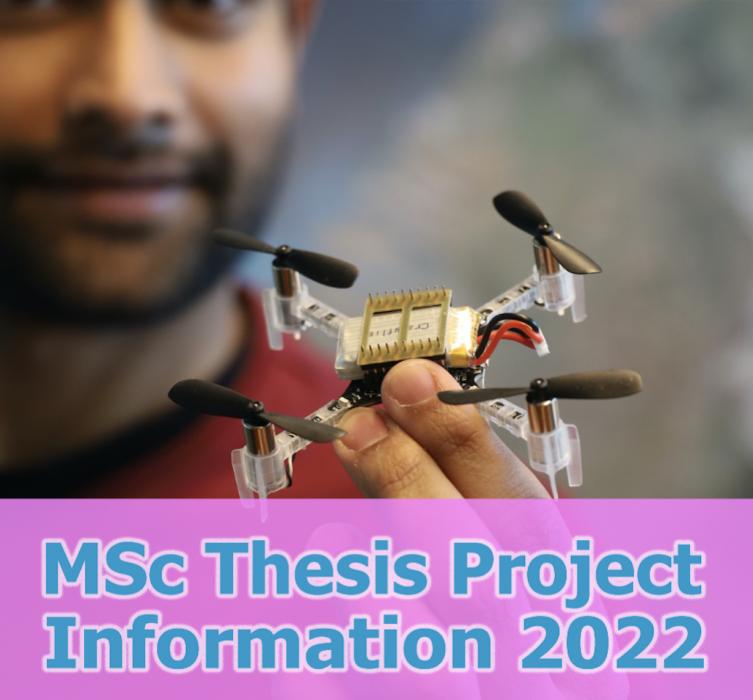
If you are a first year MSc EE student (Signals & Systems, WiCos), then on Thu 24 March, come visit the CAS group at the 17th floor on the EWI tower, meet the professors, and learn about graduation topics for next year.
Signal Processing Seminar
- Thursday, 17 March 2022
- 13:30-14:30
- Online [zoom]
RL-based Path Planning and Coverage for Autonomous UAVs in Unknown Environments
Gianpietro BattoclettiUnmanned Aerial Systems (UASs) have become a relevant sector in the aerospace industry. In the last decade, the increase in the capabilities of Unmanned Aerial Vehicles (UAVs), paired with a drop in their price, has led them to be used in many different applications where they are employed for their versatility and efficiency. A challenge that is being addressed in this field is that of autonomous UAVs fleets, i.e., the coordinated use of multiple UAVs to perform a common task. A particularly interesting application of UAV fleets is their use in the exploration and mapping of unknown or critical environments. This topic brings with it a significant number of challenges, from the design of the policy used to coordinate the fleet to the path planning algorithm that each UAV uses to move in the environment while exploring it. In my research, I studied and implemented a Reinforcement Learning (RL)-based approach for the cooperative exploration of unknown environments by a fleet of UAVs is presented. Different approaches have been considered and compared. A Reinforcement Learning-based approach has been developed taking inspiration from some of the other methods studied. Two RL agents are trained to address the exploration problem: the first has the task of coordinating the coverage task, optimizing the way the UAVs spread in the unknown area by assigning some waypoints to them. The waypoints are placed in order to optimize the distribution of the fleet and to maximize the exploration process efficiency. The second RL agent is a path planning algorithm and is used by each UAV to move in the environment to reach the region pointed by the first agent. The combined use of the two agents allows the fleet to coordinate in the execution of the exploration task.
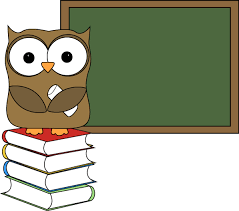
CAS MSc Midterm Presentations
- Thursday, 17 March 2022
- 13:30-14:30
- Online [zoom]
Analyzing Dynamic Functional Connectivity using State-Space Models on Mice fUS Data
Ruben Wijnands
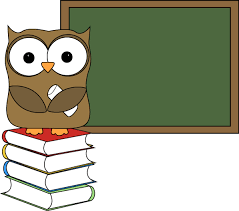
CAS MSc Midterm Presentations
- Thursday, 10 March 2022
- 13:30-14:30
- Online [zoom]
Link Adaptation for Underwater Acoustic Communication using Machine Learning
Mauries van Heteren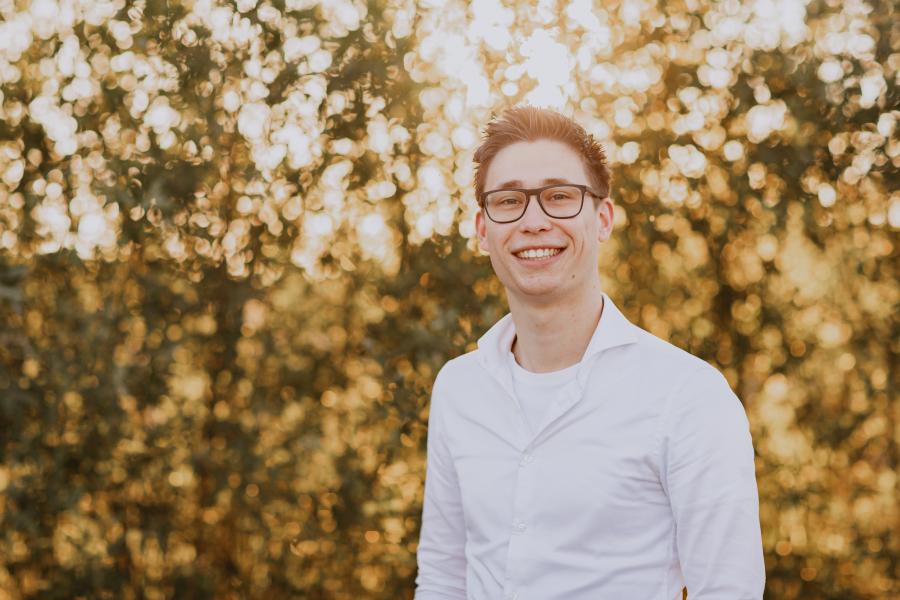
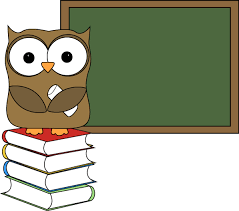
CAS MSc Midterm Presentations
- Thursday, 10 March 2022
- 13:30-14:30
- Online [zoom]
Image Search Engine Based on Deep Learning for Digital History
Yuanyuan YaoAdditional information ...
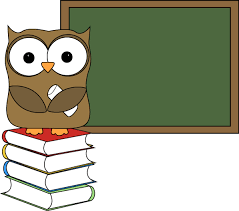
CAS MSc Kick-off Presentations
- Thursday, 10 March 2022
- 13:30-14:30
- Online [zoom]
ICA Through Diagonalization of the Implicitly Formed Higher Order Cumulant Tensor
Pierre-Antoine Denarié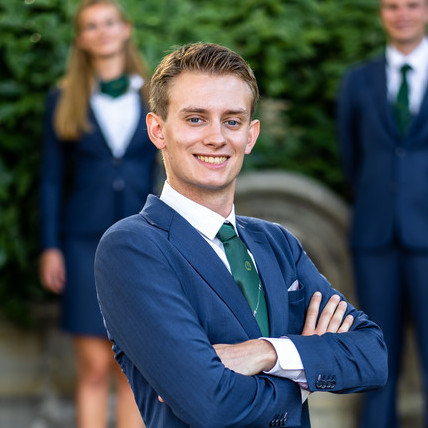
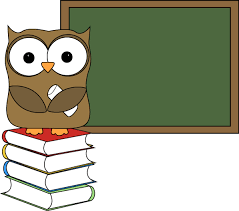
CAS MSc Kick-off Presentations
- Thursday, 24 February 2022
- 13:30-14:30
- Online [zoom]
Detect and Avoid Control System for Autonomous Drones in Cluttered Environments
Mosab Diab
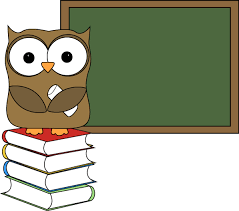
CAS MSc Midterm Presentations
- Thursday, 24 February 2022
- 13:30-14:30
- Online [zoom]
Improving the Estimation of Atrial Activation Times Using Spatial Information
William Hunter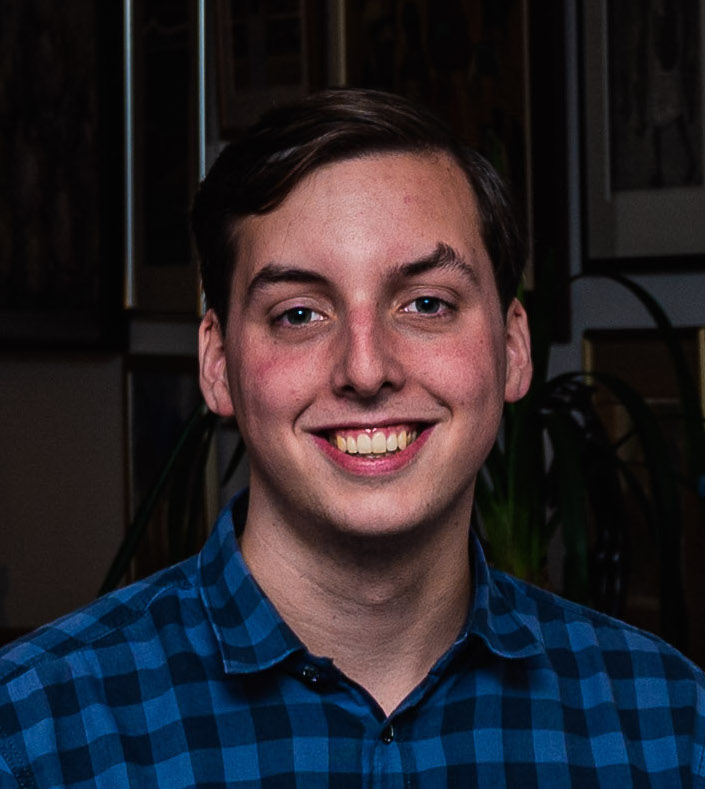
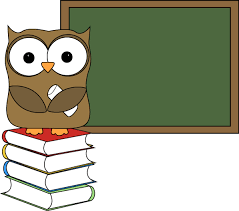
Signal Processing Seminar
- Thursday, 24 February 2022
- 13:30-14:30
- Online [zoom]
Sampling and Reconstruction in IoT Networks on Graphs
Josefine Holm
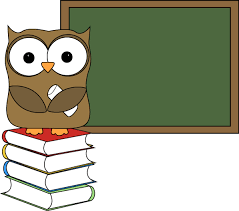
Microelectronics Colloquium
- Thursday, 24 February 2022
- 16:30-05:00
- Online via TEAMS
An inclusive EEMCS faculty: An emphatic approach.
Jorge Martinez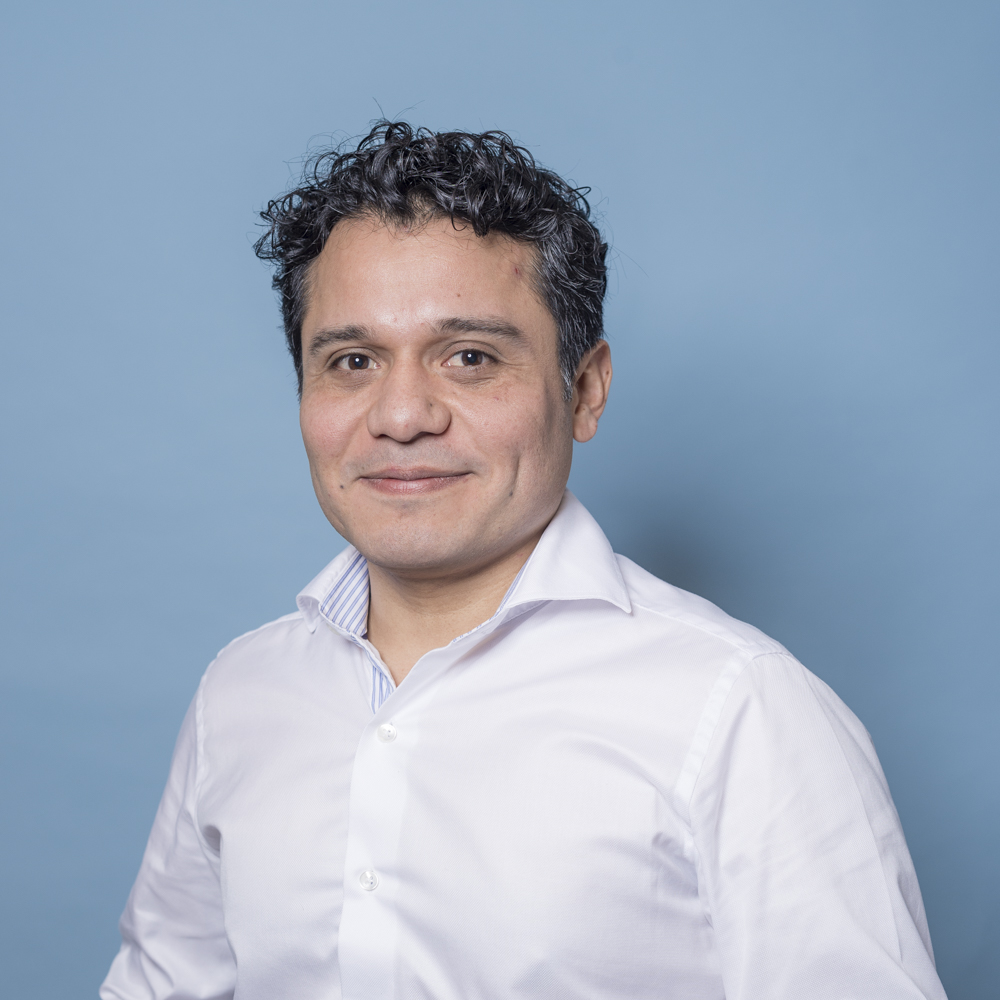
Our faculty consists of a vibrant and diverse community. Diversity is a catalyst that allows us to achieve broad knowledge, and a base upon we can drive scientific innovation and improve education.
Moreover, diversity is one of the core values of TUDelft and our faculty and comes with great responsibility. Without equality and inclusion diversity becomes an empty gesture. But realising a safe, equal and inclusive environment requires the participation of everyone in our community. It starts by having a dialog, stablish communication channels at different levels, and debunking taboos with respect to the visible and invisible differences among each other and our students. An empathic approach for this process can play a key role in realising this ambition.
In this colloquium Jorge talks about his experience within EDIT: EEMCS Diversity & Inclusion Team. Join us to know more about EDIT, and for an informal discussion on the current advancements on addressing issues like harassment, discrimination, and gender (in)equality. Or if you want to know what are the channels and means within our faculty and our University to reach for advice or help in case you encounter any issues related to these important topics.
Additional information ...
Signal Processing Seminar
- Thursday, 17 February 2022
- 13:30-14:30
- Online [zoom]
Sparsity-Based Vascular Ultrasound Imaging Through Compressive Spatial Coding
Didem Dogan Baskaya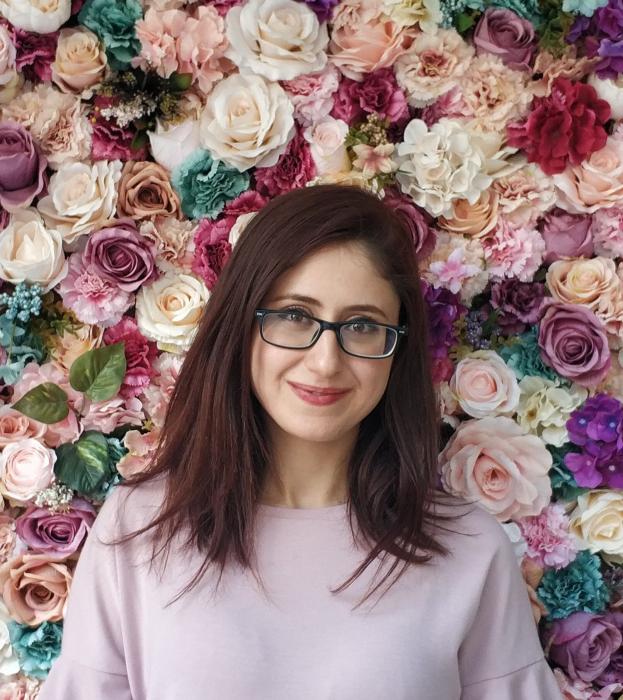
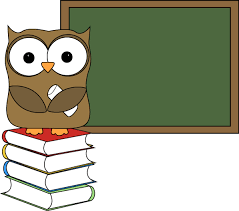
CAS MSc Kick-off Presentations
- Thursday, 17 February 2022
- 13:30-14:30
- Online [zoom]
Extreme Weather Forecasting Using Deep Generative Model
Haoran Bi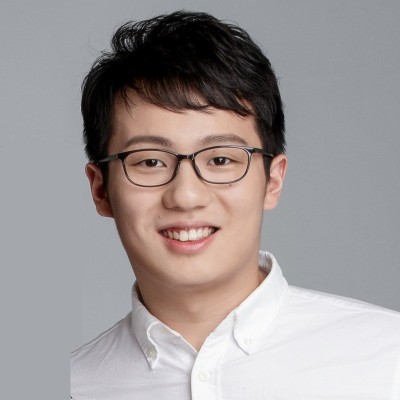
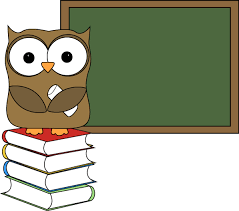
CAS MSc Kick-off Presentations
- Thursday, 17 February 2022
- 13:30-14:30
- Online [zoom]
Truncated Array Sound Field Synthesis
Michael Kraaijeveld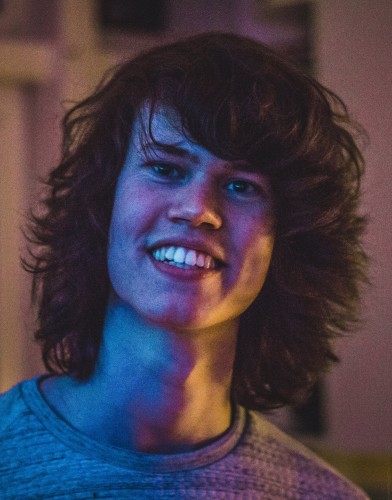
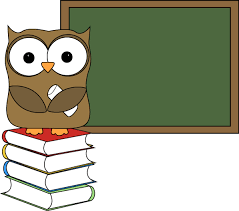
CAS MSc Midterm Presentations
- Thursday, 10 February 2022
- 13:30-14:30
- Online [zoom]
Estimation of Atrial Activity using Beamforming in Epicardial Electrograms
Tijs Moree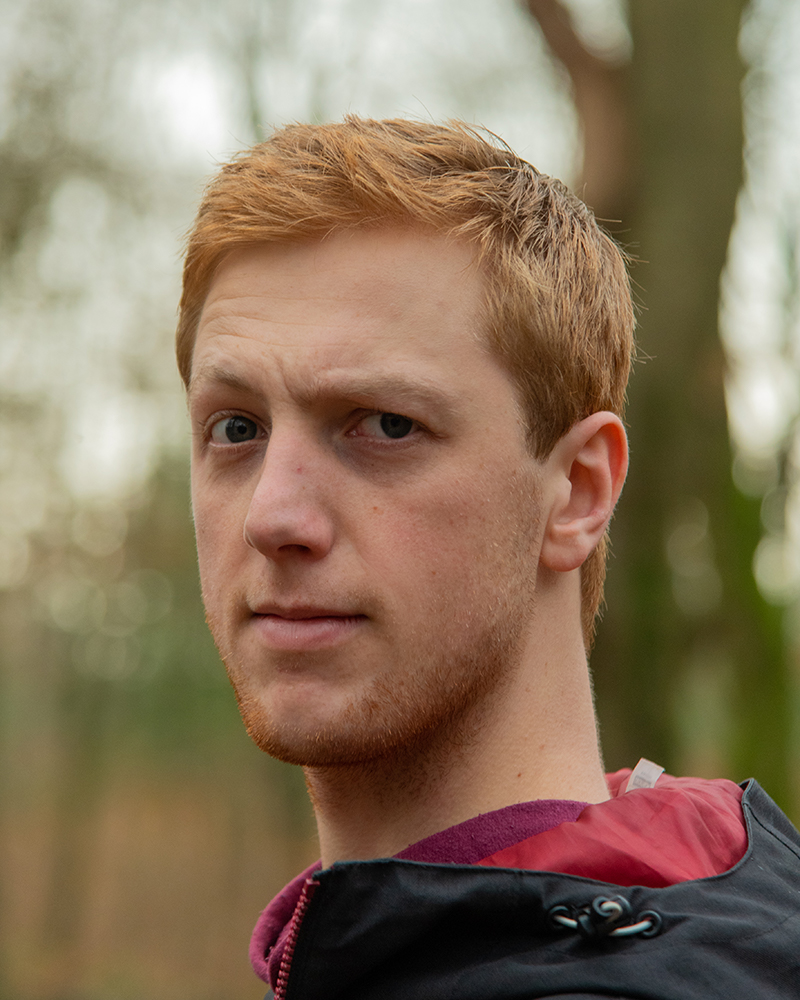
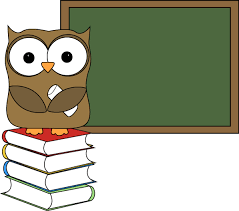
CAS MSc Kick-off Presentations
- Thursday, 10 February 2022
- 13:30-14:30
- Online [zoom]
Energy-Efficient Seizure Detection for Wearable EEG
Xiaoning Shi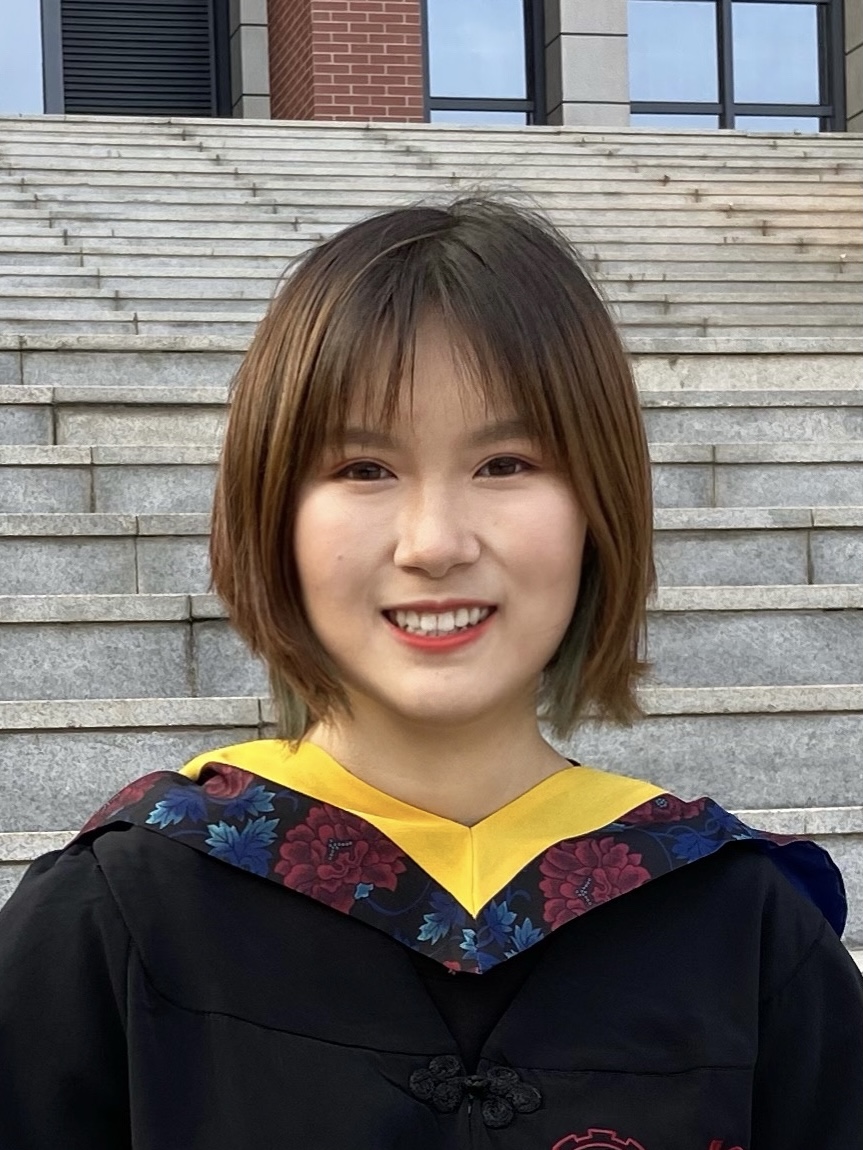
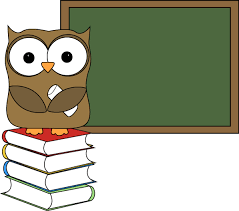
CAS MSc Kick-off Presentations
- Thursday, 10 February 2022
- 13:30-14:30
- Online [zoom]
Cooperative Localization of Drones using ADS-B Data
Xuzhou Yang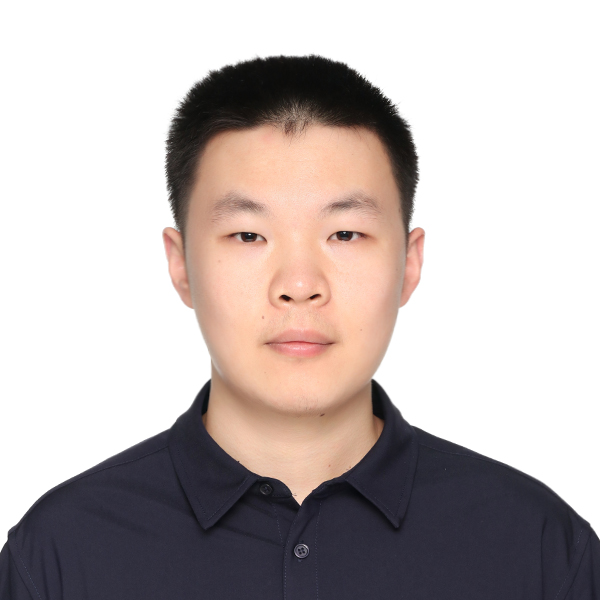
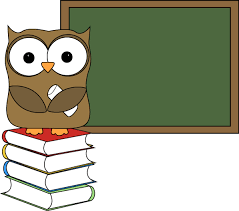
CAS MSc Kick-off Presentations
- Thursday, 10 February 2022
- 13:30-14:30
- Online [zoom]
Distributed Gaussian Process for Multi-Agents System
Peiyuan Zhai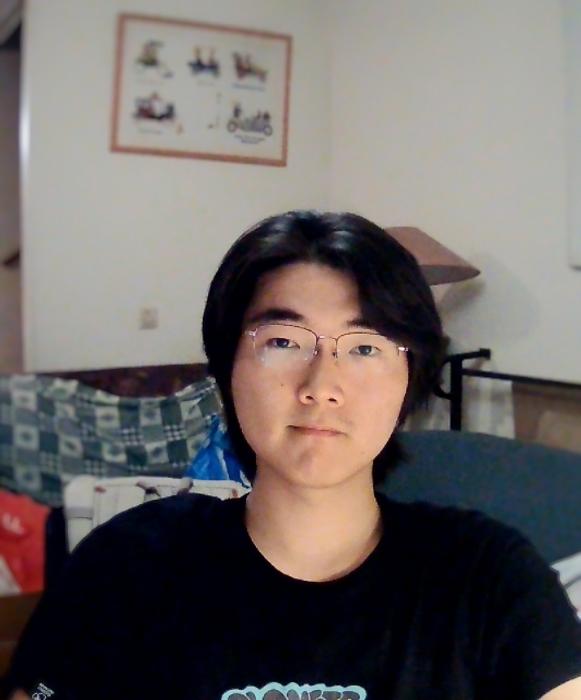
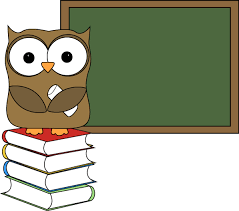
CAS MSc Kick-off Presentations
- Thursday, 27 January 2022
- 13:30-14:30
- Online [zoom]
Detecting Medical Instruments in Surgical Nets with Deep Learning
Renjie Dai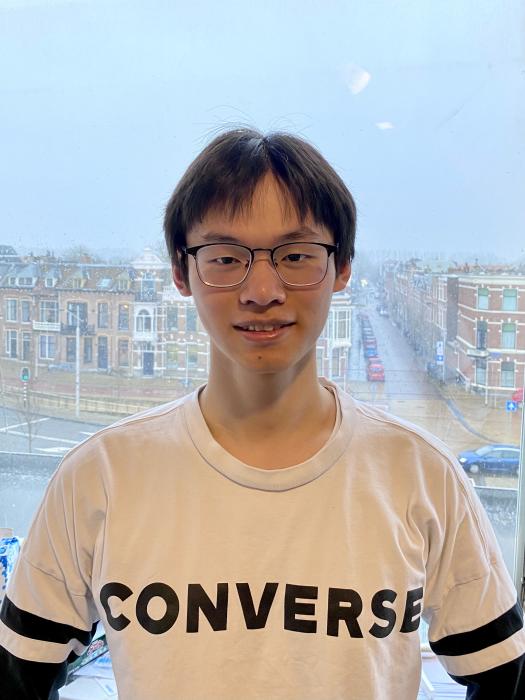
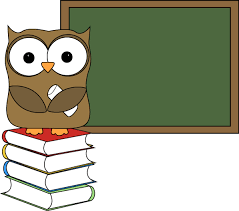
CAS MSc Kick-off Presentations
- Thursday, 27 January 2022
- 13:30-14:30
- Online [zoom]
Extreme-Value Deep Generative Models for Weather Forecasting
Maksym Kyryliuk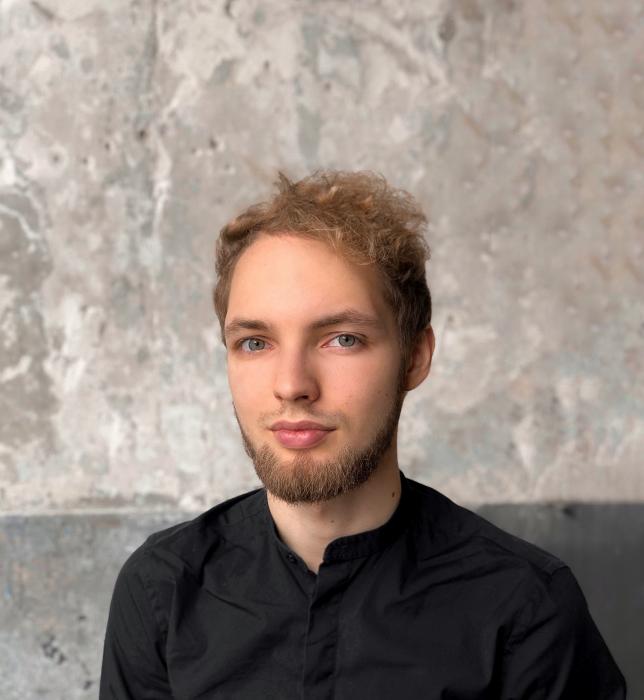
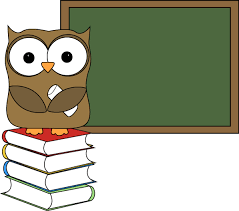
CAS MSc Kick-off Presentations
- Thursday, 27 January 2022
- 13:30-14:30
- Online [zoom]
Link Adaptation for Acoustic Underwater Communication Using Machine Learning
Mauries van Heteren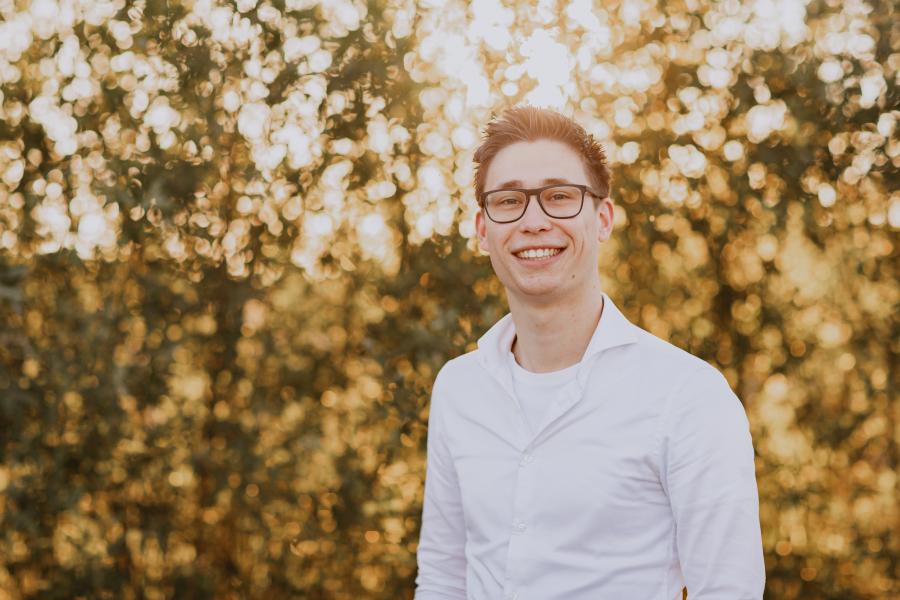
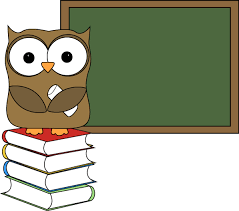
Signal Processing Seminar
- Thursday, 27 January 2022
- 13:30-14:30
- Online [zoom]
Introduction
Yanbo Wang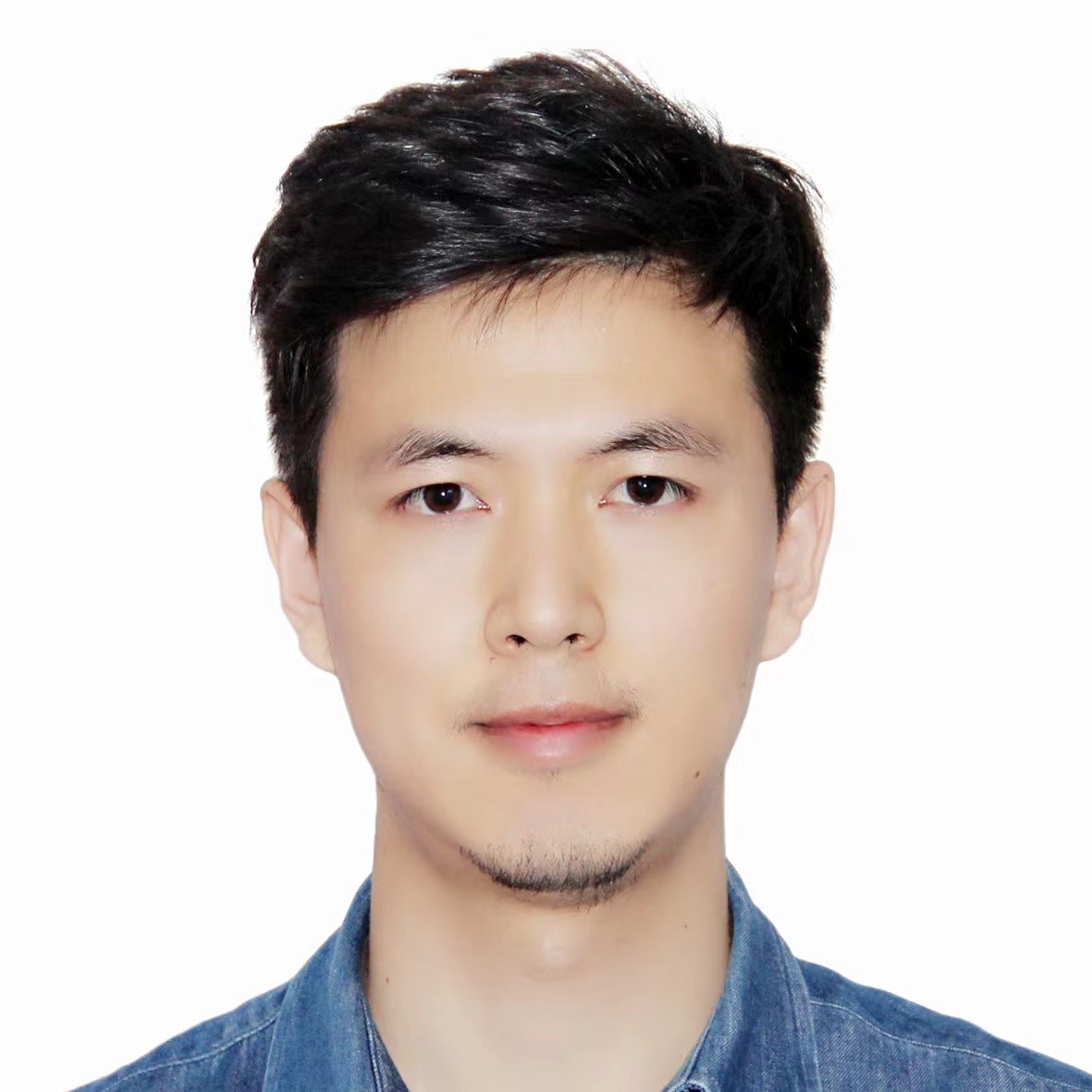
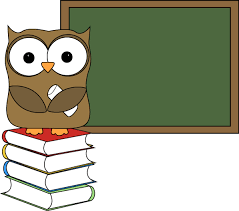
CAS MSc Kick-off Presentations
- Thursday, 20 January 2022
- 13:30-14:30
- Online [zoom]
Extreme-Value Neural Networks for Weather Forecasting
Zhiyi WANG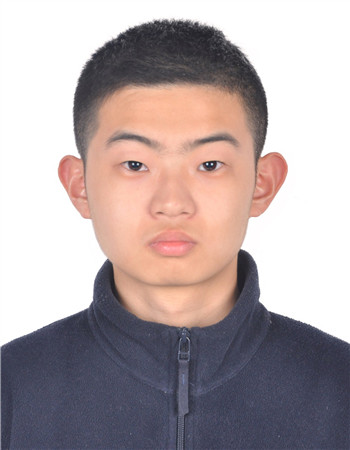
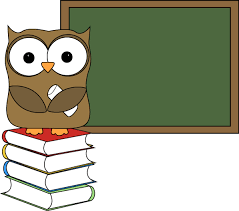
CAS MSc Kick-off Presentations
- Thursday, 20 January 2022
- 13:30-14:30
- Online [zoom]
Distributed Particle Filtering
Rui TANG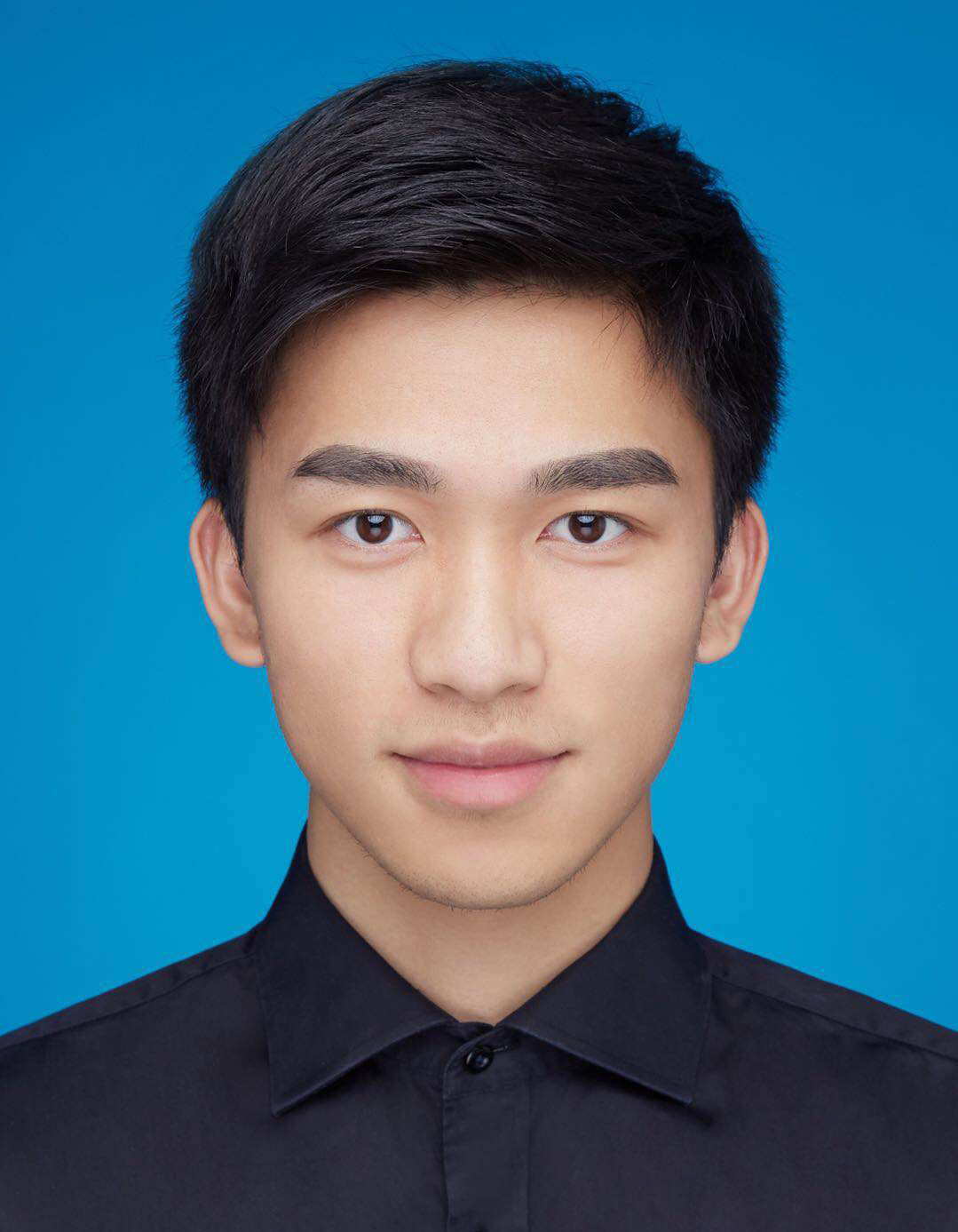
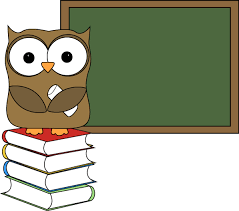
CAS MSc Midterm Presentations
- Thursday, 20 January 2022
- 13:30-14:30
- Online [zoom]
Towards Sustainable Satellite Swarms
Calum Turner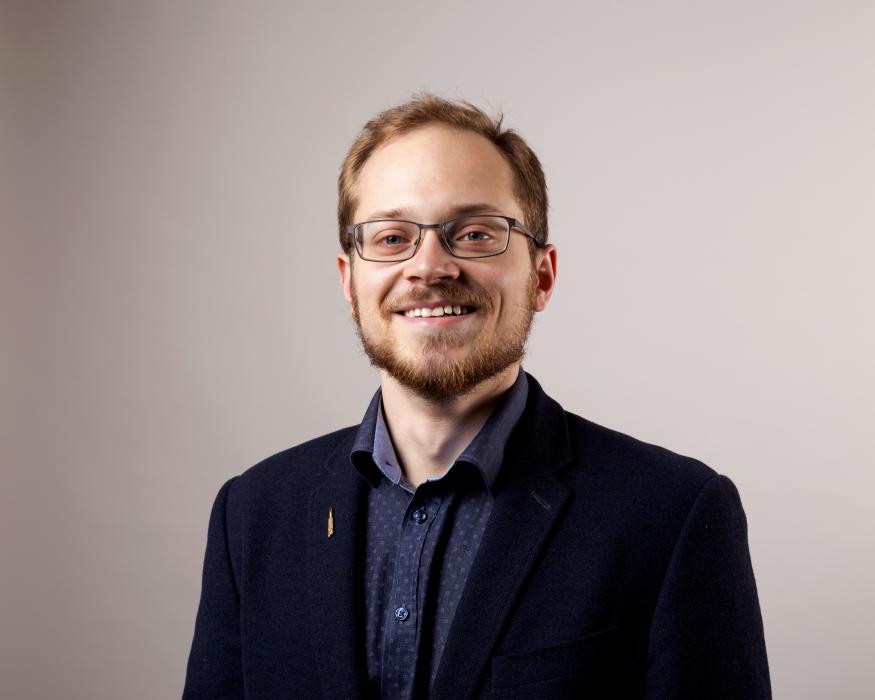
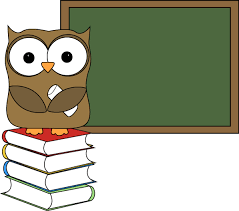
CAS MSc Midterm Presentations
- Thursday, 13 January 2022
- 13:30-14:30
- Online [zoom]
Towards Gridless Sound Field Reconstruction
Ids van der Werf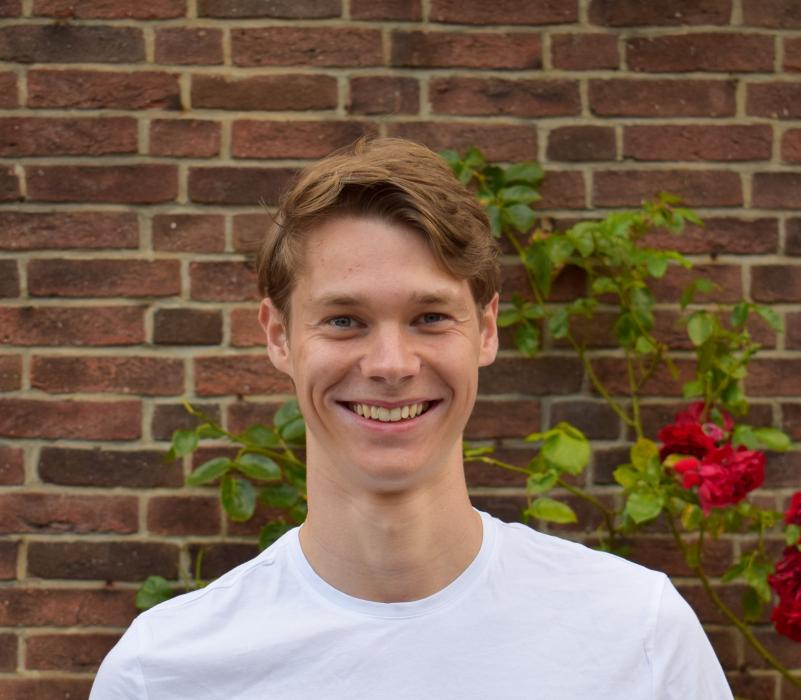
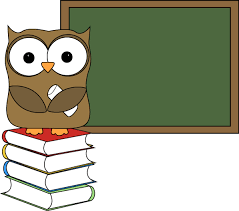
CAS MSc Kick-off Presentations
- Thursday, 13 January 2022
- 13:30-14:30
- Online [zoom]
3D Person Tracking in the Catheterization Laboratory
Yingfeng Jiang
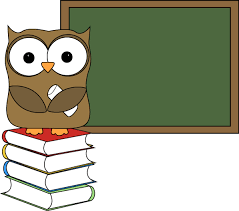
CAS MSc Kick-off Presentations
- Thursday, 13 January 2022
- 13:30-14:30
- Online [zoom]
Autonomous Landing of an UAV
Siddhy Ganesh Shetty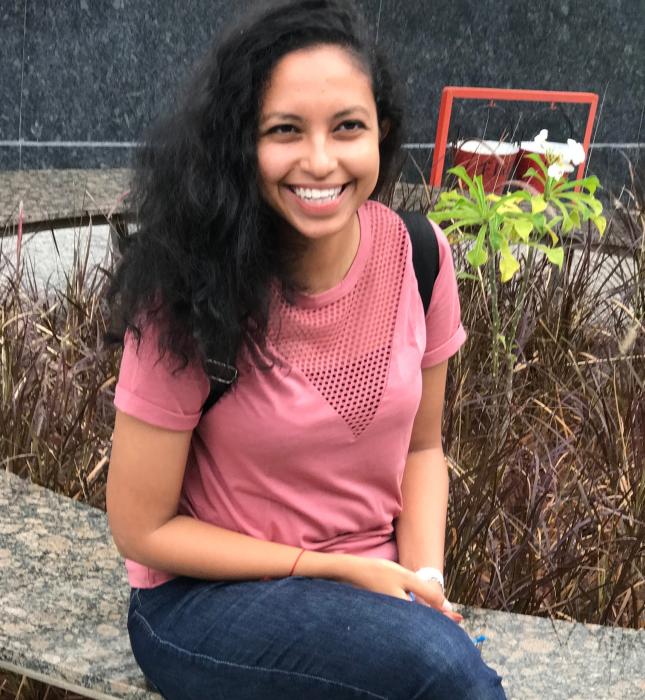
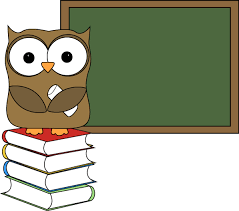
CAS MSc Kick-off Presentations
- Thursday, 13 January 2022
- 13:30-14:30
- Online [zoom]
Frequency Domain Joint Estimation of HRF and Stimulus from Functional Ultrasound Data
Yitong Tao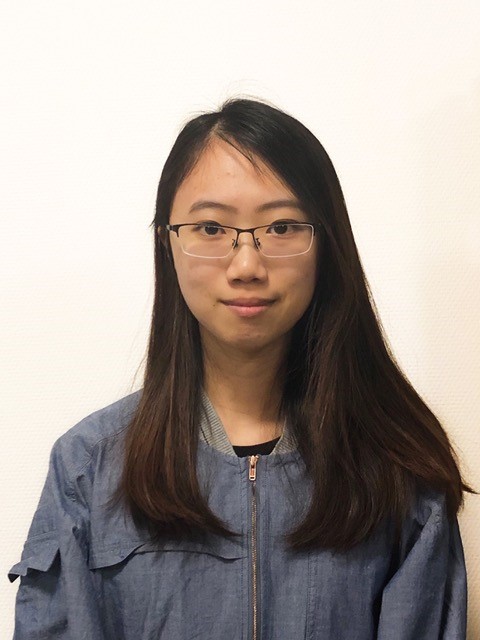
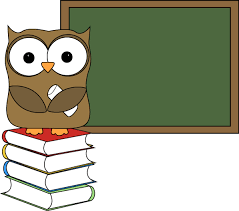
MSc SS Thesis Presentation
- Friday, 17 December 2021
- 09:30-10:15
A Decentralized Key Management System for the European Railway Signalling System
David Kester
MSc Biomedical Engineering Thesis Presentation
- Thursday, 16 December 2021
- 09:30-10:05
- zoom
An Expanded IPFM Model for Heart Rhythm Analysis
Arthur Kordes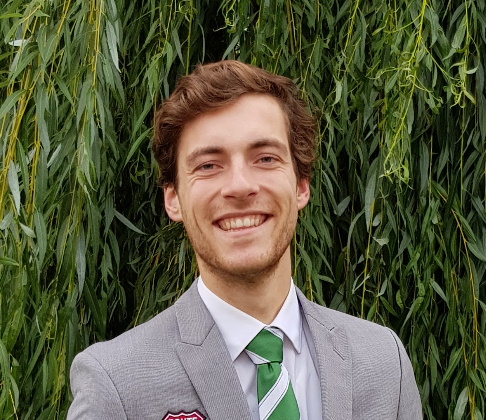
Atrial Fibrillation affects millions of people worldwide. It is associated with an impaired quality of life and an increased risk of stroke, cardiac failure and mortality. Treatments exist, but early detection and treatment is crucial, due to the progressive nature of the disease. Algorithms can help with early detection.
Machine learning algorithms are commonly trained to diagnose based on ECG data, but the interpretability is low. A physiological model that simulates the heart gives more insight into the situation of the patient. Current approaches, like the IPFM model, simulate only the SA node and generate RR intervals as output, while completely neglecting the interaction between the AV and SA node. By using an IPFM model and including the AV node as well, an extended and more accurate physiological model was built to more accurately detect Atrial Fibrillation. The AV node model is able to estimate PR intervals when the P waves are annotated. This result shows that the model extension is able to capture information about the signal conduction.
When the SA node model and the AV node model are cascaded and only the R peaks are considered, the classification accuracy does not improve compared to the SA node model alone. The R peaks alone do not contain sufficient information for accurate parameter estimation. The parameters governing the behavior of the AV node seem different for NSR compared to AF, but more data is needed to confirm this. The ability of the model to predict PR intervals gives hope that the inclusion of P wave data should improve the performance of the classification with the extended physiological model.
MSc CE Thesis Presentation
- Tuesday, 14 December 2021
- 14:00-14:35
- zoom
Neuromorphic computing application of emerging memory technologies for spiking neural networks
Jan Maarten Buis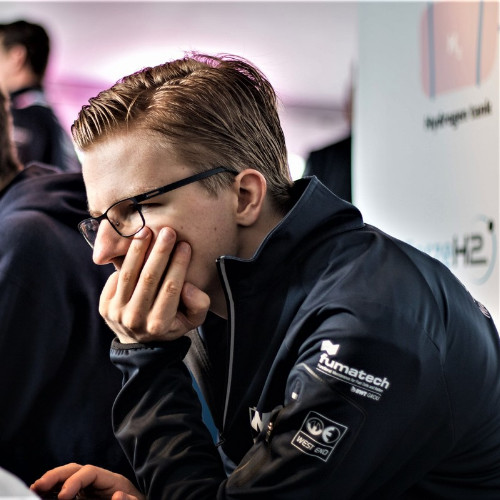
Renewed interest in memory technologies such as memristors and ferroelectric devices can provide opportunities for traditional and non-traditional computing systems alike. To make versatile, reprogrammable AI hardware possible, neuromorphic systems are in need of a low-power, non-volatile and analog memory solution to store the weights of the spiking neural network (SNN). In addition to being used for memory, memristive memory can be read out passively and thus also replaces digital-to-analog circuitry.
In this thesis, two solutions are proposed: one is based on a generalized memristor, the other is based on ferroelectric memory. Both solutions are implemented and simulated in SystemC AMS and tested with a spiking neural network (SNN). As a final test, both memory solutions are integrated into a full-sized SNN and simulated against the MNIST dataset. The simulation results validate the capabilities of memristive and ferroelectric memory when it comes to providing a sensible weight storage solution for neuromorphic systems.
Additional information ...
CAS MSc Kick-off Presentations
- Thursday, 9 December 2021
- 13:30-14:30
- Online [zoom]
Finding Camera Position and Orientation using Marker-Less Computer Vision in the Catheterization Laboratory
Jinchen ZENG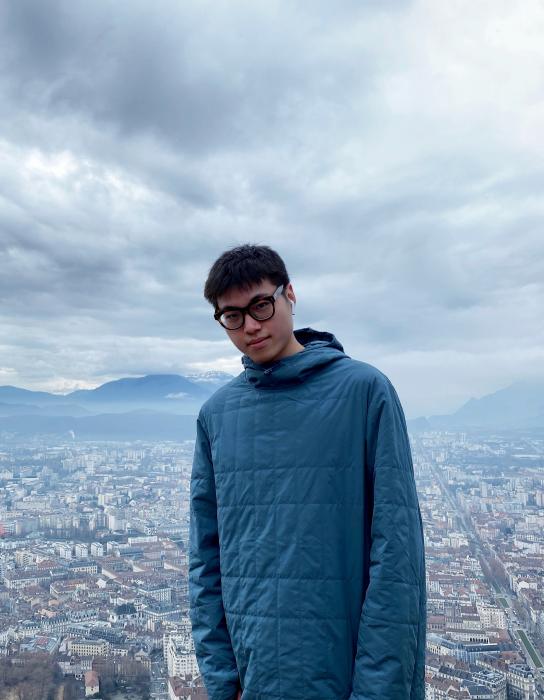
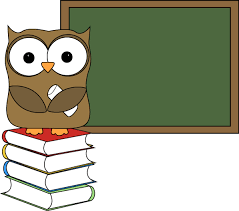
Signal Processing Seminar
- Thursday, 9 December 2021
- 13:30-14:30
- Online [zoom]
Nonlinear and Time-Varying Aspects of the Functional Ultrasound Response
Aybüke Erol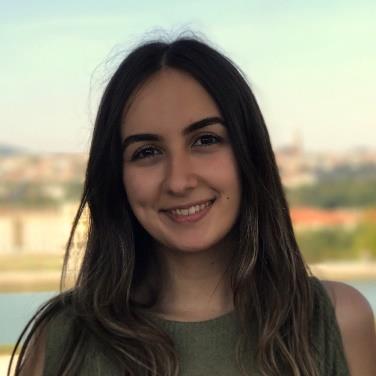
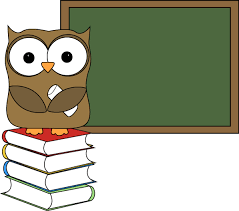
CAS MSc Kick-off Presentations
- Thursday, 9 December 2021
- 13:30-14:30
- Online [zoom]
The Impact of Jamming and Spoofing on GNSS Signals
Pim Jansen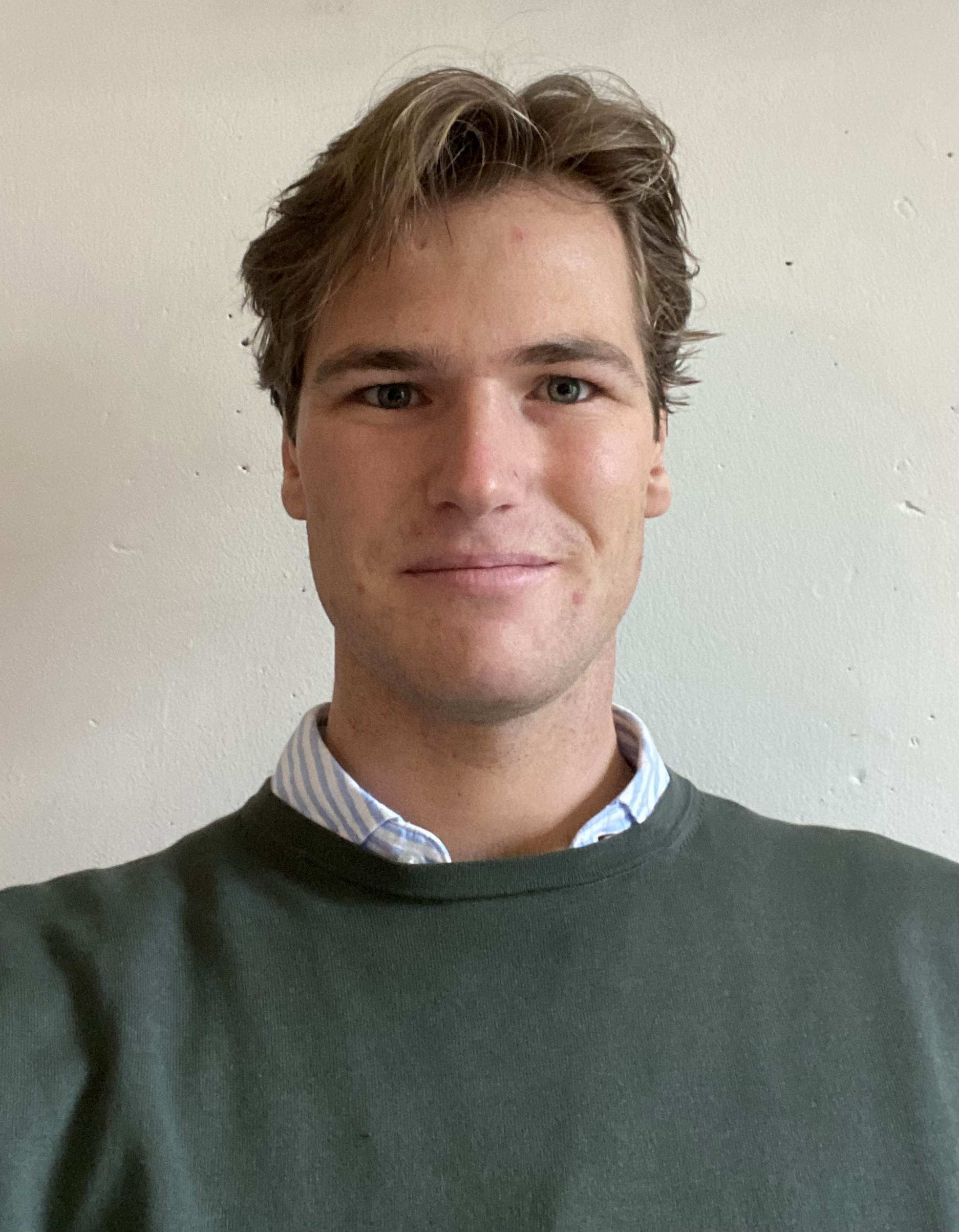
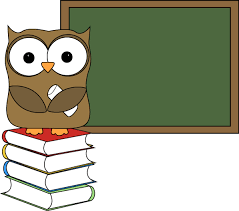
CAS MSc Kick-off Presentations
- Thursday, 9 December 2021
- 13:30-14:30
- Online [zoom]
An image processing solution to building inspection
Chuhan WANG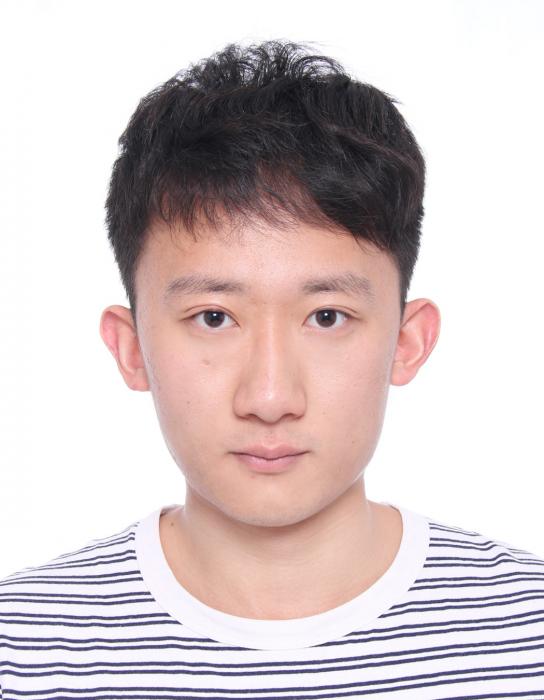
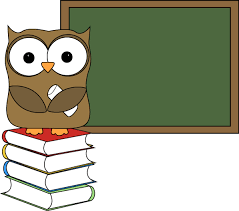
MSc SS Thesis Presentation
- Friday, 3 December 2021
- 15:00-15:35
Analyzing Functional Ultrasound Images of the Brain Using Tensor Decompositions
Arda Kaygan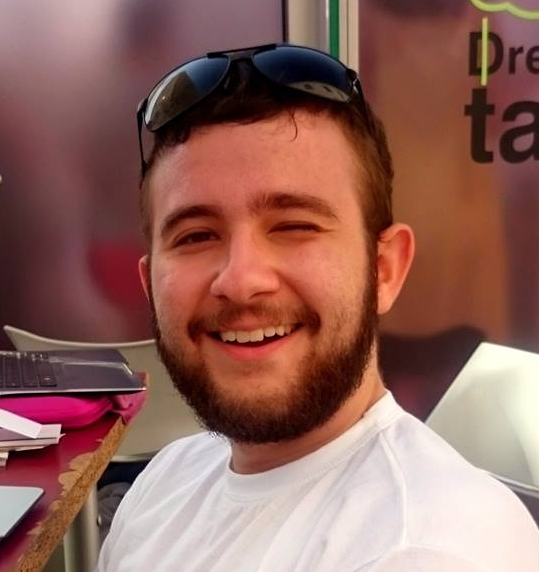
Functional ultrasound (fUS) is a neuroimaging modality that offers high spatial and temporal resolution while also providing portability. In this thesis, neuroimaging data acquired with fUS at Center for Ultrasound and Brain imaging at Erasmus MC (CUBE) is processed. Due to the fact that fUS data is inherently multidimensional, we propose using tensor decompositions, tensors here referring to generalizations of matrices, for processing of fUS data.
We define two main research questions regarding fUS data analysis. First, for compressing the large-scale raw beamformed fUS data, we apply sequentially truncated multilinear singular value decomposition. This compression method is compared against ensemble averaging used in the conventional pipeline, and shown to provide a higher compression rate while preserving more temporal resolution for specific ranks. Furthermore, it is observed to denoise the data, resulting in a more precise extraction of the active region of Superior Colliculus using correlation maps.
Secondly, in order to investigate the advantage of multi-slice processing that incorporates 3-D information, blind-source separation methods are applied to single slice and two-slice fUS recordings. After applying independent component analysis (ICA) to the matricized data as a benchmark method, block termdecomposition (BTD) is used as a way of processing the data as it is, in its natural 3-D structure without vectorization. Through a simulation study, it is shown that the method is able to separate two images even when using a rank that is lower than the true rank, as well as in noisy conditions. Subsequently, BTD is applied to real 4-D fUS data formed by concatenation of slices in a new dimension. However, this method is seen to perform worse than single slice ICA in terms of extracting the active regions. In order to amplify common information between slices, a new 3-D data structure is then formed by summing the fUS data of two slices. For extraction of this common information, a BTD is then applied to the aggregate 3-D data. The findings of this decomposition reveal that both taking a longer portion of single slice data and incorporating the second slice helps to achieve better results.
Additional information ...
CAS MSc Kick-off Presentations
- Thursday, 2 December 2021
- 13:30-14:30
- Online [zoom]
Developing a Data-Driven Model to Predict to the Noise Attenuation Level in Earplugs
Stephy Annie Curie Rakesh Arya
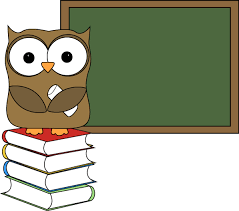
Signal Processing Seminar
- Thursday, 2 December 2021
- 13:30-14:30
- Online [zoom]
Faster-Than-Nyquist Signaling Short-Packet Communication
Mostafa Mohammadkarimi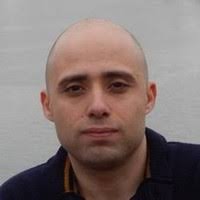
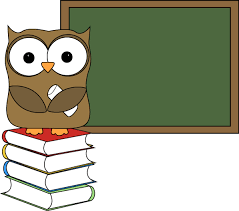
CAS MSc Kick-off Presentations
- Thursday, 2 December 2021
- 13:30-14:30
- Online [zoom]
Sparse Recurrent Independent Mechanisms for Vision Tasks
Nan Lin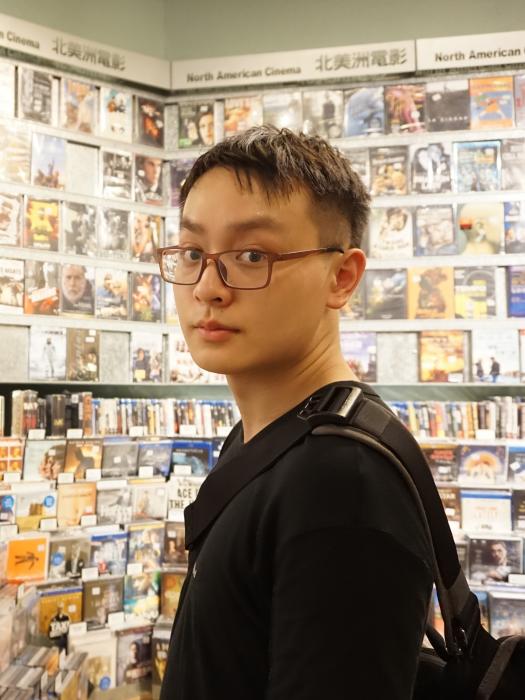
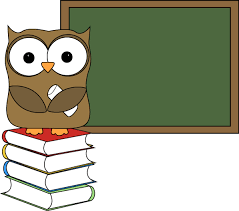
MSc CE Thesis Presentation
- Tuesday, 30 November 2021
- 14:00-14:35
- Building 33 Pulse A0.400 Technology
Spiking CA-CFAR Implementation for Radar Target Detection
Bastiaan van Otterloo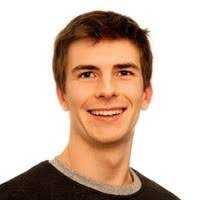
Radar systems have been used for decades to detect targets on the ground and in the air. The radar signal is transformed into a rangedoppler image that distinguishes each detected object by range and velocity to process radar data. A target detection algorithm is used to filter noise and unwanted reflections. Each target can be in a region with different noise levels; a simple threshold will yield false positives or miss detections depending on its value. To solve this problem, a Constant False Alarm Rate or CFAR is desirable.
A CFAR detector estimates the noise surrounding each target and has a dynamic threshold based on this. Spiking Neural Networks are the third generation of Artificial Neural Networks where, instead of continuous signals, the input is encoded into trains of spikes over time. These networks have a potentially efficient hardware implementation instead of the older generation Artificial Neural Networks and could run directly at the sensor edge, lowering latency and power consumption.
This thesis will explore a Spiking Cell Averaging CFAR implementation and attempt to use its desirable properties like a temporal average over multiple radar frames, mimicking the non-coherent integration sometimes done in radar processing. It is shown that some configurations will behave similarly in a simulated environment with additive white Gaussian noise.
MSc SS Thesis Presentation
- Tuesday, 30 November 2021
- 09:00-09:35
- zoom
Investigation of focal epilepsy using graph signal processing
Gaia Zin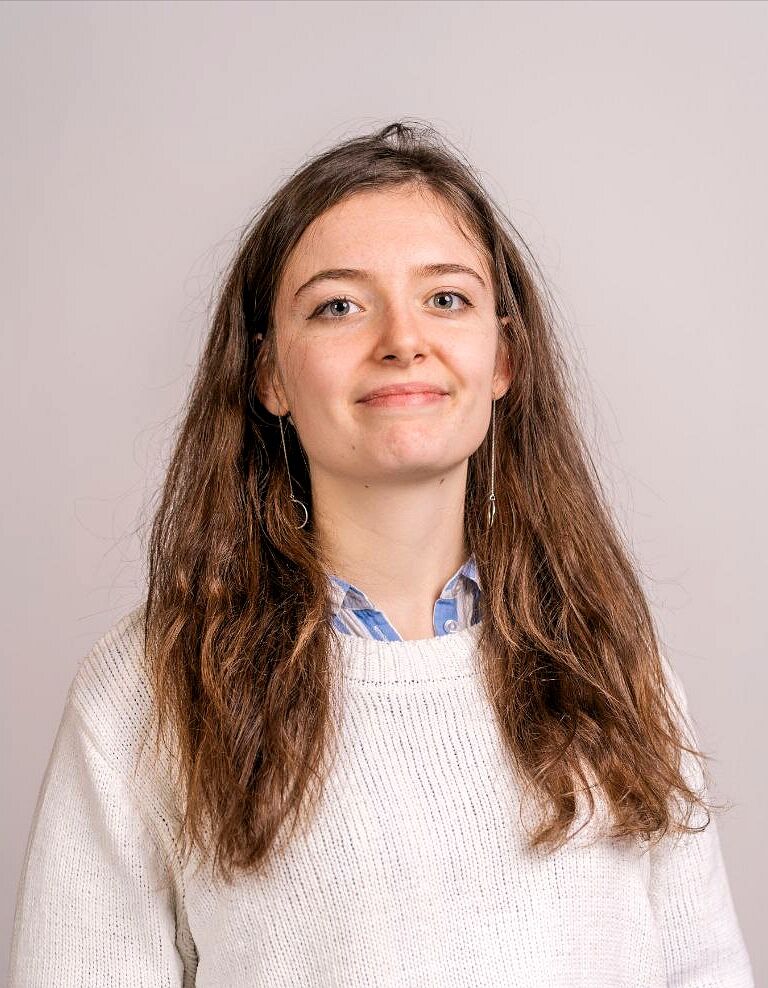
Epilepsy is one of the most common neurological disorders worldwide. Its manifestations, the seizures, are due to a group of neurons’ abnormal and synchronous activity. The unpredictable nature of these events hinders the quality of life of those affected. In particular, focal seizures show a localized onset of abnormal activity and are the most common ones. Correct detection of the episodes can help clinicians to give the best medical treatments. This research project arises from the need to have automatic algorithms for seizure detection with a high number of correctly detected seizures for low false alarm rates.
Recent studies have shown disorganization in how brain areas interact with each other before and during a seizure. We decided to model this change in connectivity patterns by inferring graphs from EEG recordings of epileptic patients. We work with seventeen subjects suffering from focal epilepsy, and we build, for each of them, a graph of the activity preceding (preictal) and during (ictal) a seizure. After that, we exploit techniques from graph signal processing to build a detector for seizures. Last, we analyze the density of connections of the inferred graphs to indicate the seizure onsets.
The obtained results are unsuitable for real-life applications, but they are a starting point for further research. Furthermore, we find that most the proposed ictal or preictal graphs show fewer connections in the nodes involved with the seizure onset.
Additional information ...
MSc CE Thesis Presentation
- Monday, 29 November 2021
- 10:00-10:35
- zoom
Hardware Spiking Neural Network based Sbox AES
Hanyu Ma
Hardware cryptographic algorithm implementation is easy to attack by side-channel attacks. The power-based side-channel attacks are powerful among several side-channel attacks. This attack methods use the relationship between the leakage model and power traces to reveal the secret key. Some existing countermeasures like mask and hide can protect the algorithms from attacking. However, they can not break the relationship between power traces and the leakage model. Based on the property of the neural network, the linear relationship can be easily broken. Furthermore, the spiking neural network is more hardware-friendly than a conventional neural network. The design replaces the sbox in AES with a pipeline spiking neural network-based sbox and implements it in hardware. The help of the FPGA attack platform demonstrates that the proposed design can resist DPA, CPA, Template Attacks, and Deep Learning-based attacks.
CAS MSc Kick-off Presentations
- Thursday, 25 November 2021
- 13:30-14:30
- Online [zoom]
Robust Formation Maneuvering of Multi-agent Systems
Zhonggang LI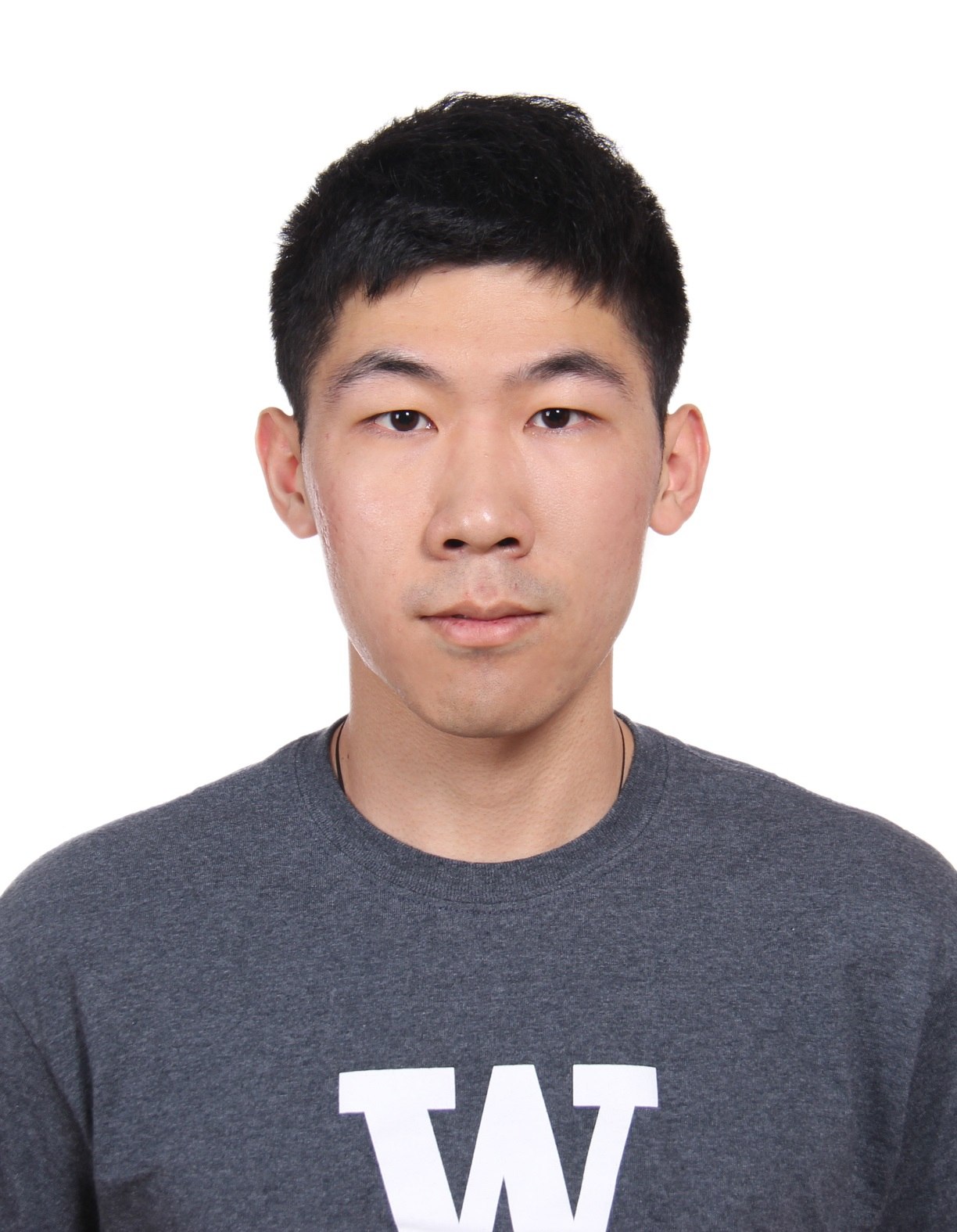
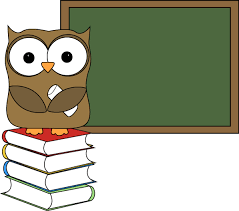
CAS MSc Kick-off Presentations
- Thursday, 25 November 2021
- 13:30-14:30
- Online [zoom]
Search by Image: A Digital History Search Engine in Online Image Repositories
Yanan HU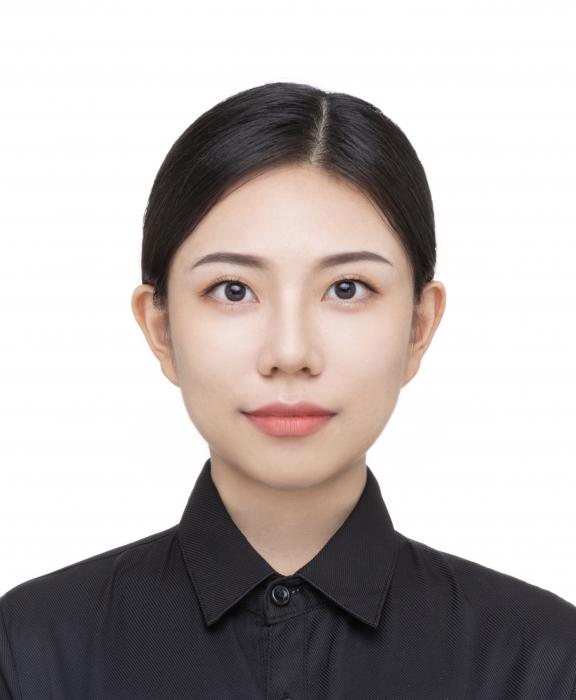
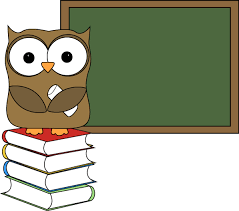
CAS MSc Kick-off Presentations
- Thursday, 25 November 2021
- 13:30-14:30
- Online [zoom]
Crack Detection for Building Inspection Using UAV with Image Alignment
Enpu CHEN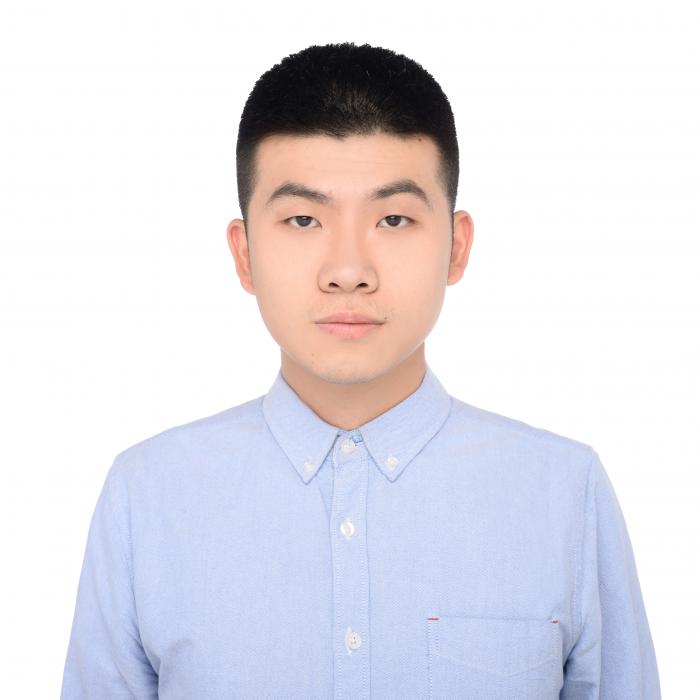
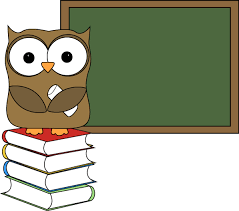
CAS MSc Kick-off Presentations
- Thursday, 11 November 2021
- 13:30-14:30
- Online [zoom]
Analyzing Dynamic Functional Connectivity Using State-Space Models
Ruben Wijnands
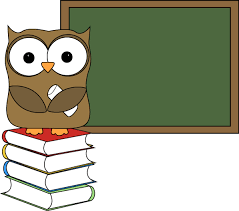
Signal Processing Seminar
- Thursday, 11 November 2021
- 13:30-14:30
- Online [zoom]
Low Complex Accurate Multi-Source RTF Estimation
Changheng Li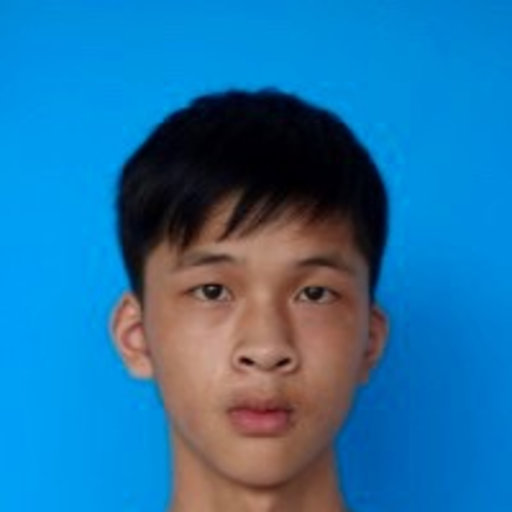
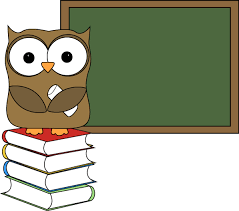
CAS MSc Midterm Presentations
- Thursday, 28 October 2021
- 11:00-12:00
- Online [zoom]
Frequency Dependence of the Impulse response in an Ultra-wideband Channel
Xiaoyao Luo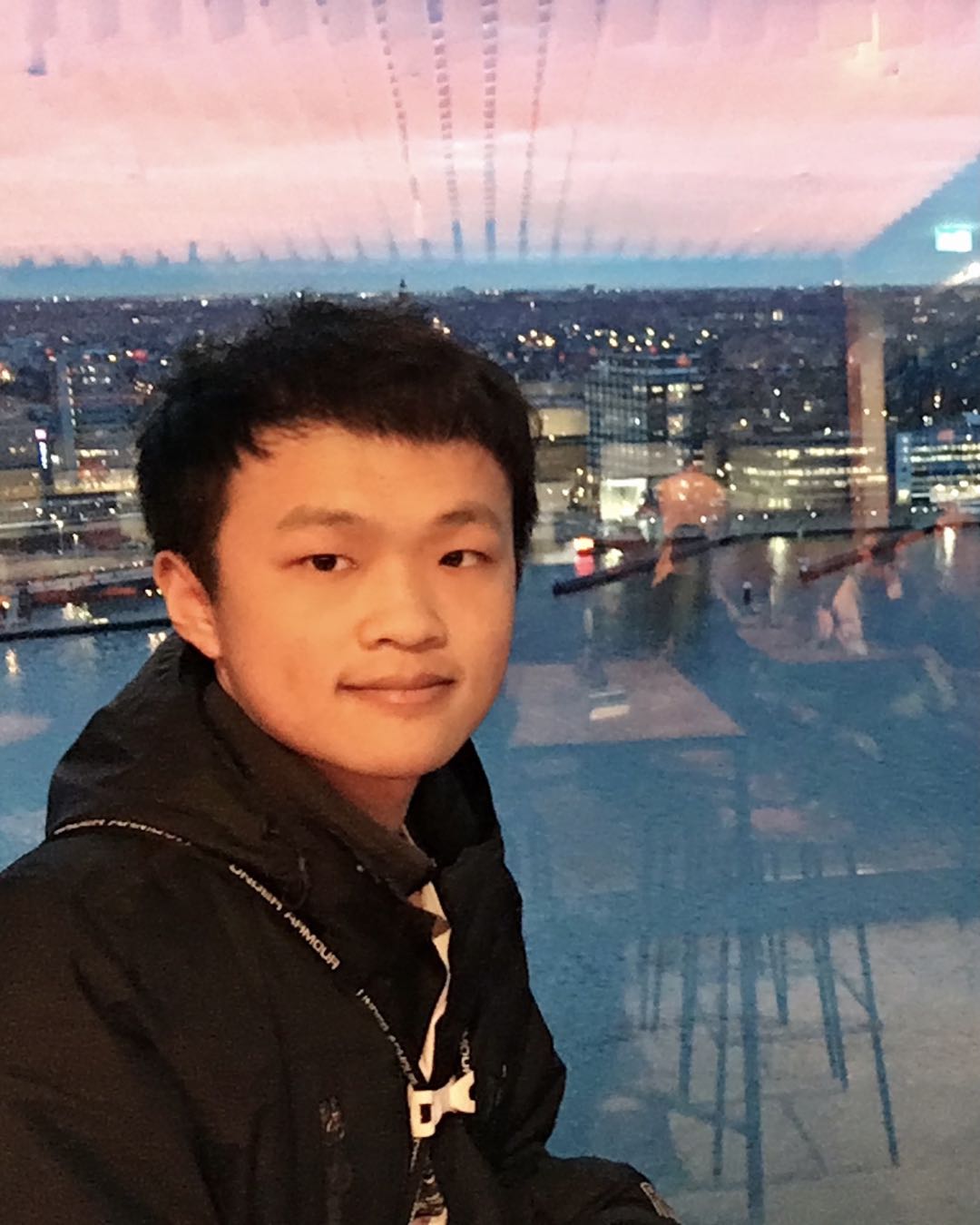
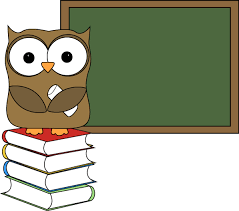
CAS MSc Kick-off Presentations
- Thursday, 28 October 2021
- 11:00-12:00
- Online [zoom]
Sound Field Recovery by Estimating Room Modes Off-the-Grid
Ids van der Werf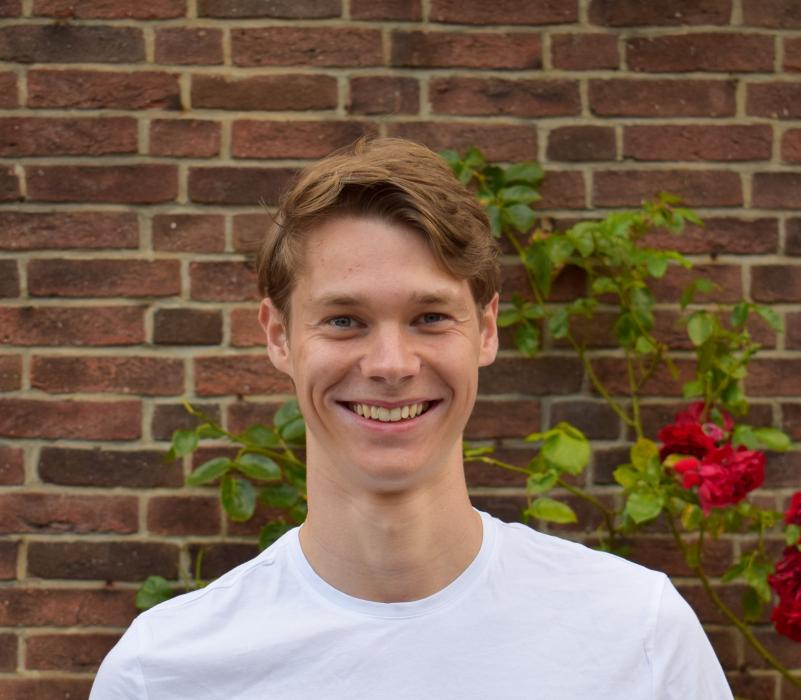
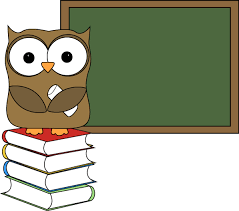
CAS MSc Kick-off Presentations
- Thursday, 28 October 2021
- 11:00-12:00
- Online [zoom]
Image Search Engine Based on Deep Learning for Digital History
Yuanyuan YaoAdditional information ...
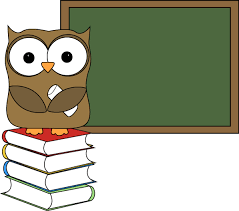
Microelectronics Colloquium
- Thursday, 28 October 2021
- 15:30-16:30
- Microsoft Teams Meeting
On my personal journey into artificial intelligence
Justin Dauwels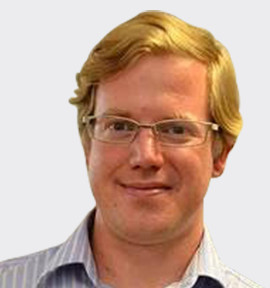
In this presentation, I will start with a brief introduction to artificial intelligence (AI). I will then elaborate on two types of AI approaches that our research team is investigating: graphical models and neural networks. Next I will summarize some of the main research results of our group. I will review some of the applications of AI that we have been working on over the years, and will present some of our future research plans. I will also say a few words about the spin-off companies that have emerged from our research group. At last, I will conclude with a few thoughts on the potential impact of AI on society and will formulate a few important open research questions in the field of AI.
Additional information ...
PhD Thesis Defence
- Wednesday, 27 October 2021
- 12:01-13:00
- Senaatszaal of Aula Building
Atrial Fibrillation Fingerprinting
Bahareh Abdi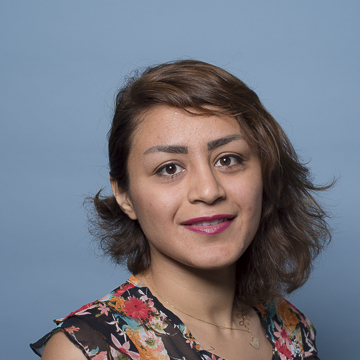
Atrial fibrillation (AF) is a common age-related cardiac arrhythmia. AF is characterized by rapid and irregular electrical activity of the heart leading to a higher risk of stroke and heart failure. During AF, the upper chambers of the heart, called atria, experience chaotic electrical wave propagation. However, despite the various mechanisms introduced in the literature, there is still an ongoing debate on a precise and consistent mechanism underlying the initiation and perpetuation of AF. Some studies show that AF is rooted in impaired electrical conduction and structural damage of atrial tissue, known as electropathology. Atrial electrograms (EGMs) recorded directly from heart’s surface, provide an important diagnostic tool to localize and quantify the degree of electropathology in the tissue. However, the analysis of the electrograms is currently constrained by the lack of suitable methods that can reveal the hidden electrophysiological parameters of the tissue. These parameters can be used as local indication of electropathology in the tissue. We believe that understanding AF and improving AF therapy starts with developing a proper forward model that is accurate enough (from a physiological point of view) and simultaneously simple enough to allow for subsequent parameter estimation. Therefore, the main focus of this thesis is on developing a simplified forward model that can efficiently explain the observed EGM based on AF relevant tissue parameters. An initial step before performing any analysis on the data is to remove noise and artefacts. All atrial electrogram recordings suffer from strong far-field ventricular activities (VA). Therefore, as the first step, we propose a new framework for removal of VA from atrial electrograms, which is based on interpolation and subtraction followed by low-rank and sparse matrix decomposition. The proposed framework is of low complexity, does not require high resolution multi-channel recordings, or a calibration step for each individual patient. In the next step, we develop a simplified electrogram model. We represent the model in a compact matrix form and show its linear dependence on the conductivity vector, enabling the estimation of this parameter from the recorded electrograms. The results show that despite the low resolution and all simplifying assumptions, the model can efficiently estimate the conductivity map and regenerate realistic electrograms, especially during sinus rhythm. In the next contribution of this dissertation, we propose a new approach for a better estimation of local activation times for atrial mapping by reducing the spatial blurring effect that is inherent to electrogram recordings using deconvolution. Employing sparsity based regularization and first-order time derivatives in formulating the deconvolution problem, improved performance of transmembrane current estimation is obtained. In the final part, we focus on translating our findings from research to clinical application. Therefore, we studied the effect of electrode size on electrogram properties including the length of the block line observed on the resulting activation map, percentage of observed low voltage areas, percentage of electrograms with low maximum steepness, and the number of deflections in the recorded electrograms.
Additional information ...
MSc SS Thesis Presentation
- Tuesday, 26 October 2021
- 14:00-16:00
- ZOOM
Temporal Synchronization of Sensors
Tanmay Manjunath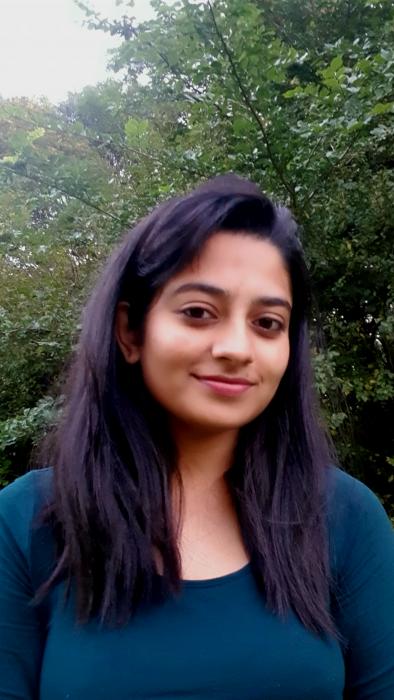
Advanced automotive vehicles are based on the real-time fusion of an increasing number of automotive sensors. For precise fusion of different sensors, measurements need to be synchronized both temporally and spatially. This thesis aims to design a hardware temporal synchronization block as part of the PRISTINE systolic array accelerator project for multi-sensor data fusion. In this process, we study and address several temporal sensor synchronization issues that are characteristic of the considered system as well as any other typical sensor fusion system. First and foremost, we handle the problem of estimating the actual time of sensor measurement by exploring well-known filtering techniques such as Kalman, mean and median filters. A suitable filter is selected for implementation based on the statistical characteristics of the observed sensor cycle times, the complexity of the filters and the quality of obtained estimates. Next, we address the issue of reconstructing incoming sensor data streams according to the estimated sensor measurement times while maintaining minimal latency and synchronization error by employing an adaptive stream buffering technique utilized in distributed multimedia systems.
An analysis of the effects of the stream synchronization algorithm’s parameters on buffering latency and synchronization error was presented.
Finally, the above synchronization solution was efficiently implemented on hardware by making certain modifications and design decisions to the algorithm. A method to evaluate the whole temporal synchronization process is proposed and the obtained results on real sensor data are presented.
https://tudelft.zoom.us/j/99844909465?pwd=OEtwbCtuS2xzdTg2Sk5lQzNOdVp2Zz09
Meeting ID: 998 4490 9465
Passcode: 375074
MSc SS Thesis Presentation
- Friday, 22 October 2021
- 10:00-12:00
- EWI building 36, lecture hall H
Adaptive Graph Partition Methods for Structured Graphs
Yanbin He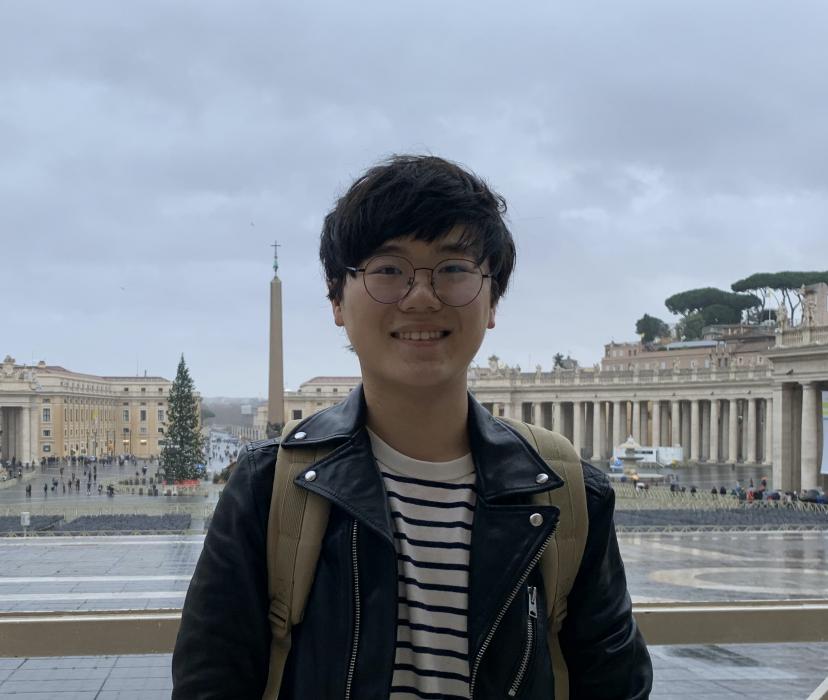
Graphs can be models for many real-world systems, where nodes indicate the entities and edges indicate the pairwise connections in between. In various cases, it is important to detect informative subsets of nodes such that the nodes within the subsets are 'closer' to each other. For example, in a cellular network, determining appropriate node subsets can reduce the operation costs. A subset is usually called a cluster. This leads to the graph clustering problem. Furthermore, plenty of systems in the real world are changing over time, and consequently, graphs as models vary with time as well. It is thus also important to update the clusters when the graph changes.
In this thesis work, we studied two problems from the cellular network background. We needed to partition graphs that have certain structures and cluster their nodes to minimize certain cost functions. In the first problem, we partitioned a bipartite graph by minimizing the so-called MinMaxCut cost function, while in the second problem, we partitioned a structured graph by minimizing the so-called Modified-MinMaxCut cost function. The structural property of the graph is incorporated in defining this new cost function. The solutions we proposed are under the framework of spectral clustering, where one relies on the eigenvectors of the graph matrices, e.g., the Laplacian matrix or the adjacency matrix, and any clustering algorithm, e.g., K-means, to partition nodes into disjoint clusters.
Furthermore, for the time-variant graph, we decomposed the problem into two steps. First, we transformed the variations in the graph topology into perturbations to the graph matrices. Then we transformed the update of the clusters into an update of the (generalized) eigenvectors of these graph matrices. We utilized matrix perturbation theory to update the generalized eigenvectors and then update the clusters. Our simulations showed that on synthetic data, the proposed method can efficiently track the eigenvectors and the clusters generated by the updated eigenvectors have almost the same cost function value as that of exact computation.
https://tudelft.zoom.us/j/95884057579?pwd=YXkzY0lCRVZuRmsvTzl3NW9xRzZ3QT09
CAS MSc Midterm Presentations
- Thursday, 21 October 2021
- 11:00-12:00
- Online [zoom]
Few-Shot Learning Using Speech and Physiological Signals for Emotion Recognition for Intelligent Voice Assistants
Mihir Kapadia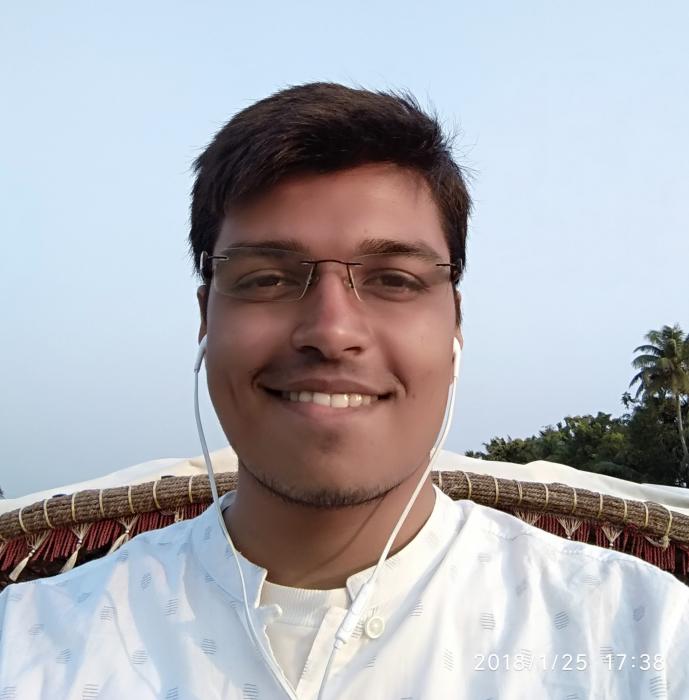
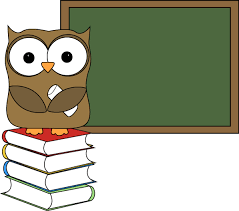
CAS MSc Kick-off Presentations
- Thursday, 21 October 2021
- 11:00-12:00
- Online [zoom]
Improving the Accuracy of the Image Search Engine for Digital History
Qi Zhang
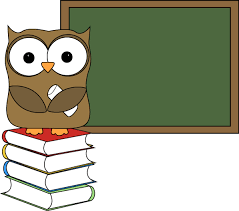
Signal Processing Seminar
- Thursday, 14 October 2021
- 11:00-12:00
- Online [zoom]
Finite Impulse Response Simplicial Filters
Maosheng Yang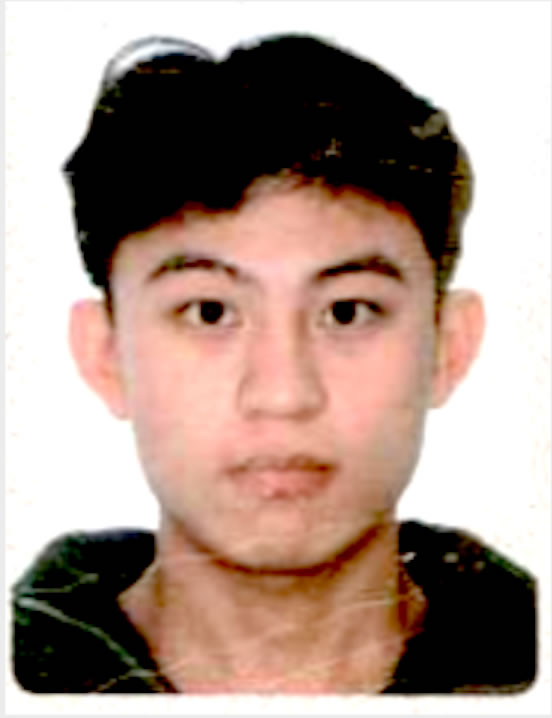
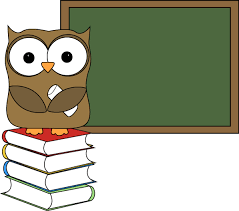
CAS MSc Kick-off Presentations
- Thursday, 14 October 2021
- 11:00-12:00
- Online [zoom]
Improving the Estimation of Local Activation Time in Atrial Electrograms
William Hunter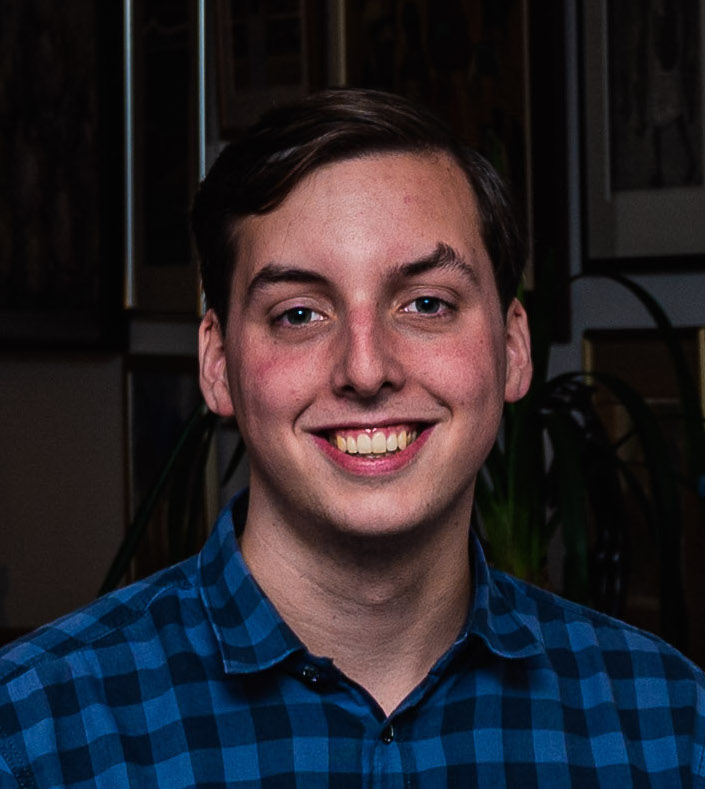
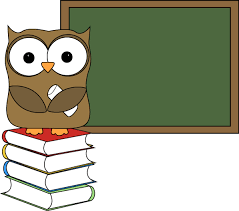
CAS MSc Kick-off Presentations
- Thursday, 14 October 2021
- 11:00-12:00
- Online [zoom]
Removing Far-Field Artifacts from Atrial Electrograms Using Temporal and Spatial Information
Tijs Moree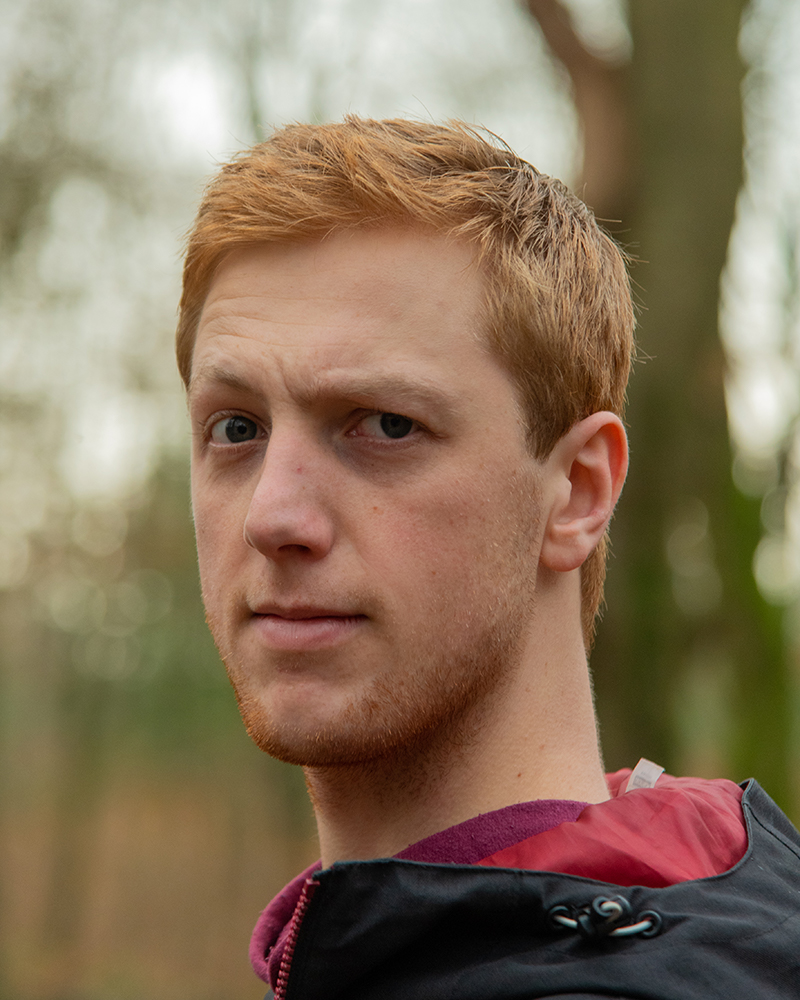
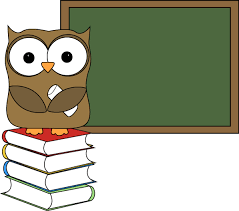
MSc SS Thesis Presentation
- Tuesday, 12 October 2021
- 14:00-17:00
- EWI Lecture Hall M
Targetless Camera-LiDAR Calibration for Autonomous Systems
Bichi Zhang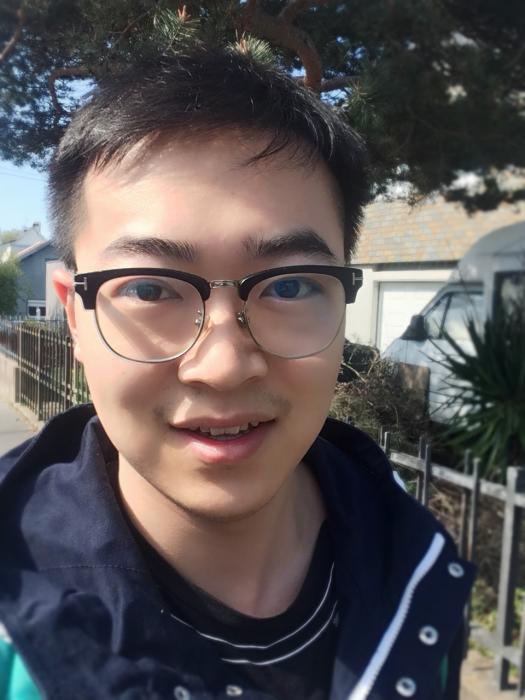
In recent decades, the field of autonomous driving has witnessed rapid development, benefiting from the development of artificial intelligence-related technologies such as machine learning. Autonomous perception in driving is a key challenge, in which multi-sensor fusion is a common feature. Due to the high resolution and rich information, the camera is one of the core perceptual sensor in autonomous systems. However, the camera provides no knowledge on distance (or depth), which is insufficient for the requirements of autonomous driving. On the other hand, LiDAR provides accurate distance measurements, however the information is sparse. The complementary characteristics of cameras and LiDAR have been exploited over the past decade for autonomous navigation. In order to be able to fuse the camera and LiDAR sensor system jointly, an efficient and accurate calibration process between sensors is essential. Conventional methods for calibrating the camera and LIDAR rely on deploying artificial objects, e.g., checkerboard, on the field. Given the impracticality of such solutions, targetless calibration solutions have been proposed over the past years, which require no human intervention and are readily applicable for various autonomous systems, e.g., automotive, drones, rovers, and robots.
In this thesis, we review and analyze several classic targetless calibration schemes. Based on some of their shortcomings, a new multi-feature workflow called MulFEA (Multi-Feature Edge Alignment) is proposed. MulFEA uses the cylindrical projection method to transform the 3D-2D calibration problem into a 2D-2D calibration problem and exploits a variety of LiDAR feature information to supplement the scarce LiDAR point cloud boundaries to achieve higher features similarity compared to camera images. In addition, a feature matching function with a precision factor is designed to improve the smoothness of the objective function solution space and reduce local optima. Our results are validated using the open-source KITTI dataset, and we compare our results with several existing targetless calibration methods. In many different types of roadway environments, our algorithm provides more reliable results regarding the shape of the objective function in the 6-DOF space, which is more conducive for the optimization algorithms to solve. In the end, we also analyze the shortcomings of our proposed solutions and put forward a prospect for future research in the field of joint camera-Lidar calibration algorithms.
This work is part of the EU ADACORSA project co-hosted by the TU Delft CAS group (link: https://cas.tudelft.nl/Research/project.php?id=160&pid=59)
The zoom link for the defense is https://tudelft.zoom.us/j/96202579105
CAS MSc Midterm Presentations
- Thursday, 7 October 2021
- 11:00-12:00
- Online [zoom]
Loudspeakers as Recording Devices in Public Address Systems
Tobias Roest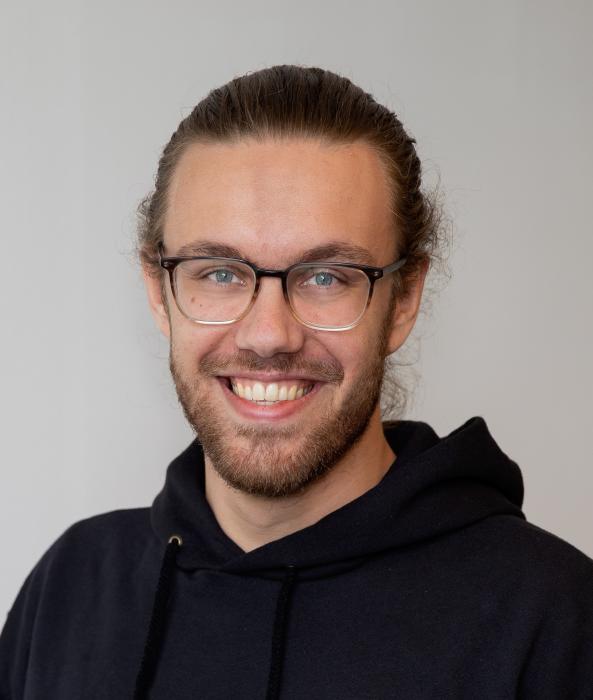
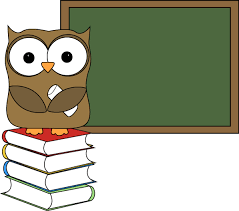
Signal Processing Seminar
- Thursday, 30 September 2021
- 11:00-12:00
- Online [zoom]
Information Theoretic Rank Estimation in Dynamic Contrast Enhanced Ultrasound Sequences
Metin Çalış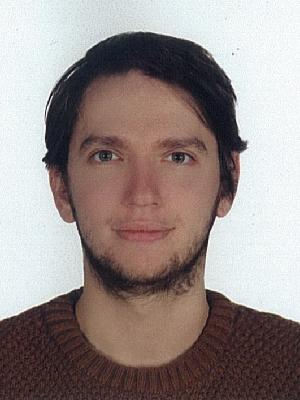
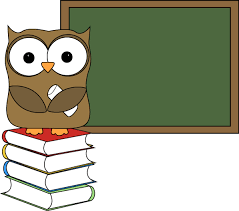
CAS MSc Thesis Presentation
- Thursday, 30 September 2021
- 14:00-15:00
- EWI Building 36, lecture hall J
Hybrid Posit and Fixed Point Hardware for Quantized DNN Inference
Zep Kleijweg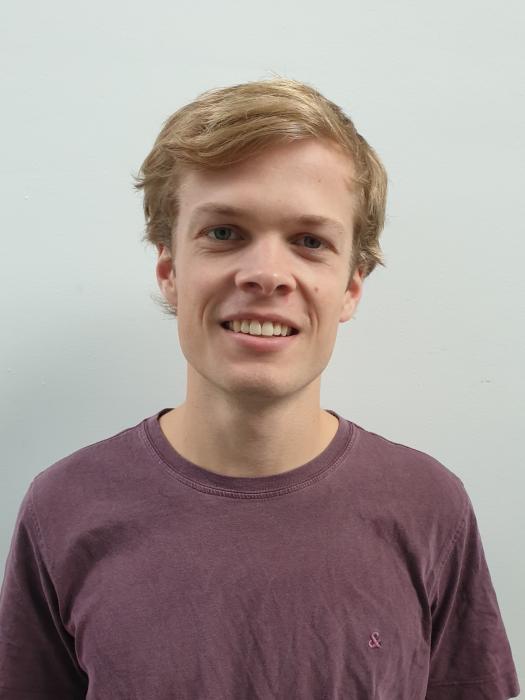
The recently introduced posit number system was designed as a replacement for IEEE 754 floating point, to alleviate some of its shortcomings. As the number distribution of posits is similar to the data distributions in deep neural networks (DNNs), posits offer a good alternative to fixed point numbers in DNNs: using posits can result in high inference accuracy while using low precision numbers. The number accuracy is most important for the first and last network layers to achieve good performance. For this reason, these are often computed using larger precision fixed point numbers compared to the hidden network layers. Instead, these can be computed using low precision posit, to reduce the memory access energy consumption and the required memory bandwidth. The hidden layer computation can still be performed using cheaper fixed point numbers.
An inference accuracy analysis is performed to quantify what the effect of this approach is on the VGG16 network for the ImageNet image classification task.
Using 8 bit posit for the first and last network layer instead of 16 bit fixed point is shown to result in a top-5 accuracy degradation of only 0.24%. The hidden layers are computed using 8 bit fixed point in both cases.
The design of a parameterized systolic array accelerator performing exact accumulation is proposed that can be used in a scale-out system along with fixed point systolic array tiles. To increase hardware utilization, a hybrid posit decoder is designed to enable fixed point computation on the posit hardware.
Using this hardware, the entire network can be computed using 8 bit data, instead of using 16 bits for some layers. This reduces energy consumption and the complexity of the memory hierarchy
CAS MSc Midterm Presentations
- Thursday, 23 September 2021
- 11:00-12:00
- Online [zoom]
Forecasting Multidimensional Graph Signals Using Graph Filters
Jelmer van der Hoeven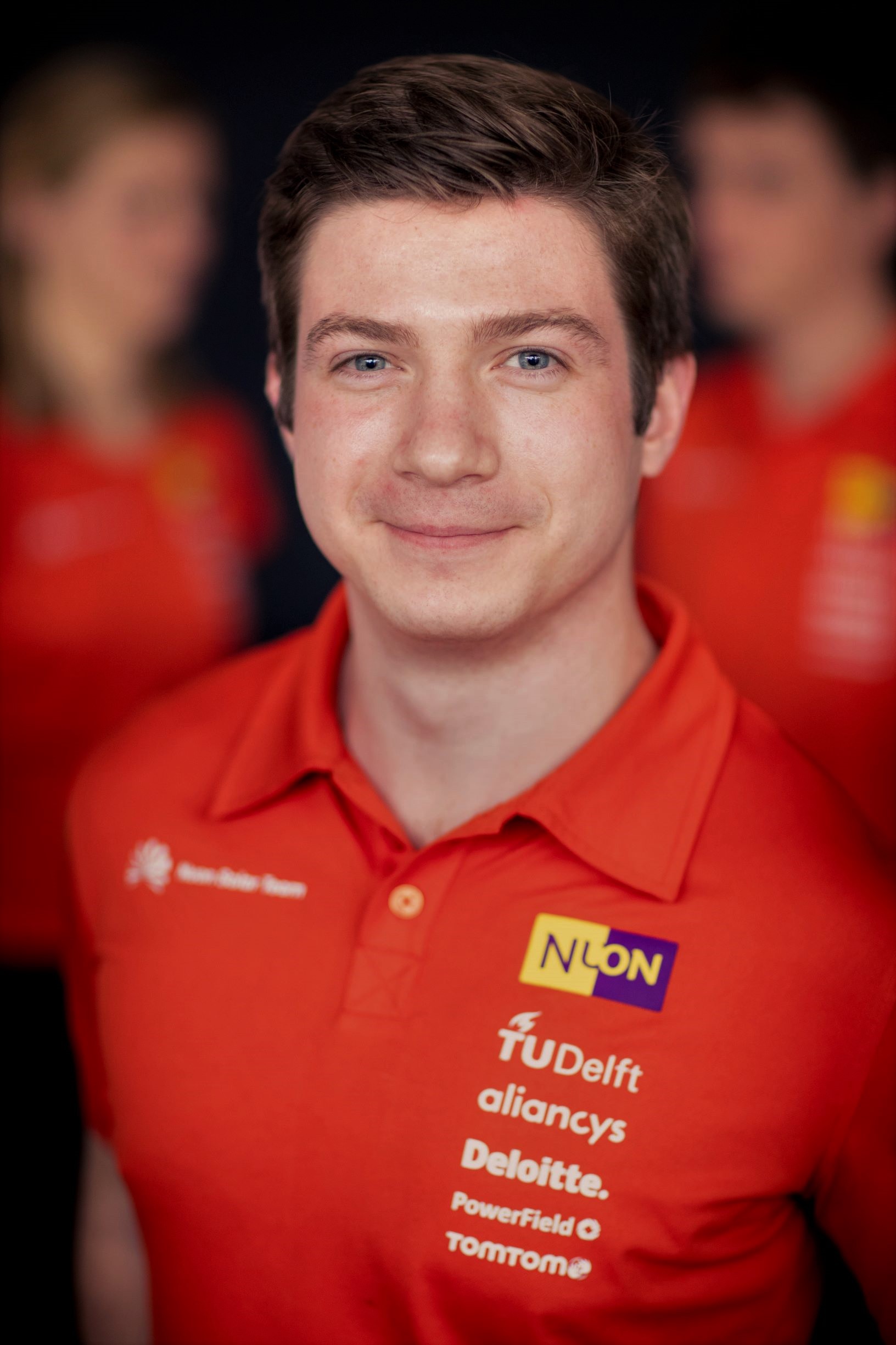
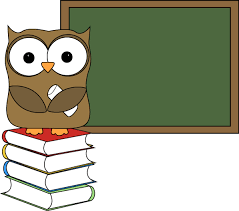
CAS MSc Midterm Presentations
- Thursday, 23 September 2021
- 11:00-12:00
- Online [zoom]
Analyzing Functional Ultrasound Images of the Brain Using Tensor Decompositions
Arda Kaygan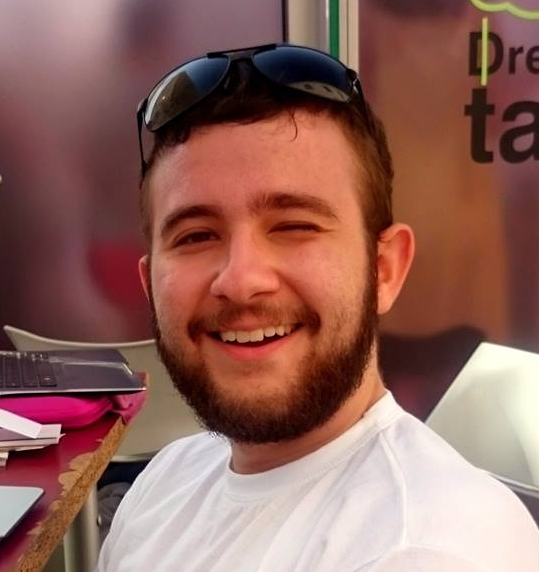
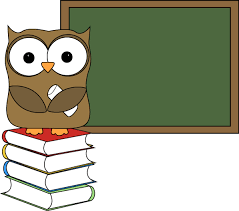
CAS MSc Midterm Presentations
- Thursday, 16 September 2021
- 11:00-12:00
- Online [zoom]
A Physiological Model for Heart Rate Analysis
Arthur Kordes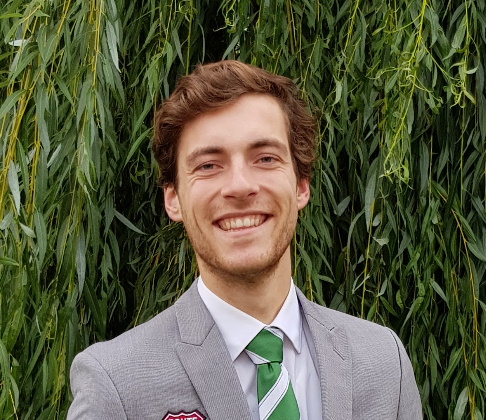
CAS MSc Midterm Presentations
- Thursday, 16 September 2021
- 11:00-12:00
- Online [zoom]
The Area of a Unipolar Electrogram to Identify the Arrhythmogenic Substrate
Eris van Twist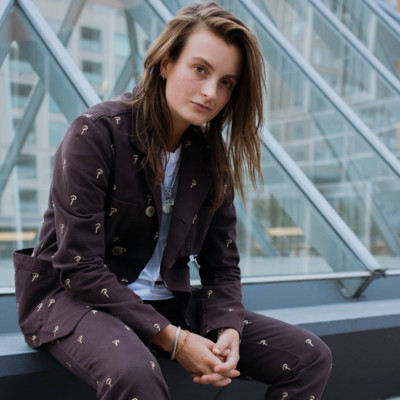
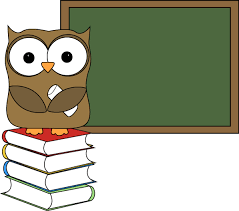
CAS MSc Thesis Presentation
- Thursday, 16 September 2021
- 10:00-11:30
- ZOOM
Sound Zones with a Cost Function based on Human Hearing
Niels de Koeijer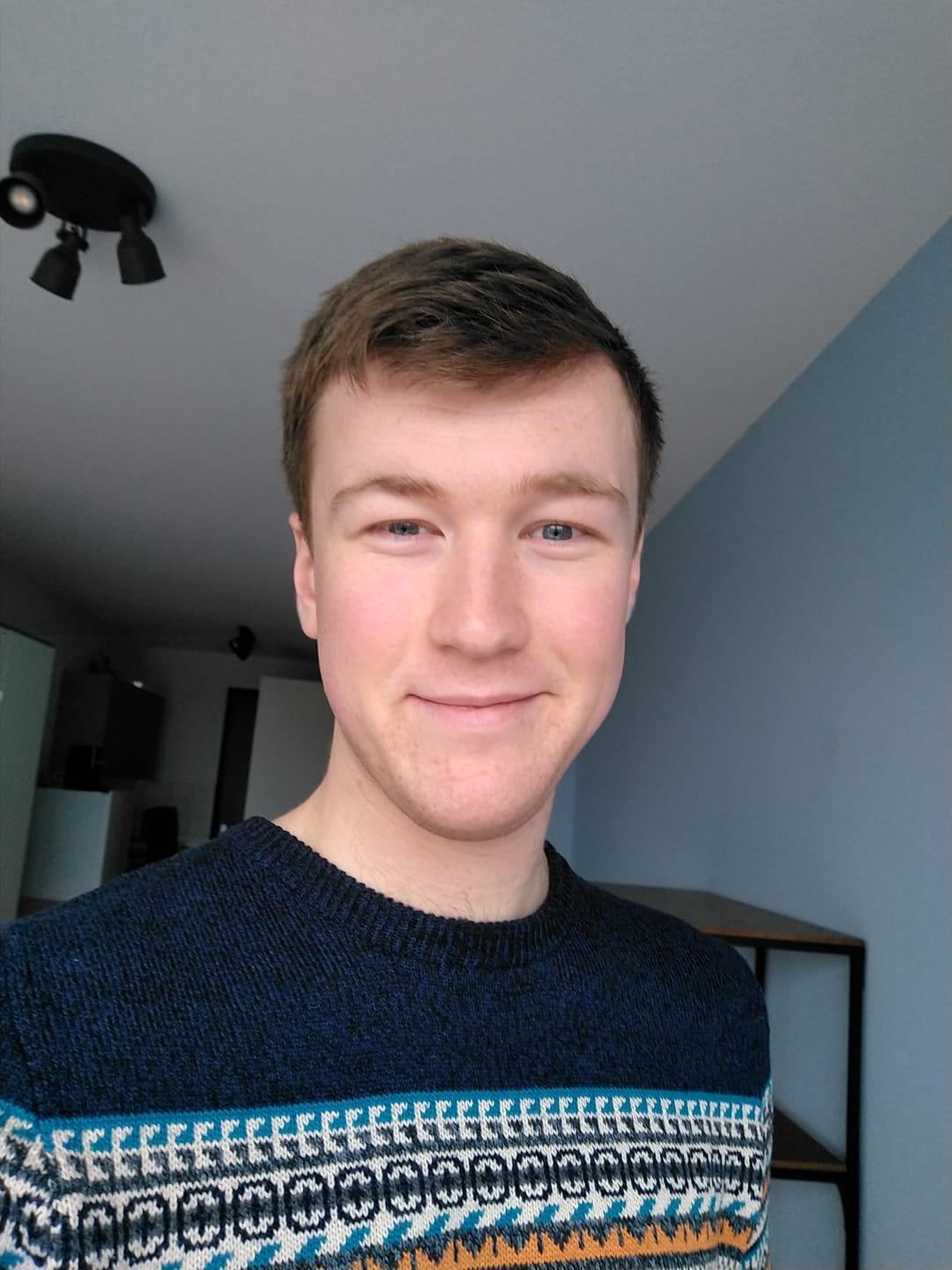
With the aid of an array of loudspeakers, sound zone algorithms seek to reproduce multiple distinct zones of audio inside an enclosure. Typical approaches determine the loudspeaker inputs by optimizing over a cost function that models the sound pressure inside the enclosure. However, recent methods propose cost functions that include a perceptual model of the human auditory system, which further models the perception of sound. This thesis investigates such an approach by proposing a framework within which sound zones are constructed through optimization over a perceptual model. The framework is used to propose two perceptual sound zone algorithms: unconstrained and constrained perceptual pressure matching. Simulations of the proposed algorithms and a reference algorithm are presented to determine the benefits of including auditory-perceptual information in sound zone algorithms. From this, it is found that the unconstrained perceptual approach outperforms the reference in terms of various perceptual measures. In addition, it is found that adding perceptual constraints to the optimization problem allows for control of sound zones which correlates well with other perceptual quality measures.
PhD Thesis Defence
- Monday, 13 September 2021
- 14:30
- Aula Senaatszaal
In-pixel temperature sensors for dark current compensation of a CMOS image sensor
Accel Abarca ProuzaThis thesis describes the integration of temperature sensors into a CMOS image sensor (CIS). The temperature sensors provide the in-situ temperature of the pixels as well as the thermal distribution of the pixel array. The temperature and the thermal distribution are intended to be used to compensate for dark current affecting the CIS. Two different types of in-pixel temperature sensors have been explored. The first type of temperature sensor is based on a substrate parasitic bipolar junction transistor (BJT). The second type of temperature sensor that has been explored is based on the nMOS source follower (SF) transistor of the same pixel. The readout system that is used for the temperature sensors and for the image pixels is based on low noise column amplifiers. Both types of in-pixel temperature sensors (IPTS) have been designed implementing different techniques to improve their accuracy. The use of the IPTSs has been proved by measuring three prototypes chips. Also, a novel technique to compensate for the dark current of a CIS by using the IPTS has been proposed.
For those who cannot attend, you can follow it by using this link:
https://collegerama.tudelft.nl/mediasite/play/84dc9775142d44db81aa38e5532c67ca1d
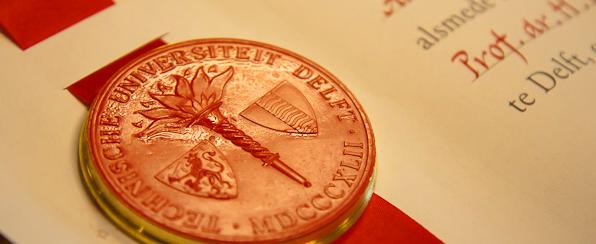
MSc SS Thesis Presentation
- Monday, 6 September 2021
- 14:30-15:15
Time Synchronization for Anchorless Satellite Networks
Felix Abel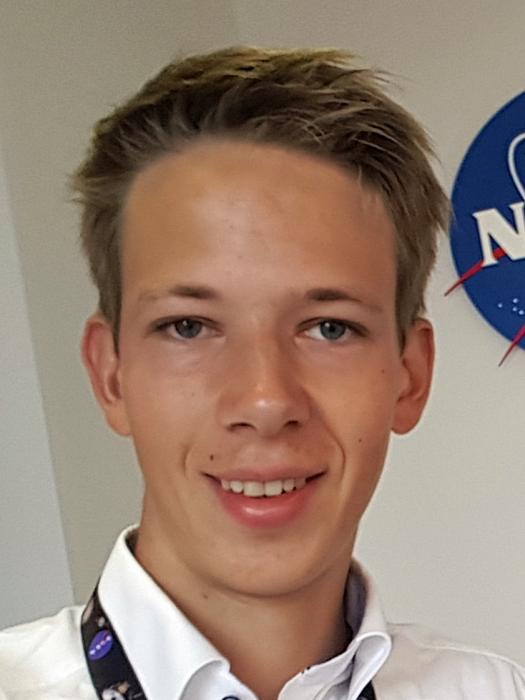
In this thesis, we propose a new class of pairwise frequency and multi-domain time synchronization and ranging algorithms and in a case study address network and mission level aspects of time synchronization on the example of the Orbiting Low Frequency Array for Radio astronomy (OLFAR), a proposed distributed radio interferometer.
The new class of frequency and multi-domain time synchronization and ranging algorithms proposed is applicable to anchorless mobile networks of asynchronous nodes. In a first step, the Frequency Pairwise Least Squares (FPLS) that estimates clock skew and relative velocity in a pairwise setup using only frequency measurements is formulated. In a second step, we extend this method to a motion model with constant acceleration. As frequency domain methods do not estimate clock offset and pairwise range, relying purely on frequency domain estimates is not feasible for most applications.
To harness the potential of frequency domain synchronization and ranging, the Combined Pairwise Least Squares (CPLS) has been developed. The combined method reduces the number of minimum required messages from 4 to 3 compared to current methods and decreases the computational complexity. Using a generic simulation with nodes in pairwise non-linear motion, we show that frequency domain methods can outperform time domain methods in clock skew and relative velocity estimation and that the proposed multi-domain method delivers better clock offset and pairwise range estimation in low to medium SNR conditions.
In the second part of our work, we apply the novel methods to OLFAR –— a spaceborne large aperture radio interferometric array platform. We address network level and mission level aspects, proposing network path planning for pairwise synchronization algorithms and determining the required resynchronization period. i
MSc SS Thesis Presentation
- Monday, 6 September 2021
- 10:00-11:30
On the Integration of Acoustics and LiDAR
Ellen Riemens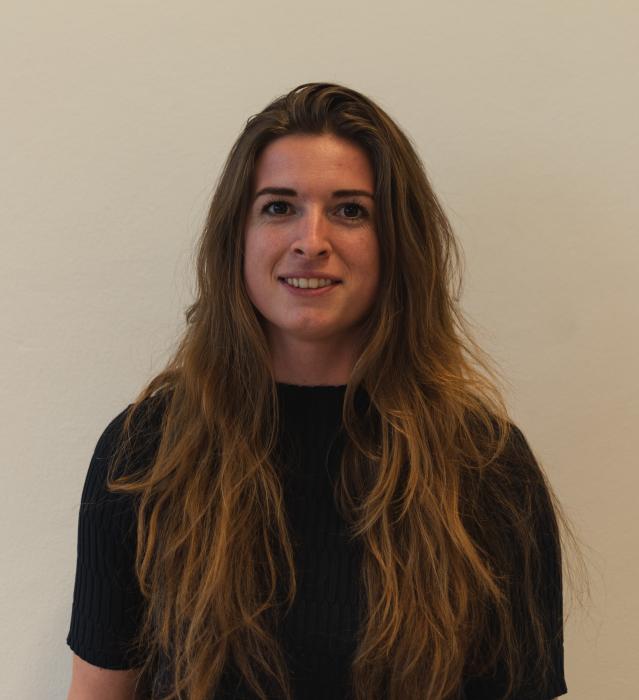
Loudspeakers are placed in an environment unknown to the loudspeaker designers. The room influences the acoustic experience for the user. Having information about the room makes it possible to better reproduce the sound field as intended. Using microphone measurements, the location of acoustic reflectors can be inferred. Current state-of-the-art methods for room boundary detection focus on a two-dimensional setting. Detection of arbitrary reflectors in three dimensions increase complexity due to practical limitations, i.e. the need for a spherical array and the increase of computational complexity. The presence of horizontal reflectors cause inaccuracy for wall detection due to model mismatch. Loudspeakers may not present an omnidirectional directivity pattern, as usually assumed in the literature, thus making the detection of acoustic reflectors in some directions more challenging.
In this thesis, a LiDAR sensor is added to a smart loudspeaker to improve wall detection accuracy and robustness. This is done in two ways. First, the horizontal reflectors that are not present in the acoustic model are sought detected with the LiDAR sensor to enable elimination of their detrimental influence. Second, a method is proposed to compensate for the challenging regions for wall detection in highly directive loudspeakers, using the LiDAR sensor. Experimental results, evaluated in different simulated scenarios are shown for comparison of the proposed method and the state-of-the-art method, that exclusively uses acoustic information.
MSc SS Thesis Presentation
- Wednesday, 25 August 2021
- 10:00-10:45
- zoom
Energy-efficient Particle Filter SLAM for Autonomous Exploration
Elke Salzmann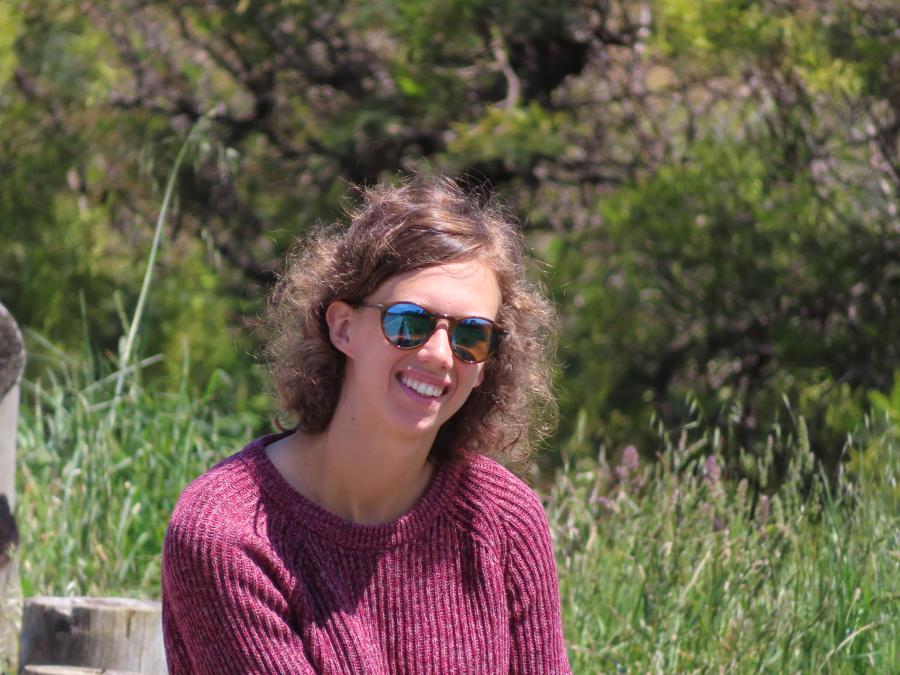
Autonomous robots are increasingly used in more and more applications, such as warehouse robots, search-and-rescue robots and autonomous vacuum cleaners. These applications are often in environments where the GPS signals are denied or inaccurate, which makes it difficult to localize the robot in an unknown environment. To overcome this problem the framework of Simultaneous Localization and Mapping (SLAM) is typically used. This solution constructs a map of the environment with the use of cameras or range sensors, while keeping track of the location of the robot in it. To extend the exploration time of these battery powered robots, the energy consumption of the SLAM algorithm could be reduced. It is assumed that if the computational load of an algorithm reduces, the energy consumption of the algorithm reduces as well. An existing paradigm to solve SLAM is the use of a particle filter, which tracks the trajectory of the robot and simultaneously maps the environment. The question answered in this thesis is how to make this algorithm more energy-efficient to be able to deploy this framework in more applications and make the existing robots more sustainable.
In this thesis two methods are investigated. In the first method, the information about the landmarks are incorporated in the trajectory estimation as spatial constraints, to try to achieve a higher accuracy with less particles and thus subsequently a smaller computational load. The proposed method is validated by simulations on synthetic datasets. This method shows improvements in terms of the estimation accuracy. However, it is more computational complex than the existing algorithms, so it is considered less energy-efficient. The second method in this thesis, is the implementation of a parallelized particle filter. This method processes the observation measurements in parallel for the different particles and communicates the information between the particles efficiently. It should reduce the computational time, to enable partial computation of the algorithm to reduce the computational load. This method shows improvements on the run time and thus on the computational load, especially for a larger number of particles and is therefore more energy-efficient. The two separate methods have been analyzed and compared with state of the art methods.
MSc SS Thesis Presentation
- Tuesday, 24 August 2021
- 10:00-10:45
- zoom
Quantifying the dynamic interactions between physiological signals to predict the exposure from chemicals
Sarthak Agarwal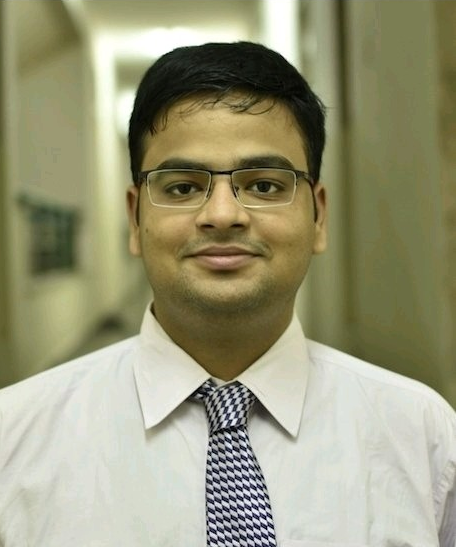
Causal inference is a familiar topic in biomedical research and a key concept in the study of connectivity in various physiological systems. This work aimed to analyse the coupling between the beat to beat parameters derived from ECG and respiration. It was the first time such an analysis was carried out in the context of finding the differences caused by chemical's exposure.
We used conditional Granger causality, a popular method to evaluate direct causal relationships. We have incorporated the cardinality constraint in the optimization function of Granger causality (GC) to deal with the high dimensionality challenge. Further, we extended the original formulation GC to evaluate the coupling between two unequally sampled signals. Finally, end to end implementation of the machine learning prediction model using causal features is well illustrated.
We found a consistent decrease in the average coupling strength of breathing parameters after the exposure. But in the case of ECG interactions, no noticeable change was observed. Surprisingly we found no significant links between the ECG and breathing parameters. The support vector machine (SVM) and random forest trained on coupling values differentiate between healthy and exposure samples. The accuracy of trained SVM and random forest on the independent test set were 78 % and 75 %, respectively.
Additional information ...
MSc SS Thesis Presentation
- Friday, 9 July 2021
- 10:00-10:35
- zoom
Edge State Kalman Filtering for Distributed Formation Control Systems
Martijn van der Marel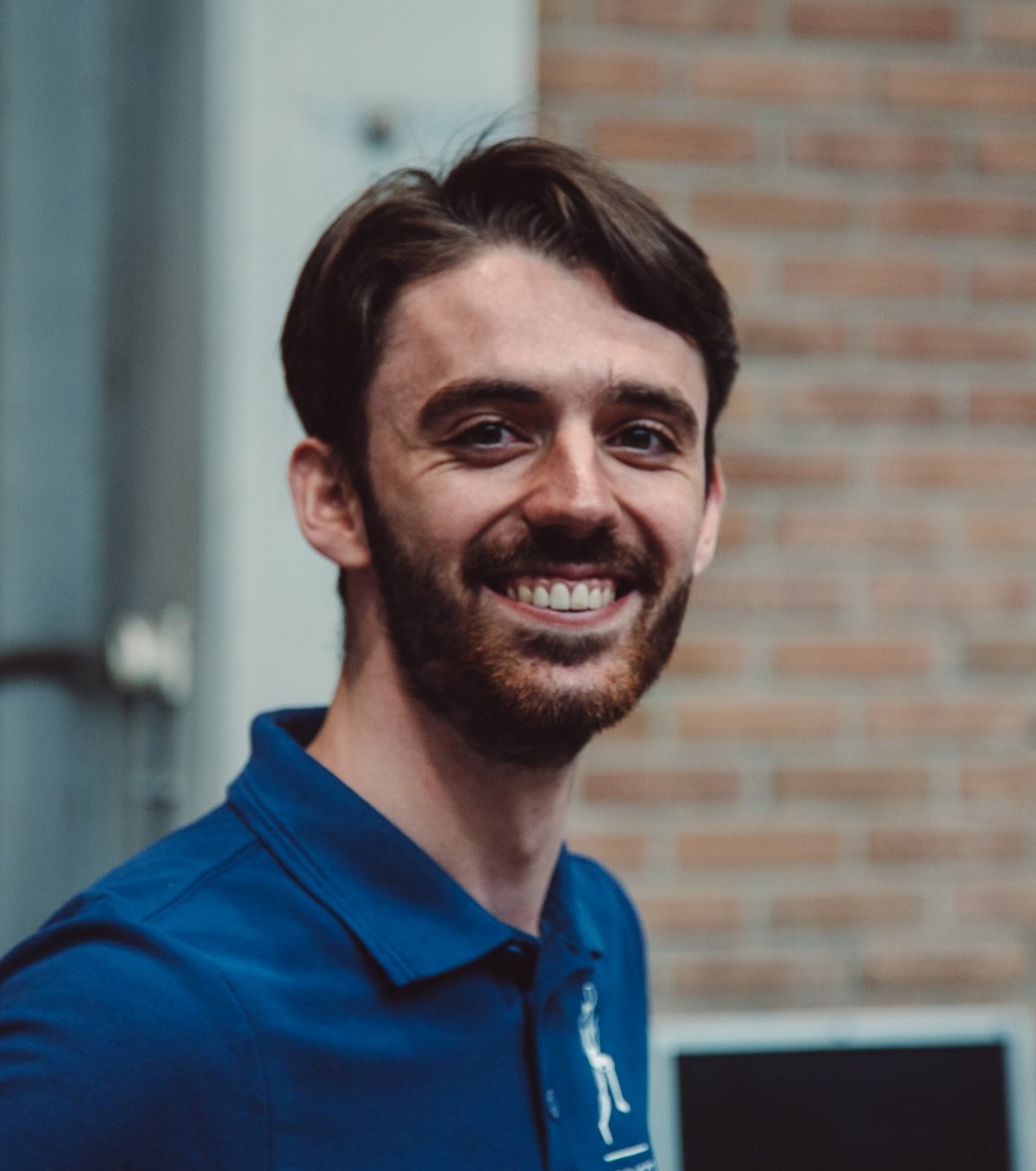
Formation control problems consider a set of mobile agents with the underlying goal of attaining and maintaining a state where the relative positions of agents are stable in accordance with the desired configuration. Navigation for formation control is typically achieved through localization in a global reference frame, e.g., via GNSS. However, when a global reference frame is not shared among agents, a relative navigation approach is required.
Distributed filtering for relative localization in formation control systems is a relatively unexplored field. The absence of absolute positioning means motivates the need for a distributed filter that operates on the edges of the sensing graph of the multi-agent system. In this thesis, a data model for relative formation control problems and two edge-based Kalman filters are proposed. The first filter is designed for an individual edge. The second is a filter designed via decoupling of the optimal global filter which allows for the joint estimation of adjacent edges. It is shown that the joint filter is optimal under the decoupling constraints.
Monte Carlo results show that when random environmental disturbances are correlated among agents, the joint filter outperforms the local edge filter in a mean square error sense.
Lastly, systems are considered where inter-agent communications are unavailable, leading to biased prediction steps of the Kalman filters. We aim to minimize this effect through the proposal of a local Wiener filter which predicts the control actions of neighboring agents.
PhD Thesis Defence
- Friday, 25 June 2021
- 15:00-16:30
- Aula Senaatszaal
Graph filter designs and implementations
Jiani Liu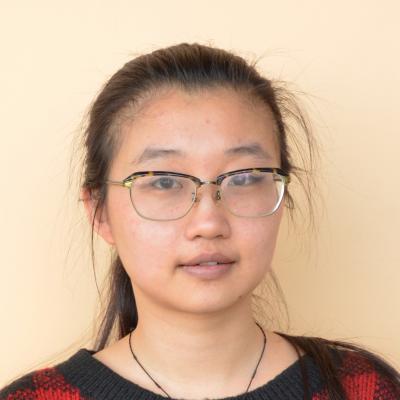
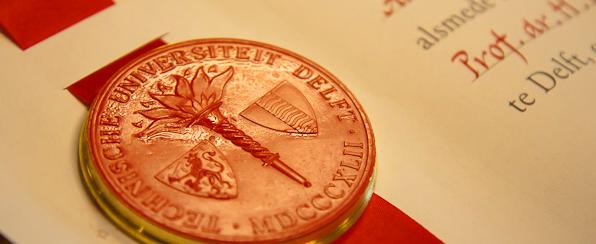
MSc SS Thesis Presentation
- Thursday, 17 June 2021
- 09:00-09:45
- zoom
SPLITTER
Stefanie Brackenhoff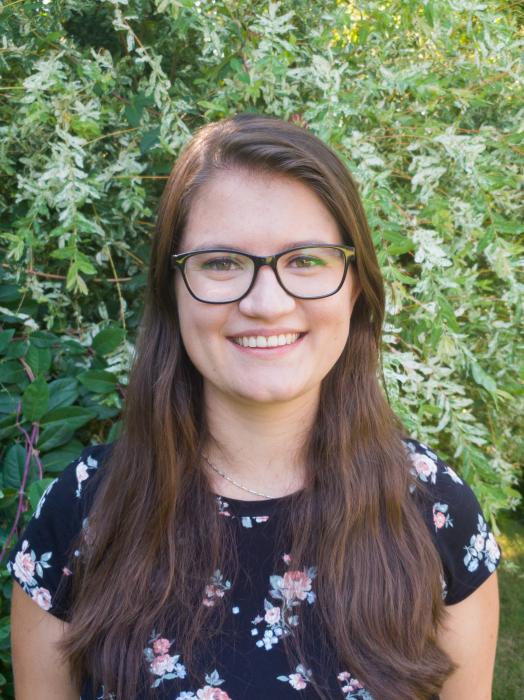
A data model and algorithm for detecting spectral lines and continuum emission of highredshift galaxies using DESHIMA 2.0
MSc SS Thesis Presentation
- Wednesday, 26 May 2021
- 10:00-10:45
- zoom
GNSS Chirp Interference Estimation and Mitigation
Daniel Kappelle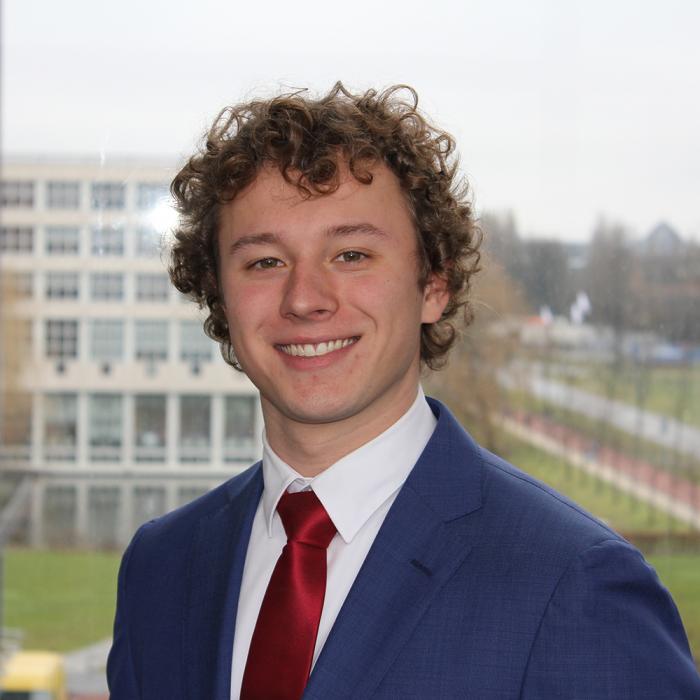
GNSS receivers can suffer severely from radio frequency interference (RFI). RFI can introduce errors in the position and time calculations or if the interference is very severe, can lead to a total loss of GNSS reception. This vulnerability of GNSS can have large implications on critical infrastructure such as power plants, telephony, aviation or search and rescue operations. RFI is a real threat to GNSS as many interfering incidents are reported every day.
A common type of RFI is chirp interference, which is a sweep over a wide range of frequencies that overlap with the frequencies used by GNSS. This is often emitted by cheap Personal Privacy Devices that can be bought online. The question in this thesis was how well such interference can be modelled and if modelling could help mitigation against it.
This thesis consists of two main parts. In the first part a novel estimator is proposed that assumes a mathematical model of a chirp and estimates its parameters from recordings of chirps. The estimator has shown to work well in simulations for chirps with an SNR of −9 dB or more. On real recordings the estimates were accurate for 66.7 % of the signals.
In the second part the estimator was used to derive a filter. The filter is based on the subtraction of a replica of the chirp interference from the received signal. It uses the proposed estimator to create the replica. In simulations, the filter is able to improve correlation strength by up to 7 dB. On real recordings the performance was worse as for only 46 % of the recordings the GNSS correlation was increased. Both the estimator and filter have many ways in which they could be improved. The estimator can be improved to allow for more complex chirps, which would in turn improve the filter. Both can also be made more computationally efficient.
Furthermore, in order to get a better understanding of Personal Privacy Devices, one such device has been tested. It was found that the signal from the device was very unstable and changed much over time, it was also highly dependent on ambient temperature. The device has also been opened up and reverse engineered to understand how it works.
MSc SS Thesis Presentation
- Wednesday, 26 May 2021
- 13:00-13:45
Correction of Field Inhomogeneities in Low-Field MRI During Image Reconstruction
Bas Liesker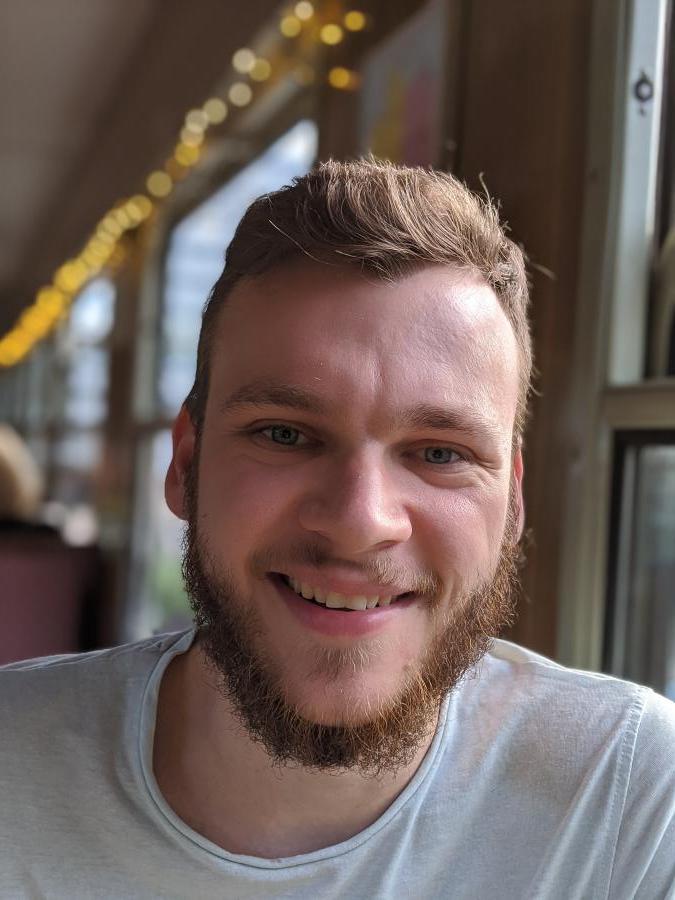
Magnetic resonance imaging (MRI) scanners are a crucial diagnostic tool for radiologists. They are able to render two and three-dimensional images of the body without exposure to harmful radiation. MRI systems are, however, costly to build and maintain. This adversely impacts access to these scanners in developing regions. In an effort to combat this problem, a low-field MRI scanner is being developed.
Conventional MRI scanners utilize a superconducting solenoid to generate the main magnetic field. The low=field scanner, on the other hand, induces the main magnetic field through a Hallbach array of permanent neodymium magnets. While beneficial for production and maintenance costs, as well as portability, the Hallbach array is not able to generate a perfectly homogeneous magnetic field.
The inhomogeneities present in the main magnetic field result in distortion of the images when reconstructed using conventional fast Fourier transform (FFT) methods. To counteract this, a reconstruction method that utilizes field information needs to be employed. In this thesis, existing methods to determine and utilize the field information to correct image distortion are explored. From this analysis, it is evident that model-based (MB) methods are most suitable for reconstruction of data from the low-field scanner. Current MB methods are only implemented for two-dimensional reconstruction. The goal of this thesis is to expand these methods to threedimensional reconstruction.
A novel MB method for three-dimensional reconstruction is presented. This new method is able to circumvent memory constraints that arise from reconstruction of large data sets.
Though the new method requires several hours to reconstruct a 128 × 128 × 30 data set, visual inspection indicates that an accurate result is achieved.
PhD Thesis Defence
- Wednesday, 21 April 2021
- 15:00-16:00
- online
Advances in graph signal processing - Graph filtering and network identification
Mario Coutino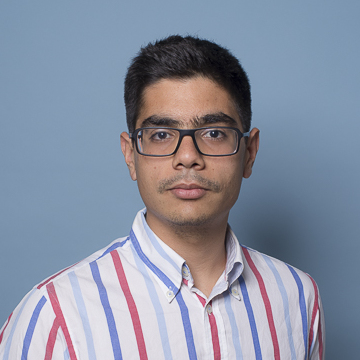
To the surprise of most of us, complexity in nature spawns from simplicity. No matter how simple a basic unit is, when many of them work together, the interactions among these units lead to complexity. This complexity is present in the spreading of diseases, where slightly different policies, or conditions, might lead to very different results; or in biological systems where the interactions between elements maintain the delicate balance that keep life running. Fortunately, despite their complexity, current advances in technology have allowed us to have more than just a sneak-peak at these systems. With new views on how to observe such systems and gather data, we aim to understand the complexity within.
One of these new views results from, the field of graph signal processing, providing models and tools to understand and process data coming from such complex systems. With a principled view, coming fromits signal processing background, graph signal processing establishes the basis for addressing problems involving data defined over interconnected systems by combining knowledge fromgraph and network theory with signal processing tools. In this thesis, our goal is to advance the current state-of-the-art by studying the processing of network data using graph filters, the workhorse of graph signal processing, and by proposing methods for identifying the topology (interactions) of a network fromnetworkmeasurements.
To extend the capabilities of current graph filters, the network-domain counterparts of time-domain filters, we introduce a generalization of graph filters. This new family of filters does not only provide more flexibility in terms of processing networked data distributively but also reduces the communications in typical network applications, such as distributed consensus or beamforming. Furthermore, we theoretically characterize these generalized graph filters and also propose a practical and numerically-amenable cascaded implementation.
As all methods in graph signal processing make use of the structure of the network, we require to know the topology. Therefore, identifying the network interconnections from networked data is much needed for appropriately processing this data. In this thesis, we pose the network topology identification problem through the lens of system identification and study the effect of collecting information only from part of the elements of the network. We show that by using the state-space formalism, algebraic methods can be applied to the network identification problemsuccessfully. Further, we demonstrate that for the partially-observable case, although ambiguities arise, we can still retrieve a coherent network topology leveraging state-of-the-art optimization techniques.
Additional information ...
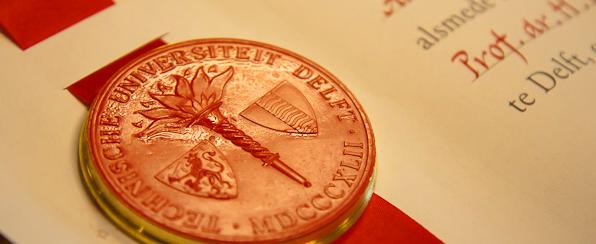
MSc SS Thesis Presentation
- Tuesday, 20 April 2021
- 10:00-10:45
- zoom
Boundary Element Method in Coil Design for Magnetic Resonance Imaging
Teun de Smalen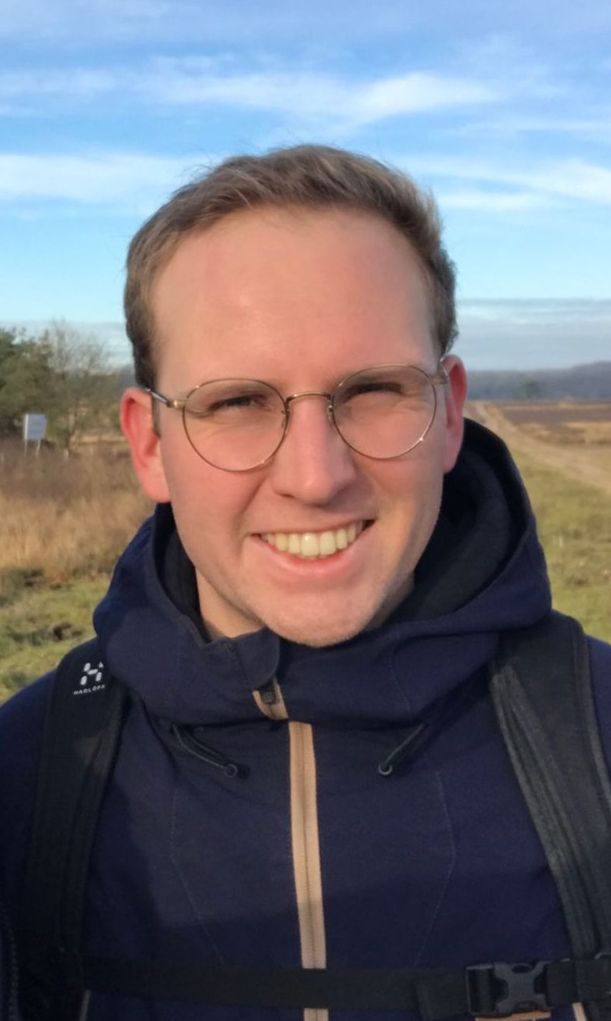
MRI is an non-invasive imaging technique used by many physicians to diagnose and treat diseases. The technique however is still very expensive and thus out of reach for developing countries. This has led to the goal to design a low-cost MRI system. The challenges that arise from this system make it necessary to design coils in a different way than conventional MRI.
In this work the inverse boundary element method is used to create a coil design method for an arbitrary surface. This method is described and the mathematical framework is analyzed. A regularization method for the inverse problem has been designed in the form of a regularization matrix. This regularization matrix is constructed such that it can handle arbitrary surfaces. The regularization matrix is applied using Tikhonov regularization.
To validate the design method a proof of concept radiofrequency coil for the low field MRI system at the LUMC has been realized. This coil is designed and has been used to image the human brain of an adult. The results from simulations beforehand are in agreement with the physically built coil showing that this method makes it possible to design and construct a physically feasible coil on an arbitrary surface.
Signal Processing Seminar
- Thursday, 15 April 2021
- 13:30-14:30
- zoom
ONLINE TIME-VARYING TOPOLOGY IDENTIFICATION VIA PREDICTION-CORRECTION ALGORITHMS
Alberto Natali.jpg)
Signal processing and machine learning algorithms for data supported over graphs, require the knowledge of the graph topology. Unless this information is given by the physics of the problem (e.g., water supply networks, power grids), the topology has to be learned from data. Topology identification is a challenging task, as the problem is often ill-posed, and becomes even harder when the graph structure is time-varying. In this paper, we address the problem of dynamic topology identification by building on recent results from time-varying optimization, devising a general-purpose online algorithm operating in non-stationary environments. Because of its iteration-constrained nature, the proposed approach exhibits an intrinsic temporal-regularization of the graph topology without explicitly enforcing it. As a case-study, we specialize our method to the Gaussian graphical model (GGM) problem and corroborate its performance.
Additional information ...
PhD Thesis Defence
- Tuesday, 13 April 2021
- 12:30-13:30
- online
Rate-constrained multi-microphone noise reduction for hearing aid devices
Jamal Amini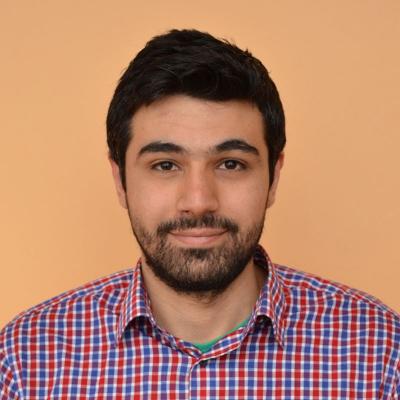
Many people around the world suffer fromhearing problems (In theNetherlands, around 11%of the population is considered hearing-impaired). To overcome their hearing problems, advanced technologies like hearing aid devices can be used. Hearing aids are meant to assist the hearing-impaired to improve the speech intelligibility and the quality of sounds that they intend to hear. Usually these include processors which are mainly designed to enhance the sound signals originating form the source of interest by reducing the environmental noise. Binaural hearing aids, on the other hand, can also help to preserve some spatial information from the acoustic scene, which can help the hearing aid user to hear the sounds from the correct locations. To construct the binaural hearing aid system, two hearing aids are needed to be placed in the left and the right ears, which can potentially communicate through a wireless link. In addition, one can think of additional assisting devices with microphones placed in the environment. One common way to reduce the noise is to use advanced binaural multi-microphone noise reduction algorithms, which aim at estimating some desired sources while reducing the power of the undesired sources. One typical method is to use spatial filtering, which aims at estimating the target signal by shaping the beam towards the location of the desired source while canceling/suppressing the other sources.
To performbinaural noise reduction, while assuming centralized processing, the signals recorded at remote microphones (for example from additional assisting devices or in the binaural hearing aid setup, the sound signals from the contralateral hearing aid) need to be transmitted to the central processor. Due to the power and bandwidth limitations, the data needs to be compressed before transmission. Therefore, the main question would be, at which rate the data should be compressed to have reasonably good noise reduction performance. This links the noise reduction problem to the data compression problem. Generally, the higher the data rate, the better the noise reduction performance. Therefore, there is a trade-off between the performance of the noise reduction algorithmand the data-rate at which the information is compressed. This problem is closely connected to the rate-distortion problem from an information-theoretic viewpoint. Studying the effect of data compression on the performance of noise reduction problems would be of great interest to reduce the power consumption of hearing assistive devices.
Oneway to incorporate data compression into the noise reduction problem is to perform quantization, which leads to a rate-constrained noise reduction problem. In the rate-constrained noise reduction, the goal is to estimate the desired sources based on the imperfect data. The observations from remote sensors are quantized and transmitted to the fusion center. The main challenge in the binaural rate-constrained noise reduction is to find the best quantization rates for the different sensors at different frequencies, given the physical constraints like bitrate and power constraints.
Another aspect of the rate-constrained noise reduction is to expand the network to receive more information on the acoustic scene using additional assistive devices. Target source estimation using information form such assistive devices (rather than only binaural hearing aids) is shown to result in better noise reduction performance. Now the question is how to allocate the bitrates to the assistive devices as well. These assistive devices can be thought of as the remote embedded microphones on the cell-phones (mobile) or wearablemicrophones placed at the users’ bodies. The binaural hearing aid system can thus be generalized to allow other assistive devices to contribute to noise reduction.
In this dissertation, we study and propose different rate-constrained multi- microphone noise reduction algorithms. We try to expand the notion of the binaural rateconstrained noise reduction to multi-microphone rate-constrained noise reduction for general wireless acoustic sensor networks (WASNs). The WASN in this case can include the binaural setup along with other assistive devices. We propose different algorithms to cover the main objectives of rate-constrained noise reduction problems. These objectives mainly include good target estimation (less environmental noise power) given the compressed data, good rate allocation strategies in WASNs, and preferably preserved spatial information of the sources in the acoustic scene to get the correct impression of the acoustic scene.
Additional information ...
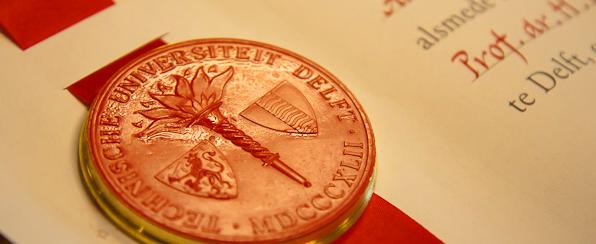
Ph.D. Thesis Defense of Sining Pan
- Monday, 12 April 2021
- 12:00-13:30
Resistor-based temperature sensors in CMOS technology
Sining Pan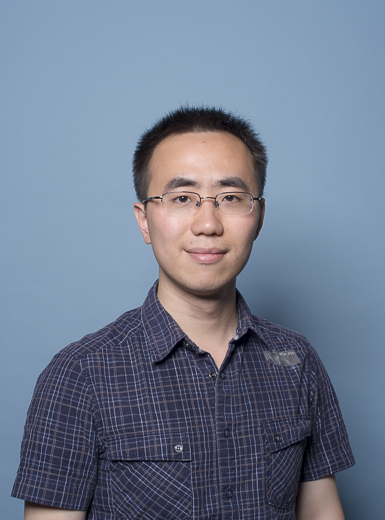
Time: Monday, 12 April 2021, 12:00-12:15 (layman’s talk), 12:30-13:30 (defense)
Abstract: This thesis describes the principle and design of an emerging type of CMOS temperature sensors based on the temperature dependency of on-chip resistors. Compared to traditional BJT-based designs, resistor-based sensors have higher energy-efficiency, better scalability, and can operate under a wider supply range. Nine design examples are shown in this thesis to demonstrate how resistor-based sensors can be optimized for accuracy, energy-efficiency, or other application-driven specifications. Among all the records the designs achieved, the energy-efficiency improvement is the most impressive: 65× better than state-of-the-art before this research, or only 6× away from the theoretical value.
Please feel welcome to join the live stream: http://collegerama.tudelft.nl/mediasite/play/be505395bbf24debb7cf8fd61454a5261d
Thesis: https://doi.org/10.4233/uuid:28108302-2d9b-4560-a806-8ba6d381812e Additional information ...
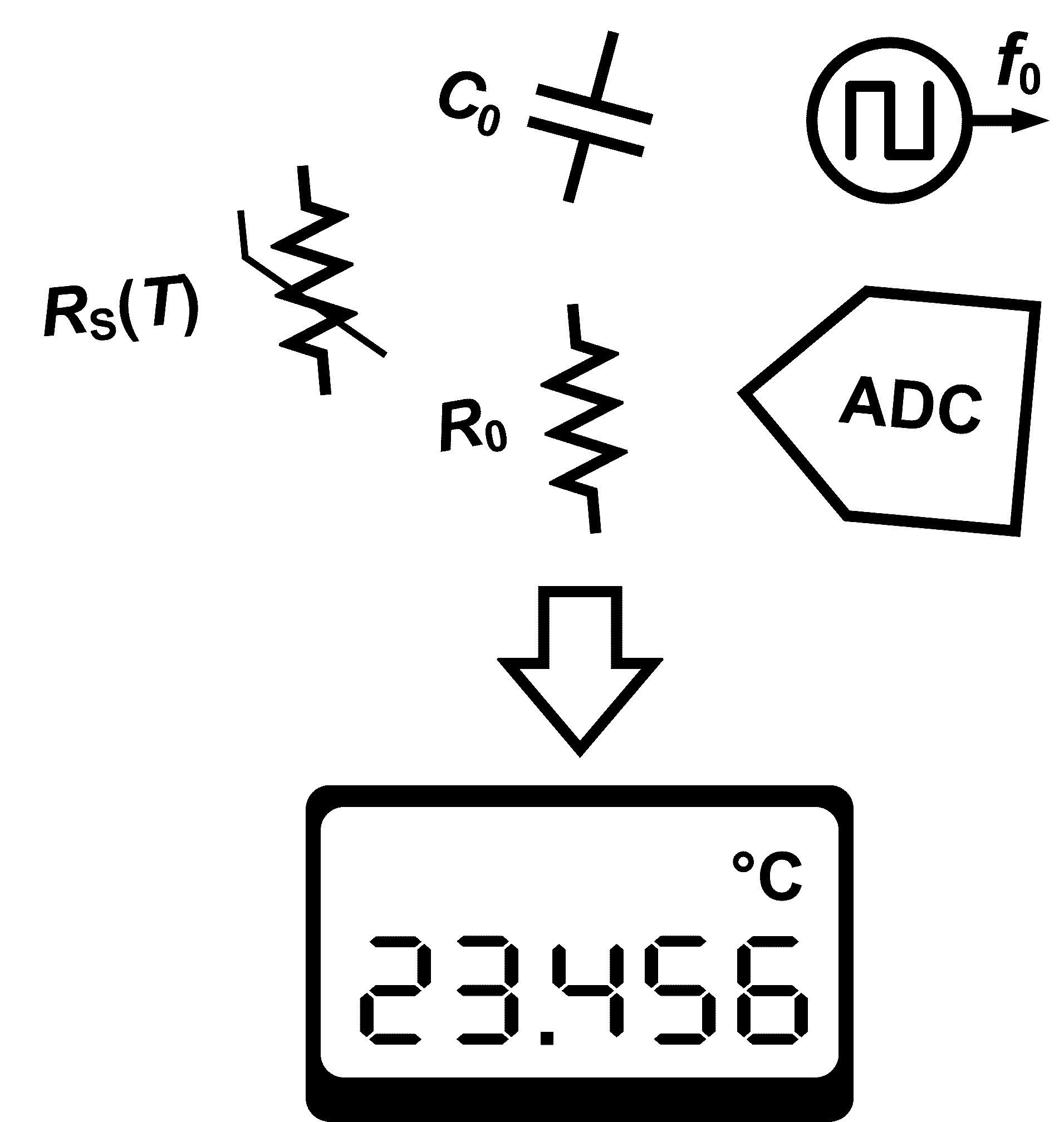
MSc Thesis Presentation
- Tuesday, 6 April 2021
- 10:45-11:30
- online
Mice tracking using infrared subcutaneous implants for error detection
Javi Guinea PerezThe objective of this thesis was to develop a rodent tracker using the FlashTrack implants, and allow for tracking data to be used for behavioural research. The task was split into three main problems: detection, tracking and error detection.
The detection was solved with basic image processing. The first step was background removal, using median filtering. Later, blob detection after some processing was done. Detections were differentiated into cases where mice are together in contact events and where they are alone. Bounding boxes were generated for the contours of lone mice, while the distance transformwas employed to detect the joint ones.
To track the moving targets, Kalman filtering was used on the bounding boxes of the detector. This approach was based on the Simple Online Realtime Tracking framework, adapting it to the particularities of mice. Other approaches were tried, but SORT was the chosen one.
The infrared subcutaneous implants of FlashTrack allowfor identity verification through code detections. To process the codes of each track, a GaussianMixtureModel is trained to be the classifier of the detections. A track handling modulewas built tomonitor the estimated tracks and code detections, and verify correct assignments. Detected erroneous tracks were discarded.
Synthetic data was used to evaluate the tool. Artificial datasets were developed in Blender. Common metrics for evaluation of multiple object trackers were gathered and discussed, as well as a comparison with one of the state of the art animal trackers.
The (parametric) Voice of Your Heart
- Thursday, 25 March 2021
- 15:30-16:30
- TEAMS
Towards Parametric Cardiac Modelling for early recognition and Treatment of AF
Richard Hendriks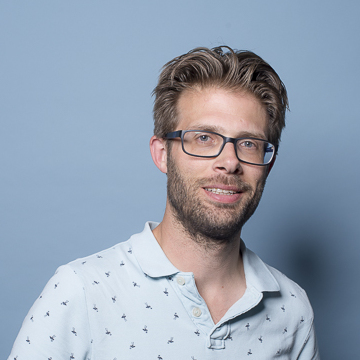
Atrial Fibrillation (AF) is the most common sustained cardiac arrhythmia, with a prevalence that continuously increased over the past decades. The risk of developing AF among people aged 40 years or older is currently about 25 %. Despite its high prevalence, the exact mechanisms underlying AF are unknown and available treatment strategies are not effective. Although AF in itself is not directly life-threatening, it can lead to many serious complications like heart failure and strokes. As the disease is progressive, early (non-invasive) detection is important.
To increase understanding on the mechanisms underlying AF and develop a better treatment strategy we have set up a collaboration between the Erasmus medical center (Unit Electrophysiology) and TUDelft, where we develop atrial parametric models that can aid in the understanding, detection and treatment of AF. In this presentation I will highlight some of the recent activities, opportunities and outcomes of this collaboration.
If you you would like to join this colloquium, send an email to secr-me-ewi@tudelft.nl and you will receive the TEAMS link.
Additional information ...
Signal Processing Seminar
- Thursday, 4 March 2021
- 13:30-14:30
- zoom

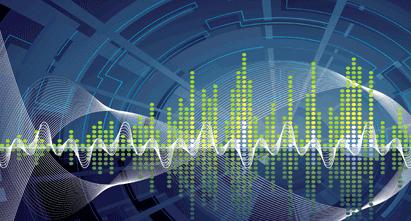
Signal Processing Seminar
- Thursday, 25 February 2021
- 13:30-14:30
- HB 17.150
MSc Kickoff presentation
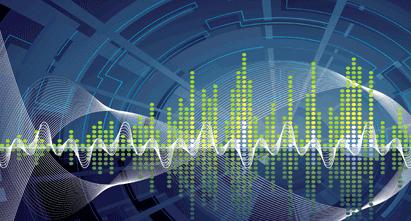
Signal Processing Seminar
- Thursday, 18 February 2021
- 13:30-14:30
- https://tudelft.zoom.us/j/95586942330
Computational Array Signal Processing
Ayush BhandariImperial College London, UK
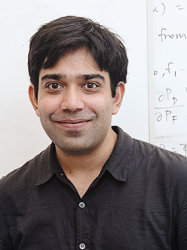
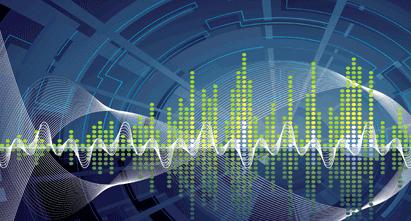
Signal Processing Seminar
- Thursday, 18 February 2021
- 16:00-16:45
- zoom
Linear systems with sparsity constraints
Geethu JosephSyracuse University, NY, USA
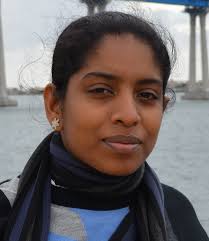
Many applications in digital signal processing, machine learning, and communications feature signals that admit a sparse representation in an appropriate basis, i.e., the vector of interest (unknown) has a few nonzeros elements compared to its dimension. The talk covers new mathematical theory and algorithms for recovering sparse vectors from a reduced set of available measurements. We discuss several real-world problems like anomaly imaging, wireless channel estimation, and image denoising and compression. For each application, we describe the inherent sparsity structure and constraints in the system. The talk explores the design and analysis of algorithms that can exploit the underlying signal structures, and the results reveal the impact of these structures on the recovery problems.
Geethu Joseph is currently a post-doctoral fellow at the Department of Electrical Engineering and Computer Science, Syracuse University, NY. Her research interests include statistical signal processing, adaptive filter theory, sparse Bayesian learning, and compressive sensing.
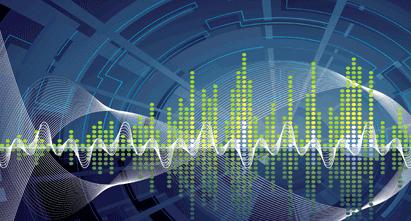
Signal Processing Seminar
- Thursday, 11 February 2021
- 13:30-14:30
- HB 17.150
MSc kick off presentation, Gaia Zin and Sybold Hijlkema
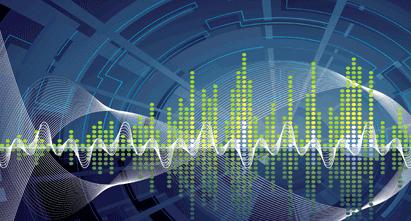
CAS MSc Midterm Presentations
- Thursday, 4 February 2021
- 13:30-14:30
- HB 17.150
Towards multimodal reflecting boundaries detection, Perceptual Sound Zones: Improving Sound Zones by Adding a Model of the Human Hearing
Ellen Riemens, Niels de Koeijer
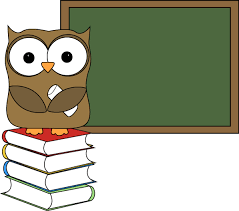
CAS MSc Midterm Presentations
- Thursday, 28 January 2021
- 13:30-14:30
- zoom
GPS spoofing detection
Daniel Kappelle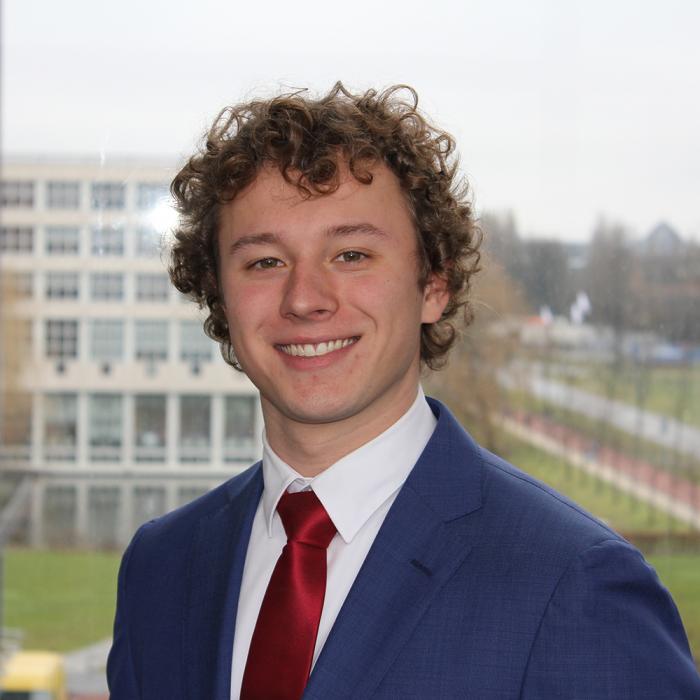
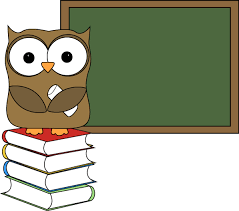
CAS MSc Midterm Presentations
- Thursday, 14 January 2021
- 11:30-12:30
- https://tudelft.zoom.us/j/99529802347
Wideband astronomical spectroscopy in high contrast
Stefanie Brackenhoff, Elke Salzmann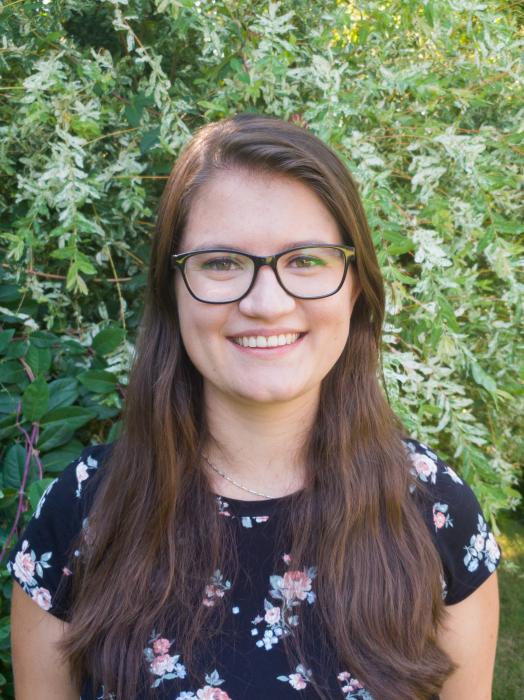
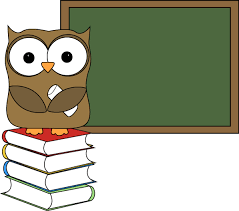
Medical Delta Café
- Thursday, 14 January 2021
- 16:00-17:00
- Online via Teams
Medical Delta Café 'Zorg naar huis, en dan….? Van monitoren tot behandelen'
Wouter Serdijn, Frank Willem Jansen (Medical Delta), Gisela Terwindt (LUMC), Ries Biggelaar van den (ErasmusMC)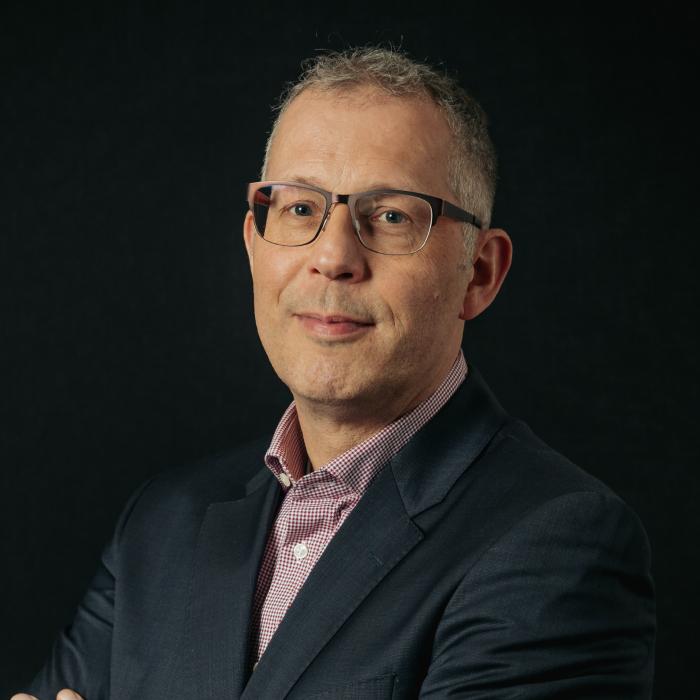
In het online Medical Delta Café 'Zorg naar huis, en dan….? Van monitoren tot behandelen' belichten prof. dr. Gisela Terwindt (LUMC) en drs. Ries van den Biggelaar (Erasmus MC) deze kwesties, waarna deelnemers worden uitgenodigd mee te discussiëren en kennis uit te wisselen in een paneldiscussie met onder andere Medical Delta hoogleraar prof. dr. ir. Wouter Serdijn (TU Delft).
Additional information ...
MSc SS Thesis Presentation
- Monday, 11 January 2021
- 10:00-10:45
- zoom
Graph-aware Anomalous Network Agent Detection
Ahmet Gercekcioglu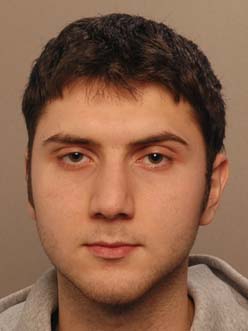
Networks with a large number of participants and a highly dynamic data exchange are better off using a distributed networking system due to network failures in centralized networks. However, with the increase in distributed networking, security problems arise in distributed processes. Injection of malicious data, for example, must be dealt with by using the tools provided by detection theory. The detection probability pd can be taken as the performance metric that we aim to optimize. In order to achieve this, one must first define a hypothesis testing problem and derive an optimization problem for pd that is dependent on which nodes are assumed to be compromised by these malicious agents that inject data.
For the injected data, there are three different models taken into consideration for the change in values over time and nodes. For every model, we assume that the outlier data can be injected with two different attack modes. These attack modes enforce different network topology related constraints on the set of compromised nodes due to different motivations. Furthermore, additional constraints are assumed due to the limited resources of the agents.
Additionally, with the given framework, we can also derive an optimization problem that can be solved with the help of the well-known linear regression method, i.e., Lasso. The problem that arise with this method is the difficulty of implementing the network topology related constraints into this optimization problem.
In order to solve these optimization problems, several methods are combined for the relaxation and solution of the optimization problems.
From numerical evaluation, it can be observed that our empirical performance is non-negligibly lower than the theoretical performance for all three models and both attacking modes. This can be linked to the dependence of the empirical distribution to the derived subset of compromised nodes, these subsets are chosen such that the cost function is optimized. Hence, we observe that the empirical values are much higher than it is theoretically assumed, in case that the network is 'clean'.
A second factor for performance evaluation is the number of wrongly indexed nodes, it can be observed how this factor is dependent on the distribution of the energy of the outlier data over the nodes and time.
Overall, this study shows that the provided framework shows an increasing performance for an increasing outlier-to-noise energy ratio. For an energy ratio higher than 0.5, the empirical and theoretical ROC-curves are nearly perfectly saturated for all models. The number of wrongly indexed nodes for our methods is generally speaking lower compared with the Lasso-method.
CAS MSc Kickoff Presentation
- Thursday, 7 January 2021
- 10:30-11:45
- zoom
Analyzing functional ultrasound images of the brain using multilinear decompositions
Arda Kaygan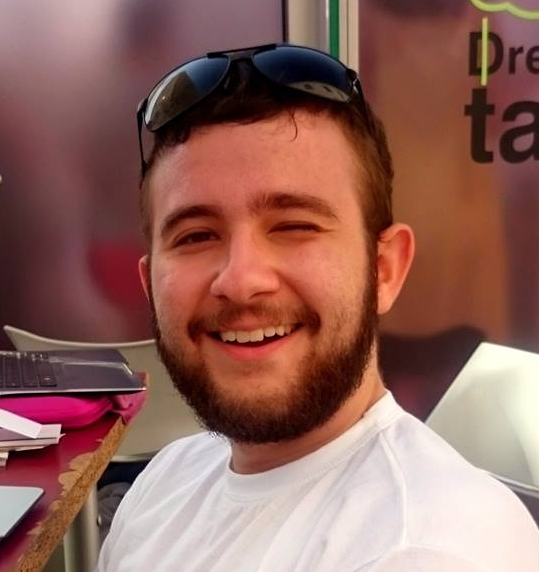
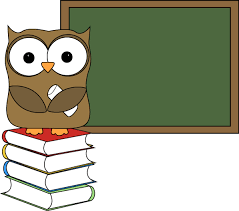
Signal Processing Seminar
- Thursday, 7 January 2021
- 11:30-12:15
- zoom
Rank detection thresholds for Hankel or Toeplitz data matrices
Alle-Jan van der Veen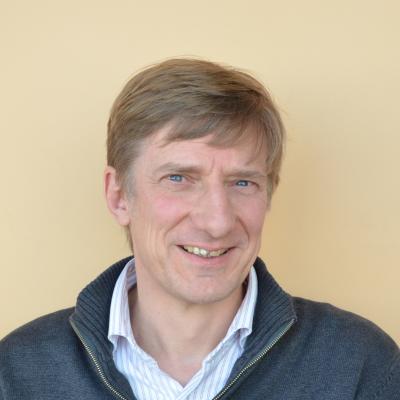
To detect the number of sources in a data matrix, we look at the singular values. What is a good threshold?
Additional information ...
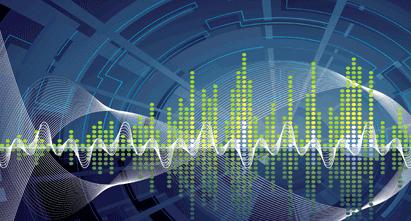
PhD Thesis Defence
- Tuesday, 22 December 2020
- 15:00-16:30
- Aula Senaatszaal / zoom
ELECTROMAGNETIC FIELDS IN MRI: Analytical Methods and Applications
Patrick Fuchs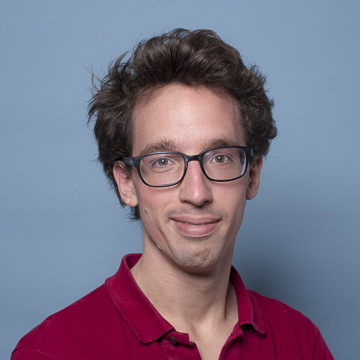
Electrical properties, the conductivity and permittivity of tissue, are quantities that describe the interaction of an object and electromagnetic fields. The properties influence electromagnetic fields and are influenced themselves by physiological phenomena such as lesions or a stroke. Therefore, they are important in identifying or diagnosing the severity of pathologies and are essential in magnetic resonance imaging (MRI) safety and efficiency by determining tissue heating or sensitivity to excitation pulses and antenna designs.
In two-dimensional electromagnetic fields, which occur in specific measurement geometries, it is possible to simplify the relationship between electromagnetic fields and electrical properties, and reconstruct these properties using essentially a forward operation, foregoing a full inversion scheme. These insights also help to find, and explain, the cause of specific artefacts, such as those caused by mismatches in incident field used in the computation of the full electromagnetic fields.
The two-dimensional field assumption necessary for the simplified relationship described above is subsequently tested, and it is shown that this assumption does not hold when the object is sufficiently translation variant in the longitudinal direction. That is, even if the fields for a translation invariant object would be two-dimensional, they become three-dimensional through the interaction of the tissue parameters with the fields, which cause out of plane current and field contributions.
Another interesting application of closed form expressions between currents and fields is the target field method, which solves the inverse source problem between electric currents and static magnetic fields in a regularised manner by constraining their relationship to a cylindrical geometry. This method is adapted for transverse oriented magnetic fields to be used with Halbach type magnet arrays, and an open source tool is developed to make the method easy to apply for various design considerations. Moving away from constraints on the field or current structure, we show the intricate relationship between electrical properties and the measured signal in an MRI scanner. This is done by deriving the electro- (and magneto-) motive force for a typical MRI scenario without any assumptions on the object or electro-magnetic fields. This model can then even be used to reconstruct electrical properties from the simplest MRI signal, namely the free induced decay (FID) signal.
To round off our investigation of electrical properties we take a small detour to the magnetic tissue property, the permeability or magnetic susceptibility. For reconstructing this tissue property a dipole deconvolution is required, where the dipole convolution loses information of the original object through the zeros of the dipole kernel. A new machine learning based approach to reconstruct the lost information is investigated in the final chapter of this thesis.
Additional information ...
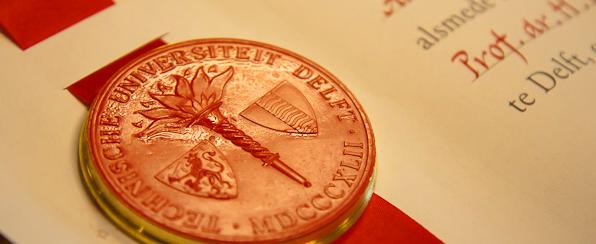
PhD Thesis Defence
- Monday, 21 December 2020
- 14:30-16:00
Integrated Circuits for Miniature 3-D Ultrasound Probes: Solutions for the Interconnection Bottleneck
Zhao Chen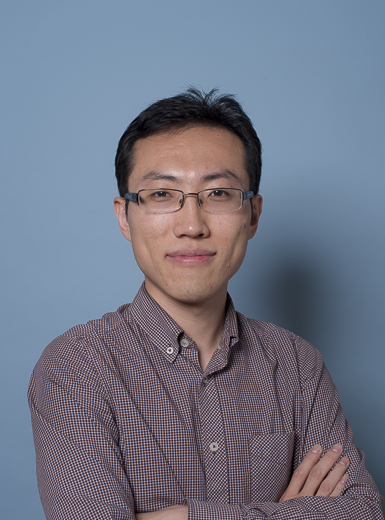
14:30-15:00 (layman’s talk), 15:00-16:00 (defence)
Please feel welcome to join the live stream
Promotors: Michiel Pertijs and Nico de Jong
Abstract: This thesis describes low-power application-specific integrated circuit (ASIC) designs to mitigate the constraint of cable count in miniature 3-D TEE probes. Receive cable-count reduction techniques including subarray beamforming and digital time-division multiplexing (TDM) have been explored and the effectiveness of these techniques has been demonstrated by experimental prototypes. Digital TDM is a reliable technique to reduce cable count, but it requires an in-probe datalink for high-speed data communication. A quantitative study on the impact of the datalink performance on B-mode ultrasound image quality has been introduced in this thesis for data communication in future digitized ultrasound probes. Finally, a high-voltage transmitter prototype has been presented for effective cable-count reduction in transmission while achieving good power efficiency. The application of these techniques is not limited to only the design of TEE probes and can be easily extended to the design of other miniature 3-D ultrasound probes, for instance intracardiac echocardiography (ICE) probes and IVUS probes, which are facing similar interconnect challenges with an increased number of transducer elements to enhance imaging quality.
Additional information ...
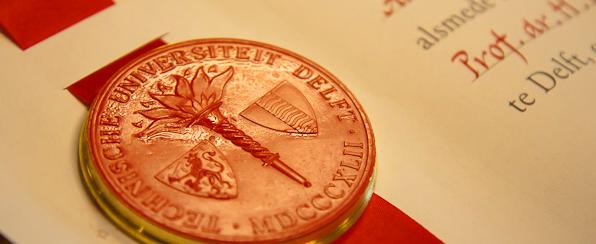
CAS MSc Midterm Presentations
- Thursday, 17 December 2020
- 11:30-12:30
- HB 17.150
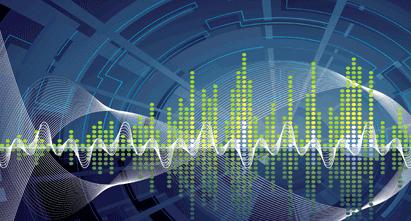
CAS MSc Midterm Presentations
- Thursday, 10 December 2020
- 11:30-12:30
- https://tudelft.zoom.us/j/98866780509
Privacy-Preserving Distributed Graph Filtering
Jane Li
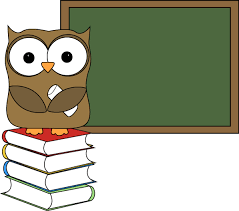
CAS MSc Midterm Presentations
- Thursday, 3 December 2020
- 11:30-12:30
- HB 17.150
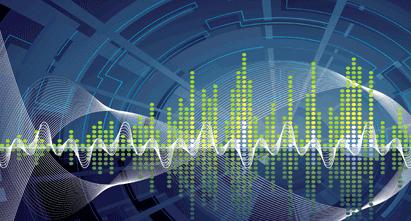
Signal Processing Seminar
- Thursday, 26 November 2020
- 15:30-16:30
- zoom
Focussing Waves in Unknown Media
Jörn Zimmerling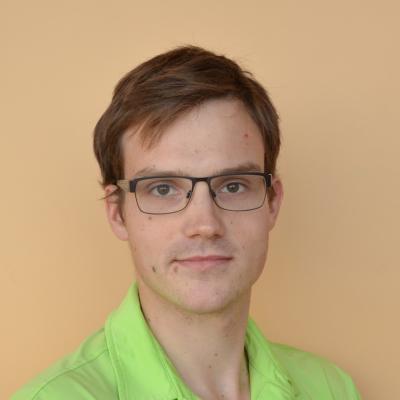
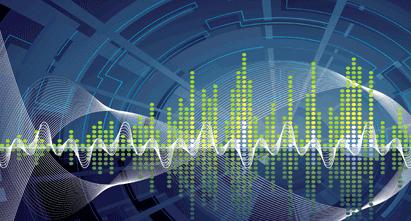
CAS MSc Midterm Presentations
- Thursday, 19 November 2020
- 11:30-12:30
- HB 17.150
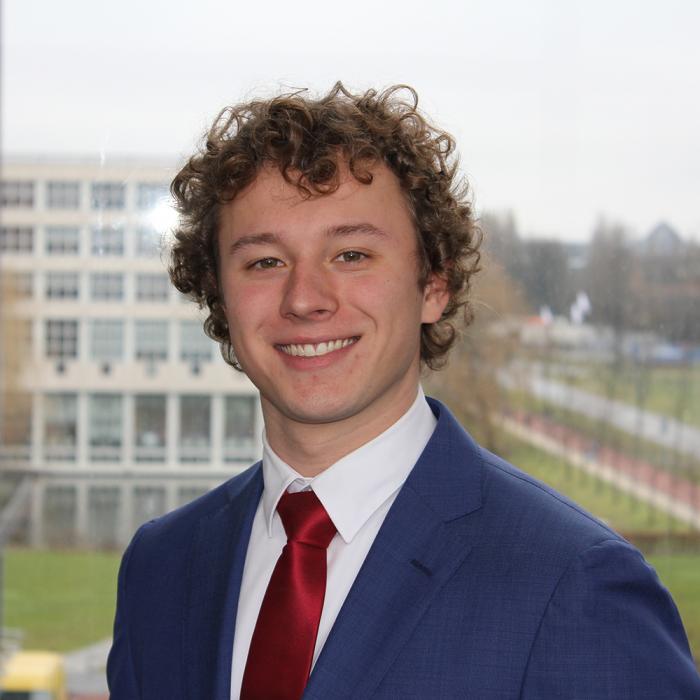
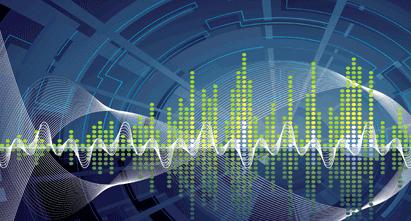
Signal Processing Seminar
- Thursday, 12 -- Thursday, 12 November 2020
- 13:30-14:30
- HB 17.150
Signal Processing for communication
Didem Doğan Başkaya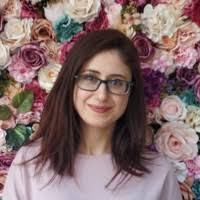
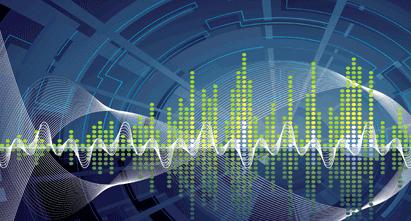
CAS MSc Midterm Presentations
- Thursday, 12 -- Thursday, 12 November 2020
- 13:30-14:30
- HB 17.150
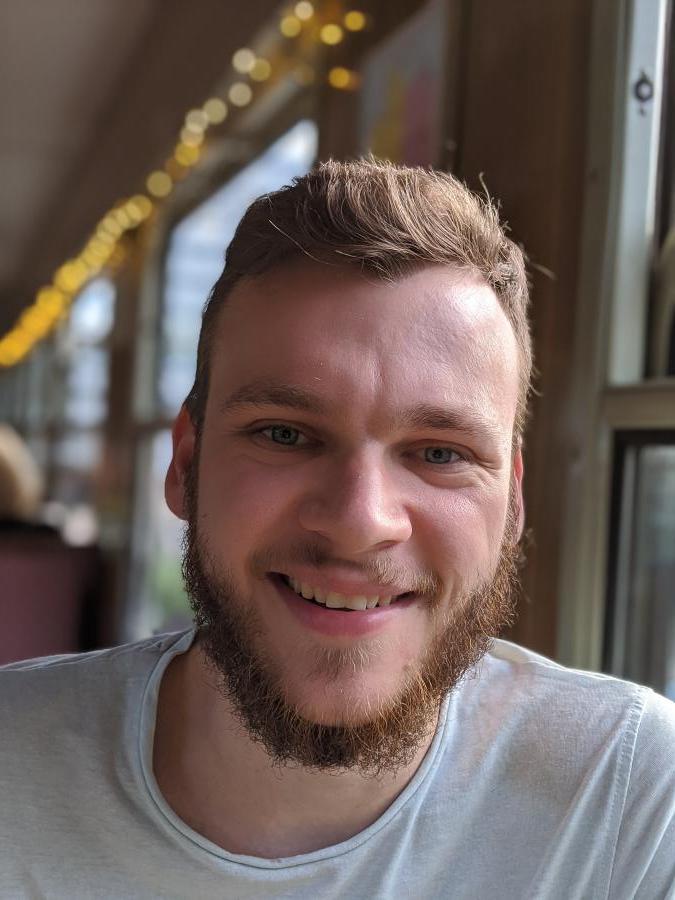
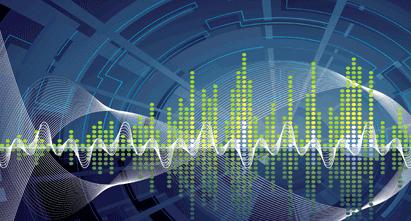
CAS MSc Midterm Presentations
- Thursday, 5 -- Thursday, 5 November 2020
- 13:30-14:30
- HB 17.150
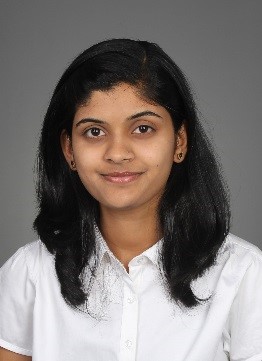
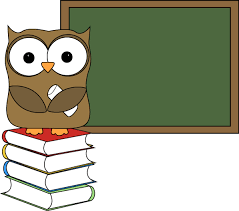
MSc SS Thesis Presentation
- Friday, 30 October 2020
- 08:30-15:00
- zoom
Deep Learning-Based Sound Identification
Shaoqing Chen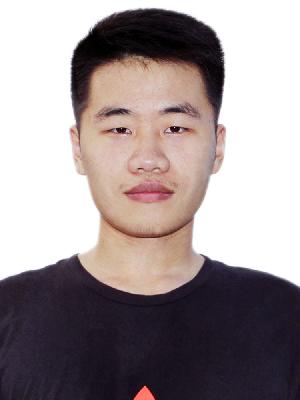
Environmental sound identification and recognition aim to detect sound events within an audio clip. This technology is useful in many real-world applications such as security systems, smart vehicle navigation and surveillance of noise pollution, etc. Research on this topic has received increased attention in recent years. Performance is increasing rapidly as a result of deep learning methods. In this project, our goal is to realize urban sound classification using several neural network models. We select log-Mel spectrogram as the audio representation and use two types of neural networks to perform the classification task. The first is the convolutional neural network (CNN), which is the most straightforward and widely used method for a classification problem. The second type of network is autoencoder based models. This type of model includes the variational autoencoder (VAE), beta-VAE and bounded information rate variational autoencoder (BIR-VAE). The encoders of these systems extract a low dimensionality representation. The classification is then performed on this so-called latent representation. Our experiments assess the performances of different models by evaluation metrics. The results show that CNN is the most promising classifier in our case, autoencoder-based models can successfully reconstruct the log-Mel spectrogram and the latent features learned by encoders are meaningful as classification can be achieved.
Signal Processing Seminar
- Thursday, 29 October 2020
- 13:30-14:30
- https://tudelft.zoom.us/j/91647928860
A Generic Framework and Algorithmic Solution for Radar Resource Management
Max Schöpe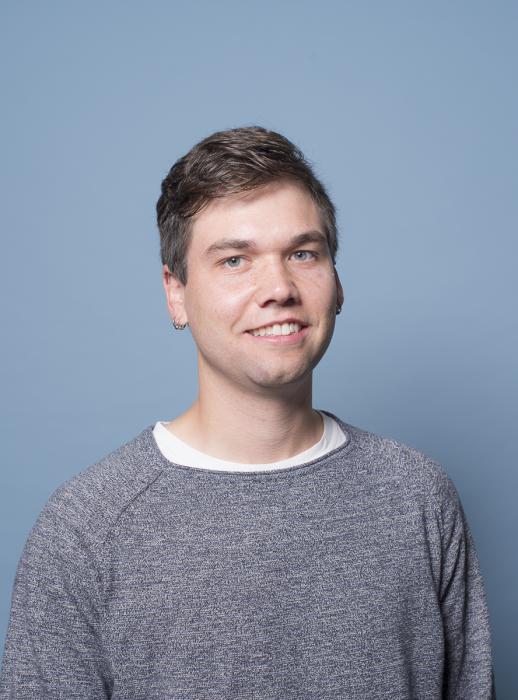
Abstract: Recent advances in multi-function radar (MFR) systems led to an increase of their degrees of freedom. As a result, modern MFR systems are capable to adjust many parameters during run-time. An automatic adaptation of the radar system to changing situations, like weather conditions, interference or target maneuvers is usually called radar resource management (RRM). After an introduction to the topic of RRM, I will discuss our approach. We model the different sensor tasks as partially observable Markov decision processes (POMDP) and solve them by applying a combination of Lagrangian relaxation and policy rollout. The algorithm has a generic architecture and can be applied to different radar or sensor systems and cost functions. I will show this through simulations of two-dimensional tracking scenarios. Moreover, I will demonstrate how the algorithm allocates the sensor time budgets dynamically to a changing environment in a non-myopic fashion.
Additional information ...
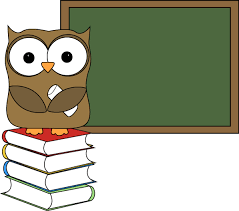
Microelectronics Colloquium
- Thursday, 29 October 2020
- 15:30-16:30
- Online
Artificial Retina: A Future Cellular-Resolution Brain-Machine Interface
Dante Muratore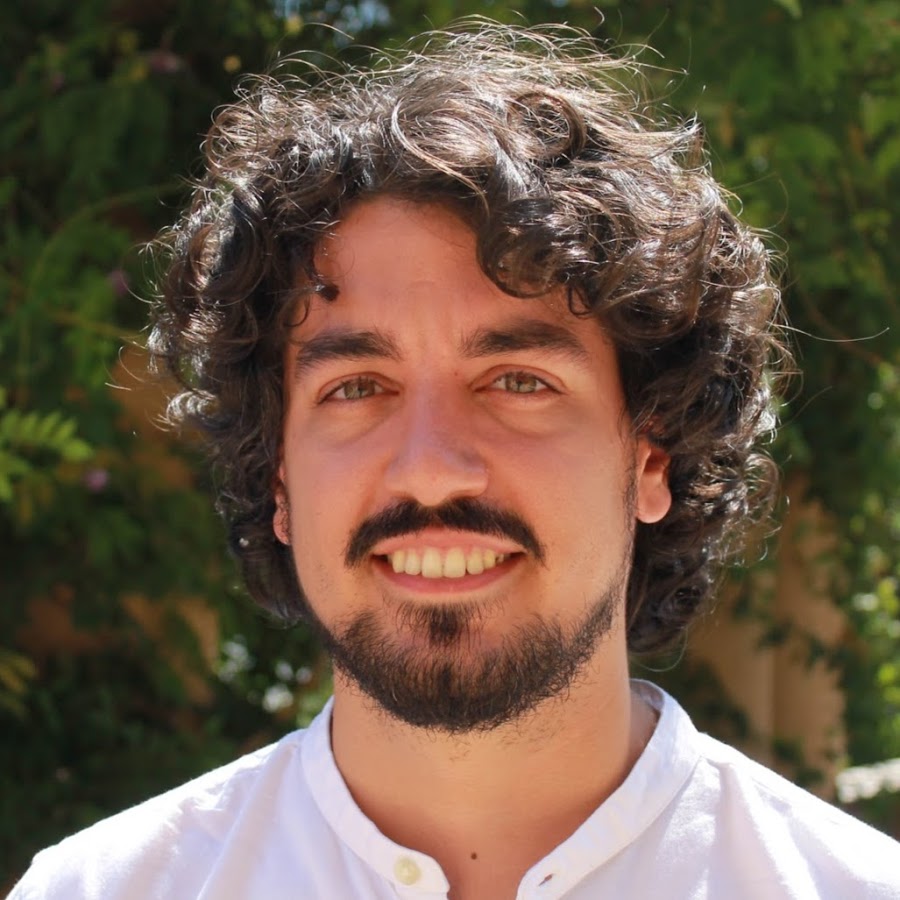
A healthy retina transduces incoming visual stimuli into patterns of neural activity, which are then transmitted to the brain via the optic nerve. Degenerative diseases, like macular degeneration or retinitis pigmentosa, destroy the ability of the retina to transduce light, causing profound blindness. An artificial retina is a device that replaces the function of retinal circuitry lost to disease. Present-day devices can elicit visual percepts in patients, providing a proof of concept. However, the patterns of neural activity they produce are far from natural, and the visual sensations experienced by patients are coarse and of limited use to patients.
A main hurdle is that there are many types of cells in the retina. For example, some cells respond to increases of light intensity, while other cells respond to decreases of light intensity. In order to reproduce a meaningful neural code, it is crucial to respect the specificity and selectivity of these cells. Because cells of different types are intermixed in the circuitry of the retina, cell type specific activation of this kind requires that a future artificial retina be able to stimulate at single cell resolution, over a significant area in the central retina.
To achieve this goal, we are designing an epi-retinal interface that operates in two modes: calibration and runtime. During calibration, the interface learns which cells and which cell types are available for stimulation, by recording neural activity from the retina. During runtime, the interface stimulates the available cells to best approximate the desired scene. I will present a system architecture we are developing that can accomplish the overall performance goals, and the implications of this architecture for brain-machine interfaces.
Additional information ...
PhD Thesis Defence
- Tuesday, 20 October 2020
- 09:30-11:30
- Aula Senaatszaal // zoom
ACCURATE STRUCTURAL HEALTH MONITORING IN COMPOSITES WITH FIBRE BRAGG GRATING SENSORS
Aydin Rajabzadeh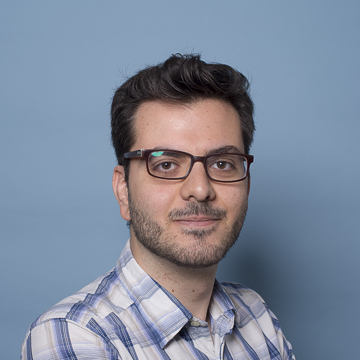
Compared to metals, composite materials offer higher stiffness, more resilience to corrosion, have lighter weights, and their mechanical properties can be tailored by their layup configuration. Despite these features, composite materials are susceptible to a diversity of damages, including matrix cracks, delamination, and fibre breakage. If these damages are not detected and mended, they can spread and result in the failure of the whole structure. In particular, when the structure is under fatigue and vibrations during flight, this process can expedite. Moreover, if such damages occur in the internal layers of the composite material, they will be difficult to detect and to characterise. There is thus a huge demand for reliable and accurate structural health monitoring methods to identify these defects. Such methods either try to monitor the structural integrity of the composite during service, or they are used for studying a desired configuration of a composite material during fatigue and tensile tests. This thesis provides structural health monitoring solutions that can potentially be used for both these categories. The structural health monitoring applications developed in this thesis range from accurate strain and displacement measurement, to detection of cracks and the identification of damages in composites.
In this thesis, fibre Bragg grating (FBG) sensors were chosen for this purpose. The miniature size and small diameter of these sensors makes them an ideal candidate for embedding them between composite layers, without severely altering the mechanical properties of the host composite material. They can thus provide us with direct information about the current state of the laminated composite, potentially at any depth. This is especially useful for acquiring information about the internal layers of the composite material, as barely visible impact damages and micro-cracks often form beneath the surface of the material without being visible on its exterior.
In spite of their interesting physical characteristics, applications of FBG sensors are typically limited to point strain or temperature sensors. Further, it is often assumed that the strain field along the sensor length is uniform. For this reason, there is currently a gap in the field of structural health monitoring in retrieving meaningful information about the non-uniform strain field to which the FBG sensor is subjected in damaged structures. The focus of this thesis is on analysing the response of FBG sensors to highly non-uniform strain fields, which are a characteristic of the existence of damage in composites.
To tackle this problem, first a new model for the analysis of FBG responses to nonuniform strain fields will be presented. Using this model, two algorithms are presented to accurately estimate the average of such non-uniform axial strain fields, which conventional strain estimation algorithms fail to deliver. In fact, it is shown that the state-of-the-art strain estimation methods using FBG sensors can lead to errors of up to a few thousand microstrains, and the presented algorithms in this thesis can compensate for such errors. It was also shown that these methods are robust against spectral noise from the interrogation system, which can pave the way for more affordable FBG based strain estimation solutions.
Another contribution of this thesis is the demonstration of two new algorithms for the detection of matrix cracks, and for accurate monitoring of the delamination growth in composites, using conventional FBG sensors. These algorithms are in particular useful for studying the mechanical behaviour of laminated composites in laboratory setups. For instance, the matrix crack detection algorithm is capable of characterising internal transverse cracks along the FBG length during tensile tests. Along the same lines, the delamination growth monitoring algorithm can accurately localise the delamination crack tip along the FBG length in mode-I tensile and fatigue tests. These algorithms can perform in real-time, which makes them ideal for dynamic measurement of crack propagation under fatigue, and their spatial resolution and accuracy is superior to the other state-of-the-art damage detection techniques.
Finally, to enhance the precision of the damage detection schemes presented in this thesis, two different methods are proposed to accurately determine the active gauge length of the FBG sensor, and its position along the optical fibre. This information is generally not provided for commercial FBG sensors with such accuracy, which can adversely affect the precision of crack tip localisation algorithms. Following the algorithms provided in this thesis, the sensor position can be marked on the optical fibre with micrometer accuracy.
Additional information ...
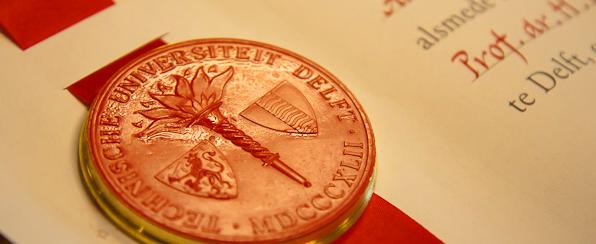
Signal Processing Seminar
- Thursday, 15 October 2020
- 13:30-14:30
- HB 17.150
Signal processing in distributed networks; audio signal processing
Metin Çalış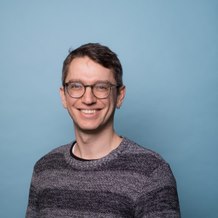
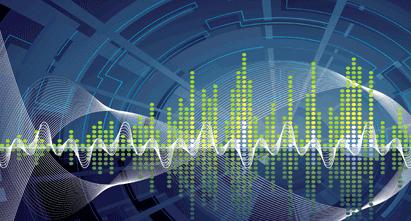
CAS MSc Midterm Presentations
- Thursday, 15 October 2020
- 13:30-13:50
- HB 17.150 / zoom
Tracking of rodents from infrared video sequences for behaviour studies
Javier Guinea Perez
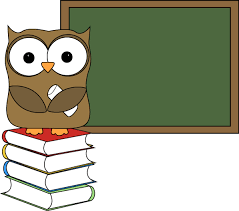
Signal Processing Seminar
- Thursday, 8 October 2020
- 13:30-14:30
- HB 17.150
Biomedical signal processing
Aybüke Erol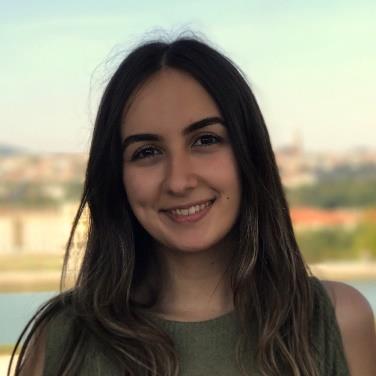
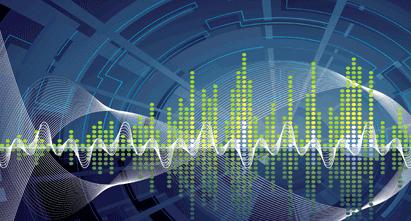
Signal Processing Seminar
- Thursday, 1 October 2020
- 13:30-14:30
- HB 17.150
Intelligent X-ray sensing for real-time image guidance in proton therapy
Dennis SchaartApplied Physics, TU Delft
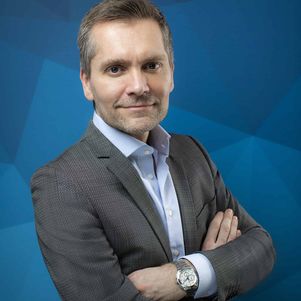
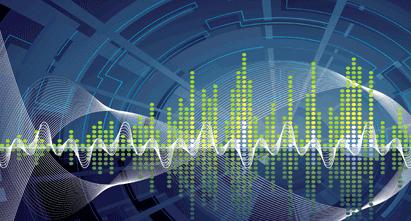
MSc SS Thesis Presentation
- Tuesday, 22 September 2020
- 09:30-10:15
- zoom
Distributed Coordination for Multi-feet Truck Platooning
Yikai ZengTruck platooning refers to coordinating a group of heavy-duty vehicles at a close inter-vehicle distance to reduce overall fuel consumption. This coordination between trucks is traditionally achieved by adjusting the schedule, velocity and routines to increase the platooning chances, and thus improve the overall fuel efficiency. However, the data model built for the coordination problem is typically integer-constrained, making it generally hard to solve. On the other hand, the interaction among self-interested fleets which are operated by different companies is not well-studied. This thesis aims to build a distributed framework for multi-fleet truck platooning coordination to enable the coordination without a third-party service provider.
The interaction among fleets is considered a non-cooperative finite game, for which we propose the best response search method, which essentially requires to solve a cooperative truck platooning optimization problem iteratively. We refer to the optimization problem as a best-response problem, which is formulated as a mixed-integer linear problem with relaxation skills.
To achieve a feasible time complexity for the best-response subproblem, we propose a decentralized algorithm, distributing the computational load to connected automated vehicles within the fleet.
The proposed method is examined under a real-world featured demand set to compare the performance in optimality and time complexity with previous studies. The result suggests that the decentralized algorithm delivers the optimal objective value in this case, while the best-response search does not deliver extra benefits as a the dominating time costs in the cost functions eliminate the potential for improvement.
MSc SS Thesis Presentation
- Monday, 21 September 2020
- 14:00-14:45
- zoom
Atrial Fibrillation classification from a short single lead ECG recordin
Yuchen Yin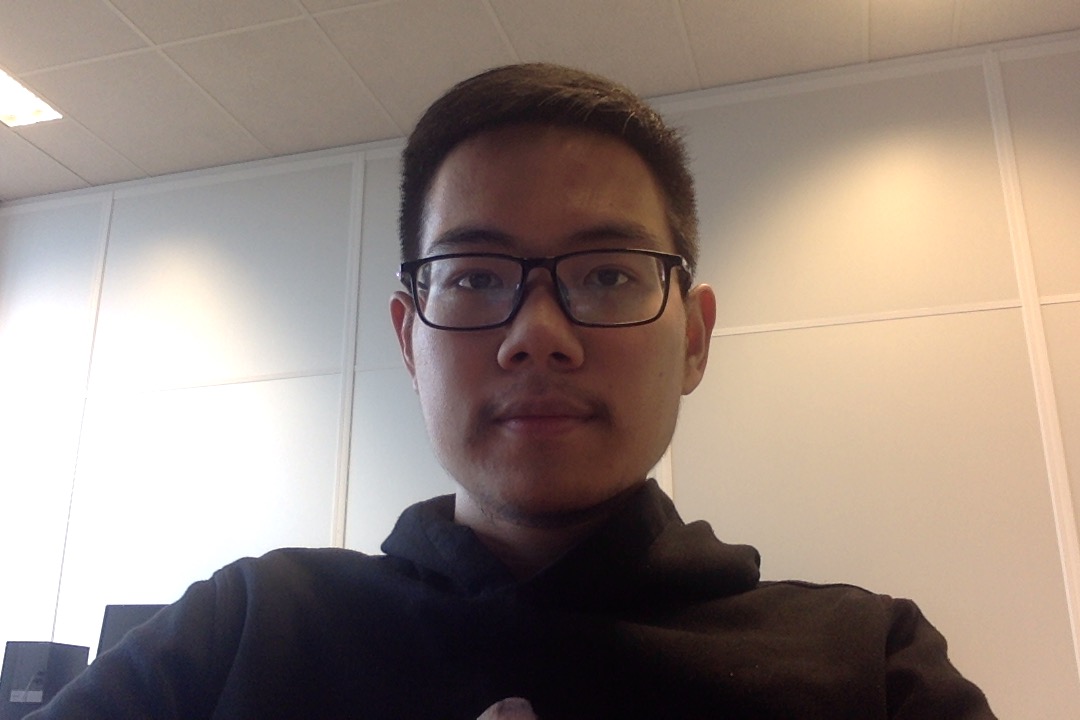
This thesis focuses on classifying AF and Normal rhythm ECG recordings. AF is a common arrhythmia occurring in millions of people every year, which could lead to blood clots, stroke or even heart failure. When AF is occurring, the P waves are often absent and RR intervals are often irregular.
This thesis proposes a new Poincaré plot based feature that exploits the distribution and position information of the plot. The Poincaré plot can visually analyze the nonlinear aspects of the heart rate dynamics both qualitatively and quantitatively. In this thesis, the Poincaré plot values are first quantized into small bins, which represent whether corresponding states are visited by the system or not, by setting ones or zeros. The bins are then given weights by the masks based on the probability of each state being visited by the system, and the relative position between the bins and the center of the plot. By calculating the element-wise multiplication and summation between the quantized Poincaré plot and the masks, the expected value of the matrix of the quantized Poincaré plot is computed, and the outliers in the plot are emphasized. Therefore, the proposed feature is assumed to have a higher value for the AF rhythms and a lower value for the Normal rhythm.
Instead of RR intervals, the Poincaré plot used in this thesis is also generated from the peak intervals in the autocorrelation function of both ECG and prediction error. The autocorrelation function aims to evaluate the self-similarity of the ECG signals and thus extracts the irregularity of the AF signals.
The dataset used in this thesis comes from the Physionet Challenge 2017, containing 5076 Normal recordings and 758 AF recordings. In total, 21 Poincaré plot based features are used to train the SVM and random forest models, which yields the F1 score of 0.80 and 0.85, respectively. When using features from the same intervals, RR intervals generate the highest F1 score of 0.77 and 0.81, followed by the peak intervals in the autocorrelation of prediction error with the F1 score of 0.74 and 0.78, followed by the peak intervals in the autocorrelation error of ECG with the F1 score of 0.63 and 0.68. Using the minimum redundancy maximum relevance algorithm, eleven features are selected based on their importance. Training the SVM and RF models with these features reaches the F1 score of 0.78 and 0.84, respectively.
Additional information ...
MSc SS Thesis Presentation
- Thursday, 10 September 2020
- 10:00-10:40
- zoom
Clock skew invariant beamforming
Laurens Buijs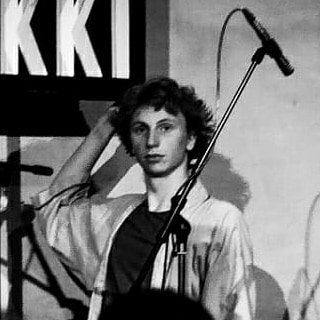
This thesis is focused on Wireless Acoustic Sensor Networks (WASNs) used for beamforming in a speech enhancement task. Since each node in aWASN has its own clock, clock osets and clock skews between the nodes are inevitable. Clock osets and clock skew can be detrimental to the beamformer performance. In this thesis we focus on the eect of clock skew on the beamformer performance. Existing methods for clock skew compensation for the speech enhancement application do this explicitly. In this thesis we investigate the possibility to formulate the beamformer such that explicit clock skew compensation is not necessary.
Instead, we propose an algorithm for implicit clock skew compensation, which takes advantage of the Generalized Eigenvalue Decomposition (GEVD) to construct beamformers (e.g. Minimum Variance Distortionless Response (MVDR)), recently proposed in the literature. Using the GEVD, no explicit compensation has to be applied to the received data. Compared to the state-of-the-art, where clock skew estimation/compensation algorithms are used, this reduces the computational complexity for beamformer processing.
The algorithm depends on exact knowledge of the noisy correlation matrix across the microphones. In practice, this matrix is unknown and estimation will reduce the performance of the proposed algorithm. We therefore quantify the error made in the estimation of the correlation matrix using the standard Welch method and also look at a recursive smoothing based method for correlation matrix estimation. Compared to a selected state-of-the-art algorithm, the proposed algorithm shows similar or better performance using this recursive smoothing method. For future work on this subject, more study can be done on correlation matrix estimation methods, as these play a key role in clock skew invariant beamforming.
Additional information ...
Signal Processing Seminar
- Thursday, 10 September 2020
- 13:30-14:30
- HB 17.150
Biomedial Signal Processing
Hanie Moghaddasi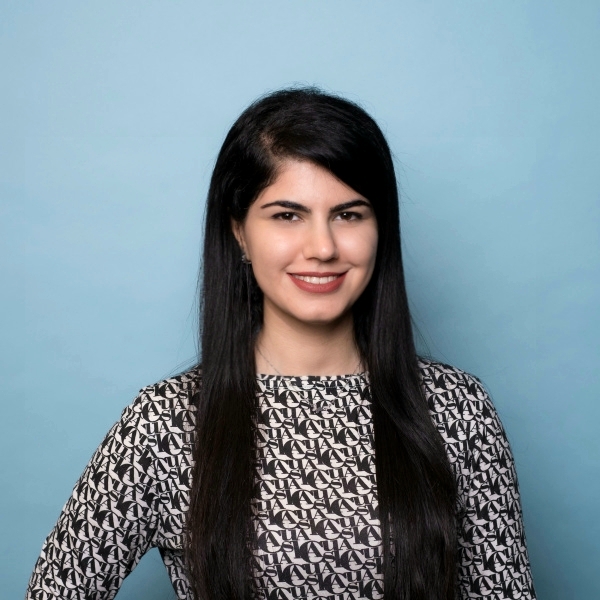
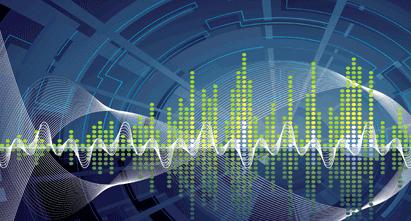
MSc SS Thesis Presentation
- Wednesday, 9 September 2020
- 15:00-15:40
- zoom
Ultra fast MRI acquisition at 7 Tesla
Alejandro Monreal Madrigal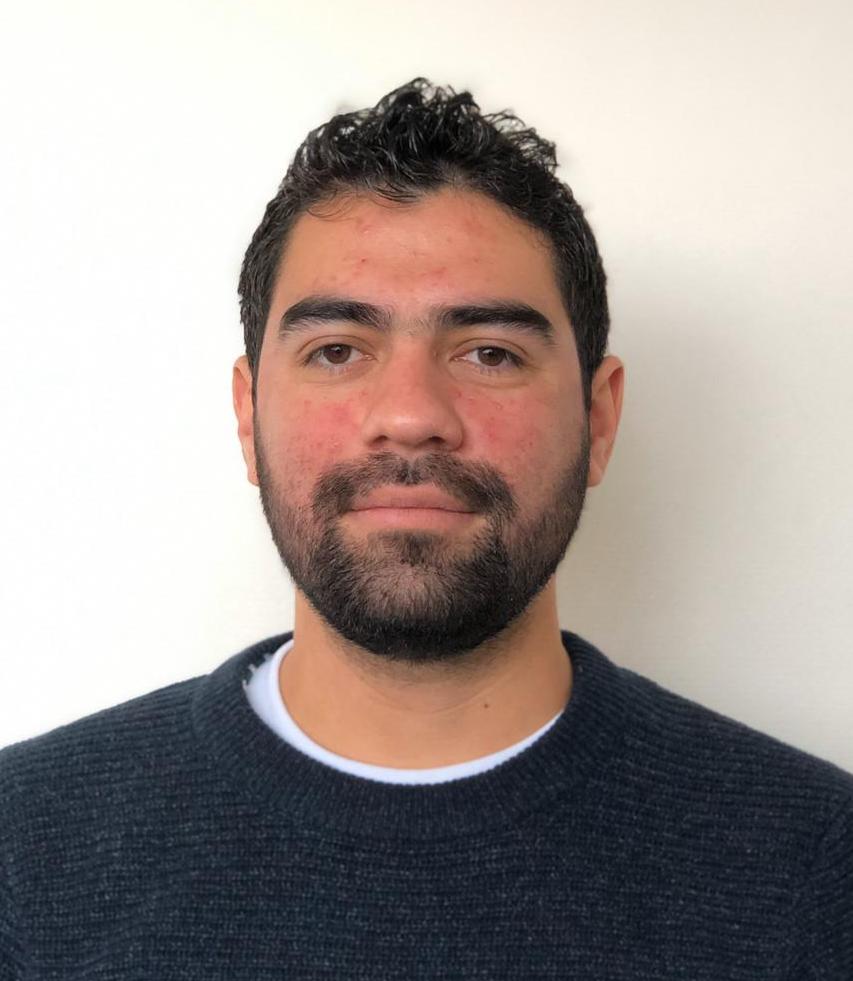
Magnetic Resonance Imaging is one of the most widely used imaging modalities nowadays and it performs especially well imaging human organs such as the brain and liver. One of its main limitations is the relatively long imaging times, to overcome this issue and speed up the data acquisition, several techniques such as Parallel Imaging or PI have been developed. These techniques require advanced hardware and software to be able to decrease the acquisition time. On the hardware side, a highly efficient insert gradient coil has been designed and built at the University Medical Center Utrecht. Specialized software has to be implemented to optimally make use of this hardware. One of the recently proposed PI methods called Wave-CAIPI has been proved to achieve a ninth fold acceleration factor without compromising image quality.
This project aims to investigate the time gain that can be achieved when combing the insert gradient coil with a Wave-CAIPI strategy. Two main aspects are reviewed. The first one is the maximum achievable under-sampling factor that does not compromise image quality. The second one is the decrease in acquisition time that can be obtained when using the insert gradient coil compared to conventional gradient systems while maintaining image quality. To do so, the strategy has been implemented and extensive simulations have been performed to optimize the MR acquisition parameters. To prove the results from the simulations, the Wave-CAIPI sequence was implemented in a 7T scanner at the UMCU, where the acquired data was retrospectively under-sampled, obtaining the wave image to be further reconstructed.
MSc SS Thesis Presentation
- Friday, 28 August 2020
- 14:30-15:15
- zoom
Automatic Depth Matching for Petrophysical Borehole Logs
Aitor García Manso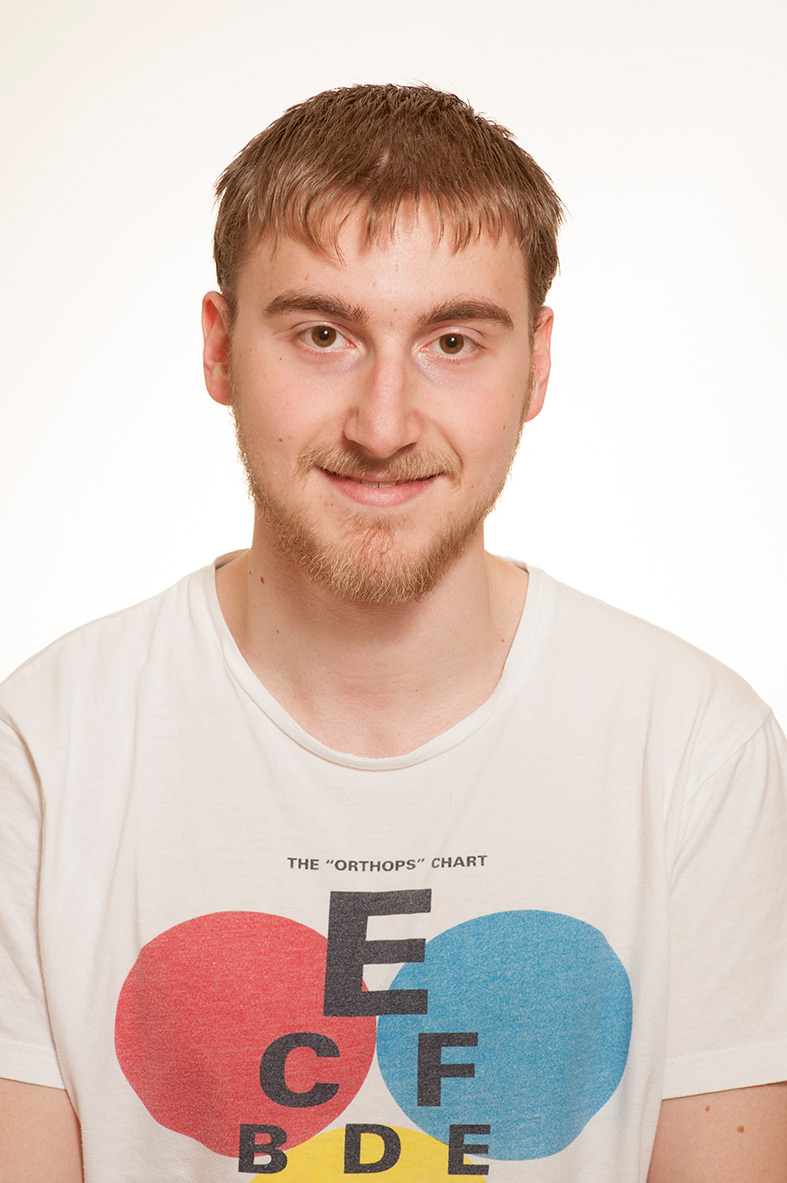
In the oil and gas industry a crucial step for detecting and developing natural resources is to drill wells and measure miscellaneous properties along the well depth. One important source of this disturbances is depth misalignment and in order to compare different measurements care must be taken to ensure that all measurements (log curves) are properly positioned in depth. This process is called depth matching. In spite of multiple attempts for automating this process it is still mostly done manually.
Based on the Parametric Time Warping (PTW), a parameterised warping function that warps one of the curves is assumed and its parameters are determined by solving an optimization problem maximizing the cross-correlation between the two curves. The warping function is assumed to have the parametric form of a piecewise linear function in order to accommodate the linear shifts that take place during the measurement process. This method, combined with preprocessing techniques such as an offset correction and low pass filtering, gives a robust solution and can correctly align the most commonly accruing examples. Furthermore, the methodology is extended to depth match logs with severe distortion by applying the technique in an iterative fashion. Several examples are given when developed algorithm is tested on real log data supplemented with the analysis of the computational complexity this method has and the scalability to larger data sets.
MSc SS Thesis Presentation
- Thursday, 27 August 2020
- 14:00-14:40
- zoom
Optimal Sensor Placement for Calibration-Involved Radio Astronomy Imaging Applications
Kaiwen ZhangIn radio astronomy (RA), one of the key tasks is the estimation of the celestial source powers, i.e. imaging. To maximize the performance, it is crucial to optimize the receiver locations before the construction of a telescope array. However, although system calibration is an integral and crucial process of imaging, it has rarely been addressed for RA sensor placement problems previously. This motivates us to investigate whether incorporating calibration can result in better array designs.
In this thesis, we focus on the calibration of the sensors’ complex-scalar gains in particular, which are treated as nuisance parameters for the image estimation. The associated Cramer-Rao bound (CRB) is derived and employed as the design criterion. The nonlinear CRB-based sensor placement problem is cast as an NP-hard combinatorial optimization problem, and we adopt two approaches to solve such by approximation: (i) greedy submodular maximization and (ii) convex optimization with semidefinite relaxation. The former is chosen for simulations due to its good performance and lower computational complexity. Extensive simulations demonstrate that compared to the calibration-excluded design, the proposed one only provides slight improvements to the imaging quality. However, the proposed array demonstrates the potential of accelerating the convergence of the gain estimation procedures. Through further investigation, we conclude that the lack of imaging quality improvident can be a consequence of the gain and image being near-orthogonal parameters.
PhD Thesis Defence
- Wednesday, 26 August 2020
- 14:30-16:30
- Aula Senaatszaal
Antenna Array Synthesis and Beamforming for 5G Applications: An Interdisciplinary Approach
Yanki Aslan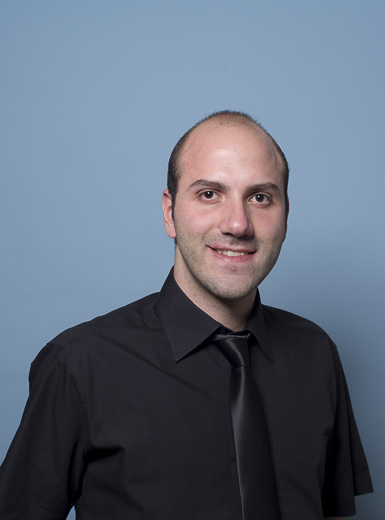
Realization of the future 5G systems requires the design of novel mm-wave base station antenna systems that are capable of generating multiple beams with low mutual interference, while serving multiple users simultaneously using the same frequency band. Besides, small wavelengths and high packaging densities of front-ends lead to overheating of such systems, which prevents safe and reliable operation. Since the strict cost and energy requirements of the first phase 5G systems favor the use of low complexity beamforming architectures, computationally efficient signal processing techniques and fully passive cooling strategies, it is a major challenge for the antenna community to design multibeam antenna topologies and front-ends with enhanced spatial multiplexing, limited inter-beam interference, acceptable implementation complexity, suitable processing burden, and natural-only/radiative cooling.Traditionally, array design has been performed based on satisfying the given criteria solely on the radiation patterns (gain, side lobe level (SLL), beamwidth etc.). However, in addition to the electromagnetic aspects, multi-beam antenna synthesis and performance evaluation in 5G systems at mm-waves must combine different disciplines, including but not limited to, signal processing, front-end circuitry design, thermal management, channel & propagation, and medium access control aspects. Considering the interdisciplinary nature of the problem, the main objective of this research is to develop, evaluate and verify innovative multibeam array techniques and solutions for 5G base station antennas, not yet used nor proposed for mobile communications. The research topics include the investigation of (i) new array topologies, compatible with IC passive cooling, including sparse, space tapered arrays and optimized subarrays, meeting key requirements of 3-D multi-user coverage with frequency re-use and power-efficient side-lobe control, (ii) adaptive multiple beamforming strategies and digital signal processing algorithms, tailored to these new topologies, and (iii) lowcost/competitive and sufficiently generic implementation of the above array topologies and multi-beam generation concepts to serve multiple users with the same antenna(s) with the best spectrum and power efficiencies. This doctoral thesis consists of three parts. Part I focuses on the system-driven aspects which cover the system modeling (including the link budget and precoding), propagation in mm-wave channels and statistical assessment of the Quality of Service (QoS). Although separate comprehensive studies exist both in the field of propagation/system modeling and antennas/beamforming, the link between the two disciplines is still weak. In this part, the aim of the study is to bridge the gap between the two domains and to identify the trade-offs between the complexity of beamforming, the QoS, and the computational cost of precoding in the 5G multi-beam base station arrays for various use cases. Based on the system model developed, a novel quantitative relation between the antenna SLLs/pattern nulls and the statistical QoS is established in a line-of-sight (LoS) dominated mm-wave propagation scenario. Moreover, the potential of using smart (low in-sector side-lobe) array layouts (with simple beam steering) in obtaining sufficiently high and robust QoS, while achieving the optimally low processing costs is highlighted. For a possible pure non-line-of-sight (NLoS) scenario, the system advantages (in terms of the beamforming complexity and the interference level) of creating a single, directive beam towards the strongest multipath component of a user are explained via ray-tracing based propagation simulations. The insightful system observations from Part I lead to several fundamental research questions: Could we simplify the multiple beamforming architecture while keeping a satisfying QoS? Are there any efficient yet effective alternative interference suppression methods to further improve the QoS? How should we deal with the large heat generation at the base station? These questions, together with the research objectives, form the basis for the studies performed in the remaining parts. Part II of the thesis focuses on the electromagnetism-driven aspects which include innovative, low-complexity subarray based multibeam architectures and new array optimization strategies for effective SLL suppression. The currently proposed multi-beam 5G base stations in the literature for beamforming complexity reduction use either a hybrid array of phased subarrays, which limits the field-of-view significantly, or employ a fully-connected analog structure, which increases the hardware requirements remarkably. Therefore, in the first half of this part, the aim is to design low-complexity hybrid (or hybrid-like) multiple beamforming topologies with a wide angular coverage. For this purpose, two new subarray based multiple beamforming concepts are proposed: (i) a hybrid array of active multiport subarrays with several digitally controlled Butler Matrix beams and (ii) an array of cosecant subarrays with a fixed cosecant shaped beam in elevation and digital beamforming in azimuth. Using the active (but not phased) multiport subarrays, the angular sector coverage is widened as compared to that of a hybrid array of phased subarrays, the system complexity is decreased as compared to that of a hybrid structure with a fully-connected analog network, and the effort in digital signal processing is reduced greatly. The cosecant subarray beamforming, on the other hand, is shown to be extremely efficient in serving multiple simultaneous co-frequency users in the case of a fairness-motivated LoS communication thanks to its low complexity and power equalization capability. Another critical issue with the currently proposed 5G antennas is the large inter-user interference caused by the high average SLL of the regular, periodic arrays. Therefore, in the second half of Part II, the aim is to develop computationally and power-efficient SLL suppression techniques that are compatible with the 5G’s multibeam nature in a wide angular sector. To achieve this, two novel techniques (based on iterative parameter perturbations) are proposed: (i) a phase-only control technique and (ii) a position-only control technique. The phase-only technique provides peak SLL minimization and simultaneous pattern nulling, which is more effective than the available phase tapering methods in the literature. The position-only technique, on the other hand, yields uniform-amplitude, (fully-aperiodic and quasi-modular) irregular planar phased arrays with simultaneous multibeam optimization. The latter technique combines interference-awareness (via multibeam SLL minimization in a predefined cell sector) and thermal-awareness (via uniform amplitudes and minimum element spacing constraint) for the first time in an efficient and easy-to-solve optimization algorithm. Part III of the thesis concentrates on the thermal-driven aspects which cover the thermal system modeling of electronics, passive cooling at the base stations, and the role of antenna researchers in array cooling. The major aim here is to form a novel connection between the antenna system design and thermal management, which is not yet widely discussed in the literature. In this part, an efficient thermal system model is developed to perform the thermal simulations. To effectively address the challenge of thermal management at the base stations, fanless CPU heatsinks are exploited for the first time for fully-passive and low-cost cooling of the active integrated antennas. To reduce the size of the heatsinks and ease the thermal problem, novel planar antenna design methodologies are also proposed. In the case of having a low thermal conductivity board, using a sparse irregular antenna array with a large inter-element spacing (such as a sunflower array) is suggested. Alternatively, for the densely packed arrays, increasing the equivalent substrate conductivity by using thick ground planes and simultaneously enlarging the substrate dimensions is proven to be useful. The performed research presents the first-ever irregular/sparse and subarray based antennas with wide scan multi-beam capability, low temperature, high-efficiency power amplifiers, and low level of side lobes. The developed antenna arrays and beam generation concepts could have also an impact over a broad range of applications where they should help overcome the capacity problem by use of multiple adaptive antennas, improve reliability and reduce interference.
Additional information ...
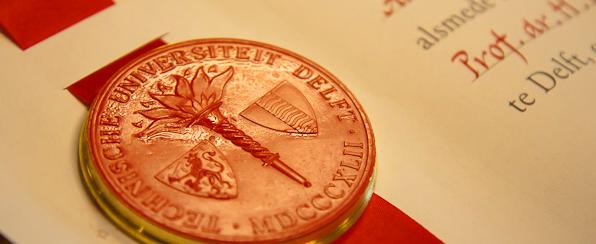
MSc SS Thesis Presentation
- Wednesday, 26 August 2020
- 10:00-10:40
- skype
Low-field MR Imaging Using a Nonuniform Fast Fourier Transform
Maria Macarulla Rodriguez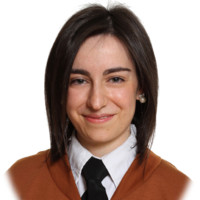
Low-field Magnetic Resonance Imaging (LF MRI) is a cheap and safe technique to visualise the internal structure of the human body. Unlike other imaging techniques, Magnetic Resonance Imaging does not use ionising radiation to generate the images. Instead, it uses magnetic fields and radio waves which are nonthreatening to the health. The LF MRI scanners are constructed out of inexpensive materials and their maintenance is affordable. Therefore, these scanners are a promising alternative for developing countries that present economic limitations. Nonetheless, since Magnetic Resonance scanners use a weak magnetic field, the process of image reconstruction requires complex algorithms that need time. This thesis will examine the way in which the computational time of the image reconstruction from a low-field Magnetic Resonance Imaging can be reduced, using an algorithm based on the fast Fourier transform.
SSCS WYE Webinar
- Friday, 7 -- Friday, 7 August 2020
- 17:00-18:00
To Academia, or to Industry, That is the Question.
Kofi Makinwa, Shin-Lien Lu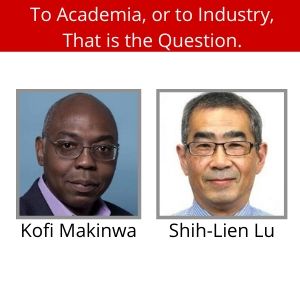
Abstract:
You are about to finish graduate school or perhaps a young or seasoned professional, contemplating a career transition. Which is better - a career in academia or industry? What are the pros and cons of one versus the other? How can you start exploring and build up your career accordingly? In this webinar, we will interview Dr. Linus Lu, a professor-turned-industry veteran, and Prof. Kofi Makinwa, an industry veteran-turned-professor, who will share their insights and perspectives from their personal journeys in both academia and industry careers. They will also address what triggered their transitions, how they staged their transitions, and offer their crystal ball projections on present and future career prospects in the solid-state-circuits profession. Additional information ...
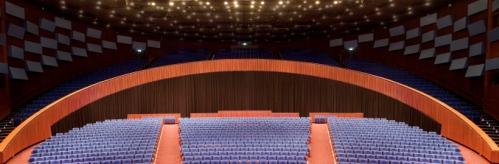
MSc SS Thesis Presentation
- Tuesday, 28 July 2020
- 14:00-14:40
- (zoom)
Multi-target Detection and Tracking with 8 GHz FMCW Radar System
Siyan Wan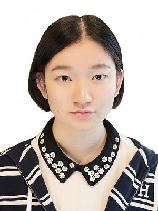
Currently, most of FMCW radar systems for target detection and localization are based on the radar system with multiple receiving antennas, but little based on the SISO system. In this project, we will show a unique signal processing pipeline based on the 8 GHz SISO FMCW radar system. An advanced algorithm of multi-target detection and tracking will be designed to monitor the range, angle, and Doppler velocity information of targets.
Signal Processing Seminar
- Thursday, 25 June 2020
- 13:30-14:30
- HB 17.150
sensor networks, rank-constrained optimization, algebraic techniques
Matthew Morency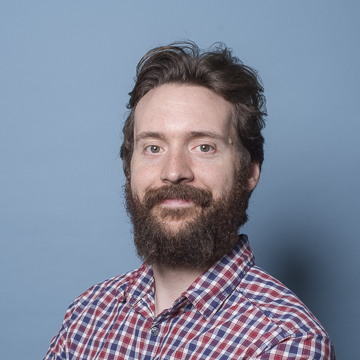
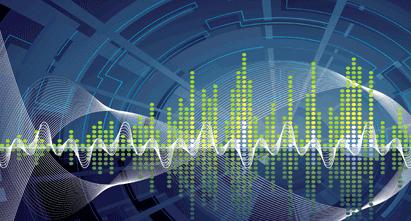
Signal Processing Seminar
- Thursday, 11 June 2020
- 13:30-14:30
- HB 17.150
Second Master presentation
Maria Macarulla Rodriguez, Aitor García Manso
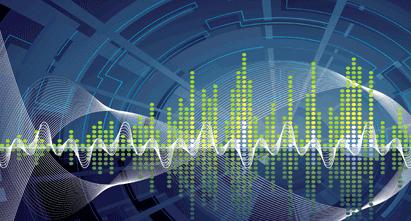
Signal Processing Seminar
- Thursday, 4 June 2020
- 13:30-14:30
- HB 17.150
Task-cognizant sparse sensing for inference (ASPIRE)
Pim van der Meulen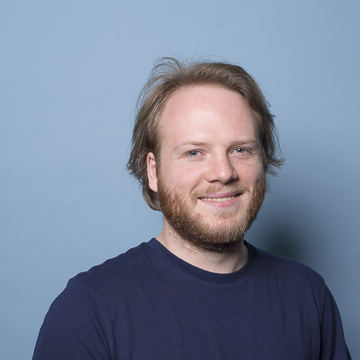
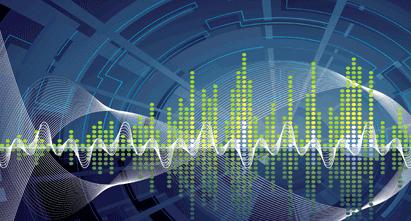
Signal Processing Seminar
- Thursday, 28 May 2020
- 13:30-14:30
- HB 17.150
Second Master presentation
Vasudha Sathyapriyan, Maarten Enthoven
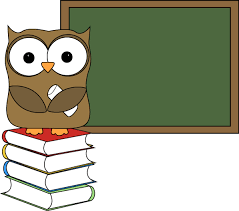
Signal Processing Seminar
- Thursday, 14 May 2020
- 13:30-14:30
- HB 17.150
Second Master presentation
Kriti Dhingra, Prernna Bhatnagar
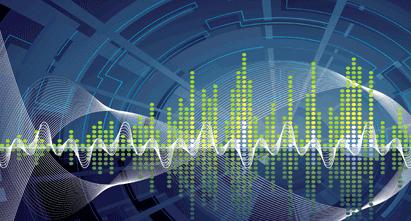
Signal Processing Seminar
- Thursday, 7 May 2020
- 13:30-14:30
- HB 17.150
Signal Processing for Communication
Zengfu Wang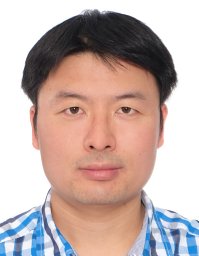
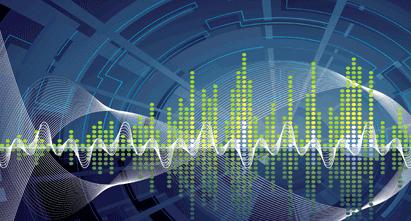
Signal Processing Seminar
- Thursday, 30 April 2020
- 13:30-13:45
- HB 17.150
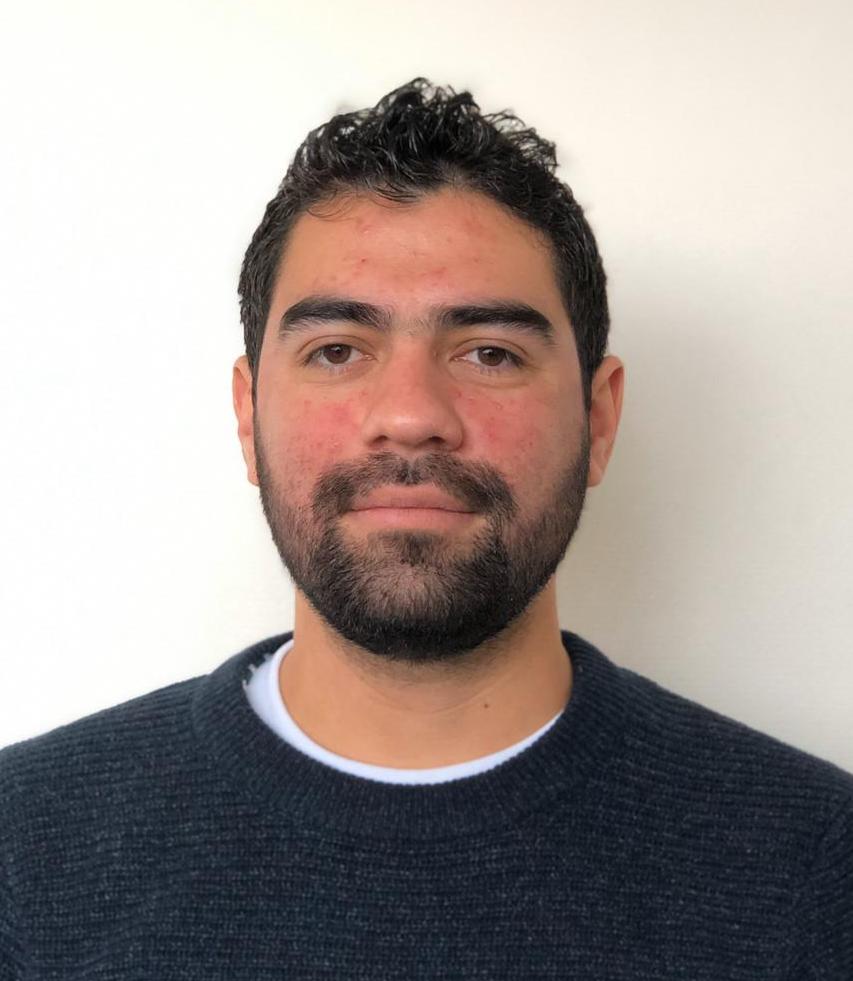
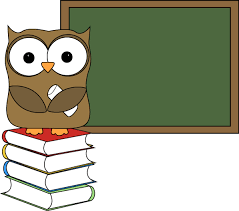
Signal Processing Seminar
- Thursday, 23 April 2020
- 13:30-14:30
- Zoom
Second Master Presentation
Yuchen Yin, Shaoqing Chen
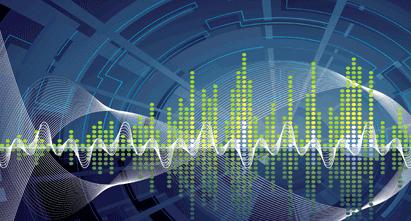
MSc SS Thesis Presentation
- Friday, 6 March 2020
- 10:00-10:45
- EWI, Elektron HB 01.230
Room geometry estimation from stereo recordings using neural networks
Giovanni Bologni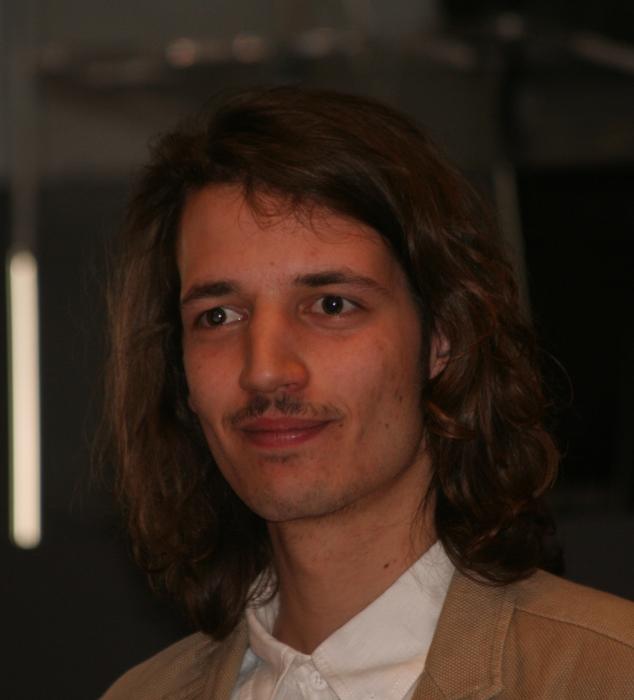
Acoustic room geometry estimation is often performed in ad hoc settings, i.e. using multiple microphones and sources distributed around the room, or assuming control over the excitation signals. To facilitate practical applications, we propose a fully convolutional network (FCN) that localizes reflective surfaces under milder assumptions, such as 1. a compact array of only two microphones is available, 2. emitter and receivers are not synchronized, and 3., both the excitation signals and the impulse responses of the enclosures are unknown.
Our FCN is designed to extract spectral and temporal patterns from stereo recordings, aggregate the temporal information over time-frames, and predict the likelihood of virtual sources corresponding to reflective surfaces being at specific locations.
Numerical experiments confirm that the network is able to generalize to mismatched microphone array sizes, sensor directivity patterns, or audio signal types, while highlighting front-back ambiguity as a prominent source of uncertainty.
When a single reflective surface is present, up to 80% of the sources are detected, while this figure approaches 50% in rectangular rooms.
Further tests on real-world recordings report similar accuracy as with artificially reverberated speech signals, validating the generalization capabilities of the framework.
Additional information ...
Signal Processing Seminar
- Thursday, 5 March 2020
- 13:30-14:30
- HB 17.150
Graph Signal Processing
Alberto Natali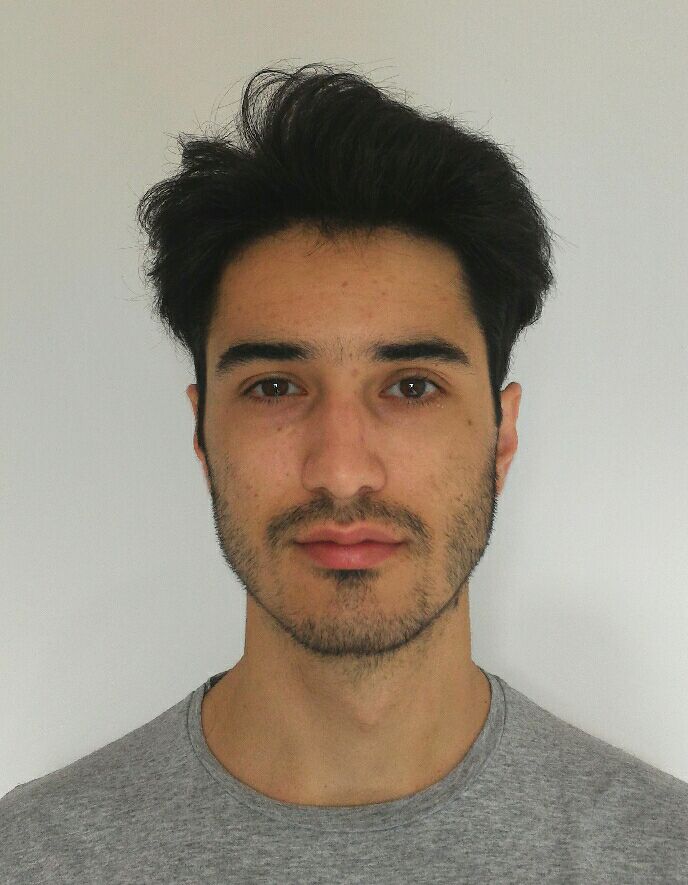
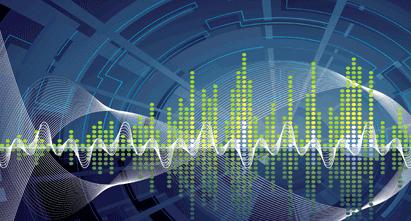
MSc SS Thesis Presentation
- Friday, 28 February 2020
- 12:00-12:45
- HB 17.150
Single-Pixel Thermopile Sensors for people counting
Erik Hagenaars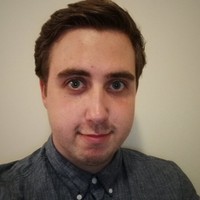
People counting data in offices is used in many applications like HVAC system control and space management to increase comfort, decrease energy consumption and optimise space utilisation. In contrast to past approaches using imaging modalities that tend to be either expensive or intrusive, we consider single-pixel thermopile sensors for people counting. These sensors may already be deployed as part of a smart lighting system to provide temperature data for HVAC controls.
Firstly, a statistical sensor model for thermopile temperature measurements is proposed. The proposed people counting method enhances the CUSUM RLS algorithm to estimate temperature change caused by people entering or leaving. We estimate mean temperature changes upon detection of an occupancy event, and then estimate the people count using a maximum likelihood on the estimated temperature change. Finally, PIR vacancy data is merged with the people count estimation to increase accuracy. We obtain an average counting error of 0.11 and 0.19 for 90\% of the instants respectively when considering 15 minute windows for simulated and experimental datasets.
A second aspect of the thesis considers the problem of commissioning plan detection. We leverage the two-sided CUSUM signals to address this problem. The two-sided CUSUM scores for a pair of sensors are used to calculate similarity measures; these features are used in a Random Forest Classifier to detect commissioning changes of the sensor pair. Using simulated data with the thermopile signal model, we show that the proposed method achieves a true positive rate (determining the correct layout) of 90.2\% and false positive rate of 1.3\%.
Additional information ...
Microelectronics colloquium
- Thursday, 27 February 2020
- 15:30-17:00
- EEMCS, Restaurantzaal
Radar; Compressive Sensing, Information Geometry and Neural Networks in Radar /Radar – Old but gold
Radmila Pribić (Thales/TUD), Francesco Fioranelli (TUD)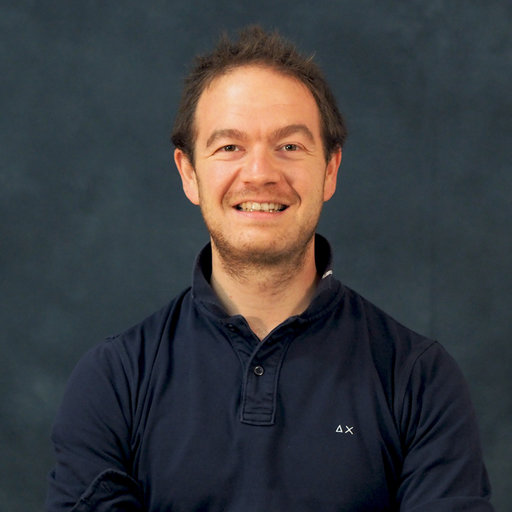
Abstract Radmila Pribić
Radar; Compressive Sensing, Information Geometry and Neural Networks in Radar
Compressive Sensing (CS) is a recent paradigm in sensing (since 2006) that works with fewer data because it is optimized to information in data rather than to the sensing bandwidth only. Most promising benefits of CS in radar are fewer data, high resolution and multi-target analysis.
Information geometry (IG) is an approach to stochastic signal processing (since the eighties) whose most promising benefits have been found in using information distances for resolution bounds, parameter estimation and analysis of accuracy and detection.
Neural networks (NNs) provide mighty numerical tools for learning radar-sensing models directly from data. IG and CS work naturally with NNs in the probabilistic inferences.
The mixture of CS, IG and NNs enables an elegant and straightforward framework for optimizing the demands of data acquisition and signal processing in radar.
Abstract Francesco Fioranelli
Radar – Old but gold
On the birthday cake for Radar we put this year 116 candles – it was indeed in 1904 that German engineer Christian Hülsmeyer publicly demonstrated for the first time radar technology to detect ships even in foggy conditions from a bridge in Köln (Cologne). However, research in sensing using transmitted and received electromagnetic signals is still enjoying exciting developments, as detecting and recognising objects at large distances and in all weather conditions is still a significant need today in aviation, navigation, autonomous systems, security.
One of these recent developments is the combination of modern artificial intelligence with radar based classification, to make our radar systems more capable, intelligent, autonomous. In my talk I will present some recent results and outstanding challenges from research activities in my previous post at the University of Glasgow and those planned and started here at TU Delft. Specifically, I will discuss applications of radar imaging (micro-Doppler signatures but not only) to the domain of human activities/gait identification in the context of healthcare, and classification of small Unmanned Aerial Vehicles (drones), relevant in security/defense context.
Additional information ...
.png)
CAS MSc Midterm Presentations
- Thursday, 27 February 2020
- 01:30-02:30
- HB 17.150
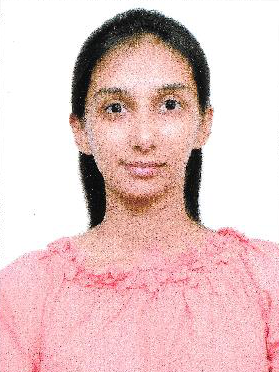
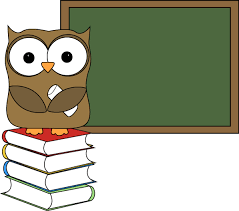
Signal Processing Seminar
- Thursday, 20 February 2020
- 13:30-14:30
- HB 17.150
Statistical and Array Signal Processing, Compressed Sensing, Tensor Analysis, Wireless Communications
Feiyu Wang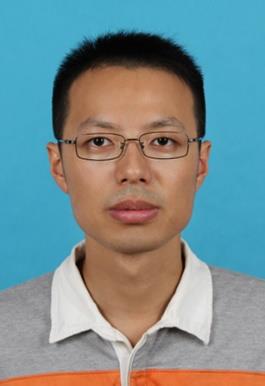
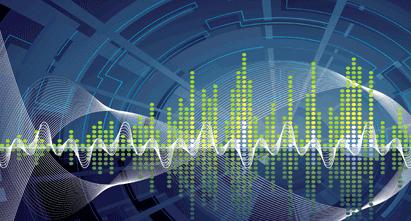
Signal Processing Seminar
- Thursday, 13 February 2020
- 13:30-14:30
- HB 17.150
PCaVision: Detection of prostate cancer using ultrasound
Mark BloemendaalCEO at Angiogenesis Analytics
Mark Bloemendaal represents his new startup company, Angiogenesis Analytics.
Additional information ...
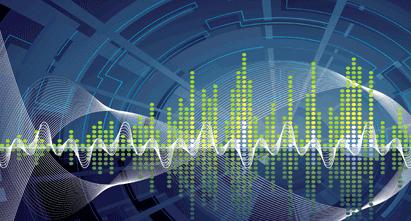
- Tuesday, 11 February 2020
- 12:30-14:00
- Bulding 28, ground floor, "Social Data Lab"
Distributed Perception and Learning Between Robots and the Cloud
Prof Sachin KattiStanford University
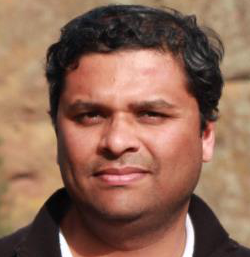
Today’s robotic fleets are increasingly facing two coupled challenges. First, they are measuring growing volumes of high-bitrate video and LIDAR sensory streams, which, second, requires them to use increasingly compute-intensive models, such as deep neural networks (DNNs), for downstream perception or control. To cope with such challenges, compute and storage-limited robots, such as low-power drones, can offload data to central servers (or “the cloud”), for more accurate real-time perception as well as offline model learning. However, cloud processing of robotic sensory streams introduces acute systems bottlenecks ranging from network delay for real-time inference, to cloud storage, human annotation, and cloud-computing cost for offline model learning.
In this talk, I will present learning-based approaches for robots to improve model performance with cloud offloading, but with minimal systems cost. For real-time inference, I will present a deep reinforcement learning based offloader that decides when a robot should exploit low latency, on-board computation, or, when highly uncertain, query a more accurate cloud model. Then, for continual learning, I will present an intelligent, on-robot sampler that mines real-time sensory streams for valuable training examples to send to the cloud for model re-training. Using insights from months of field data and experiments on state-of-the-art embedded deep learning hardware, I will show how simple learning algorithms allow robots to significantly transcend their on-board sensing and control performance, but with limited communication cost.
Please confirm your presence by sending an email to Ms. Marsha Ginsberg (M.Ginsberg@tudelft.nl).
Additional information ...
Signal Processing Seminar
- Thursday, 6 February 2020
- 13:30-14:30
- HB 17.150
Graph Signal Processing, Data Science
Alberto Natali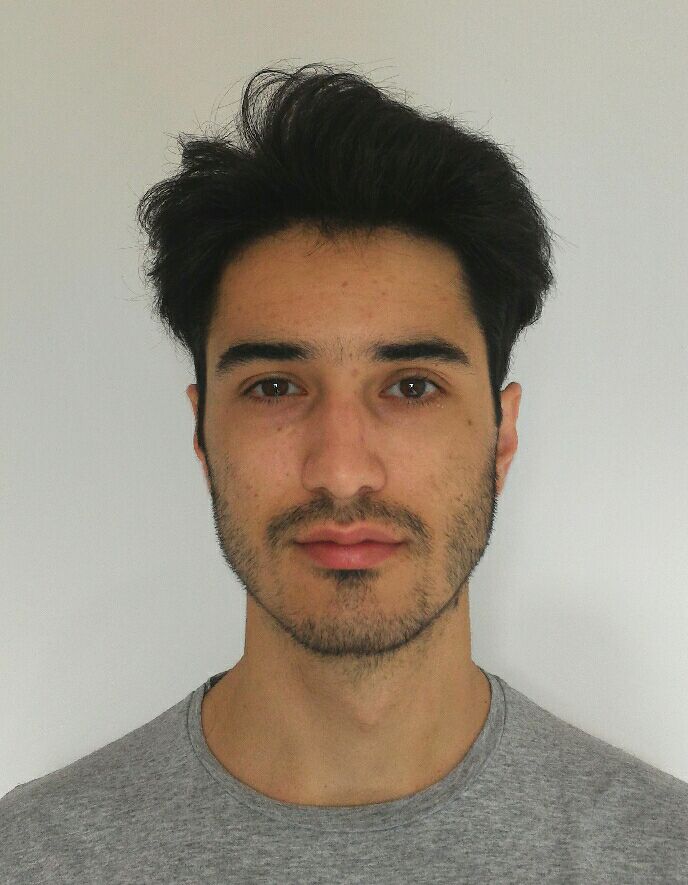
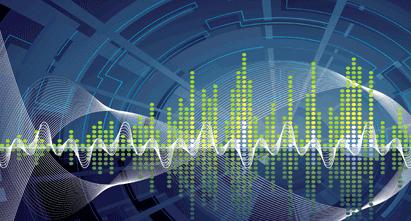
CAS MSc Midterm Presentations
- Thursday, 30 January 2020
- 13:30-14:30
- HB 17.150
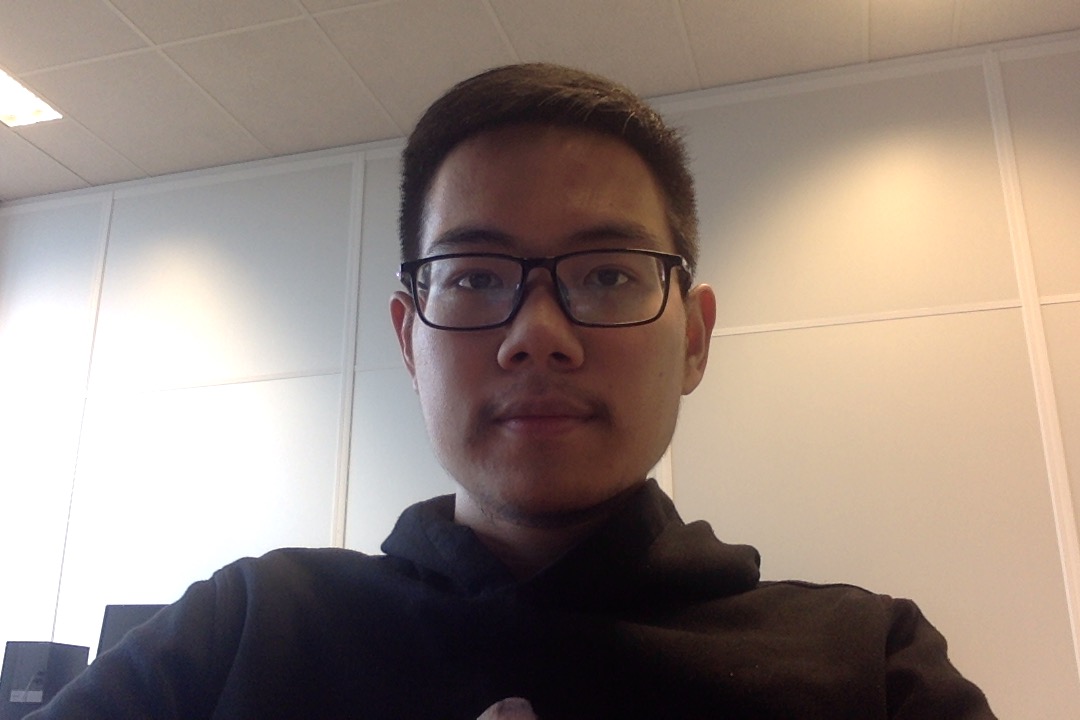
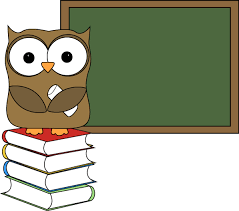
Signal Processing Seminar
- Thursday, 23 January 2020
- 13:30-14:30
- HB 17.150
Signal processing for communications
Tarik Kazaz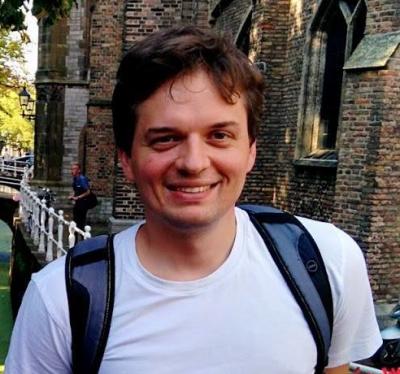
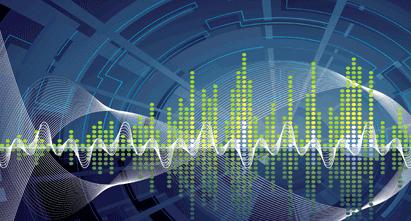
MSc SS Thesis Presentation
- Wednesday, 22 January 2020
- 09:30-10:15
- HB17.150
Two-Dimensional Blood Flow Estimation in the Brain with Ultrafast Ultrasound
Karishma Kumar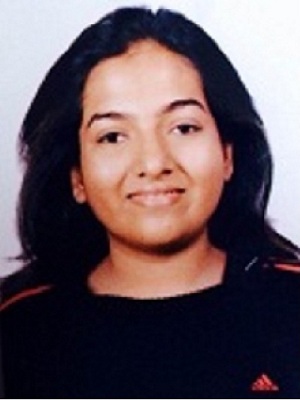
Detailed imaging of blood flow may improve the understanding of brain functions. The current state-of-the-art non-invasive flow imaging of the brain is limited to a one-dimensional Doppler setting. We propose a method to estimate the two-dimensional flow vector in the fine vascular network of the brain by using a speckle tracking technique. The framework of Orthogonal Matching Pursuit is used for speckle tracking where prior constraints are applied to guide the matching process to find the best possible displacements between two frames. The prior constraint is in the form of a directional constraint which determines the probability of vector flow in all the given directions according to the orientation of the vessels. The orientation of the vessels is computed using Power Doppler Imaging. In this work, the proposed method for two-dimensional vector estimation is compared with the standard block matching technique of Normalised Cross-Correlation. We see that the variance of the final velocity estimates is less and the direction of blood flow is found within the curvature of the vessel.
CAS MSc Midterm Presentations
- Thursday, 16 January 2020
- 13:30-11:30
- HB 17.150
Master Presentations
Jente Zandstra, Laurens Buijs, Maosheng Yang, Siyan Wan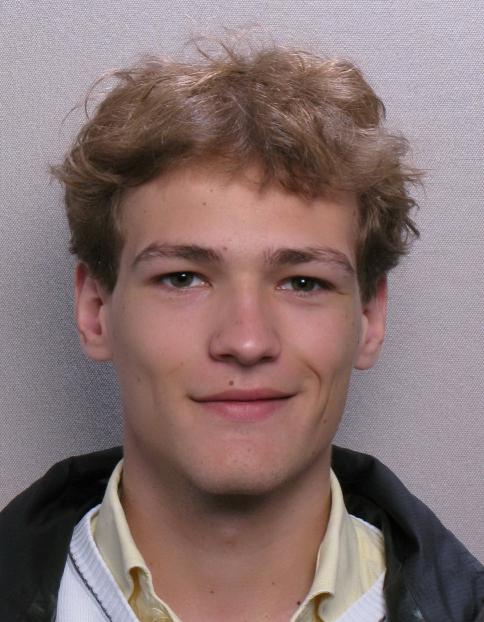
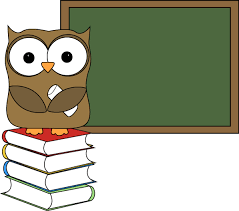
PhD thesis defense
- Wednesday, 15 January 2020
- 09:30-12:00
- Aula
ENERGY-AWARE NOISE REDUCTION FOR WIRELESS ACOUSTIC SENSOR NETWORKS
Jie Zhang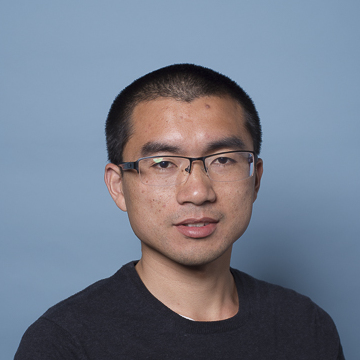
Signal Processing Seminar
- Wednesday, 15 January 2020
- 14:15-15:15
- HB 17.150
Statistical Signal Processing and Machine Learning for Speech Processing
Timo GerkmannUniversität Hamburg
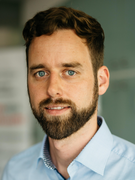
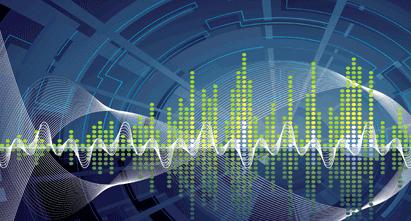
Signal Processing Seminar
- Wednesday, 8 January 2020
- 10:00-11:00
- HB 17.150
Efficient Algorithms for Convolutional Inverse Problems in Multidimensional Imaging
Didem Doğan Başkaya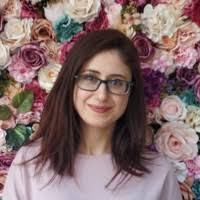
Computational imaging is the process of indirectly forming images from measurements using image reconstruction algorithms that solve inverse problems. In many inverse problems in multidimensional imaging such as spectral and depth imaging, the measurements are in the form of superimposed convolutions related to the unknown image. In this presentation, we first provide a general formulation for these problems named as convolutional inverse problems, and then present fast image reconstruction algorithms that exploit sparse models in analysis and synthesis forms. These priors involve sparsifying transforms or data-adaptive dictionaries that are patch-based and convolutional. The numerical performance of the developed algorithms is evaluated for a three-dimensional image reconstruction problem in spectral imaging. The results demonstrate the superiority of the convolutional dictionary prior over others. The developed algorithms are also extended to the compressive setting with compressed convolutional measurements.
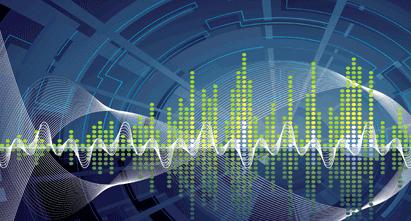
MSc CE Thesis Presentation
- Monday, 16 December 2019
- 16:00-16:45
- HB 17.150
An Object Detecting Architecture using Spiking Neural Networks
Davide Spessot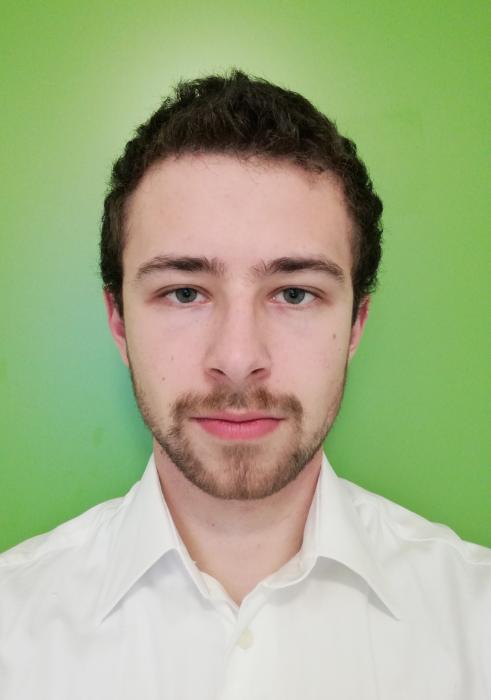
Recent trends in the embedded consumer market increased the need for low-power and reliable classification engines. Spiking Neural Network (SNN) is a new technology that promises to deliver 4 orders of magnitude more performance per watt than competing solutions. Moreover, the adoption of RADAR for gesture detection provides higher reliability compared to image sensors. However, no accepted topology for a temporal SNN classifier focusing on RADAR data exists. In addition, previous research didn’t account for several design limitations necessary to export the design in analog hardware.
In this work, we explore the possible SNN topologies and propose a Liquid State Machine (LSM) with fully-supervised readout, suitable to be exported to a mixed-signal neuron array. A complete parametric model of the architecture and learning rule has been implemented in a simulation environment. Following, the design space was explored in search for the optimal operating region. By analysing the results, the effects of several parameters and the trade-off between accuracy and power consumption were highlighted and explained. In particular, our work puts emphasis on a good balance between global excitation and inhibition in the LSM.
The final design contains 87 neurons and has a peak classification performance on 6 classes of 50%. The limitations of the proposed design point to the importance of an adequate feature extraction for a stable LSM behaviour and to the unpredictable nature of the SNN backpropagation algorithm, caused by the non differentiability of the spike signals.
MSc SS Thesis Presentation
- Friday, 13 December 2019
- 15:00-15:45
- LB01.010 Snijderszaal
Wave Dynamics in Inverse Krylov Subspaces
Joris Belier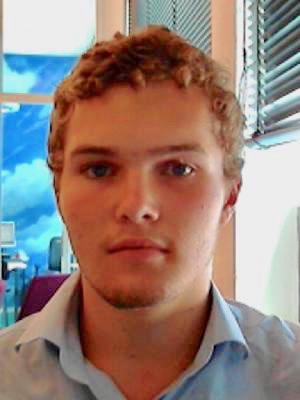
Recent studies have shown an increased interest in modal solutions of wave problems with resonating structures. These studies demonstrate that resonating structures with physical dimensions close to a wavelength can be accurately described by a few relevant resonating modes. The physical dimensions of the demonstrated resonating structures were close to a wavelength, which suggests that these highly-resonating modes have relatively low eigenvalues. Those resonating-modes are therefore dominantly present in Krylov subspaces generated by inverse projections of the wave-operator. Relevant wave dynamics can, therefore, be effectively computed from inverse Krylov subspaces. Furthermore, inverse Krylov subspaces are computationally stable and are therefore a powerful way to compute high-fidelity modal solutions. With interesting applications in high Q-factor wave problems.
The aim of this work is on improving the performance of inverse Krylov subspaces. Improvements to inverse Krylov subspace can be grouped into two approaches. In the first approach symmetry is exploited in the inverse wave-operator for reduced computational complexity and in the second approach the wave-operator is conditioned for desirable characteristics at the relatively low side of the spectrum. We will study several wave-operator configurations and optimize according to those approaches.
Earlier studies have shown that in the dimensions with pseudo-periodic boundary conditions, the double-curl is efficiently eigendecomposed as spatial derivatives are diagonal operators acting on frequency representations. We extend this work by providing an alternative, more compact presentation in the continuous domain of the eigendecomposition of the double-curl. This eigendecomposition is used to create a nullspace free eigenvalue problem.
Consecutively, we analyse the characteristics of the inverse wave-operator with Perfectly Matched Layers (PML). This analysis shows that in terms of inverse Krylov subspaces, the PML is not the obvious choice for the optimal absorbing boundary condition. Most notably, the PML introduces undesirable effects at the lower end of the spectrum, significantly impeding the performance of inverse Krylov subspaces, which leads to the conclusion that absorbing boundary conditions should be reassessed in terms of inverse Krylov subspaces behaviour.
Lastly, we will study the so-called Fixed-Frequency PML (FF-PML), which is a PML inspired time-independent absorbing boundary condition. Our study has shown that the FF-PML is a more suitable absorbing boundary condition candidate for inverse Krylov subspaces. It does not have the undesirable effects at the lower end of the spectrum, which the traditional PML has. Furthermore, and even more importantly, we derive analytic expressions of the inverse wave operator with FF-PML absorbing boundary conditions. This simple and novel insight is easily exploited to invert the wave-operator efficiently, which enables a new approach to the computation of modal solutions of open scattering problems. The first results of which look promising.
CAS MSc Midterm Presentations
- Thursday, 12 December 2019
- 13:30-14:00
- HB 17.150
Optimization of Radio Astronomy Sensor Placement with Calibration Taken into Account
Kaiwen Zhang
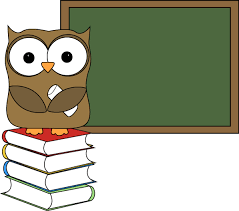
MSc BME Thesis Presentation
- Wednesday, 11 December 2019
- 09:03-10:15
- HB 13.100
Investigating brain function and anatomy through ICA-based functional ultrasound imaging
Mado Ntekouli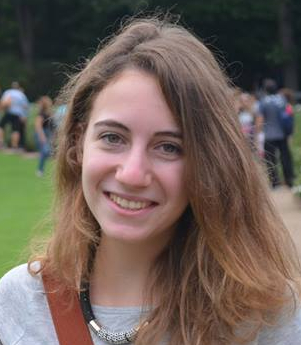
Understanding the hidden organizational principles existing in the human brain was always one of the great challenges in Neuroscience. To uncover the way the brain functions, advancements in the fields of Medical Imaging and Computational Science have been of great importance. Powerful imaging tools, such as functional magnetic resonance imaging (fMRI) and positron emission tomography (PET), have already enabled scanning the whole brain volume and visualizing the brain functioning, both at rest and during task execution, to a signicant degree.
However, several limitations especially in spatiotemporal resolution led to the need for further advancements in the eld of functional imaging. An alternative technique, that overcomes most of the previously existing problems, is functional ultrasound (fUS). fUS is capable of imaging even the microvasculature blood-flow dynamics in response to brain activation with high spatiotemporal resolution. The wealth of fUS-acquired data calls for advanced data-analytic methods to uncover new information, beyond the well-applied simple univariant correlation method.
This is the main goal of this MSc thesis, to use a proper analysis technique, mainly borrowed from the same-principle fMRI technique, in order to produce powerful inferences. For this reason, a detailed literature review regarding fUS imaging and fMRI analysis methods is introduced. Then, the main analysis part is focused on the Independent Component Analysis (ICA) method, trying to segregate the brain into spatially independent components that share a similar activity response. Here, the whole processing pipeline is established, describing all the necessary preprocessing steps along with ICA parameters and approaches (single- and group-ICA) using the ICASSO software package. As a post-processing step, functional images-to-Allen brain atlas registration is also performed in order to identify the different regions represented in the ICA-derived spatial components. The effectiveness of the methods is assessed based on the collected results on different datasets, obtained from 2D visual-stimulation as well as 3D resting-state experiments conducted on mice at the Neuroscience department of the Erasmus MC. As a conclusion, ICA was able to separate different anatomical and functional sub-networks. More specically, from the visual-stimulation experiments, brain regions such as Lateral geniculate nucleus (LGN) that play a role in the visual pathway are identied, while from the resting-state the spatial continuity of different regions is confirmed.
MSc TC Thesis Presentation
- Friday, 6 December 2019
- 13:00-13:45
- HB.03.230
Space-Time Codes for Massive MIMO Systems
Vishnu RachuriUbiquitous connectivity requirements and stringent quality-of-services (QoS) in recent wireless communications demand new revolutionary wireless network technologies to support the exponentially increasing traffic growth. Massive multiple-input-multiple-output (MIMO) with the capability of high spectral efficiency achieved by large multiplexing and diversity gains grabbed a lot of attention as a promising solution for future cellular networks. Achieving ultra-reliable and low latency communication is very challenging without increasing the network infrastructure cost, or extra processing complexity. Adapting space-time-block-codes (STBC) can improve the reliability of the system, but this increases the downlink pilot overhead. Two precoders are devised to exploit full spatial diversity with the blind combining process to avoid downlink pilot overhead.
MSc CE Thesis Presentation
- Friday, 29 November 2019
- 09:30-10:15
- HB 9.150
Neuromorphic Retina Design for LIDAR
Rahul Vyas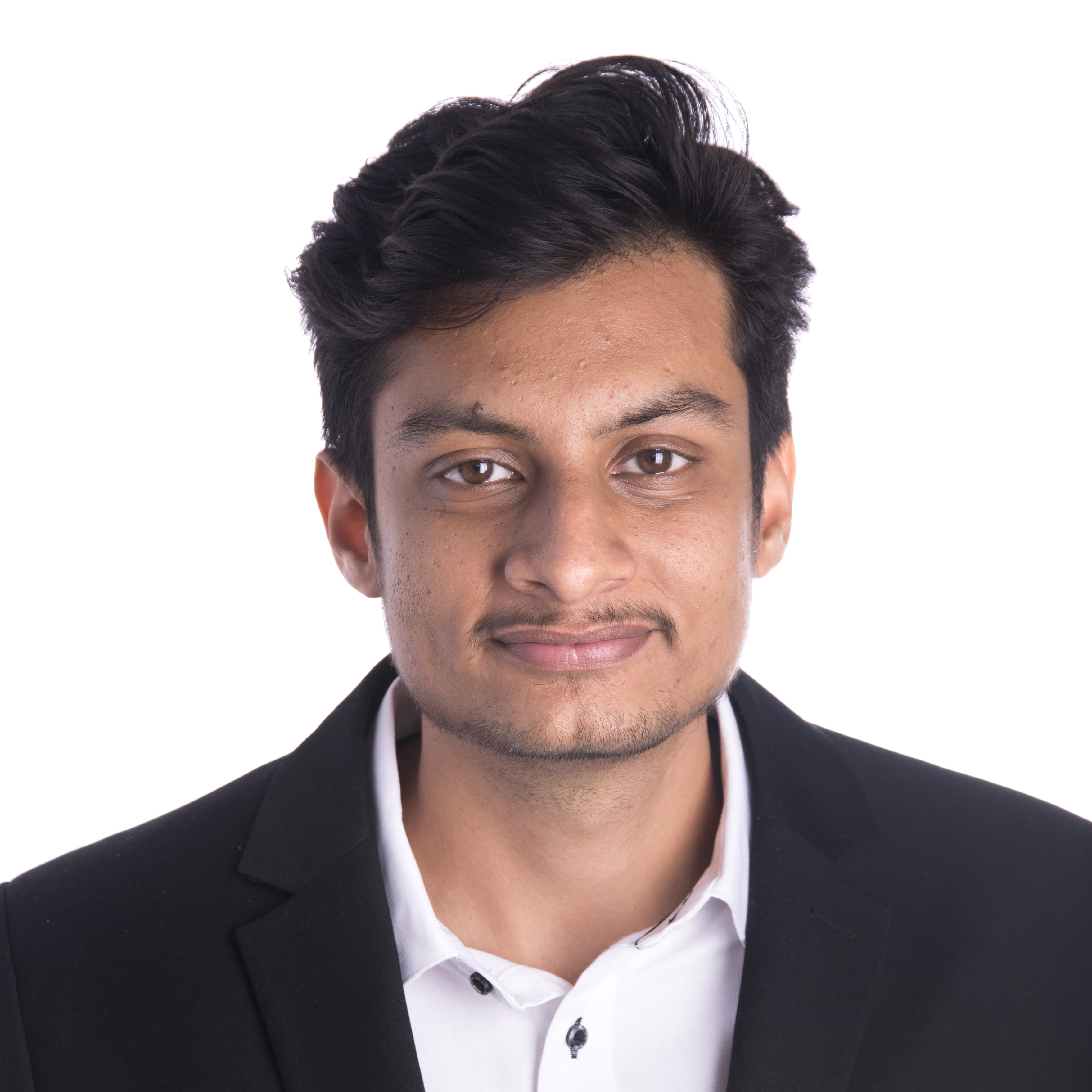
Autonomous vehicle (AV technology) relies heavily on vision based applications like object recognition, obstacle/collision avoidance etc. In order to achieve this, understanding and estimating the dynamics in the environment is extremely important. LIDARs are proven to detect both shape as well as the speed/movement of the objects in the scene but one of the biggest challenges faced in adapting LIDAR technology is the huge amount of data it produces and the way it is processed. Most of this data is redundant static information which results in wastage of system memory, computational resources, power and time. Inspired from biological retina, first Neuromorphic-Retina for LIDAR is proposed that is able to extract and encode movement happening at particular distance, particular angle and with particular velocity from raw LIDAR temporal pulses into unique spike sequences so that the information about the dynamic environment can be efficiently classified and processed by event based and low powered Neuromorphic processing unit.
The system is designed in such a way that it avoids consumption of large amount of computational resources and system memory. Simulation results show that the Retina is able to filter out redundant static information from the LIDAR data stream thereby reducing data throughput of around 50 - 70 % with 5 - 22 % spatial quality loss (based on scenario) as well as remove noise caused due to luminous reflections. This has tremendous impact on system latency and power consumption due to drop in memory accesses.
Additional information ...
MSc SS Thesis Presentation
- Friday, 29 November 2019
- 09:30-10:15
- HB 17.150
Particle Filter based Speaker Tracking in Distributed Pairwise Microphone Networks
Lantian KouThe particle filter (PF) algorithm is appropriate to solve the problem of speaker tracking in a reverberant and noisy environment using distributed pairwise microphone networks. First, complete the tracking task based on PF algorithm in centralized manner, a processing center is required to collect the signal from all microphones to carry out the PF processing. The computation complexity and time consumption of the particle filter algorithm are relatively high, mainly because of the large number of particles exploited in the filtering process since the effectiveness and accuracy of the particle filter particularly rely on the sample set size.
However, almost all the existing particle filtering algorithms exploit the fixed number of particles, especially in the field of acoustic source tracking. To deal with this matter, Kullback-Leibler distance (KLD) sampling method was utilized as an adaptation technique to adjust the sample size instead of setting fixed number.
Two approaches based on particle filter algorithm for tracking speaker in distributed way are proposed. Compared to the centralized scheme, each microphone pair in the distributed network executes the local PF individually and exchanges local weights or posterior parameters among neighboring nodes to efficiently achieve the global estimate of the sound source position. Finally, simulation experiments demonstrate these two methods are feasible to track the speaker in distributed microphone networks with a variable number of particles.
MSc SS Thesis Presentation
- Wednesday, 27 November 2019
- 10:00-10:45
- HB 17.150
Active Semi-Supervised Learning For Diffusions on Graphs
Bishwadeep Das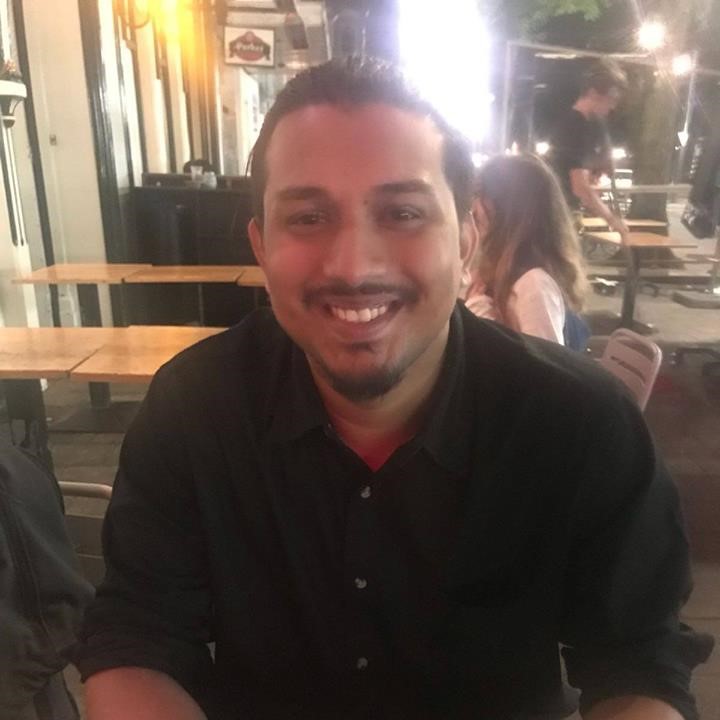
In statistical learning over large data-sets, labeling all points is expensive and time-consuming. Semi-supervised classification allows learning with very few labels. Naturally, selecting a few points to label becomes crucial as the performance relies heavily on the labeled points. The motivation behind active learning is to build an optimal training set keeping the classifier in mind. Random or heuristic-driven selection does not care for the classification process or are trivially defined.
We are interested in the graph structure formed by the data, as seen in citation, social and biological networks. Accordingly, active semi-supervised learning on graphs labels nodes to enhance the performance of classification.
We propose a new methodology to perform active learning for diffusion-based semi-supervised classifiers. In particular, we focus on a classifier which diffuses probability distributions over the graph through random walks. We postulate the active learning problem as i) a linear inverse problem with a sparse starting distribution over the nodes; ii) a model output selection problem. For the former, we use sparsity-regularized inverse problems to select nodes. For the latter, we use tools from Compressed Sensing and Sparse Sensing to select the nodes with the relevant model output. We show that we can select all the relevant nodes in a single shot fashion, hence avoiding reliance on multiple training phases.
Results on simulated as well as real data-sets show the proposed methods outperform random labeling, thereby proving to be relevant for active semi-supervised learning on graphs.
Additional information ...
MSc SS Thesis Presentation
- Monday, 25 November 2019
- 13:00-13:45
- HB 17.150
Magnetic Resonance Imaging Motion Correction in k-Space
Rajesh Rajwade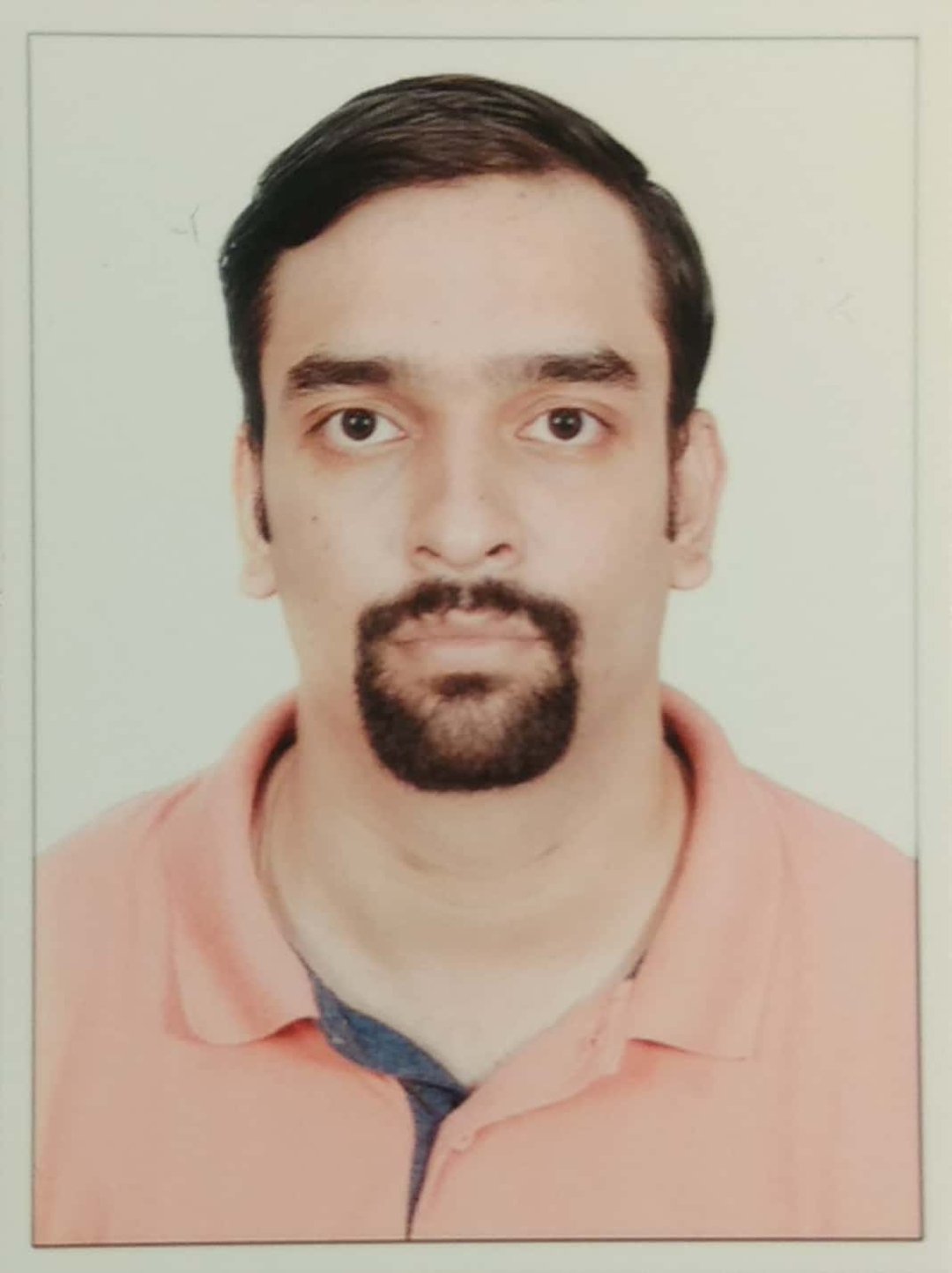
Magnetic Resonance Imaging is a widely used technique to obtain images of the interior of the human body for diagnosis and treatment. MRI machines capture the raw signal in spatial frequency domain i.e. k-space and the image is obtained via Fourier transform.
The Cartesian acquisition is one of the most commonly used acquisition patterns in MRI and is most susceptible to the patient's motion. Due to long scanning times, the possibility of the patient's movement is higher which introduces bulk motion artifacts reducing the quality of the image. Motion artifacts can affect the diagnosis and the necessity of re-scanning can cause significant financial costs as well as delays in diagnostics.
Current methods for correcting motion artifacts work in image domain which need completely sampled k-space for reconstruction and hence are not useful for real-time artifacts correction.
In this thesis, machine learning methods that can detect, estimate and correct motion artifacts in k-space were investigated making it possible to correct artifacts in real-time without the necessity of reconstruction. For each of these methods, we analyze the performance and discuss the merits and demerits.
Additional information ...
MSc CE Thesis Presentation
- Friday, 22 November 2019
- 14:00-14:45
- HB 17.150
Object Detecting Architecture using Spiking Neural Networks
Joppe Lauriks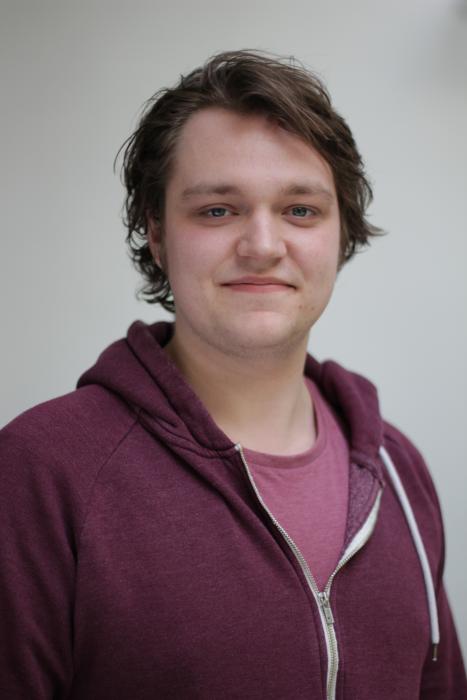
Spiking Neural Networks have opened new doors in the world of Neural Networks. This work implements and shows a viable architecture to detect and classify blob-like input data. An architecture consisting of three parts a region proposal network, weight calculations, and the classifier is discussed and implemented.
The region proposal network is build based on a blob detecting Laplacian of Gaussian function. The architecture is tested and verified on the Multi MNIST dataset that is generated based on the MNIST dataset that consists of handwritten digits. Results show that, on average, the region proposal network can locate the blobs in the input with an accuracy of within a single pixel distance from the ground truth. Two different ways of decoding the rate data coming from the region proposal network where discussed the Peak based decoder could propose regions even if these regions are situated closely together. A Center of Mass decoder is slightly more accurate than the Peak based decoder but at a higher computational cost and performance degradation when the regions are close together.
The region proposal network at worst only accounts for 3.19% of inaccuracy. The implementation shows that the architecture is a viable way of detecting and classifying multiple objects within the input. The data shows that the region proposal network itself is a feasible way of detecting blob-like objects within its input.
Signal Processing Seminar
- Thursday, 21 November 2019
- 13:30-14:30
- HB 17.150
Partial discharges recognition and (localization) in Gas Insulated Systems (GIS) using the cross wavelet transform.
Fabio Muñoz MuñozTU Delft
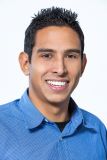
Partial Discharges (PD) detection is an essential tool for the diagnosis of high-voltage equipment because of their accuracy to detect and quantify defects and damages in the dielectric insulation, where the detection implies the measurement, acquisition, storage and processing of the PD phenomenon. Nowadays, the most widespread PD detection system is based on electrical measurements, in which the PD signals are acquired in the form of individual or series of electrical pulses.
In spite of the PD measurement has been exhaustively researched over the years, the separation of PD pulses from noise is one of the main challenges, especially in online applications. Noise contamination still one of the significant problems for PD measurements. Several studies have focused on the PD pulses separation and denoising techniques for PD measurements, in which the wavelet transform has been extensively used because is capable of locating time and frequency components allowing the analysis of aperiodic signals with irregular and transition features, such as the partial discharges. However, a major problem that most of these denoising techniques face is the ingress of external interferences having time-frequency characteristics similar to the partial discharge signals: periodic pulse-shaped interferences from power electronics, PD and corona discharges from the external power system, electrical pulses from switching operations, lightings, etc. This external noise can cause a false indication of PD activity, reducing the effectivity of the PD measurements as a diagnostic tool.
In PD measurement systems multiple signals can be simultaneously acquired for each PD event. Recording each signal through different sensors may provide extra useful information about the real nature of the waveform recorded. Tools like the correlation and trend analysis can provide the significance of relationships between the signals recorded. Nevertheless, these tools may not detect correlations if the signals are phase shifted; for instance, a phase shift of 180 ° between the signals may appear uncorrelated. The cross-correlation and the cross-spectral analysis can detect the phase shift, but only as average values and in stationary signals. For analysing aperiodic signals with irregular and transition features, the most suitable tool is the cross wavelet transform because it exposes regions with high common power and reveals the local relative phase between both signals.
In this presentation, we introduce the partial discharge measurements, the PD signals propagation in GIS, the cross wavelet as a tool to separate the PDs from the external disturbances, and some of the challenges that we are facing in the PD localization and detection in GIS.
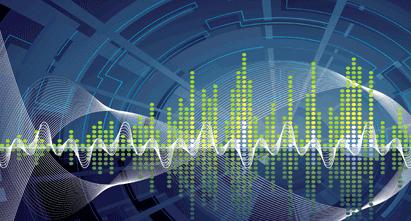
Signal Processing Seminar
- Wednesday, 20 November 2019
- 10:00-11:00
- HB 17.150
On Unlimited Sampling and Reconstruction: A New Way to Sense the Continuum
Ayush Bhandari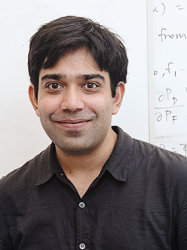
Almost all forms of data are captured using digital sensors or analog-to-digital converters (ADCs) which are inherently limited by dynamic range. Consequently, whenever a physical signal exceeds the maximum recordable voltage, the digital sensor saturates and results in clipped measurements. For example, a camera pointed towards the sun leads to an all-white photograph. Motivated by a variety of applications including scientific imaging, communication theory and digital sensing, a natural question that arises is: Can we capture a signal with arbitrary dynamic range?
In this work, we introduce the Unlimited Sensing framework which is a novel, non-linear sensing architecture that allows for recovery of an arbitrarily high dynamic range, continuous-time signal from its low dynamic range, digital measurements. Our work is based on a radically different ADC design, which allows for the ADC to reset rather than to saturate, thus producing modulo or folded samples.
In the first part of this talk, we discuss a recovery guarantee akin to Shannon’s sampling theorem which, remarkably, is independent of the maximum recordable ADC voltage. Our theory is complemented with a stable recovery algorithm. Moving further, we reinterpret the unlimited sensing framework as a generalized linear model and discuss the recovery of structured signals such as continuous-time sparse signals. This new sensing paradigm that is based on a co-design of hardware and algorithms leads to several interesting future research directions. On the theoretical front, a fundamental interplay of sampling theory and inverse problems raises new standalone questions. On the practical front, the benefits of a new way to sense the world (without dynamic range limitations) are clearly visible. We conclude this talk with a discussion on future directions and relevant applications.
Additional information ...
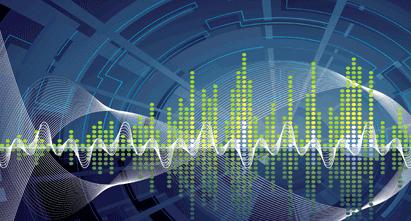
Signal Processing Seminar
- Friday, 15 November 2019
- 13:30-14:30
- HB 17.150
Sparse Bayesian Learning: A Beamforming and Toeplitz Approximation Perspective
Bhaskar RaoUCSD
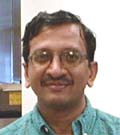
Sparse Bayesian Learning (SBL) methods that employ a Gaussian scale mixture prior have been successfully applied for solving the sparse signal recovery (SSR) problem. The SBL-EM based inference algorithm will be examined and interpreted using a beamforming framework. A contrast with the classical minimum power distortionless response (MPDR) beamformer will be drawn and the benefits highlighted. An interesting finding is the ability of SBL to deal with correlated sources. For a uniform linear array (ULA), the Toeplitz approximation property of SBL will be discussed and the potential benefits for a nested array demonstrated.
Speaker Biography
Bhaskar D. Rao received the B.Tech. degree in electronics and electrical communication engineering from the Indian Institute of Technology, Kharagpur, India, in 1979 and the M.S. and Ph.D. degrees from the University of Southern California, Los Angeles, in 1981 and 1983, respectively. Since 1983, he has been with the University of California at San Diego, La Jolla, where he is currently a Distinguished Professor in the Electrical and Computer Engineering department. He is the holder of the Ericsson endowed chair in Wireless Access Networks and was the Director of the Center for Wireless Communications (2008-2011). Prof. Rao’s interests are in the areas of digital signal processing, estimation theory, and optimization theory, with applications to digital communications, speech signal processing, and biomedical signal processing.Prof. Rao was elected fellow of IEEE in 2000 for his contributions to the statistical analysis of subspace algorithms for harmonic retrieval. His work has received several paper awards; 2013 best paper award at the Fall 2013, IEEE Vehicular Technology Conference for the paper “Multicell Random Beamforming with CDF-based Scheduling: Exact Rate and Scaling Laws,” by Yichao Huang and Bhaskar D Rao, 2012 Signal Processing Society (SPS) best paper award for the paper “An Empirical Bayesian Strategy for Solving the Simultaneous Sparse Approximation Problem,” by David P. Wipf and Bhaskar D. Rao published in IEEE Transaction on Signal Processing, Volume: 55, No. 7, July 2007, 2008 Stephen O. Rice Prize paper award in the field of communication systems for the paper “Network Duality for Multiuser MIMO Beamforming Networks and Applications,” by B. Song, R. L. Cruz and B. D. Rao that appeared in the IEEE Transactions on Communications, Vol. 55, No. 3, March 2007, pp. 618 630. (http://www.comsoc.org/ awards/rice.html), among others. Prof. Rao is also the recipient of the 2016 IEEE Signal Processing Society Technical Achievement Award.
Prof. Rao has been a member of the Statistical Signal and Array Processing technical committee, the Signal Processing Theory and Methods technical committee, the Communications technical committee of the IEEE Signal Processing Society and is currently chair of the Machine learning for Signal Processing technical committee.
Additional information ...
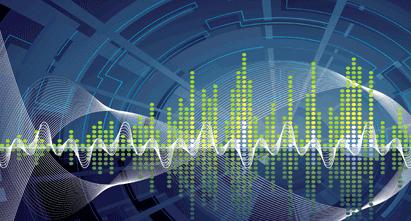
Signal Processing Seminar
- Thursday, 14 November 2019
- 16:00-17:00
- HB 17.150
Realizing the potential of precision medicine with mobile sensing and analytics
Emre ErtinOhio State University
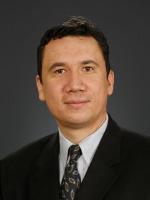
Recent advances in wearable sensing and mobile computing have given researchers the ability to collect unprecedented amounts of data about everything from biology to behavior that can explain and improve people's health status. Day-to-day data from wearable sensors allows for better and more personalized decisions in regard to health care and management. Specifically, continuous monitoring of physiology and behavior can help us to assess disease risks, perform disease prevention and early detection of chronic conditions. However, there still exist a multitude of challenges to implement this vision of precision medicine. Wearable sensors provide large, noisy, complex data streams about the many facets of our life and health, but there is still a a gaping need for computational techniques that can transform sensor data into set of useful bio-markers readily interpretable by clinicians. This talk will describe our recent work in pairing rich probabilistic models with Bayesian methods to dramatically expand the scale and quality of physiological data we can obtain in the field while minimizing the burden to participants.
Signal Processing Seminar
- Thursday, 7 November 2019
- 13:30-14:30
- HB 17.150
Privacy-Preserving Distributed Optimization via subspace perturbation: A generalized convex optimization approach
Qiongxiu Li (Jane)Audio Analysis Lab, Aalborg University
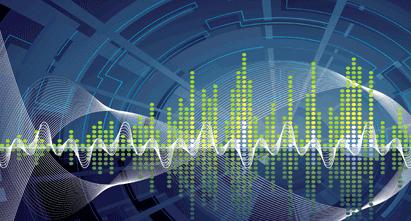
MSc ME Thesis Presentation
- Thursday, 31 October 2019
- 15:00-15:30
- Building 34, Lecture Hall E (Robert Hooke)
A Dynamic Zoom ADC for Audio Applications
Efraïm Eland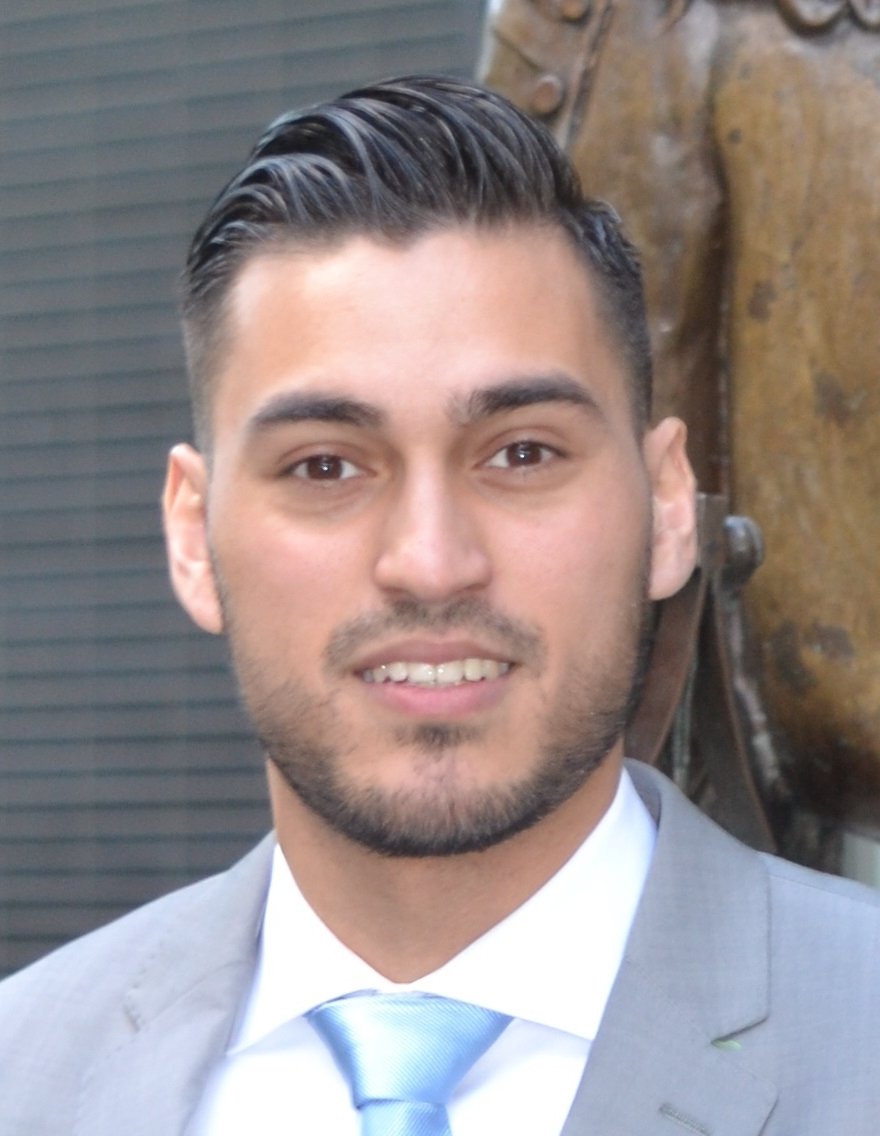
Audio ADCs used in high-fidelity portable audio and IoT are not only required to have high linearity and dynamic range (DR) but are also expected to be very energy efficient and occupy minimum silicon area. Zoom-ADCs combine a coarse asynchronous SAR with a fine Delta-Sigma Modulator (∆ΣM) to satisfy these requirements. Existing zoom ADC architectures are limited in terms of SQNR due to the need for the fine ADC to have some over-ranging. That, together with the leakage of the SAR ADC’s quantization noise, “fuzz,” into the audio band, puts a lower limit on the sampling frequency.
This thesis describes the design of a zoom-ADC for an audio bandwidth of 20kHz. Using a 4-level quantizer, instead of a conventional 1b quantizer, mitigates the adverse effects of over-ranging, making it possible to keep a very low sampling frequency. On top of that, it makes use of a simple, low power analog “fuzz” cancellation scheme to prevent the SAR quantization noise from leaking into the audio band.
The chip has been prototyped in a standard 160nm CMOS technology and consumes 339μW with 107.7dB DR and 105dB SNDR. Compared to state-of-the-art ADCs with a similar bandwidth, this work achieves a 2x lower OSR (fs = 2.5MHz), significantly improving the energy efficiency and achieving a Schreier FoM of 185.4dB.
MSc ME Thesis Presentation
- Thursday, 31 October 2019
- 12:30-13:00
- Building 34, Lecture Hall E (Robert Hooke)
Rail-to-rail input and output amplifier for ADC front-end applications.
Shubham Khandelwal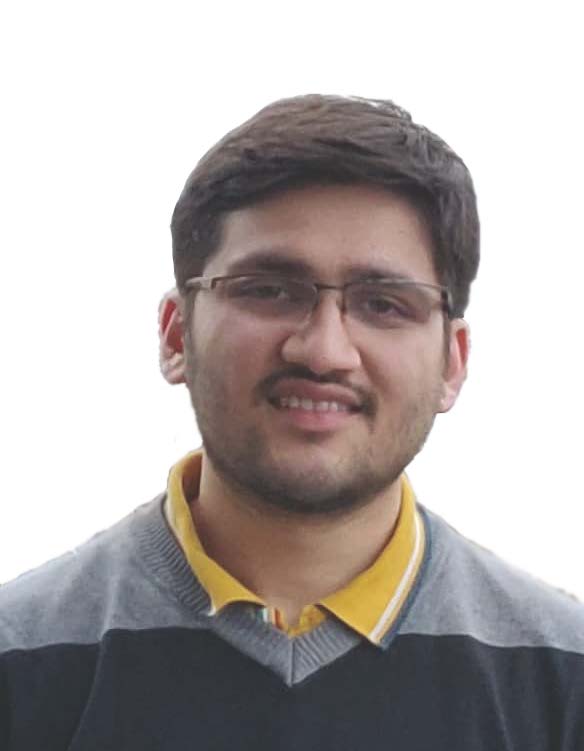
This work presents a unity-gain stable operational amplifier for an ADC front-end application. The op-amp focuses on delivering high linearity with low noise and offset while driving a switched capacitor load. To accomplish this the op-amp employs Current Spillover, Chopping and Gain-Boosting techniques. The op-amp achieves THD of -108 dB at 10kHz, offset of 2.7 µV and input noise density of 19.3 nV/√Hz while consuming 504 µW; resulting in an NEF of 12.28. The op-amp is fabricated in 0.16 µm CMOS technology and occupies 0.1 mm2 area.
MSc SS Thesis Presentation
- Monday, 28 October 2019
- 09:30-10:15
- Timmanzaal (LB 01.170)
Estimating the room impulse response
Gabriele Zacca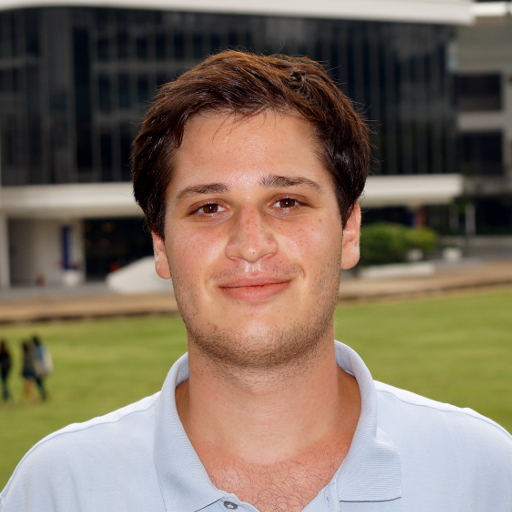
The response of a sound system in a room primarily varies with the room itself, the position of the loudspeakers and the listening position. The room boundaries cause reflections of the sound that can lead to undesired effects such as echoes, resonances or reverberation. Therefore the location of these large reflecting surfaces is important information for sound field estimation in a room.
This work focuses on exploiting the inherent information present in echoes measured by microphones, to infer the location of nearby reflecting surfaces. A built-in microphone array is used that is co-located with the loudspeaker. The loudspeaker probes the room by emitting a known signal. A signal model is proposed which provides a relationship between reflector locations and measured microphone signals.
The locations of reflections are estimated by fitting a sparse set of modeled reflections with measurements. We present two novelties with respect to prior art. First, the method is end-to-end where from raw microphone measurements it outputs an estimate of the location of reflectors. Where specifically for the compact uniform circular microphone array the symmetry is exploited to create an algorithm that is of reduced computational complexity. Secondly, the model is extended to include a loudspeaker model that is aware of the inherent directivity pattern of the loudspeaker.
The performance of the proposed localization method is compared in simulation to the existing state-of-the-art localization methods. Real world measurements are also used to validate the proposed loudspeaker model.
MSc SS Thesis Presentation
- Monday, 28 October 2019
- 13:15-14:30
- Lecture hall D@ta
Atrial Fibrillation: Estimation of the local activation time in high-resolution mapping data
Bart Kölling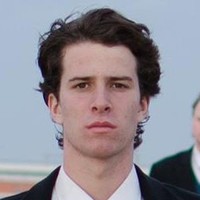
A common cardiac arrhythmia is atrial fibrillation, which is becoming more widespread worldwide. Currently there is some understanding about the mechanisms behind atrial fibrillation, however more insight into the conduction of the atrial tissue is desired.
Therefore, invasive mapping studies have been performed where an array of electrodes is used to record the electrical activity on the heart’s surface during open-chest surgery. The moment in time when the tissue under an electrode depolarizes, called the local activation time can be used to reconstruct the propagation pattern of the signal that triggers the tissue to contract.
In this thesis, the application of the cross-correlation for estimation of the local activation time of the atria is investigated. Specifically, the benefits of not only cross-correlating electrode pairs that are close, but also pairs that are far away are evaluated. A framework is constructed, based on a graph, that defines these higher order neighbouring pairs of electrodes.
This is compared to the golden standard of using the steepest deflection of an electrogram, as well as to other methods using the cross-correlation. Experiments are done on simulated electrograms where the true activation times are available, as well as on natural data recorded from patients. Finally some future research is proposed to investigate for which morphologies the proposed cross-correlation based methods may be most effective.
Additional information ...
MSc SS Thesis Presentation
- Friday, 25 October 2019
- 14:00-14:45
- HB 17.150
Signal Modelling and Imaging of Low Field MRI
Sherine Brahma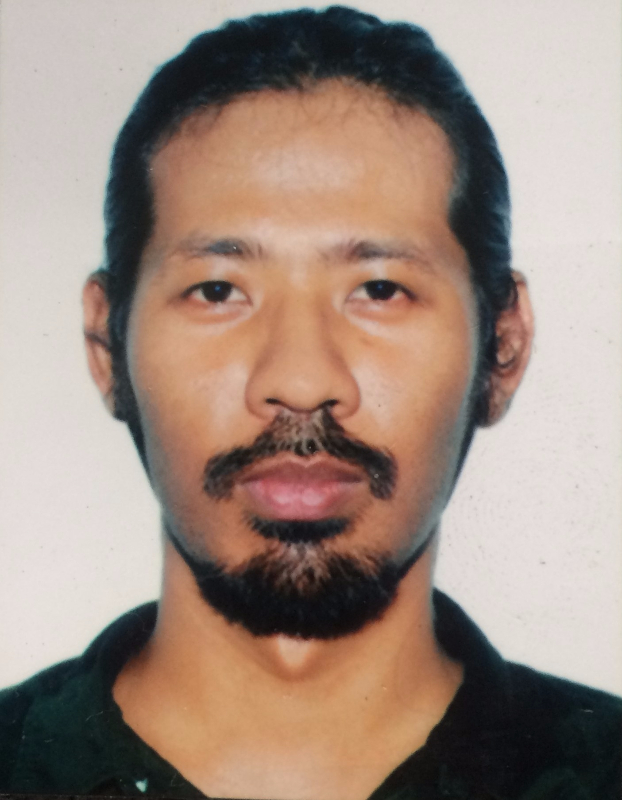
MRI machines are devices that are used to non-invasively obtain images of the internal anatomy and physiological processes of the human body. It is safe to use as the patient is not exposed to any harmful radiation, and there are no known side effects. But such machines that are commercially available are very expensive. Due to this reason, it eludes access to a large portion of the population, particularly in developing countries.
This thesis investigates an inexpensive MRI machine that is based on a rotating inhomogeneous magnetic field map. Unlike conventional scanners, because of the rotating field, the signal model of this device has to account for it. The objective of this work is to examine the aforementioned model, and also to implement Krylov subspace-based reconstruction algorithms available in the IRTools package.
Additional information ...
Signal Processing Seminar
- Thursday, 24 October 2019
- 13:30-14:30
- HB 17.150
Delamination monitoring in composites with fibre Bragg grating sensors
Aydin Rajabzadeh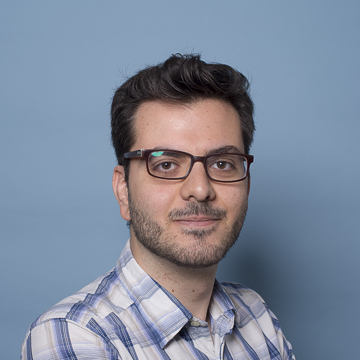
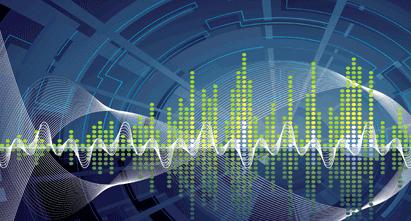
MSc ES Thesis Presentation
- Thursday, 24 October 2019
- 10:00-10:45
- HB 17.150
N-Shot Training Methodology
Ninad Joshi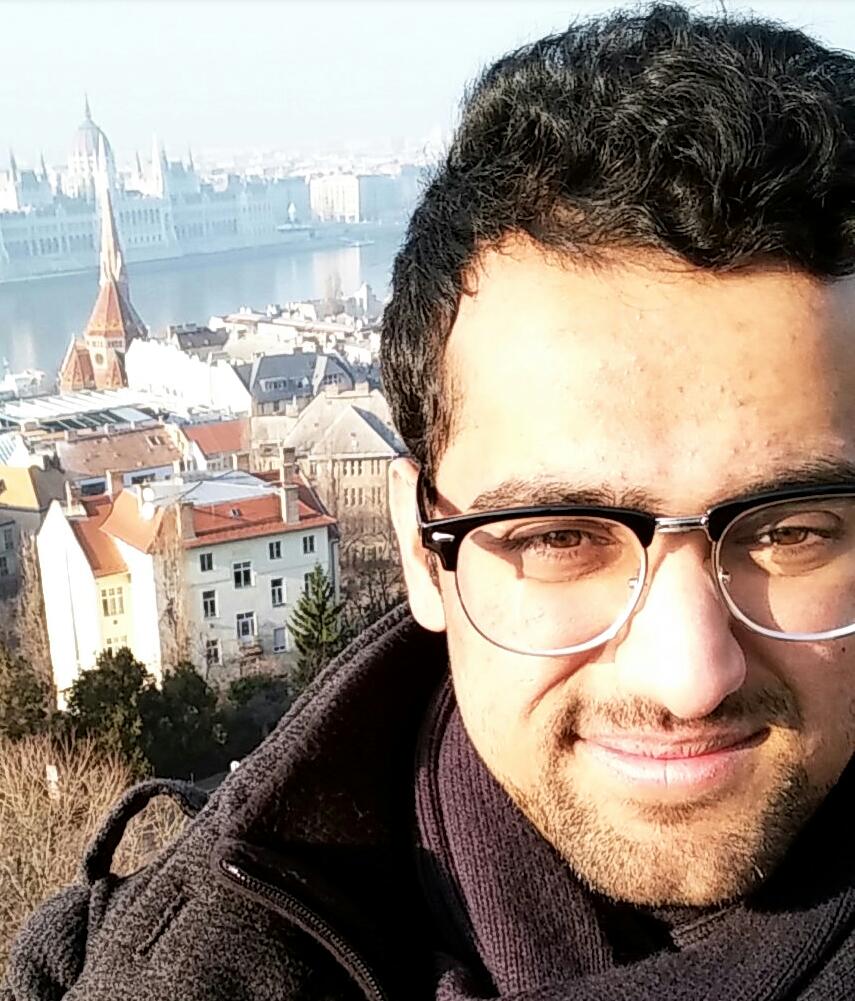
Traditional Artificial Neural Networks(ANNs)like CNNs have shown tremendous opportunities in various domains like autonomous cars, disease diagnosis, etc. Proven learning algorithms like backpropagation help ANNs in achieving higher accuracy. But there is a serious challenge with the increasing popularity of traditional ANNs is of energy consumption and computational complexity.
Spiking Neural Networks (SNNs) are considered to be next-generation neural networks that are capable of doing complex deep learning applications at fraction of energy that is needed in current deep learning applications because of its similarity to biological neurons. However, SNN is still not able to match the classification accuracy of ANNs which poses a big challenge for wide acceptance of SNN in various applications as traditional learning methods like backpropagation are not possible in SNN.
During training of a neural network the weight matrix is of the highest importance as it eventually decides the trajectory of learning. Currently, one existing solution is to just manually convert ANNs into SNNs to get weight matrix which doesnot focus on getting weight matrix from a small dataset and doesn’t consider spiking neuron parameters.
We aim to address this challenge by proposing a novel N-shot training methodology that is capable of providing a weight matrix for SNN and can give sufficient classification accuracy. The methodology not only provides the weight matrix but can do training with a very small dataset(up to 1 image per class) and still can give considerably higher accuracy. For a reduced MNIST dataset, the method can give an accuracy of 71.68% 10 images per class.
Additional information ...
MSc ME Thesis Presentation
- Wednesday, 23 October 2019
- 15:00-15:30
- LB 01.010 Snijderszaal
A PLL-based eddy current displacement sensor for button applications
Matheus Ferreira PimentaThis thesis presents an eddy current sensor (ECS) for button readout applications. The interface embeds the coil sensor in a digitally controlled oscillator (DCO) and uses a highly digital phase locked loop (PLL) to convert the displacement information into a digital output.
The sensor achieves more than 12bit effective resolution, which translates into an equivalent displacement resolution in excess of 10nm RMS. The interface consumes less than 235µA from a 1.8V supply, resulting in a very power efficient architecture.
MSc SS Thesis Presentation
- Thursday, 17 October 2019
- 10:00-10:45
- HB 21.120
Indoor localization using narrowband radios and switched antennas in indoor environment
Ye Cui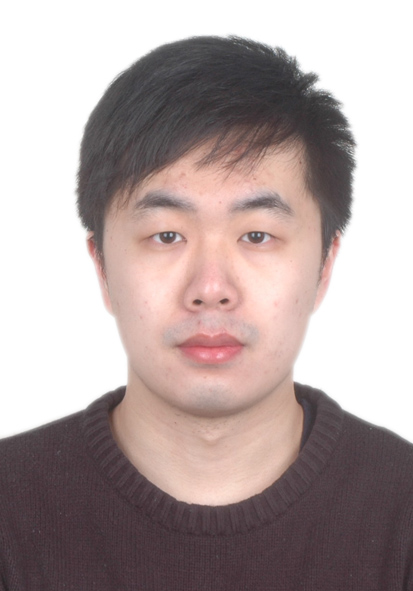
In this thesis, we explore the potential of indoor localization using Bluetooth narrowband radios. To start with, a data model according to the property of the conducted measurement data is developed. The conducted measurement data is radio channel measure- ments based on channel sounding technique. Then the data model is developed as a channel impulse response model and multipath signals are indicated by different time delays.
Delays are estimated after subspace estimation of the data covariance matrix. Smoothing techniques are employed to improve the covariance matrix estimate. To detect the rank of the subspace, two techniques are investigated, namely the MDL algorithm and the threshold method. New estimates for the thresholds are derived, valid for Hankel-structured data matrices. Experiments are conducted to investigate the performance and reliability of those two techniques, under different parameter values.
Next, we consider subspace-based super-resolution algorithm, in particular the MUSIC algorithm. The functionality of the MUSIC algorithm on narrowband radios measurements is tested and evaluated firstly by simulation experiments, which demonstrate the practicability of applying MUSIC algorithm on narrowband radios measurements. Then experiments are extended to the measurement data that conducted from real indoor environments, for the purpose of indoor localization realization using narrowband radios.
MS3 seminar
- Wednesday, 16 October 2019
- 15:30-18:00
- Snijderzaal
Ultra Wide Band Surveillance Radar
Dr. Mark E. Davis, IEEE Fellow, IEEE Distinguished LecturerIEEE
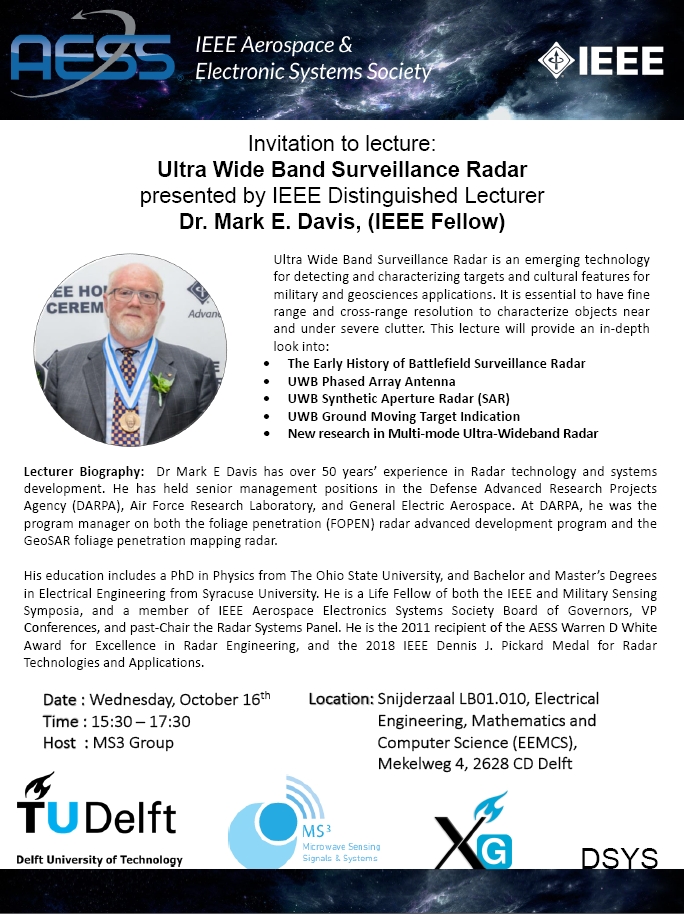
Ultra Wide Band Surveillance Radar is an emerging technology for detecting and characterizing targets and cultural features for military and geosciences applications. It is essential to have fine range and cross-range resolution to characterize objects near and under severe clutter. This lecture will provide an in-depth look into:
- The early history of battlefield surveillance radar
- UWB phased array antenna
- UWB Synthetic aperture radar (SAR)
- UWB ground moving target indication
- New research in multi-node ultra wind band radar
Lecturer Biography: Dr Mark E Davis has over 50 years’ experience in Radar technology and systems development. He has held senior management positions in the Defense Advanced Research Projects Agency (DARPA), Air Force Research Laboratory, and General Electric Aerospace. At DARPA, he was the program manager on both the foliage penetration (FOPEN) radar advanced development program and the GeoSAR foliage penetration mapping radar.
His education includes a PhD in Physics from The Ohio State University, and Bachelor and Master’s Degrees in Electrical Engineering from Syracuse University. He is a Life Fellow of both the IEEE and Military Sensing Symposia, and a member of IEEE Aerospace Electronics Systems Society Board of Governors, VP Conferences, and past-Chair the Radar Systems Panel. He is the 2011 recipient of the AESS Warren D White Award for Excellence in Radar Engineering, and the 2018 IEEE Dennis J. Pickard Medal for Radar Technologies and Applications.
MSc SS Thesis Presentation
- Tuesday, 15 October 2019
- 13:30-14:15
- HB 17.150
Radio astronomy image formation using Bayesian learning techniques
Yajie Tang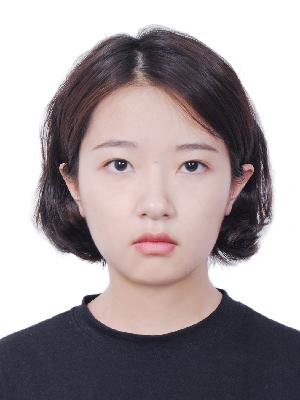
Radio astronomy image formation can be treated as a linear inverse problem. However, due to physical limitations, this inverse problem is ill-posed. To overcome the ill-posedness, side information should be involved. Based on the sparsity assumption of the sky image, we consider L1-regularization. We formulate the image formation problem as a L1-regularized weighted least square (WLS) problem and associate each variable with one regularization parameter. We use Bayesian learning to learn the regularization parameters from data by maximizing the posterior density. With the iterative update of the regularization parameters, the solution is updated until convergence of the regularization parameters. We involve a stopping rule based on the noise level to improve the computational eachciency and control the sparsity of the solution. We compare the performance of this Bayesian learning method with other existing imaging methods by simulations. Finally, we propose some future research directions in improving the performance of this Bayesian learning method.
Signal Processing Seminar
- Thursday, 10 October 2019
- 13:30-14:30
- HB 17.150
Analytical Full-Wave Free Induction Decay Signal Model for MRI
Patrick Fuchs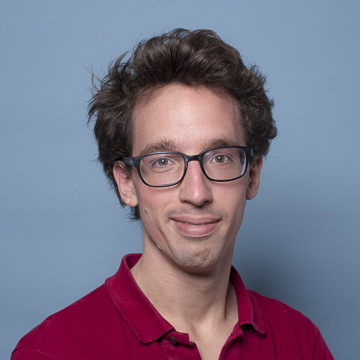
The derivation of the standard signal model in Magnetic Resonance Imaging (MRI) is based on a quasi-static electromagnetic field approximation and is essentially obtained through an application of the Biot-Savart law. Such an approach works fine for relatively low MR background fields (up till 1.5 T, say), but the model may lose its validity at higher static background fields, since the oscillation frequency of the electromagnetic radio-frequency fields is linearly related to the magnitude of this background field via the well-known Larmor equation. Consequently, an increase in the strength of the static background field leads to an increased Larmor frequency and the quasi-static field approximation may no longer be applicable.
In this presentation, We derive a signal model based on the full Maxwell system and no quasi-static field approximations are applied. We show that the measured signal consists of a direct term that relates the measured signal to the time-varying magnetization within the sample and a scattering term that is due to the dielectric contrast of the sample with respect to its surroundings (assuming no contrast in the permeability). Similar to the quasi-static case, the contribution of each term to the measured signal can be expressed in terms of so-called electric and magnetic receive fields, which basically act as frequency-dependent sensitivity functions. Furthermore, the scattering term is expressed in terms of the electric field strength inside the sample but this field is unknown in general. However, if the dielectric constitution of the sample is known then this field can be determined in principle. Additionally, for low-contrast samples, the Born approximation may be applied leading to an explicit analytic full-wave signal model in terms of the magnetization of the sample, its conductivity, and its permittivity. Finally, through simulations, we illustrate the receive field sensitivity functions for different measurement scenarios and show which terms in the signal model provide the largest contribution to the measured signal as a function of receiver location, frequency, and dielectric composition and size of the sample under test.
Additional information ...
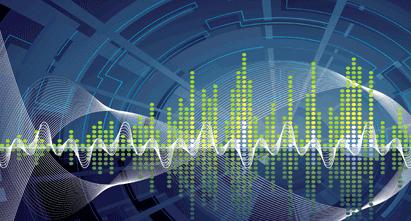
CAS MSc Midterm Presentations
- Tuesday, 8 October 2019
- 10:30-11:00
- HB 17.150
CAS MSc Midterm Presentations
Marnix Abrahams (mid)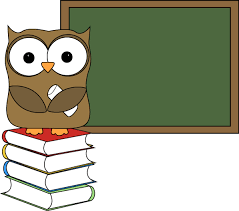
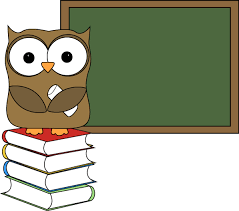
Signal Processing Seminar
- Thursday, 3 October 2019
- 13:30-14:30
- HB 17.150
A Data Scientific Approach to Efficient Submillimeter Astronomical Spectroscopy
Akio TaniguchiNagoya University, Japan
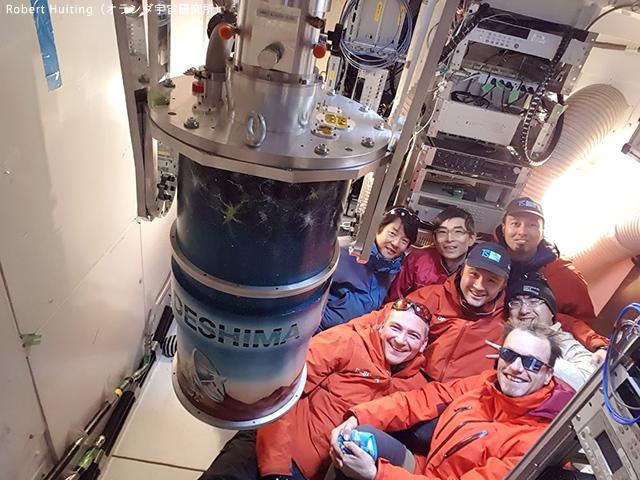
Astronomical data have become huge, as a result of recent advances in wide-field and wide-band instruments. To efficiently extract astronomical signals from observations using these instruments, data scientific approaches are essential. In the (sub)millimeter waveband, spectroscopy with ground-based single-dish telescopes is the best method for surveying interstellar molecules and atoms. However, such observations are not efficient yet, because they always suffer from the intense and time-varying atmosphere of the Earth.
In this talk, I present a statistical method to remove the atmospheric emission from a large spectroscopic dataset by using its intrinsic frequency correlation or spectral shape. As an application, I introduce a recent development of frequency modulation (FM) spectroscopy, which is three times more efficient than a conventional method [1]. As a collaboration with TU Delft, I introduce another application of spectral-cleaning for an ultra-wide-band (UWB) spectrometer DESHIMA [2]. Grasping the UWB atmospheric characteristics by using our data analysis software [3], it removes atmospheric effects on an astronomical spectrum much better than a conventional method.
[1] Akio Taniguchi, Yoichi Tamura et al., "A new off-point-less observing method for millimeter and submillimeter spectroscopy with a frequency-modulating local oscillator (FMLO)", submitted to Publications of the Astronomical Society of Japan (2019)
[2] Akira Endo, Kenichi Karatsu, Yoichi Tamura, Tai Oshima, Akio Taniguchi, ..., Jochem J. A. Baselmans, "First light demonstration of the integrated superconducting spectrometer", Nature Astronomy (2019), Advanced Online Publication https://rdcu.be/bM2FN
[3] Akio Taniguchi, Tsuyoshi Ishida, "De:code - DESHIMA code for data analysis", DOI 10.5281/zenodo.3384216
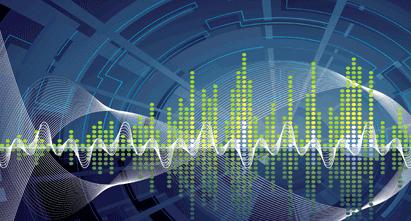
Signal Processing Seminar
- Thursday, 26 September 2019
- 13:30-14:30
- HB 13.100
Local Activation Time estimation in Atrial Electrograms
Bahareh Abdi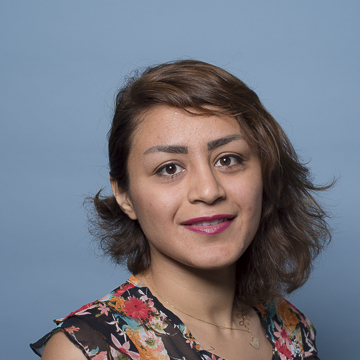
The interpretation of unipolar electrograms is complicated by interference from nonlocal activities of neighbouring tissue. This happens due to the spatial blurring that is inherent to electrogram recordings. In this study, we aim to exploit the high-resolution multi-electrode recordings during atrial mapping to amplify local activities and suppress non-local activities in each of the electrograms. This will subsequently improve the annotation of local deflections and local activation times (LATs) of the electrograms.
According to electrophysiological models, electrogram array can be modelled as a spatial convolution of per cell transmembrane currents with an appropriate distance kernel, which depends on cells’ distances to the electrodes. By deconvolving the effect of the distance kernel from the electrogram array, we undo the blurring and estimate the underlying transmembrane currents as our desired local activities. However, deconvolution problems are typically highly ill-posed and result in unstable solutions.
To overcome this issue, we propose to use a regularization term that exploits the sparsity of the first-order time derivative of the electrograms. We also discuss, in summary, the required electrode array specifications including the spatial resolution and electrode diameter for an appropriate electrogram array recording and subsequent deconvolution.
We perform experiments on simulated two-dimensional tissues, as well as clinically recorded electrograms during paroxysmal atrial fibrillation. The results show that the proposed approach for deconvolution can efficiently amplify the local deflection in fractionated electrograms and attenuate nonlocal activities. This, in turn, improves the annotation of the true LAT in the fractionated electrogram.
Additional information ...
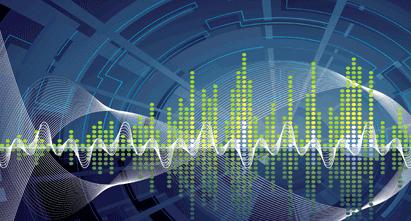
MSc SS Thesis Presentation
- Friday, 20 September 2019
- 10:00-10:45
- HB 17.150
A Generative Neural Network Model for Speech Enhancement
Husain Kapadia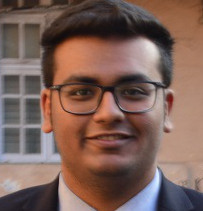
Listening in noise is a challenging problem that affects the hearing capability of not only normal hearing but especially hearing impaired people. Since the last four decades, enhancing the quality and intelligibility of noise corrupted speech by reducing the effect of noise has been addressed using statistical signal processing techniques as well as neural networks. However, the fundamental idea behind implementing these methods is the same, i.e., to achieve the best possible estimate of a single target speech waveform. This thesis explores a different route using generative modeling with deep neural networks where speech is artificially generated by conditioning the model on previously predicted samples and features extracted from noisy speech. The proposed system consists of the U-Net model for enhancing the noisy features and the WaveRNN synthesizer (originally proposed for text-to-speech synthesis) re-designed for synthesizing clean sounding speech from noisy features. Subjective results indicate that speech generated by the proposed system is preferred over listening to noisy speech, however, the improvement in intelligibility is limited.
Additional information ...
Signal Processing Seminar
- Thursday, 12 September 2019
- 13:30-14:30
- HB 17.150
Signal Processing for reduced hardware radio astronomy imaging
Manuel Stein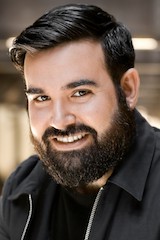
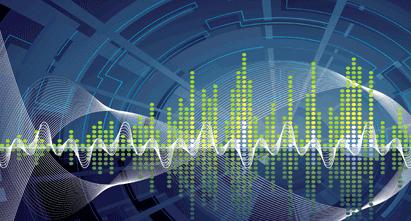
CAS MSc Midterm Presentations
- Tuesday, 10 September 2019
- 10:30-11:00
- HB 17.150
Erik Hagenaars (mid)
Erik Hagenaars (mid)
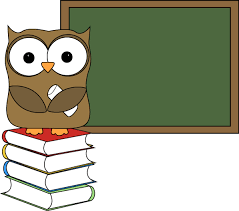
MSc ME Thesis Presentation
- Friday, 30 August 2019
- 10:00-10:30
- Lecture Hall 10 in PULSE, building 33a
A low-noise amplifier for ultrasound imaging with continuous time-gain compensation
Qiyou Jiang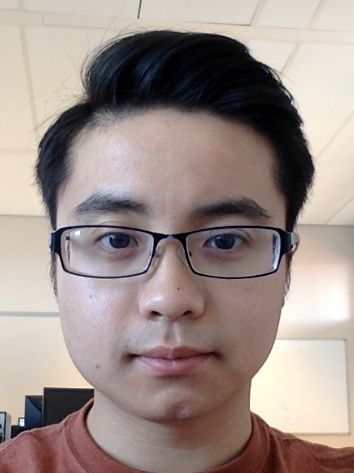
This work presents a low-noise amplifier (LNA) for ultrasound imaging with built-in continuous time-gain compensation (TGC), which compensates for the time-dependent attenuation of the received echo signal and thus significantly reduces its dynamic range (DR).
The proposed design combines the LNA and TGC functions in a single variable-gain current-to-current amplifier. Compared to conventional ultrasound front-ends, which implement the TGC function after an LNA that needs to handle the full DR of the echo signal, this approach can highly reduce the power consumption and the size. Compared to earlier programmable gain LNAs with discrete gain steps, the continuous gain control avoids switching transients that may lead to imaging artefacts.
The TGC function is realized by a novel feedback network consisting of a double differential pair that feeds a fraction of the output current back to the input. This fraction can be changed continuously using a control voltage that is applied to the gates of the differential pairs, to realize a gain range from -20 dB to +20 dB. To achieve an approximately constant closed-loop bandwidth in the presence of the changing feedback factor, a loop amplifier has been implemented whose gain is changed along with the feedback factor by dynamically changing its bias currents. This loop amplifier employs a current-reuse architecture to achieve high power-efficiency. In addition, a variable bias current source has been designed to appropriately bias the TGC feedback network. By employing a similar double differential pair topology as in the feedback network, this current source provides the required low noise at the highest gain setting and high current at the lowest gain setting within the available headroom.
The LNA with built-in TGC function has been realized in 180nm CMOS technology. It has been optimized to interface with a 7.5 MHz capacitive micro-machined ultrasonic transducer (CMUT). Simulation results show that it achieves a 3dB bandwidth higher than 40 MHz across the full gain range. At the highest gain setting, its input current noise is 0.96 pA/rt-Hz at 7.5 MHz. This leads to an input dynamic range of 93 dB, which is compressed into an output dynamic range of 53 dB by means of the 40 dB variable gain. The amplifier consumes 10.8 mW from a 1.8V supply, and occupies an estimated 320 x 320 um2 die area.
MSc SS Thesis Presentation
- Thursday, 29 August 2019
- 10:00-10:45
- EWI HB 17.150
Spoofing detection in a loosely coupled GNSS and INS system via Synthetic Arrays
Kostadin Biserkov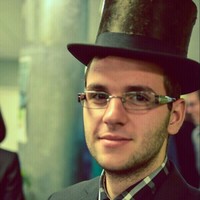
Master thesis defence
- Monday, 26 August 2019
- 11:00
Multiple-input Multiple-output Grating Lobe Selection Scheme for Radar Applications
Nick Cancrinus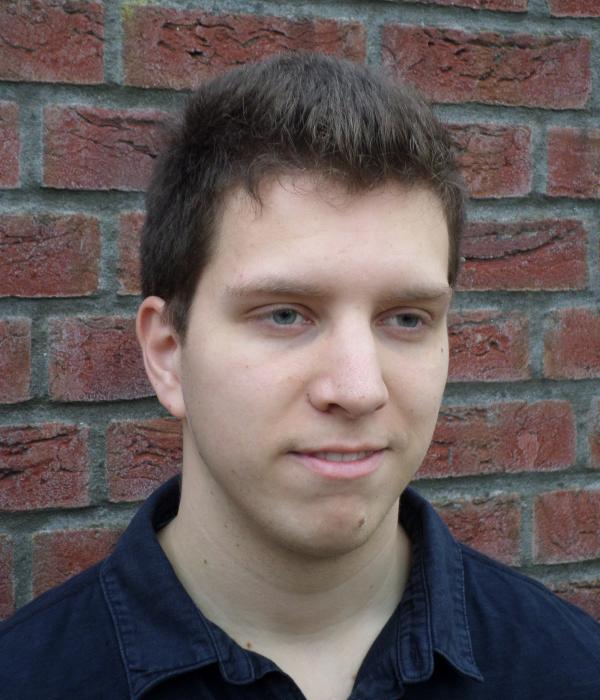
MSc SS Thesis Presentation
- Thursday, 22 August 2019
- 14:00-14:45
- EWI HB 17.150
Calibration of single element 3D ultrasound with a aberration mask
Bram Visser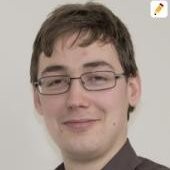
Previous work has demonstrated the possibility of high-resolution 3D ultrasound imaging through the use of a single element and an aberration mask. This thesis will expand on the previous work by examining the proposed method for errors in the creation of the model.
The analysis is performed by examining the various aspects of the measurements setup and underlying theoretical model, after which measurements are performed to determine their contribution and correctness with regard to the model. Results demonstrated a systematic error of a non-linear frequency scaling and semi-linear phase shift. The origin of the error lies in the unwanted addition of transfer functions of some of the components. A Tikhonov regularized least squares method is proposed to estimate this transfer function and supply compensation based on all the measurements.
The results of the application of this method on the uncalibrated model are demonstrated through 1D imaging experiments. The result of which shows a significant improvement over the previous uncalibrated results. After which the possibility of calibration due to a singular measurement is explored and an adaptation of the Tikhonov regularized least squares method is proposed for a close approximation of the previously found transfer function. Further to obtain an indication of possible remaining hurdles and successes with this method, extensive simulations are performed to examine the individual impact of various sources of noise and interference.
Additional information ...
CAS MSc Midterm Presentations
- Tuesday, 16 July 2019
- 10:00-11:30
- HB 17.150
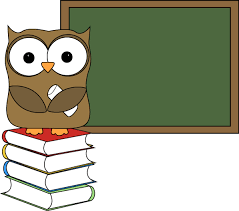
CAS MSc Midterm Presentations
- Tuesday, 16 July 2019
- 11:00-11:30
- HB 17.150
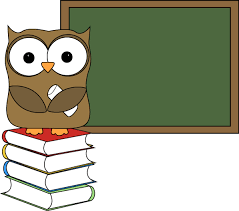
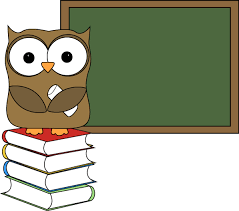
MSc TC Thesis Presentation
- Thursday, 11 July 2019
- 10:00-10:40
- EWI HB 17.150
Development of Data Processing Algorithms for UWB Radar-based Long-Term Health Monitoring
Yiting Lu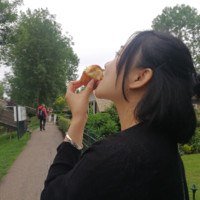
In the last two decades, a lot of attention has been focused on contactless radar-based vital signs monitoring (heartbeat and respiration rate) as an emerging and complementary value to our medical care. It is very challenging in real indoor environments to perform concurrent localization and reliable vital signs monitoring of multiple subjects within practical distance ranges. In fact, the multipath propagation results in the reflected signal dispersed in time, which not only causes false ToF (Time of Flight) estimation but also leads to inter-subject interference, jeopardizing the vital signs extraction and the localization.
Here we show a methodology based on radar techniques to automatically locate multiple subjects in indoor environments while keep monitoring their vital signs. This approach, based on the parametric models both of the propagation channel and of the radar signals, is able to cancel the undesired contributions from static clutters and multipath components, by which it is possible to accurately locate the subjects and extract their heart rates and respiration rates.
CAS MSc Midterm Presentations
- Tuesday, 2 July 2019
- 10:00-11:30
- HB 17.150
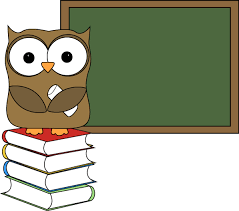
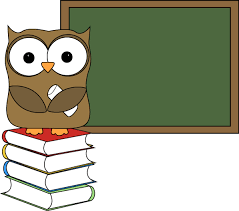
Signal Processing Seminar
- Thursday, 27 June 2019
- 13:30-14:30
- HB 17.150
Biomedical Signal Processing
Miao Sun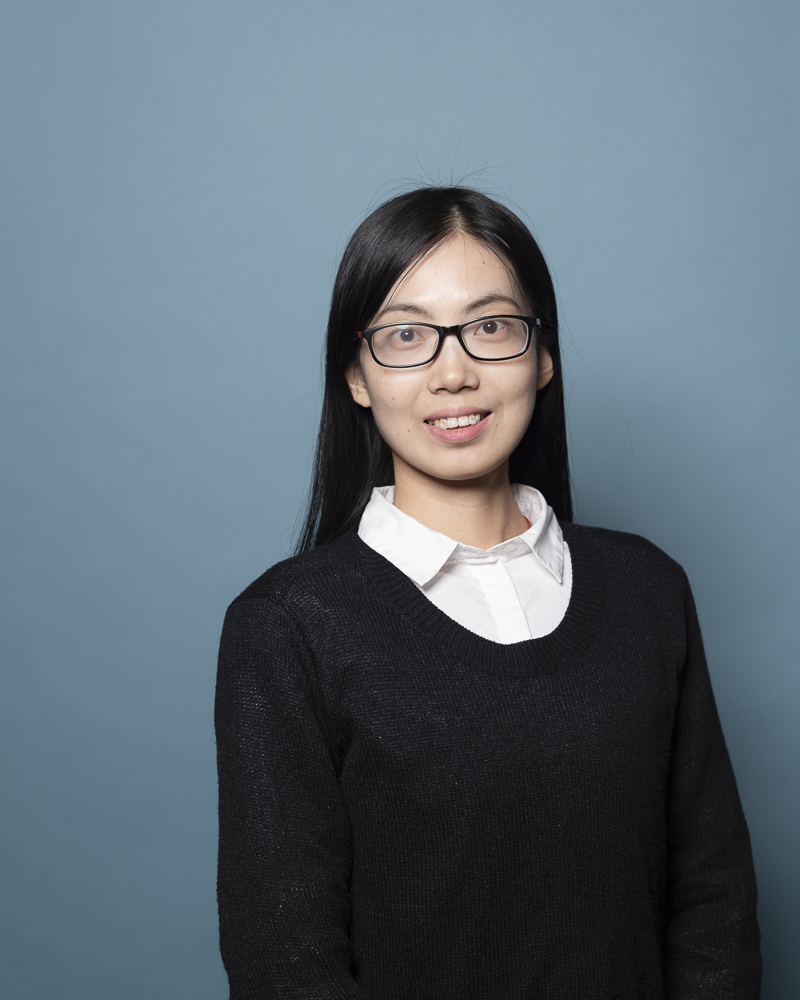
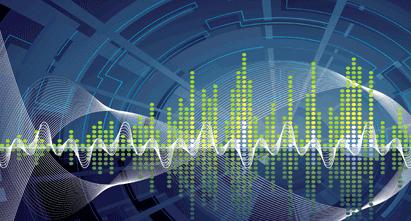
MSc SS Thesis Presentation
- Wednesday, 26 June 2019
- 10:00-10:45
- Snijderszaal LB01.010
Gradient Coil Design and Construction for a Halbach Based MRI System
Bart de Vos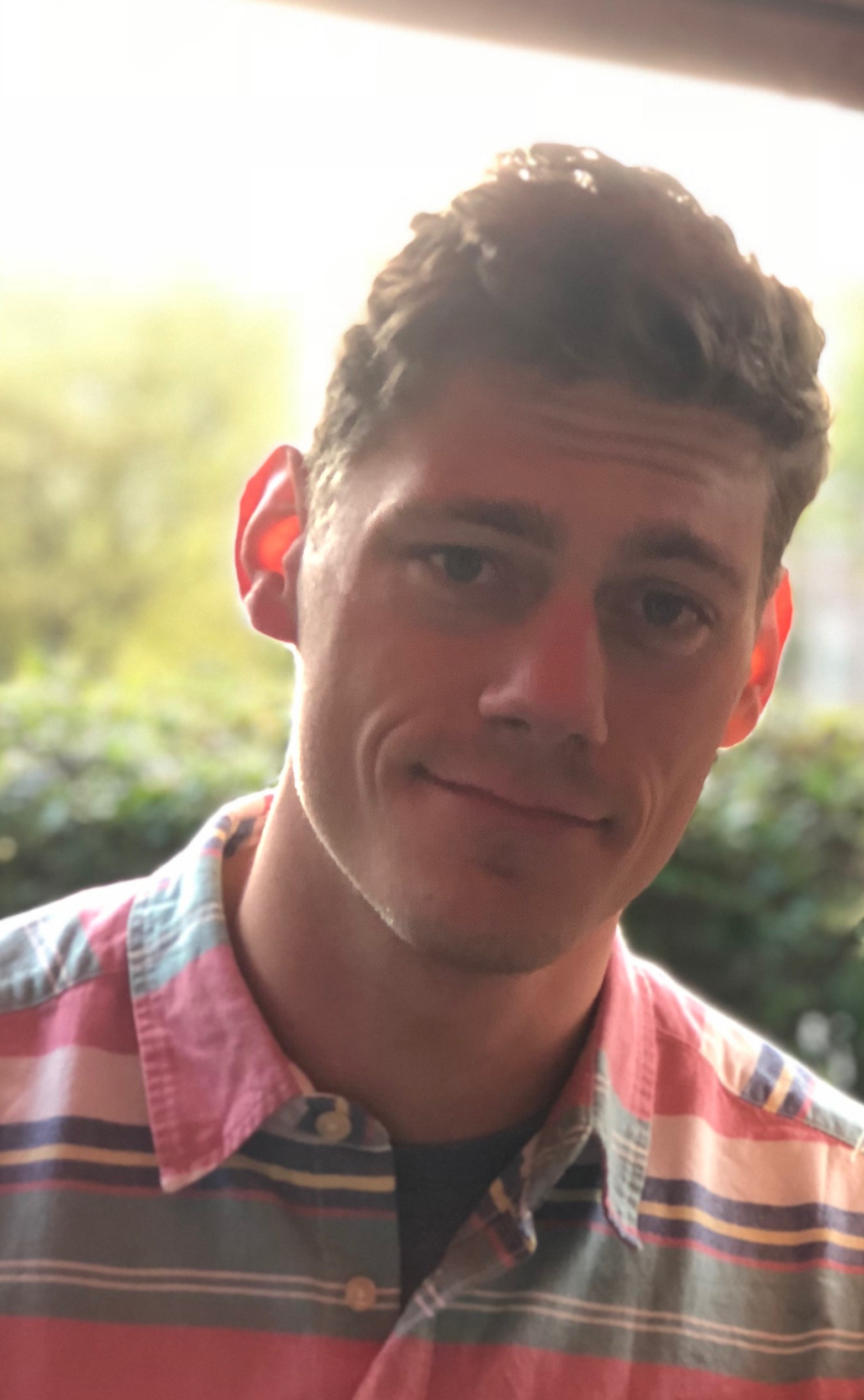
MRI as a medical diagnostics tool is still unavailable to the majority of the developing world. Therefore the design and development of new low-cost hardware are essential. The design of gradient coils corresponding to this hardware is necessary for conventional imaging and reconstruction methods to be used.
The target field method, which was originally developed to deal with longitudinal main magnetic fields, is applied to a transverse field, as produced by a Halbach permanent magnet array. Using this method current densities for gradient fields in the three spatial directions are derived. Subsequently, using stream functions, wire patterns for the three gradient coils are determined. These are verified using a commercial magneto-static solver. Furthermore, one of the gradients is constructed to validate the performance of the method.
The measured fields are in good agreement with the simulations and their prescribed target fields. This confirms that the proposed method provides a reliable way to design and manufacture gradient coils for various requirements. Based on the experimental review of the constructed coil three optimized gradients are proposed for the low field MRI system developed at the LUMC in cooperation with the TU Delft. The method can also be readily generalized to other geometries and requirements due to the robust fundamental physical basis and accuracy with respect to computer simulations.
Microelectronics Colloquium
- Tuesday, 25 June 2019
- 11:00-12:00
- EWI-Lecture hall Chip
Quantum Computer on a Chip
Bogdan StaszewskiUniversity College Dublin
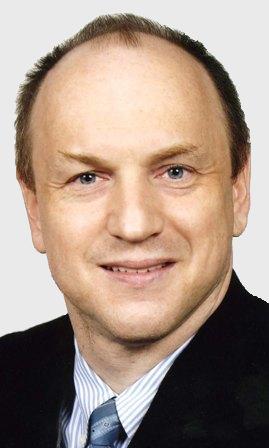
Quantum computing is a new paradigm that exploits fundamental principles of quantum mechanics, such as superposition and entanglement, to tackle problems in mathematics, chemistry and material science that are well beyond the reach of supercomputers. Despite the intensive worldwide race to build a useful quantum computer, it is projected to take decades before reaching the state of useful quantum supremacy. The main challenge is that qubits operate at the atomic level, thus are extremely fragile, and difficult to control and read out. The current state-of-art implements a few dozen magnetic-spin based qubits in a highly specialized technology and cools them down to a few tens of millikelvin. The high cost of cryogenic cooling prevents its widespread use. A companion classical electronic controller, needed to control and read out the qubits, is mostly realized with room-temperature laboratory instrumentation. This makes it bulky and nearly impossible to scale up to the thousands or millions of qubits needed for practical quantum algorithms.
As part of our startup company, we propose a new quantum computer paradigm that exploits the wonderful scaling achievements of mainstream integrated circuits (IC) technology which underpins personal computers and mobile phones. Just like with a small IC chip, where a single nanometer-sized CMOS transistor can be reliably replicated millions of times to build a digital processor, we propose a new structure of a qubit realized as a CMOS-compatible charge-based quantum dot that can be reliably replicated thousands of times to construct a quantum processor. Combined with an on-chip CMOS controller, it will realize a useful quantum computer which can operate at a much higher temperature of 4 kelvin.
Additional information ...
MS3 seminar
- Friday, 21 June 2019
- 14:00-16:30
- EWI Van der Poelzaal
Diversity and nonlinear processing: trends for future radar systems
Prof. Dr. Stéphanie BidonDepartment of Electronics, Optronics and Signal at ISAE-SUPAERO, Université de Toulouse, France
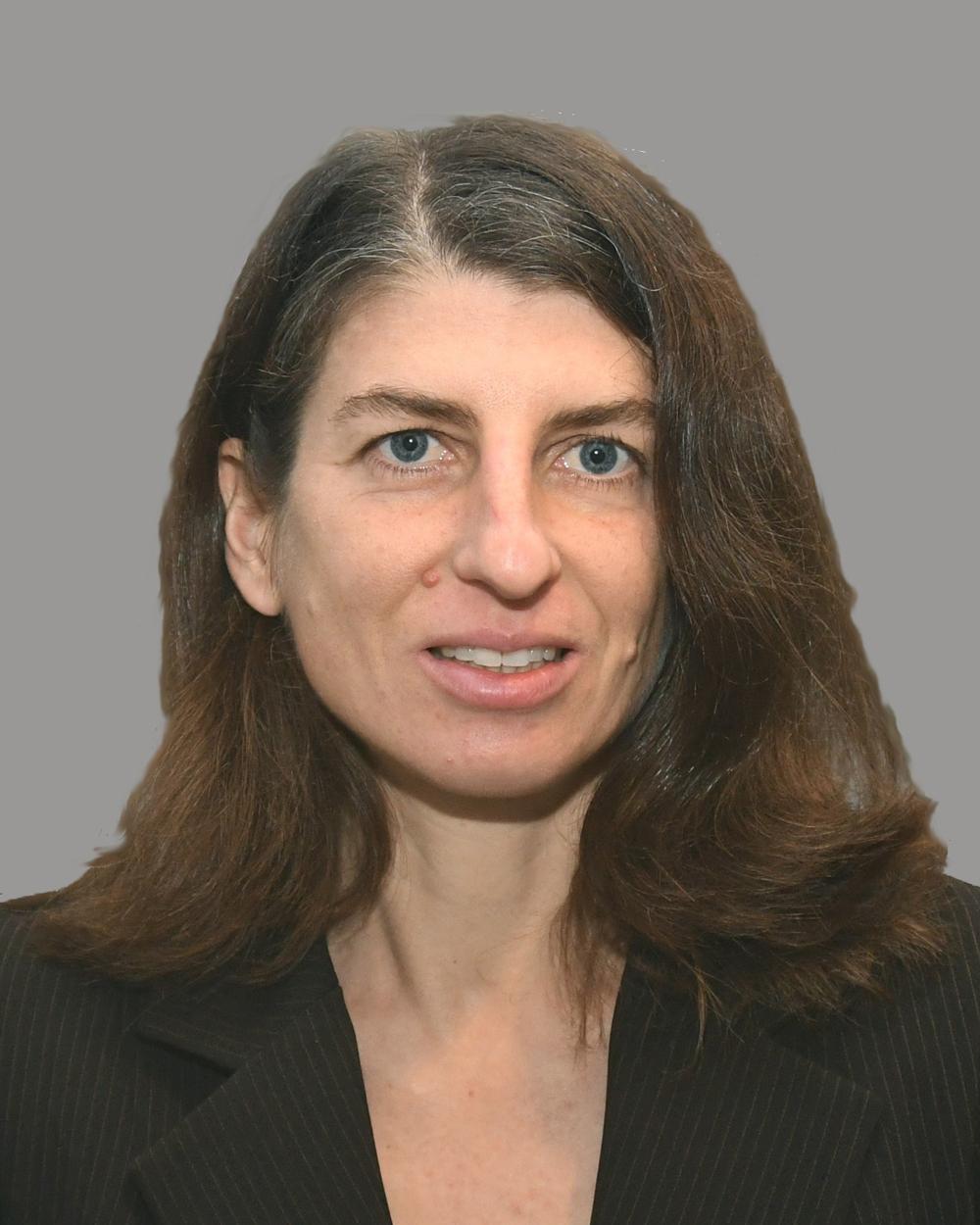
Radar is an exciting field where systems are constantly evolving thanks to technical advances in several domains including RF, electronics and signal processing. Focusing on the latter, this talk illustrates two important trends that are contributing to the development of future radar systems, namely diversity and nonlinear techniques. On the one hand, diversity brings redundant information about the radar scene thereby enabling target discrimination in a given space. On the other hand, nonlinear techniques produce outputs that are not linearly related to the input signals thereby enabling relevant processing in complex scenarios. Benefits of combining both diversity and nonlinear algorithms will be presented in two radar applications: 1) to estimate range migrating targets in blind velocities with a wideband waveform 2) to estimate targets hidden in the pedestal inherent to a multicarrier waveform.
Short bio
Stéphanie Bidon received the engineer degree in aeronautics and the master degree in signal processing from ENSICA, Toulouse, in 2004 and 2005 respectively. She obtained the Ph.D. degree and the Habilitation à Diriger des Recherches in signal processing from INP, Toulouse, in 2008 and 2015 respectively.
She is currently with the Department of Electronics, Optronics and Signal at ISAE-SUPAERO, Université de Toulouse, France, as a professor.
Her research interests include digital signal processing particularly with application to radar systems (STAP, wideband radar detection, RadCom) and GNSS (robust phase tracking).
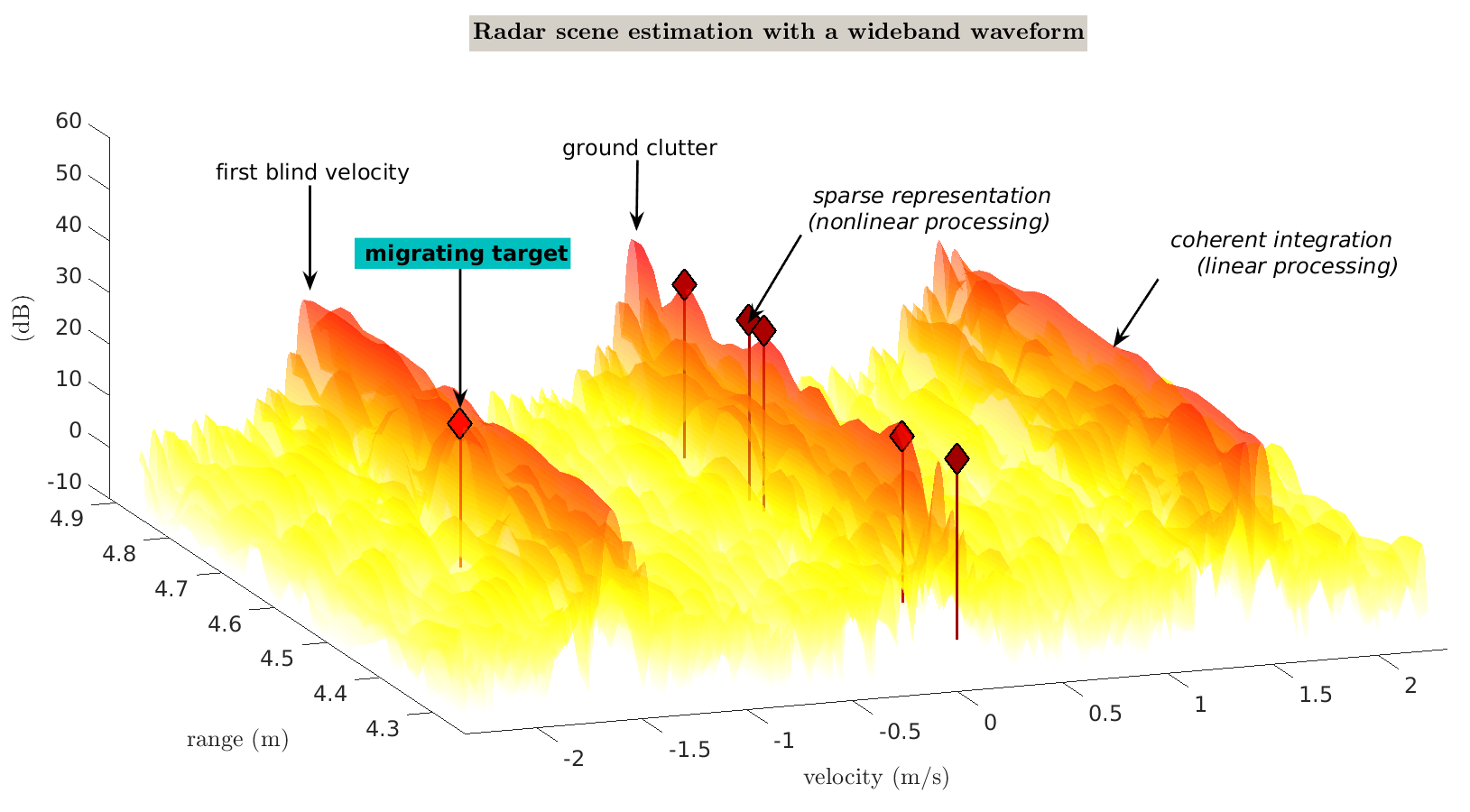
MS3 seminar
- Friday, 21 June 2019
- 14:00-16:30
- EWI Van der Poelzaal
Misspecification, Robustness and Cognition in Radar Signal Processing: Some Results
Prof. Dr. Maria Sabrina GrecoDept. of Information Engineering of the University of Pisa
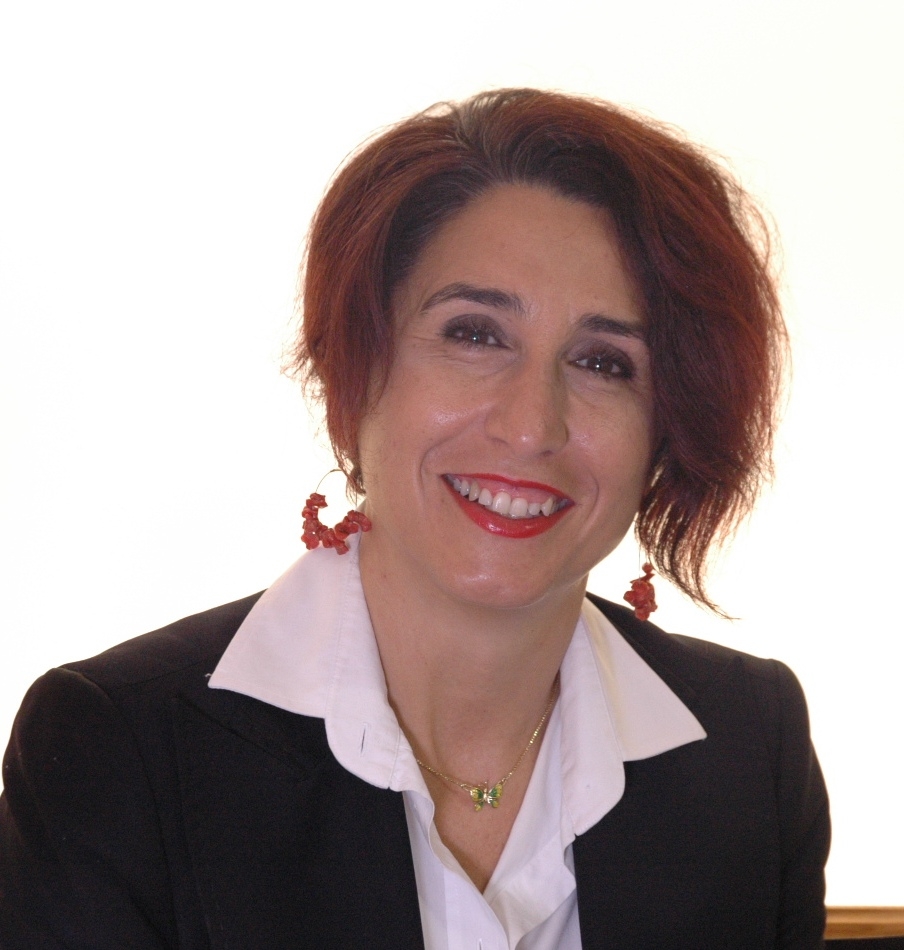
After a brief survey of the activities of the Radar Signal Processing Group of the Dept. of Information Engineering, University of Pisa, the talk will focus on some of the recent and on-going research topics in which Prof. Greco is involved.
Any scientific experiment which aims to gain some knowledge about a real-word phenomenon, in radar systems as in other applications, starts with the data collection. In statistical signal processing, all the available knowledge about a physical phenomenon of interest is summarized in the probability density function (pdf) of the collected observations. In practice, the pdf or/and its characteristic parameters are partly or fully unknown, then any inference procedure starts with its estimation. The easy case is when the hypothesized statistical model and the true one are the same, so they are matched. However, a certain amount of mismatch is often inevitable in practice. The reasons for a model misspecification can be various: it may be due to an imperfect knowledge of the true data model or to the need to fulfill some operative constraints on the estimation algorithm (processing time, simple hardware implementation, and so on).
The first part of the talk aims at providing a short overview on the misspecified estimation framework with a particular focus on the Misspecified Cramér-Rao bound (MCRB). Then a possible approach to minimize the misspecification risk is presented. Specifically, a more general semiparametric characterization of the statistical behavior of the collected data is addressed and some application to the radar scenario is shown.
The talk will then continue with a short introduction to the concept of cognition applied to passive and active radars highlighting the limits and the path forward and will describe some new results regarding the application of some machine learning techniques to “cognitive” MIMO radar.
Short Bio
Maria Sabrina Greco graduated in Electronic Engineering in 1993 and received the Ph.D. degree in Telecommunication Engineering in 1998, from University of Pisa, Italy. From December 1997 to May 1998 she joined the Georgia Tech Research Institute, Atlanta, USA as a visiting research scholar where she carried on research activity in the field of radar detection in non-Gaussian background.
In 1993 she joined the Dept. of Information Engineering of the University of Pisa, where she is Full Professor since 2017. She’s IEEE fellow since Jan. 2011 and she was co-recipient of the 2001 and 2012 IEEE Aerospace and Electronic Systems Society’s Barry Carlton Awards for Best Paper and recipient of the 2008 Fred Nathanson Young Engineer of the Year award for contributions to signal processing, estimation, and detection theory. In May-June 2015 and in January-February 2018 she visited as invited Professor the Université Paris-Sud, CentraleSupélec, Paris, France.
She has been general-chair, technical program chair and organizing committee member of many international conferences over the last 10 years. She has been guest editor of the special issue on “Machine Learning for Cognition in Radio Communications and Radar” of the IEEE Journal on Special Topics of Signal Processing, lead guest editor of the special issue on "Advanced Signal Processing for Radar Applications" of the IEEE Journal on Special Topics of Signal Processing, December 2015, guest co-editor of the special issue of the Journal of the IEEE Signal Processing Society on Special Topics in Signal Processing on "Adaptive Waveform Design for Agile Sensing and Communication," published in June 2007 and lead guest editor of the special issue of International Journal of Navigation and Observation on” Modelling and Processing of Radar Signals for Earth Observation published in August 2008. She’s Associate Editor of IET Proceedings – Sonar, Radar and Navigation, member of the Editorial Board of the Springer Journal of Advances in Signal Processing (JASP), and Senior area chair of the IEEE Transactions on Signal Processing. She’s member of the IEEE AESS Board of Governors and has been member of the IEEE SPS BoG (2015-17) and Chair of the IEEE AESS Radar Panel (2015-16). She has been as well SPS Distinguished Lecturer for the years 2014-2015, and now she's AESS Distinguished Lecturer for the years 2015-2019, and AESS VP Publications.
Her general interests are in the areas of statistical signal processing, estimation and detection theory. In particular, her research interests include clutter models, coherent and incoherent detection in non-Gaussian clutter, CFAR techniques, radar waveform diversity and bistatic/mustistatic active and passive radars, cognitive radars. She co-authored many book chapters and more than 190 journal and conference papers.
Signal Processing Seminar
- Thursday, 20 June 2019
- 13:30-14:30
- HB 17.150
Low-complexity 1-bit A/D conversion
Manuel Stein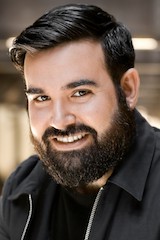
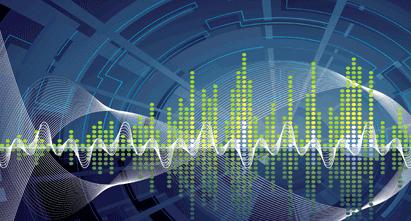
Signal Processing Seminar
- Thursday, 20 June 2019
- 14:15-15:00
- HB 17.150
Deep learning in ultrasound imaging
Ruud van SlounTU Eindhoven
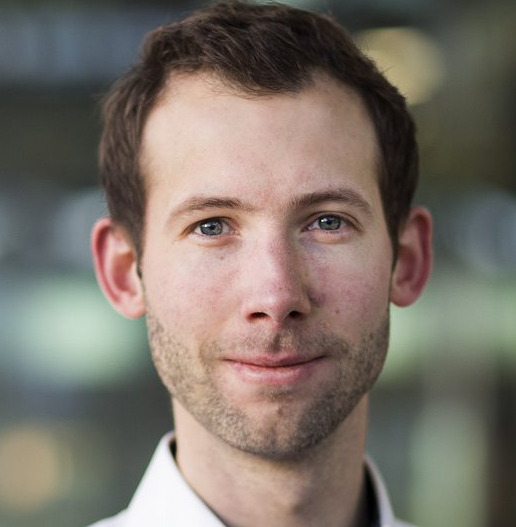
This talk will elaborate on deep learning strategies in ultrasound systems, from the front-end to advanced applications, thereby discussing the possible impact of deep learning methodologies on many aspects of ultrasound imaging. In particular, it will outline methods that lie at the interface of signal acquisition and machine learning, exploiting both data structure (e.g. sparsity in some domain) and data dimensionality (big data) already at the raw radio-frequency channel stage. Several illustrative examples will be given, covering efficient and effective deep learning solutions for adaptive beamforming and adaptive spectral Doppler through artificial agents, learning of compressive encodings for color Doppler, and a framework for structured signal recovery by learning fast approximations of iterative minimization problems, with applications to clutter suppression and super-resolution ultrasound. These emerging technologies may have a considerable impact on ultrasound imaging, showing promise across key components in the receive processing chain.
Additional information ...
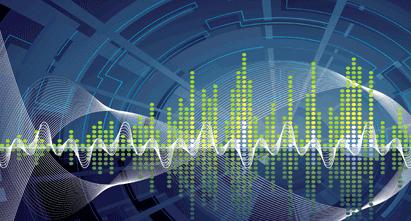
MSc CE Thesis Presentation
- Wednesday, 19 June 2019
- 13:30-14:15
- HB 17.150
A Real-time Low Latency Signal Concentrator for Ship Tracking using AIS
Ramkoemar Bhoera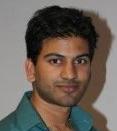
Global AIS coverage is not possible with terrestrial AIS as base stations are required to be build on sea, which is impractical. With the use of LEO satellites, the field of view of a single receiver is increased and is capable of communicating with many AIS cells simultaneously. As many vessels are transmitting data to the same receiver, message collisions occur which results in data loss.
In order to increase the performance of the AIS receiver, blind beamforming techniques are used. This makes it possible to separate multiple collided messages. This solution is build in a hardware receiver which returns analog signals.
The goal of this thesis is to build a low latency data acquisition system, in order to process the signals from the hardware receiver. This system requires a processing board to send the samples over to the single user receiver, which is build in software.
The Raspberry Pi is used as the processing board, but as it was unable to do the realtime work, a microcontroller is added for this specific task. Fetching data from the ADC is realized through the popular Industrial IO subsystem which allows easy integration with other IIO compliant software and is used to transfer sample data over the network to the software receiver. The software receiver decodes the AIS data and sends it to chart plotter applications which can be used to plot data on a map.
Additional information ...
CAS MSc Midterm Presentations
- Tuesday, 18 June 2019
- 10:30-10:45
- HB 17.150
CAS MSc Midterm Presentations
Marnix Abrahams (1st)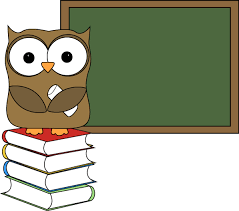
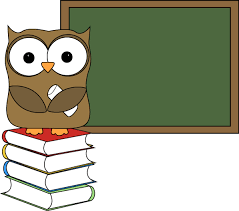
CAS MSc Midterm Presentations
- Tuesday, 18 June 2019
- 10:00-10:30
- HB 17.150
CAS MSc Midterm Presentations
Xi An (mid)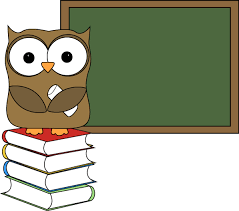
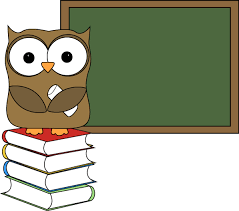
CAS MSc Midterm Presentations
- Tuesday, 18 June 2019
- 11:00-11:30
- HB 17.150
CAS MSc Midterm Presentations
Joris Belier (mid)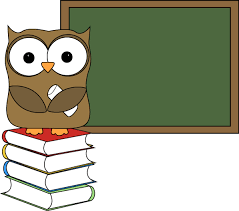
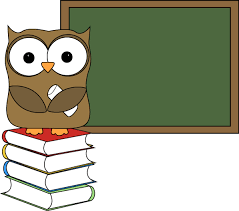
CAS MSc Midterm Presentations
- Tuesday, 18 June 2019
- 10:00-11:30
- HB 17.150
CAS MSc Midterm Presentations
Joppe Lauriks (mid)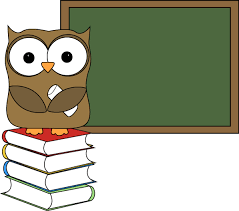
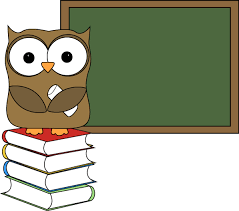
Signal Processing Seminar
- Thursday, 13 June 2019
- 13:30-14:30
- HB 17.150
Task-cognizant sparse sensing for inference (ASPIRE)
Pim van der Meulen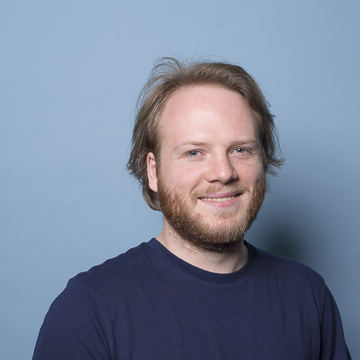
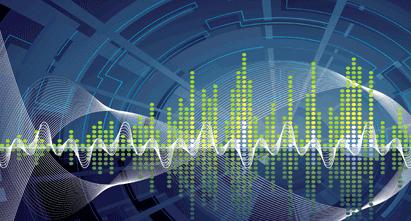
Signal Processing Seminar
- Thursday, 6 June 2019
- 13:30-14:30
- HB 17.150
Acoustic signal processing
Jamal Amini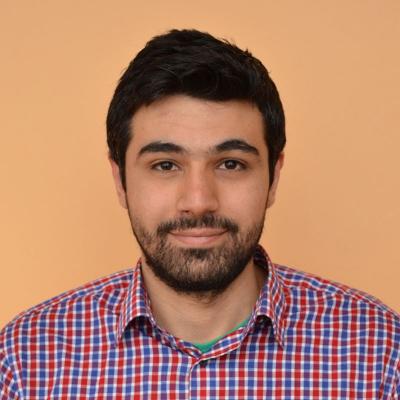
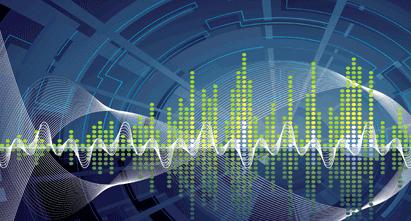
CAS MSc Midterm Presentations
- Tuesday, 4 June 2019
- 10:00-11:30
- HB 17.150
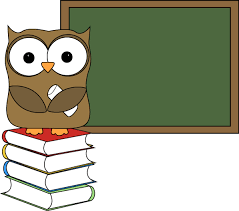
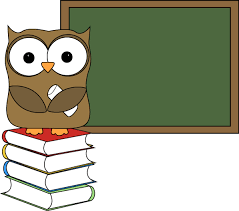
Signal Processing Seminar
- Thursday, 30 May 2019
- 13:30-14:30
- HB 17.150
Biomedical signal processing
Bahareh Abdi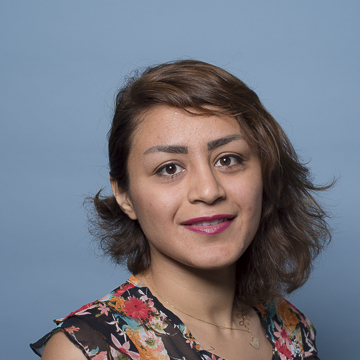
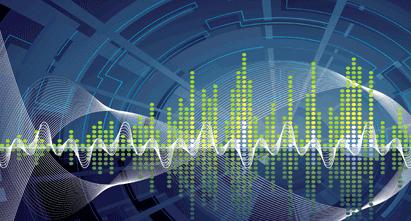
Signal Processing Seminar
- Wednesday, 29 May 2019
- 10:00-10:45
- HB 17.150
Acoustic localization
Michał MachnickiMicroflown
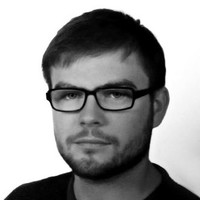
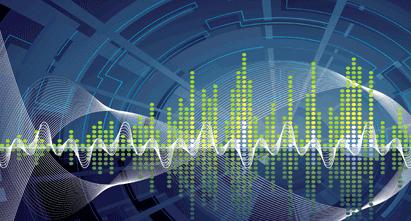
Signal Processing Seminar
- Friday, 24 May 2019
- 14:30-15:30
- HB 17.150
Adaptive classification of radar emitters
Aybuke ErolMETU, Turkey
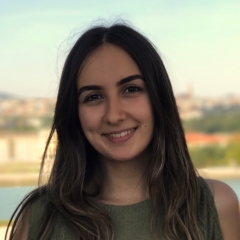
Radar receivers collect interleaved signals from all electromagnetic sources in the environment. The ultimate goal of electronic intelligence is to separate these sources (deinterleaving) and find their types (emitter identification). Knowing the type of a source, it is possible to comment on its mission and operation. All in all, deinterleaving and emitter identification together build a system that solves an adaptive classification problem. One of the biggest challenges in this problem is that the system does not know all emitter types in the world since a great part of this information is confidential within each country. In addition, radar receivers sequentially provide radar pulses to the system. Therefore, the classifier should be able to increase its number of classes whenever an unfamiliar emitter type is encountered. What’s more, it should be able to distinguish between the unfamiliar emitter types, which enforces online learning.
The proposed system solves deinterleaving using fuzzy ARTMAP due to several reasons. First, it is supervised which makes the system able to start with a priori information or data. Secondly, it works with sequential input and enables online learning. Last but not least, it can increase its number of classes. After fuzzy ARTMAP, radar clusters are formed. Next, a representation for each cluster should be found, to be compared with the representations of already known emitter types. The challenge here is that describing an emitter type by single numeric values would not be fair as radar features are generally interval based. For example, emitters today do not operate on a single frequency, they rather have a frequency range in which they can operate. Hence, the representation and comparison of emitter types and radar clusters are considered under symbolic data analysis. Both parts, solved with fuzzy ARTMAP and symbolic data analysis, are improved in terms of classification accuracy from their baseline methods with the use of Jaccard index.
CAS MSc Midterm Presentations
- Tuesday, 21 May 2019
- 10:00-11:30
- HB 17.150
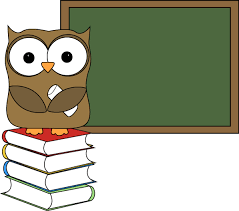
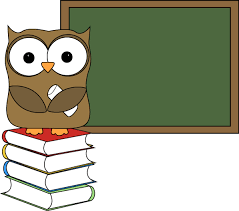
CAS MSc Midterm Presentations
- Tuesday, 21 May 2019
- 11:00-11:30
- HB 17.150
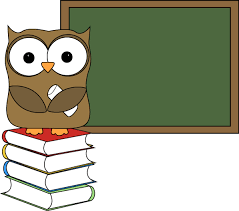
MSc SS Thesis Presentation
- Tuesday, 21 May 2019
- 10:00-10:45
- HB 17.150
Detecting Electrode Array Tip Fold-over in Cochlear Implantation
Juriaan van der Graaf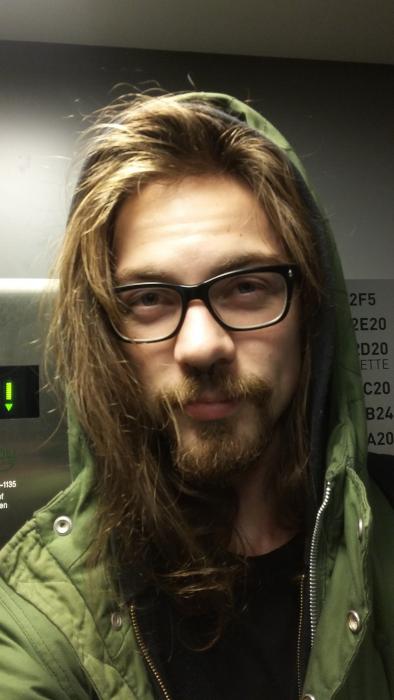
In cochlear implantation surgery, the appropriate placement of the electrode array into the cochlea is vital. Suboptimal placement of the electrode array may lead to reduced hearing performance and speech recognition after the surgery. Currently, there are methods to confirm the electrode position post-operatively (e.g. through a CT scan), but it is not possible to monitor the insertion intra-operatively. This, combined with the fact that there is difference in surgical precision and insertion technique between surgeons, leads to great variability in electrode placement and in some cases to electrode malpositioning issues. One of the more problematic issues that may arise is folding of the electrode tip. Folding of the tip causes the electrode array to not reach deep enough into the cochlea, and it is likely to cause trauma due to the increased pressure on cochlear walls and membranes. On top of that, you effectively have “less” contacts to work with because contacts can be positioned very close to eachother due to the folding. Folding of the electrode array also disrupts the tonotopic organization of the cochlear implant (the contacts near the end of the array no longer correspond to the lowest frequencies). The effectivity of the treatment is thus reduced in patients with tip fold-overs. However, many modern day cochlear implants possess telemetry features. These are primarily used to check the implant’s proper functioning, but may also be helpful in monitoring the insertion of the electrode array. The telemetry features of a cochlear implant make it possible to measure the intracochlear electrical potential. The measured current spread is related to the electrode array’s shape and position, and thus may provide a way to detect folding of the tip. This can be done post-operatively, but can possibly also be done intra-operatively to monitor the insertion in real time. This application could be a useful tool to aid surgeons and clinicians. When used post-operatively, it may provide a cost-free method to detect tip fold-over. When used intra-operatively, it may provide a way to detect and prevent both fold-over and trauma to the cochlea.
Additional information ...
Conferences
- Monday, 20 -- Friday, 24 May 2019
- Lecce, Italy
IEEE-EURASIP Summer School on Network- and Data-driven Learning: Fundamentals and Applications
Geert Leus, e.a.IEEE-EURASIP
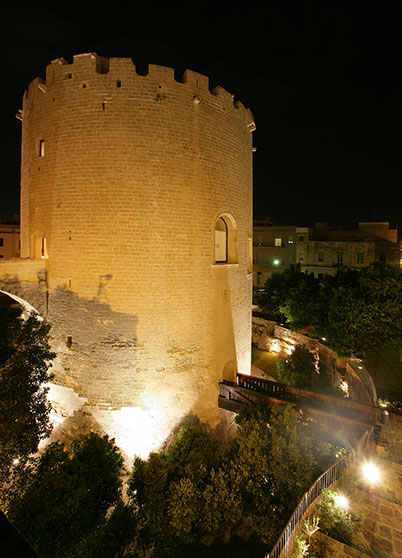
The 2019 IEEE-EURASIP Summer School on “*Network- and Data-driven Learning: Fundamentals and Applications*,” will take place from May 20 to May 24 in the beautiful city of Lecce, Italy.
It will bring together researchers to share exciting advances in network and data sciences theory and applications.
The event will host students interested in signal processing, offering them opportunities to network with world-renowned professors and industry researchers as well as to engage in hands-on tutorials in signal processing and machine learning. In addition to the beautiful ambiance offered by /“The Florence of the South of Italy,” /attendants will benefit from a stimulating environment to learn about the latest advances in an exciting field. Students will have the possibility to present their current research work in a poster session.
The technical focus of this summer school is on fundamentals and algorithmic advances for learning from large volumes of data, with emphasis on network (i.e., graph) data.
More information about the Technical Program, the speakers and the registration procedure, can be found at the webpage.
Additional information ...
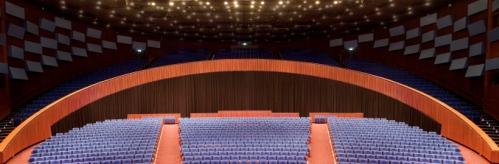
Inauguration Earl McCune and Cicero Vaucher
- Friday, 17 -- Friday, 17 May 2019
- 15:00-18:00
- TU Delft Auditorium
Who's talking, who's listening?
Earl McCune, Cicero VaucherTU Delft
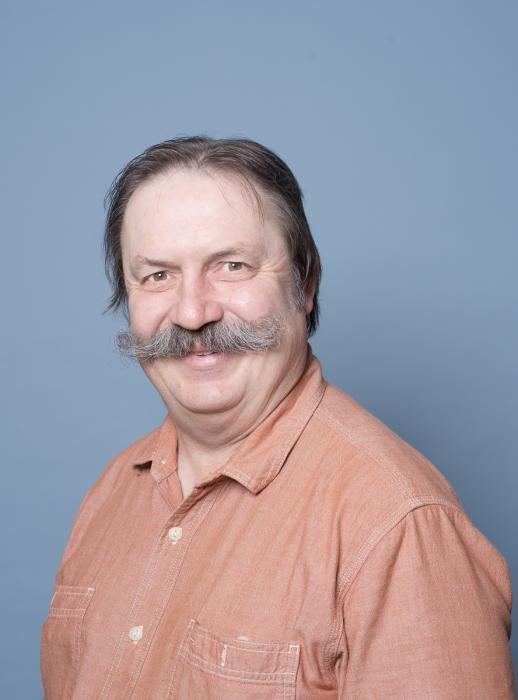
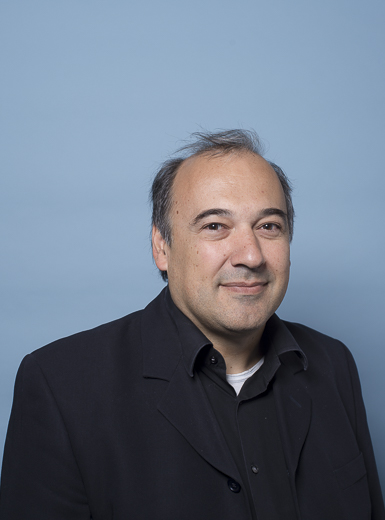
CAS MSc Midterm Presentations
- Tuesday, 14 May 2019
- 11:00-11:30
- HB 17.150
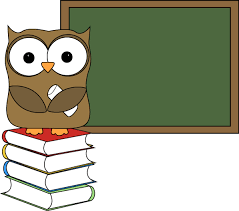
CAS MSc Midterm Presentations
- Tuesday, 7 May 2019
- 10:00-11:30
- HB 17.150
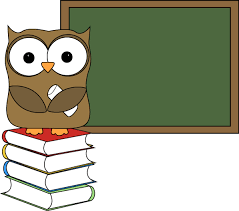
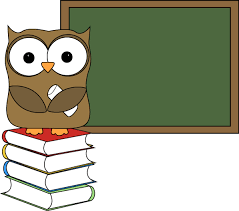
CAS MSc Midterm Presentations
- Tuesday, 30 April 2019
- 10:00-11:30
- HB 17.150
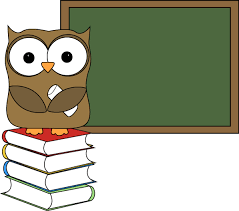
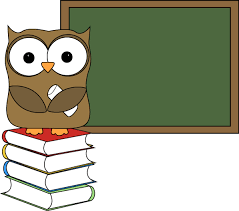
Signal Processing Seminar
- Thursday, 25 April 2019
- 13:30-14:30
- HB 17.150
sensor networks, rank-constrained optimization, algebraic techniques
Matthew Morency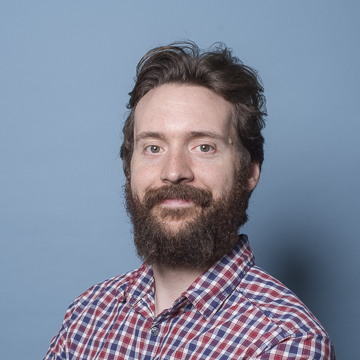
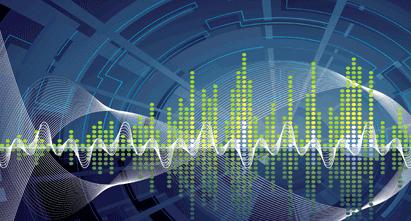
MSc ME Thesis Presentation
- Wednesday, 17 April 2019
- 16:00-16:45
- HB 17.150
A Highly Concurrent, Memory-Efficient AER Architecture for Neuro-Synaptic Spike Routing
Joris Coenen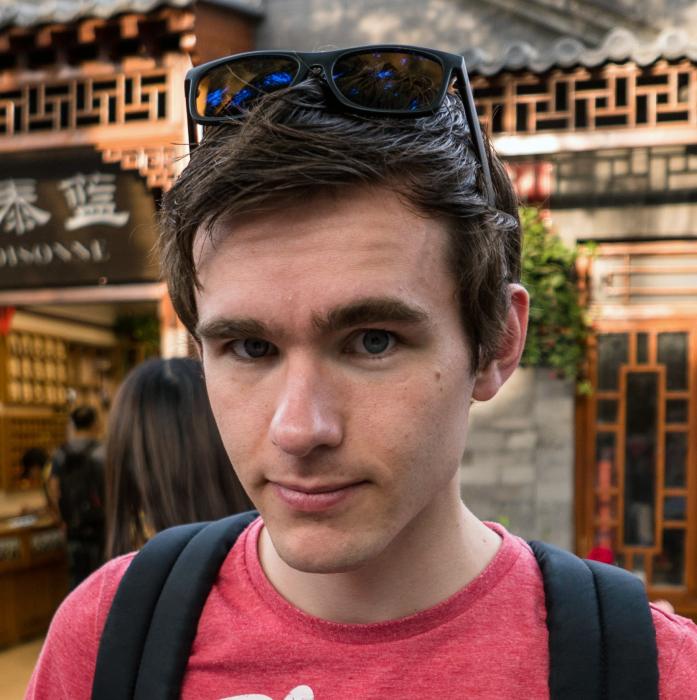
One of the challenges of neuromorphic computing is efficiently routing spikes from neurons to their connected synapses. The aim of this thesis is to design a spike-routing architecture for flexible connections on single-chip neuromorphic systems. A model for estimating area, power consumption, memory, spike latency and link utilisation for neuromorphic spike-routing architecture is described This model leads to the proposal for a new spike-routing architecture with a hybrid addressing scheme and a novel synaptic encoding scheme.
The proposed architecture is implemented in a SystemC simulation tool with a supporting tool for encoding arbitrary SNN topologies for the synapse encoding scheme.
Running the simulations with synthetic benchmarks and a handwriting recognition SNN shows that the proposed architecture is memory-efficient and provides low latency spike-routing with high synaptic activation concurrency.
Additional information ...
CAS MSc Midterm Presentations
- Tuesday, 16 April 2019
- 10:00-11:30
- HB 17.150
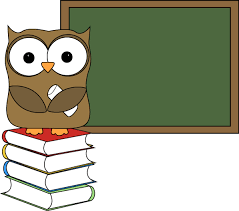
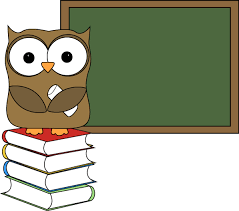
- Wednesday, 10 April 2019
- 14:00-17:30
- EWI Lecture hall Boole
NEXT-GENERATION MOLECULAR IMAGING AND PARTICLE THERAPY SEMINAR
Symposium on advances in Positron Emission Tomography, in context of the PhD defense of Esteban Venialgo
Additional information ...
CAS MSc Midterm Presentations
- Tuesday, 2 April 2019
- 10:00-11:30
- HB 17.150
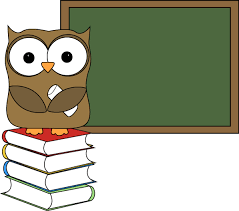
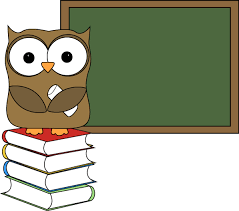
MSc CE Thesis Presentation
- Friday, 29 March 2019
- 14:30-15:15
- HB 17.150
Area Minimization of DTB Multiplexer - A Chip Component with High Wire Density and Congestion
Reynaldi Canggaputra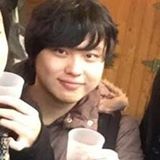
DTB Multiplexer is a component within an NXP chip called the BAP3. This component provides a testing functionality for the chip. This component is purely combinational, and requires no clock, however this makes the component wiring-costly. This high wiring requirement leads to the area constraint imposed by the wiring demand rather than cell area, and this also leads to the DTB multiplexer reducing the placement area available for other modules.
In this thesis, the wiring area is going to be estimated as the amount of congestion, which would cause detour in the design which results in extra wiring. In this thesis, DTB multiplexer is placed by external method instead of using the place and route tools usually used by the design team. Instead, the placement is done on MATLAB which is later ported to the place and route tools using script. The placement algorithm implemented in MATLAB is primarily based on two algorithm, Dplace for initial preplacement, which in turn utilizes diffusion preplacement algorithm, and modified C-ECOP for the congestion reduction. More detailed congestion estimation done by using an additional routing estimation algorithm which is based on One-Steiner routing algorithm.
The result indicates that the modified C-ECOP can be used to reduce congestion, thus wiring area when paired with a good initial placement algorithm, but the initial placement algorithm and detailed congestion estimation algorithm with one-steiner could be further improved, and further work is needed to integrate the result with commercial place and route tools.
Additional information ...
Micro electronics colloquium
- Thursday, 28 March 2019
- 15:30-17:00
- EEMCS, Restaurantzaal
High performance data converters; Rethink analog IC design
Nan Sun, Muhammed Bolatkale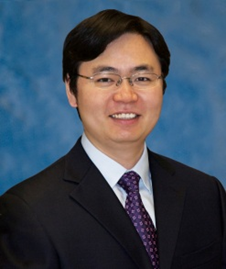
Nan Sun: Rethink Analog IC Design
I will present several unconventional data conversion architectures. First, I will talk about how we can make use of noise, which is usually deemed as an undesirable thing, to estimate the conversion residue and increase the SNR of a SAR ADC. It is an interesting example of stochastic resonance, in which the presence of noise can lead to not SNR degradation but SNR enhancement. Second, I will talk about how we can perform data conversion below the Nyquist rate by exploiting the sparsity of the input signal. I will show two example compressive sensing ADCs and how the effective ADC conversion rate can be reduced by 4 times but without losing information. Third, I will show how we can prevent the seemingly inevitable kT/C noise in a Nyquist-rate pipelined ADC by using a continuous-time SAR based 1st-stage. This can substantially reduce the requirement on the ADC input capacitance, greatly reducing the ADC driver power and reference buffer power
Biography of Nan Sun
Nan Sun is Associate Professor at the University of Texas at Austin. He received the B.S. from Tsinghua in 2006 and Ph.D. degree from Harvard in 2010. Dr. Sun received the NSF Career Award in 2013. He serves on the Technical Program Committee of the IEEE Custom Integrated Circuits Conference and the IEEE Asian Solid-State Circuit Conference. He is an Associate Editor of the IEEE Transactions on Circuits and Systems – I: Regular Papers, and a Guest Editor of the IEEE Journal of Solid-State Circuits. He also serves as IEEE Circuits-and-Systems Society Distinguished Lecturer from 2019 to 2020.
Muhammed Bolatkale: High Performance Data Converters
A next generation automotive radio receiver, an all-digital Class-D amplifier, and an advanced Bluetooth transceiver have one thing in common: they rely on high-performance data converter architectures to enable best in class performance. This talk will give an overview of GHz-sampling data converters, especially focusing on wideband delta-sigma and hybrid data converter architectures. We will touch upon state-of-the-art systems and circuit level designs fabricated in advance CMOS nodes.Bio Muhammed Bolatkale
Muhammed Bolatkale is Senior Principle Scientist at NXP Semiconductors and part-time Associate Professor in the Electronics Instrumentation Laboratory at Delft University of Technology. He received his B. Sc. (high honors) degree from Middle East Technical University, Turkey, in 2004 and the M. Sc. (cum laude) and Ph.D. degrees in Electrical Engineering from Delft University of Technology, the Netherlands, in 2007 and 2013. Since 2007, Dr. Bolatkale has worked for NXP Semiconductors, specializing in wideband Delta-Sigma ADCs for wireless communications and automotive applications. Dr. Bolatkale received the ISSCC 2016 and 2011 Jan Van Vessem Award for Outstanding European Paper and the IEEE Journal of Solid-State Circuits 2016 and 2011 Best Paper Award.Additional information ...
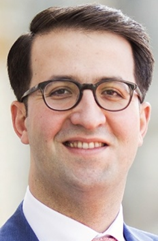
MSc SS Thesis Presentation
- Tuesday, 26 March 2019
- 15:00-15:45
- HB 17.150
Localization using Time-of-Arrival Estimation in the LoRa Network
Ming DAI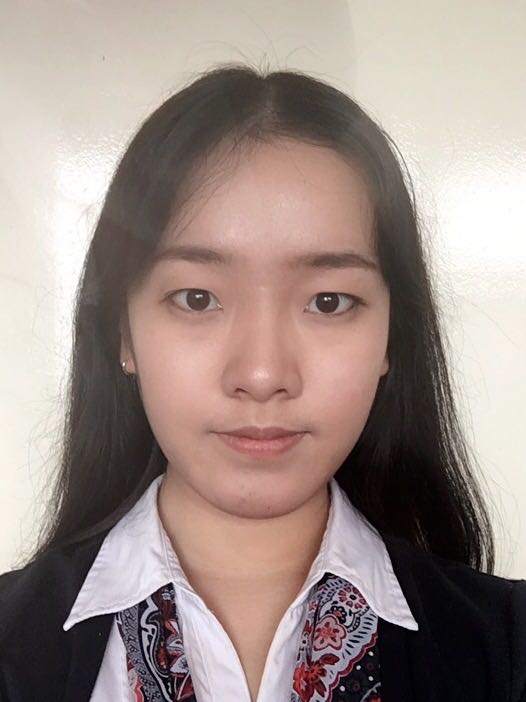
LoRa (Long Range) is a low-power, long-range and low-cost wireless communication system that can facilitate a wide variety of infrastructures for the Internet of Things (IoT). Current algorithms to locate LoRa tags have a resolution of ca 100 m in practice, and a question is if that can be improved without changing the tags or adding too much to the gateways (basestations).
Conventional delay estimation ranging algorithms extract useful information from the channel frequency response and use this information to estimate delays. In this thesis, three localization techniques are presented: the matched filter, FBCM-MUSIC and TLS-ESPRIT algorithms. Then a multiband architecture is proposed and integrated into the matched filter. These algorithms are implemented in the LoRa system model. The simulations indicate that FBCM-MUSIC and TLS-ESPRIT have better performance than the matched filter in NLOS channels. The results also show that TLS-ESPRIT is more effective and robust compared to MUSIC. The proposed multiband architecture can improve the resolution of TOA estimation and decreases the 90th percentile error by around 40%
MS3 seminar
- Friday, 22 March 2019
- 09:30-10:30
- EWI Snijderzaal (LB01.010)
Radar Adaptivity: Antenna Based Signal Processing
Alfonso FarinaIEEE SPS Distinguished Industry Speaker
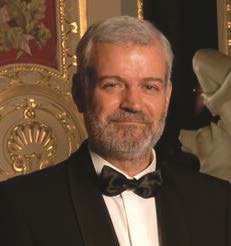
This lecture will provide an in-depth look into the history of radar systems and radar signal processing, from the beginning of radar to adaptive antenna arrays, including examples such as ground based radar systems, land and naval phased arrays, STAP for airborne radars, knowledge-based STAP, and Over the Horizon radar systems.
Biography
Alfonso FARINA , LFIEEE, FIET, FREng, Fellow of EURASIP, received the doctor degree in Electronic Engineering from the University of Rome (IT) in 1973. In 1974, he joined Selenia, then Selex ES, where he became Director of the Analysis of Integrated Systems Unit and subsequently Director of Engineering of the Large Business Systems Division. In 2012, he was Senior VP and Chief Technology Officer of the company, reporting directly to the President. From 2013 to 2014, he was senior advisor to the CTO. He retired in October 2014.From 1979 to 1985, he was also professor of “Radar Techniques” at the University of Naples (IT). He is the author of more than 800 peer-reviewed technical publications and of books and monographs (published worldwide), some of them also translated in to Russian and Chinese.
CAS MSc Midterm Presentations
- Friday, 22 March 2019
- 10:45-11:15
- HB 17.150
Improving processing of epicardial sensor array data for robust detection of anomalies
Bart Kölling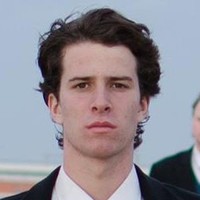
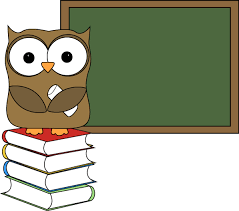
Signal Processing Seminar
- Thursday, 21 March 2019
- 13:30-14:30
- HB 17.150
Network Data Science
Huijuan Wang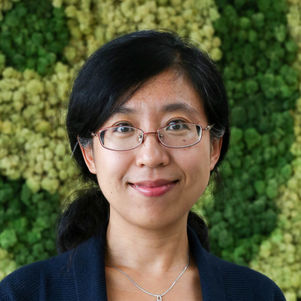
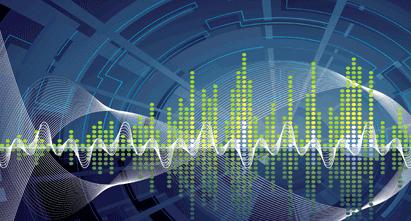
CAS MSc Midterm Presentations
- Tuesday, 19 March 2019
- 10:00-11:30
- HB 17.150
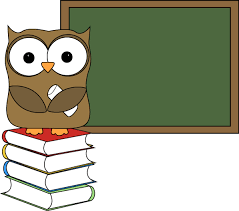
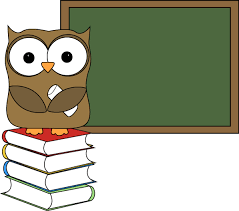
Microelectronics Colloquium
- Friday, 8 March 2019
- 16:00-17:15
- CiTG 0.98 (lecture room opposite PSOR)
Introducing new CAS professors
Andrew Webb, Borbála Hunyadi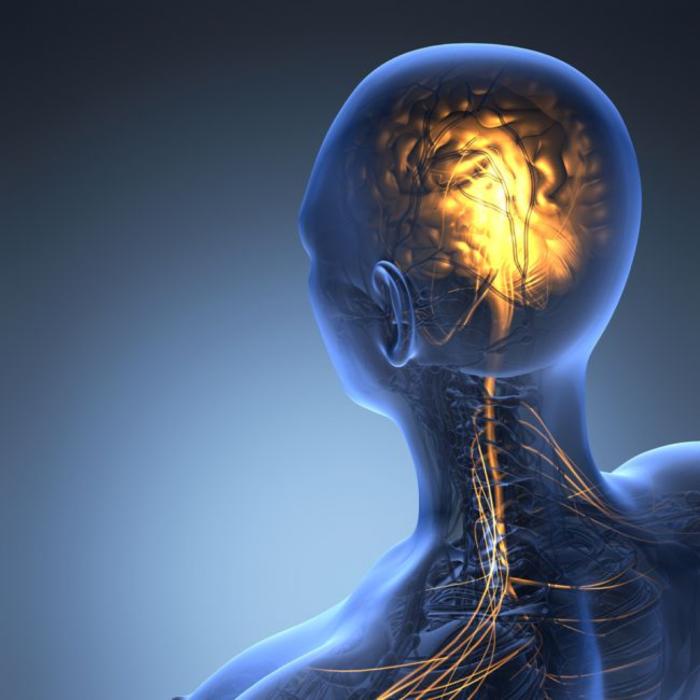
Andrew Webb:
MRI is one of the most important clinical imaging modalities for diagnosis and treatment monitoring. Recent trends have been towards ever higher magnetic fields and operating frequencies. This talk outlines some of the technical challenges faced by very high field and conversely very low field MRI, and the roles that electromagnetics and signal processing can play in improving image qualityBorbala Hunyadi
Electroencephalography (EEG) and functional magnetic resonance imaging (fMRI) record a mixture of ongoing neural processes, physiological and non-physiological noise. The pattern of interest is often hidden within this noisy mixture. This talk gives an overview of signal processing and machine learning techniques to address this issue by capturing the spatiotemporal structure in the (multimodal) data. Special attention is given to tensor-based blind source separation techniques, with applications in epilepsy research.
Signal Processing Seminar
- Thursday, 7 March 2019
- 13:30-14:30
- HB 17.150
Sensor and Machine Learning at The Arizona State University
Andreas SpaniasArizona State University - SenSIP
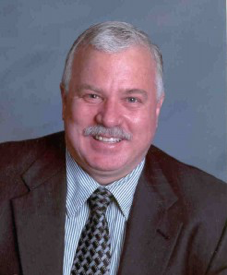
This seminar provides a description of the ASU Sensor Signal and Information Processing (SenSIP) center and its application-driven research projects. The center research activities include algorithm development for extracting information from sensors and IoT systems. More specifically center activities are focused on developing signal processing and machine learning methods for various applications including AI-enabled sensing for automotive, IoT solar energy system monitoring, surveillance systems, health monitoring, and sound systems. The center has several industry members that define and monitor research projects typically for Ph.D. student work. SenSIP has also affiliated faculty working on sensor circuits, flexible sensors, radar, smart cameras, motion estimation, secure sensor networks and other systems.
Biography
Andreas Spanias is Professor in the School of Electrical, Computer, and Energy Engineering at Arizona State University (ASU). He is also the director of the Sensor Signal and Information Processing (SenSIP) center and the founder of the SenSIP industry consortium (now an NSF I/UCRC site). Member companies of the NSF SenSIP center and industry consortium on sensor information processing include: Intel, National Instruments, LG, NXP, Raytheon, Sprint and several SBIR type companies. He is an IEEE Fellow and he recently received the IEEE Phoenix Section Award for Patents and Innovation. He also received the IEEE Region 6 section award (across 12 states) for education and research in signal processing. He is author of more than 300 papers,15 patents, two text books and several lecture monographs. He served as Distinguished lecturer for the IEEE Signal processing society in 2004.Additional information ...
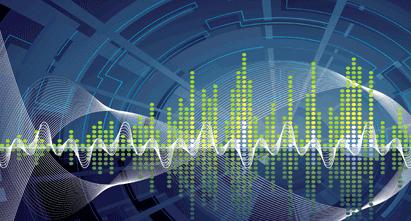
Radio-frequency engineering for space
- Tuesday, 5 March 2019
- 13:45-15:45
- lecture hall Boole, EWI building
European Space Agency
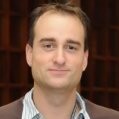
The key challenges in the design of radio-frequency instruments for space will be reviewed. Space environmental aspects will be discussed as well as the practical measures that need to be implemented to assure a high level of reliability. Selected examples will be presented, covering a wide spectrum of applications: from new satellite communication trends, such as active reconfigurable antennas to future scientific RF instruments that will be placed on other planets. Special focus will be put on high-power amplification concepts and integration solutions.
Speaker Bio: Václav Valenta was born in Czechoslovakia and received Master and Doctoral degrees in radio engineering and mathematics from the Brno University of Technology in the Czech Republic and Université Paris-Est in France, respectively. In the past, Dr. Valenta has designed and demonstrated active and passive radar systems operating up to a frequency of 140 GHz. His expertise is in the area of multi-functional RFIC design (SiGe BiCMOS and III-V) covering key functions from amplification, frequency generation/conversion, modulation/demodulation, and heterogenous RFIC integration. Dr. Valenta is currently with the European Space Agency, RF Equipment and Technology Section, running and supporting several R&D projects. Dr. Valenta is responsible for the development of the radio-science instrument "LaRa", which is a scientific payload that will be launched to Mars in the frame of the mission ExoMars 2020.
CAS MSc Midterm Presentations
- Tuesday, 5 March 2019
- 10:00-11:30
- HB 17.150
Problem statement presentations by new MSc students
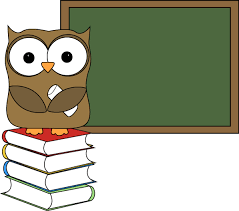
Signal Processing Seminar
- Thursday, 28 February 2019
- 13:30-14:30
- HB 21.120
From matrices to tensors: the power of tensor methods in signal processing
Borbála Hunyadi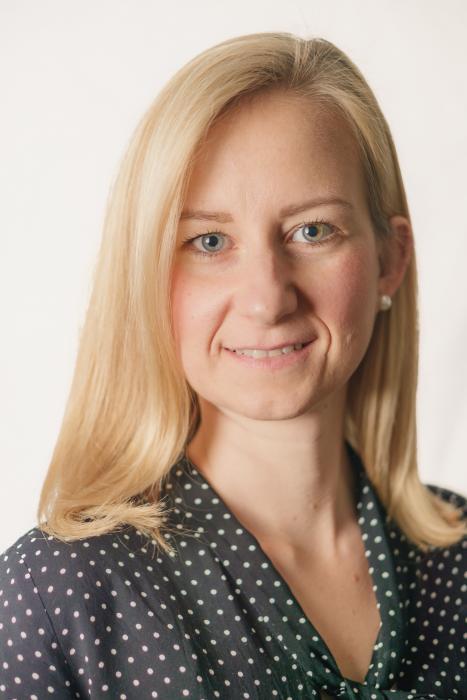
In this presentation, I give an overview of the fundamental differences between linear and multilinear algebra. After generalising the basic concepts of matrix rank and matrix SVD to tensors, the richness of tensor representations and decompositions become clear. The richness of tensor algebra, in turn, provides powerful models for signal processing.
Additional information ...
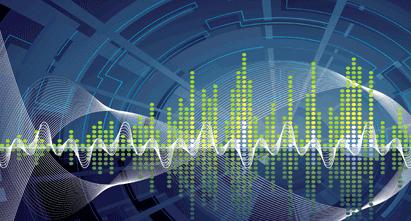
CAS MSc Midterm Presentations
- Tuesday, 19 February 2019
- 10:00-11:30
- HB 17.150
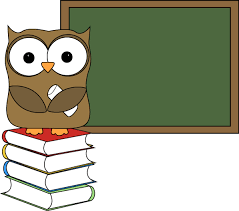
Signal Processing Seminar
- Thursday, 14 February 2019
- 13:30-14:30
- HB 17.150
Signal processing for communication, sensor networks
Jac Romme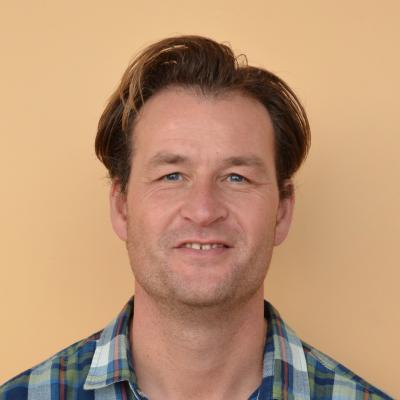
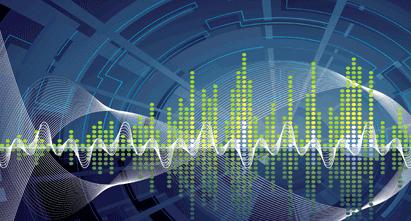
Signal Processing Seminar
- Thursday, 31 January 2019
- 13:30-14:30
- HB 17.150
Wireless communication and networking, Digital Design
Tarik Kazaz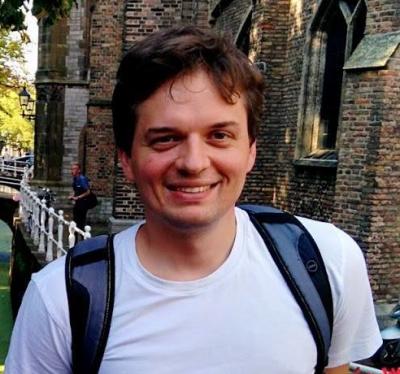
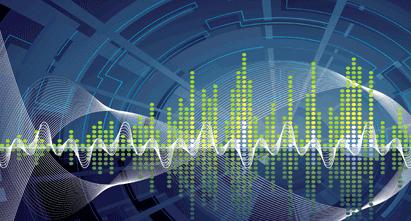
PhD Thesis Defence
- Monday, 28 January 2019
- 12:00-14:30
- Aula Senaatszaal
Graph-Time Signal Processing Filtering and Sampling Strategies
Elvin Isufi
The necessity to process signals living in non-Euclidean domains, such as signals defined on the top of a graph, has led to the extension of signal processing techniques to the graph setting. Among different approaches, graph signal processing distinguishes itself by providing a Fourier analysis of these signals. Analogously to the Fourier transform for time and image signals, the graph Fourier transform decomposes the graph signals decomposes in terms of the harmonics provided by the underlying topology. For instance, a graph signal characterized by a slow variation between adjacent nodes has a low frequency content.
Along with the graph Fourier transform, graph filters are the key tool to alter the graph frequency content of a graph signal. This thesis focuses on graph filters that are performed distributively in the node domain–that is, each node needs to exchange information only within its neighbor to perform a given filtering operation. Similarly to the classical filters, we propose ways to design and implement distributed finite impulse response and infinite impulse response graph filters.
One of the key contributions of this thesis is to bring the temporal dimension to graph signal processing and build upon a graph-time signal processing framework. This is done in different ways. First, we analyze the effects that the temporal variations on the graph signal and graph topology have on the filtering output. Second, we introduce the notion of joint graph-time filtering. Third, we presentpr a statistical analysis of the distributed graph filtering when the graph signal and the graph topology change randomly in time. Finally, we extend the sampling framework from the reconstruction of graph signals to the observation and tracking of time-varying graph processes.
We characterize the behavior of the distributed autoregressivemoving average (ARMA) graph filters when the graph signal and the graph topology are time-varying. The latter analysis is exploited in two ways: i ) to quantify the limitations of graph filters in a dynamic environment, such as a moving sensors processing a time-varying signal in a sensor network; and i i ) to provide ways for filtering with low computation and communication complexity time-varying graph signals.
We develop the notion of distributed graph-time filtering, which is an operation that jointly processes the graph frequencies of a time-varying graph signal on one hand and its temporal frequencies on the other hand. We propose distributed finite impulse response and infinite impulse response recursions to implement a two-dimensional graphtime filtering operation. Finally, we propose design strategies to find the filter coefficients that approximate a desired two-dimensional frequency response.
We extend the analysis of graph filters to a stochastic environment, i.e., when the graph topology and the graph signal change randomly over time. By characterizing the first and second order moments of the filter output, we quantify the impact of the graph signal and the graph topology randomness into the distributed filtering operation. The latter allows us to develop the notion of graph filtering in the mean, which is also used to ease the computational burden of classical graph filters.
Finally, we propose a sampling framework for time-varying graph signals. Particularly, when the graph signal changes over time following a state-space model, we extend the graph signal sampling theory to the tasks of observing and tracking the time-varying graph signal froma few relevant nodes. The latter theory considers the graph signal sampling as a particular case and shows that tools from sparse sensing and sensor selection can be used for sampling.
Additional information ...
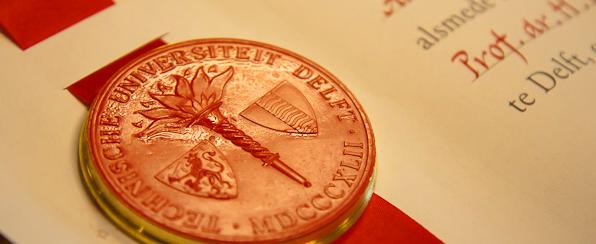
Signal Processing Seminar
- Monday, 28 January 2019
- 10:00-11:00
- EWI Snijderzaal (LB01.010)
Graph Neural Networks and Graph Scattering Transforms
Alejandro RibeiroUniversity of Pennsylvania
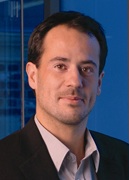
Convolutional Neural Networks (CNN) are layered information processing architectures in which each of the layers is itself the composition of a convolution operation with a pointwise nonlinearity. Graph Neural Networks (GNNs) replace the regular convolution operation with a graph convolution operation. We will discuss graph convolutions, their use in building GNN architectures, and explore stability properties of GNN operators. The stability results establish that a GNN is stable to graph deformations that are close to permutations. This result provides a theoretical basis to characterize classes of machine learning problems in which we expect GNNs to work well.
Biography
Alejandro Ribeiro received the B.Sc. degree in electrical engineering from the Universidad de la Republica Oriental del Uruguay, Montevideo, in 1998 and the M.Sc. and Ph.D. degree in electrical engineering from the Department of Electrical and Computer Engineering, the University of Minnesota, Minneapolis in 2005 and 2007. From 1998 to 2003, he was a member of the technical staff at Bellsouth Montevideo. After his M.Sc. and Ph.D studies, in 2008 he joined the University of Pennsylvania (Penn), Philadelphia, where he is currently Professor of Electrical and Systems Engineering. His research interests are in the applications of statistical signal processing to collaborative intelligent systems. His specific interests are in wireless autonomous networks, machine learning on network data and distributed collaborative learning. Papers coauthored by Dr. Ribeiro received the 2014 O. Hugo Schuck best paper award, and paper awards at CDC 2017, SSP Workshop 2016, SAM Workshop 2016, Asilomar SSC Conference 2015, ACC 2013, ICASSP 2006, and ICASSP 2005. His teaching has been recognized with the 2017 Lindback award for distinguished teaching and the 2012 S. Reid Warren, Jr. Award presented by Penn’s undergraduate student body for outstanding teaching. Dr. Ribeiro is a Fulbright scholar class of 2003 and a Penn Fellow class of 2015.Additional information ...
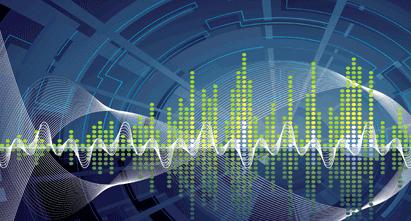
PhD Thesis Defence
- Thursday, 24 January 2019
- 14:30-16:30
- Aula Senaatszaal
Efficient computational methods in Magnetic Resonance Imaging
Jeroen van Gemert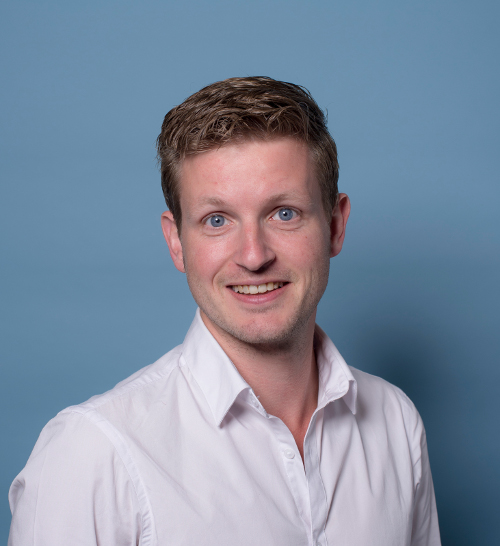
This dissertation describes how to design dielectric pads that can be used to increase image quality in Magnetic Resonance Imaging, and how to accelerate image reconstruction times using a preconditioner.
Image quality is limited by the signal to noise ratio of a scan. This ratio is increased for higher static magnetic field strengths and therefore there is great interest in high-field MRI. The wavelength of the transmitted magnetic RF field decreases for higher field strengths, and it becomes comparable to the dimensions of the human body. Consequently, RF interference patterns are encountered which can severely degrade image quality because of a low transmit efficiency or because of inhomogeneities in the field distribution. Dielectric pads can be used to improve this distribution as the pads tailor the field by inducing a secondary magnetic field due to its high permittivity. Typically, the pads are placed tangential to the body and in the vicinity of the region of interest. The exact location, dimensions, and constitution of the pad need to be carefully determined, however, and depend on the application and the MR configuration. Normally, parametric design studies are carried out using electromagnetic field solvers to find a suitable pad, but this is a very time consuming process which can last hours to days. In contrast with these design studies, we present methods to efficiently model and design the dielectric pads using reduced order modeling and optimization techniques. Subsequently, we have created a design tool to bridge the gap between the advanced design methods and the practical application by the MR community. Now, pads can be designed for any 7T neuroimaging and 3T body imaging application within minutes.
In the second part of the thesis a preconditioner is designed for parallel imaging (PI) and compressed sensing (CS) reconstructions. MRI acquisition times can be strongly reduced by using PI and CS techniques by acquiring less data than prescribed by the Nyquist criterion to fully reconstruct the anatomic image; this is beneficial for patient's comfort and for minimizing the risk of patient's movement. Although acquisition times are reduced, the reconstruction times are increased significantly. The reconstruction times can be reduced when a preconditioner is used. In this thesis, we construct such a preconditioner for the frequently used iterative Split Bregman framework. We have tested the performance in a conjugate gradient framework, and show that for different coil configurations, undersampling patterns, and anatomies, a five-fold acceleration can be obtained for solving the linear system part of Split Bregman.
Additional information ...
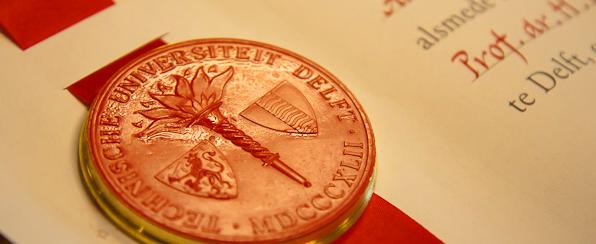
MSc SS Thesis Presentation
- Thursday, 24 January 2019
- 09:30-10:30
- Lipkenszaal (HB 01.150)
Improving Ultrafast Doppler Imaging using Subspace Tracking
Bastian Generowicz
Ultrafast Doppler imaging provides a new way to image blood motion at thousands of frames per second. It has gained popularity due to its high spatio-temporal resolution, which is required to distinguish blood motion from clutter signals caused by slow moving tissue. By conducting functional UltraSound (fUS) experiments on the brain using this method, we are able to better understand the underlying processes during brain activity through neurovascular coupling. fUS relies on optimized signal processing techniques to acquire and process high frame-rate images in real-time.
For my thesis I have set up the backbone to allow for fUS experiments as well as created the analysis framework required to analyse and interpret the incoming data. Furthermore, I have developed a more computationally efficient method of obtaining vascular images, based on the Projection Approximation Subspace Tracking (PAST) method. The PAST algorithm is able to display accurate representations of the blood subspace, while maintaining a lower computational complexity than the state-of-the-art method, making it suitable for Doppler imaging. When applied to functional ultrasound, the exponentially weighted PASTd method achieves similar Pearson Correlation coefficients compared to the current state-of-the-art method, over multiple functional experiments. These findings highlight the potential of applying PAST to Ultrafast Doppler imaging.
Additional information ...
MSc SS Thesis Presentation
- Wednesday, 23 January 2019
- 10:00-10:40
- Katwijkzaal (HB 03.230)
An Investigation of the Medical Ultrasound Image Sparse Spaces used for Model-Based Imaging
Zheheng Liu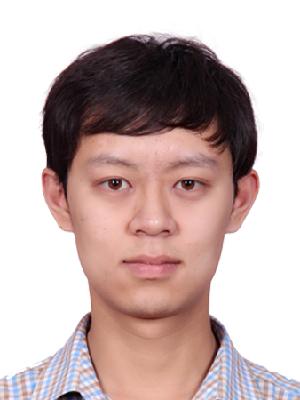
In this thesis, we investigate a sparse basis for ultrasound images, so that we can use sparse regularization in imaging. Actually, there are few previous researches explicitly demonstrating that medical ultrasound images can be sparsified for some dictionary. We consider various orthogonal transforms such as wavelet transforms, cosine transforms and wave atom transforms. Then, we perform those transforms on various ultrasound images and analyzes their sparsity. These ultrasound images include the images of two computer ultrasound phantoms and beamformed ultrasound images with good quality from real people. We looked at sparsity of the true pre-beamformed images, as well as beamformed images. We also consider constructing a specific ultrasound image dictionary using the K-SVD algorithm. We observed that, the pre-beamformed images hardly have no sparse basis, and the sparsity of beamformed images will only increase slightly if we use different 1D-DWT in each direction. We also found that the wide overdetermined dictionary generated by K-SVD significantly increases sparsity. After this, we simulate the ultrasound image reconstruction from the ultrasound RF measurements, and we analyze the effects of the different sparse spaces on the reconstruction performance. We observed that, the l1-regularization can work for ultrasound imaging better than l2-regularization, but the orthogonal transforms as well as the dictionary do not improve the reconstruction image quality much.
Signal Processing Seminar
- Thursday, 17 January 2019
- 13:30-14:30
- HB 17.150
audio signal processing
Wangyang Yu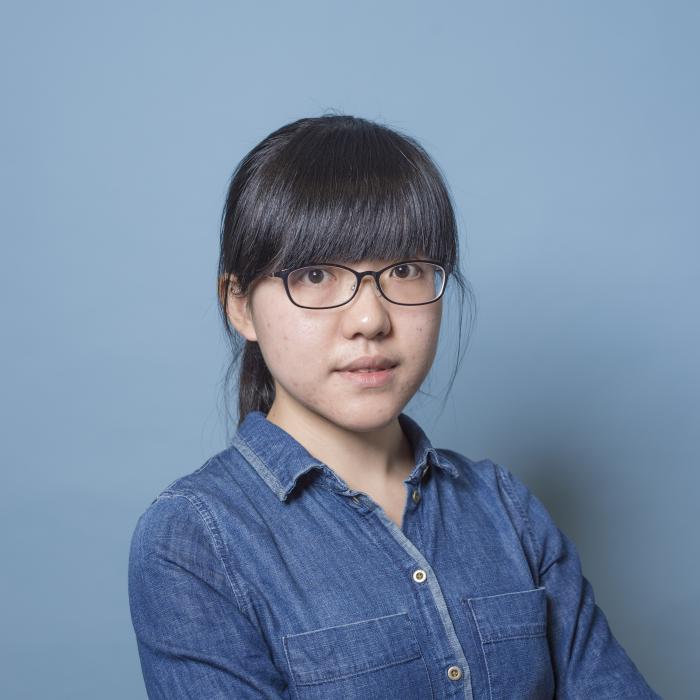
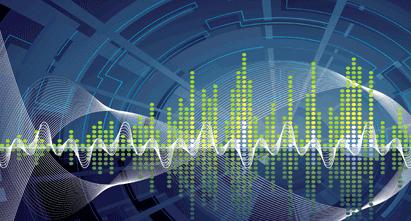
Signal Processing Seminar
- Thursday, 10 January 2019
- 13:30-14:30
- HB 17.150
Introductory presentation
Seyedmahdi Izadkhast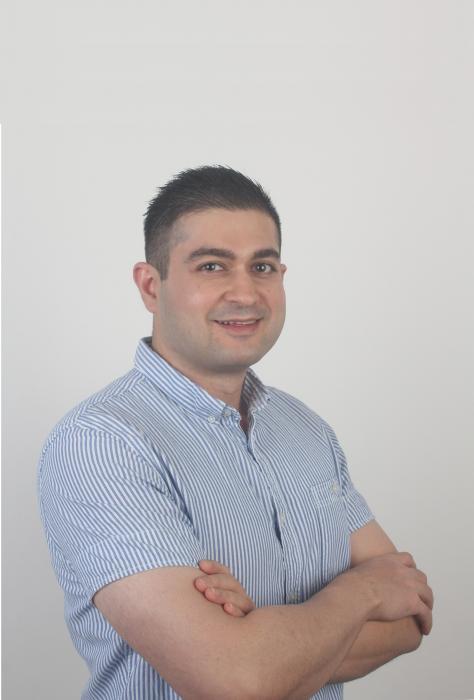
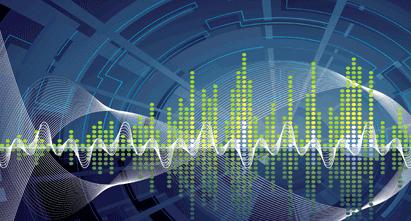
PhD Thesis Defence
- Friday, 21 December 2018
- 12:00-14:30
- Aula Senaatszaal
Multi-Microphone Noise Reduction for Hearing Assistive Devices
Andreas Koutrouvelis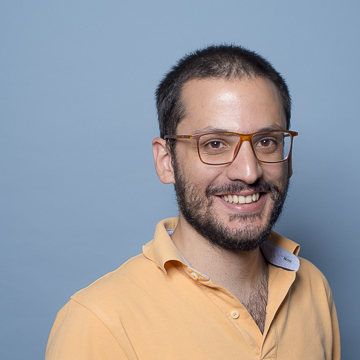
The paramount importance of good hearing in everyday life has driven an exploration into the improvement of hearing capabilities of (hearing impaired) people in acoustic challenging situations using hearing assistive devices (HADs). HADs are small portable devices, which primarily aim at improving the intelligibility of an acoustic source that has drawn the attention of the HAD user. One of the most important steps to achieve this is via filtering the sound recorded using the HAD microphones, such that ideally all unwanted acoustic sources in the acoustic scene are suppressed, while the target source is maintained undistorted. Modern HAD systems often consist of two collaborative (typically wirelessly connected) HADs, each placed on a different ear. These HAD systems are commonly referred to as binaural HAD systems. The noise reduction filters designed for binaural HAD systems are referred to as binaural beamformers.
Binaural beamformers typically change the magnitude and phase relations of the microphone signals by forming a beam towards the target's direction while ideally suppressing all other directions. This may alter the spatial impression of the acoustic scene, as the filtered sources now reach both ears with possibly different relative phase and magnitude differences compared to before processing. This will appear unnatural to the HAD user. Therefore, there is an increasing interest in the preservation of the spatial information (also referred to as binaural cues) of the acoustic scene after processing. The present dissertation is mainly concerned with this particular problem and proposes several alternative binaural beamformers which try to exploit the available degrees of freedom to achieve optimal performance in both noise reduction and binaural-cue preservation.
Additional information ...
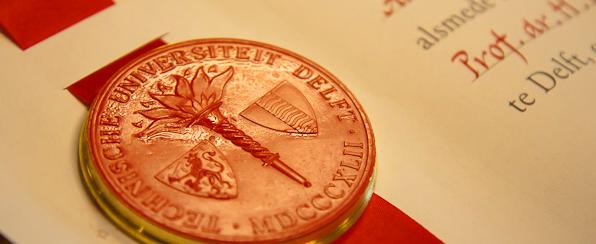
Signal Processing Seminar
- Thursday, 13 December 2018
- 13:30-14:30
- HB 17.150
audio signal processing, localization
Jie Zhang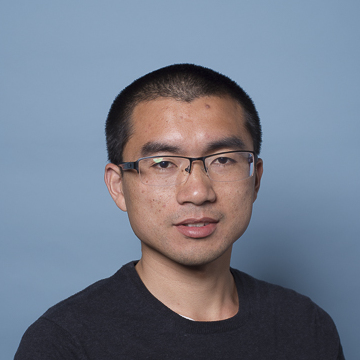
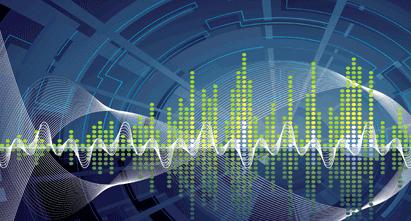
PhD Thesis Defence
- Friday, 7 December 2018
- 12:30-13:30
- Aula Senaatszaal
Image formation for future radio telescopes
Shahrzad Naghibzadeh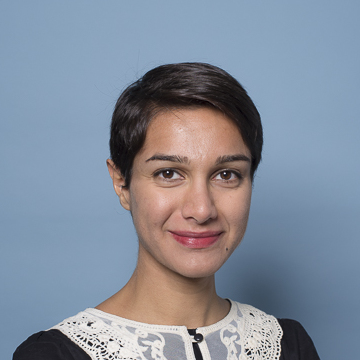
Fundamental scientific questions such as how the first stars were formed or how the universe came into existence and evolved to its present state drive us to observe weak radio signals impinging on the earth from the early days of the universe. During the last century, radio astronomy has been vastly advancing. Important discoveries on the formation of various celestial objects such as pulsars, neutron stars, black holes, radio galaxies and quasars are the result of radio astronomical observations. To study celestial objects and the astrophysical processes that are responsible for their radio emissions, images must be formed. This is done with the help of large radio telescope arrays.
Next generation radio telescopes such as the Low Frequency Array Radio Telescope (LOFAR) and the Square Kilometer Array (SKA), bring about increasingly more observational evidence for the study of the radio sky by generating very high resolution and high fidelity images. In this dissertation, we study radio astronomical imaging as the problem of estimating the sky spatial intensity distribution over the field of view of the radio telescope array from the incomplete and noisy array data. The increased sensitivity, resolution and sky coverage of the new instruments pose additional challenges to the current radio astronomical imaging pipeline. Namely, the large amount of data captured by the radio telescopes cannot be stored and needs to be processed quasi-realtime.
Many pixel-based imaging algorithms, such as the widely-used CLEAN [3] algorithm, are not scalable to the size of the required images and perform very slow in high resolution scenarios. Therefore, there is an urgent need for new efficient imaging algorithms. Moreover, regardless of the amount of collected data, there is an inherent loss of information in themeasurement process due to physical limitations. Therefore, to recover physically meaningful images additional information in the form of constraints and regularizing assumptions are necessary. The central objective of the current dissertation is to introduce advanced algebraic techniques together with custom-made regularization schemes to speed up the image formation pipeline of the next generation radio telescopes.
Signal processing provides powerful tools to address these issues. In the current work, following a signal processing model of the radio astronomical observation process, we first analyze the imaging system based on tools from numerical linear algebra, sampling, interpolation and filtering theory to investigate the inherent loss of information in the measurement process. Based on these results, we show that the imaging problem in radio astronomy is highly ill-posed and regularization is necessary to find a stable and physically meaningful image. We continue by deriving an adequate model for the imagingproblem in radio interferometry in the context of statistical estimation theory. Moreover, we introduce a framework to incorporate regularization assumptions into the measurement model by borrowing the concept of preconditioning from numerical linear algebra.
Radio emissions observed by radio telescopes appear either as distributed radiation from diffuse media or as compact emission from isolated point-like sources. Based on this observation, different source models need to be applied in the imaging problem formulation to obtain the best reconstruction performance. Due to the ill-posedness of the imaging problem in radio astronomy, to guarantee a reliable image reconstruction, propermodeling of the source emissions and regularizing assumptions are of utmost importance. We integrate these assumptions implementing a multi-basis dictionary based on the proposed preconditioning formalism.
In traditional radio astronomical imaging methods, the constraints and priormodels, such as positivity and sparsity, are employed for the complete image. However, large radio sky images usually manifest individual source occupancy regions in a large empty background. Based on this observation, we propose to split the field of view into multiple regions of source occupancy. Leveraging a stochastic primal dual algorithm we apply adequate regularization on each facet. We demonstrate the merits of applying facet-based regularization in terms of memory savings and computation time by realistic simulations.
The formulation of the radio astronomical imaging problem has a direct consequence on the radio sky estimation performance. We define the astronomical imaging problem in a Bayesian-inspired regularized maximum likelihood formulation. Based on this formalism, we develop a general algorithmic framework that can handle diffuse as well as compact source models. Leveraging the linearity of radio astronomical imaging problem, we propose to directly embody the regularization operator into the system by right preconditioning. We employ an iterative method based on projections onto Krylov subspaces to solve the subsequent system. The proposed algorithmis named PRIor-conditioned Fast Iterative Radio Astronomy (PRIFIRA). We motivate the use of a beamformed image as an efficient regularizing prior-conditioner for diffuse emission recovery. Different sparsity-based regularization priors are incorporated in the algorithmic framework by generalizing the core algorithm with iterative re-weighting schemes.
We evaluate the performance of PRIFIRA based on simulated measurements as well as astronomical data and compare to the state-of-the-art imaging methods. We conclude that the proposed method maintains competitive reconstruction quality with the current techniques while remaining flexible in terms of different regularization schemes. Moreover, we show that the imaging efficiency can be greatly improved by exploiting Krylov subspace methods together with an appropriate noise-based stopping criteria.
Based on the results from this thesis we can conclude that with the help of advanced techniques from signal processing and numerical linear algebra, customized algorithms can be designed to tackle some of the challenges in the next generation radio telescope imaging. We note that since radio interferometric imaging can be considered as an instance of the broad area of inverse imaging problems, the numerical techniques as well as regularization methods developed in this dissertation have a direct impact on many different imaging application areas, such as biomedical and geophysics/seismic imaging.
Additional information ...
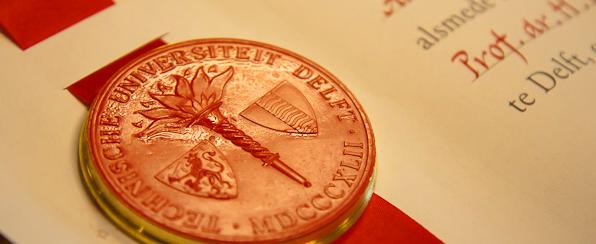
Signal Processing Seminar
- Friday, 7 December 2018
- 10:00-10:45
- LB 1.220 van der Poelzaal
Image Reconstruction Using Training Images
Per Christian HansenTechnical University of Denmark
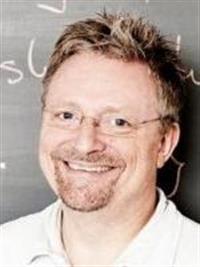
Priors are essential for computing stable solutions to ill-posed problems, and they take many different forms. Here we consider priors in the form of cross-section images of the object, and this information must be used in a fast, reliable, and computationally efficient manner. We describe an algorithmic framework for this: From a set of training images we use techniques from machine learning to form a dictionary that captures the desired features, and we then compute a reconstruction with a sparse representation in this dictionary. We describe how to stably compute the dictionary through a regularized non-negative matrix factorization, and we study how this dictionary affects the reconstruction quality. Simulations show that for textural images our approach is superior to other methods used for limited-data problems.
About the speaker
Professor Per Christian Hansen has worked with numerical regularization algorithms for 30 years, and he has published 4 books and 100+ papers in leading journals. He has developed a number of software packages, of which Regularization Tools (now in its 4th version) is a popular toolbox for analysis and solution of discrete inverse problems. His current research projects involve algorithms for tomographic reconstruction and iterative image deblurring algorithms. He is a SIAM fellow in recognition of his work on inverse problems and regularization.Additional information ...
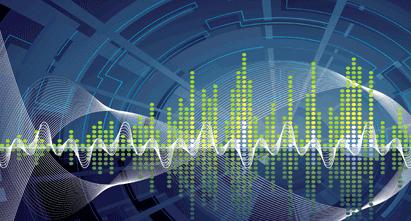
Signal Processing Seminar
- Thursday, 6 December 2018
- 13:30-14:30
- HB 17.150
Audio processing
Andreas Koutrouvelis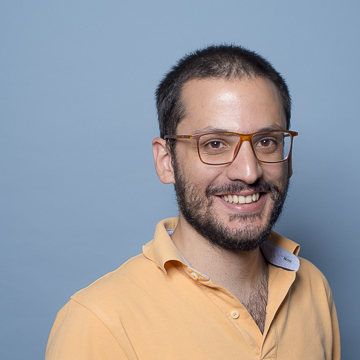
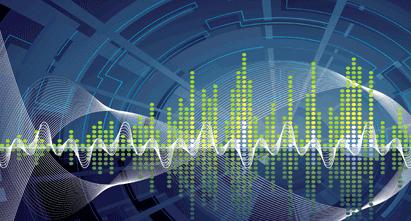
Signal Processing Seminar
- Thursday, 29 November 2018
- 13:30-14:30
- HB 17.150
Array signal processing, Sensor networks, Optimization, Numerical Lineal Algebra
Mario Coutiño Minguez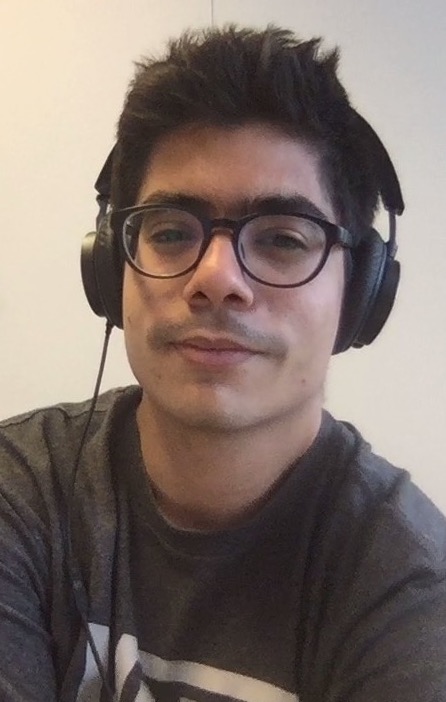
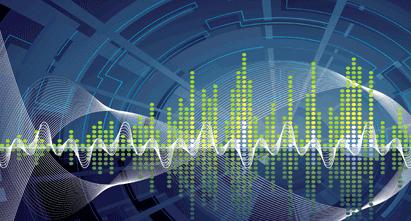
Signal Processing Seminar
- Thursday, 22 November 2018
- 13:30-14:30
- HB 17.150
Distributed Convex Optimization: A Monotone Perspective
Thomas Sherson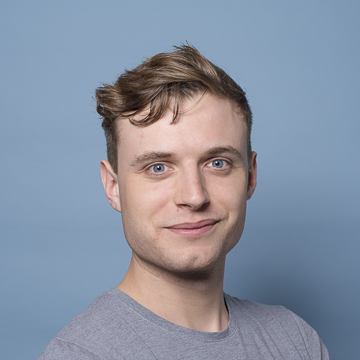
Over the last few decades, methods of parallel and distributed computation have become essential tools in a wide range of applications such as machine learning, wireless sensor network processing and big data signal processing. Motivated by this point and the synergy between signal processing and convex optimization, in this work we demonstrate recent results in the area of distributed optimisation to facilitate such computation. In particular we highlight the primal dual method of multipliers (PDMM), a relatively recent algorithm proposed for distributed optimization. We demonstrate how PDMM can be derived from classic monotone operator theory which in turn provides insight into previously unknown convergence results for the algorithm. Using this insight we generalise PDMM to solve the class of separable problems with separable constraints and analyze how, in the case of strongly convex and smooth functions, the convergence rate of PDMM is influenced by the underlying network topology.
Additional information ...
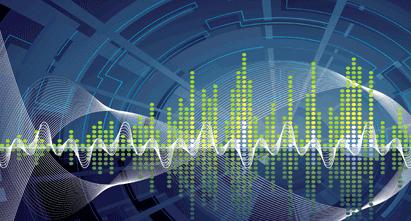
MSc SS Thesis Presentation
- Thursday, 22 November 2018
- 13:00-13:45
- Lipkenszaal (LB 01.150)
Respiration monitoring based on information fusion from Impedance pneumography and Electrocardiography
Yuyang Wang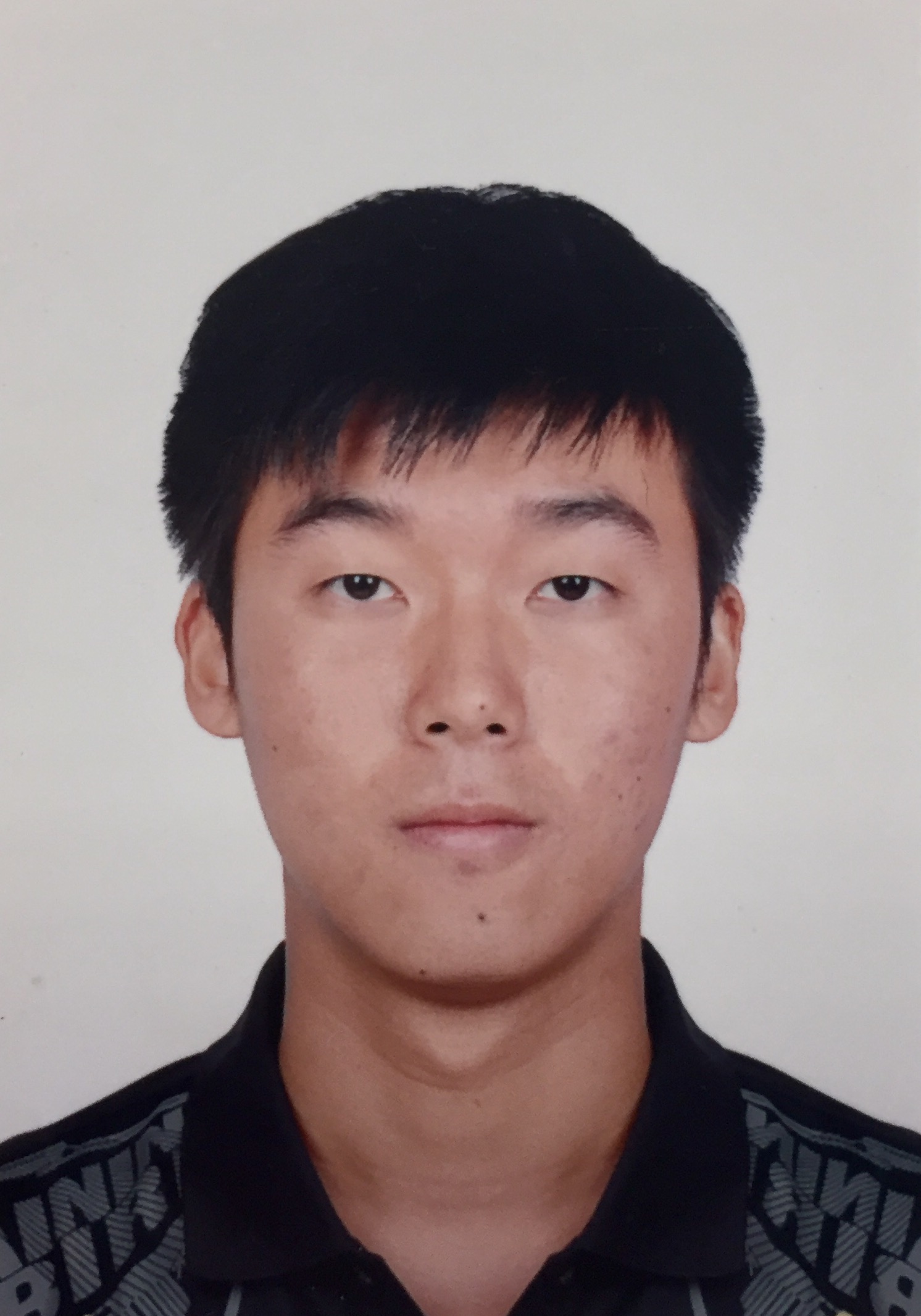
Wearable health has become a striking area in our daily life. Electrocardiogram (ECG) is one of the biomedical signals collected by the wearable or portable devices, which is widely used in heart rate monitoring and cardiac diagnosis. However, automatic ECG signal analysis is dicult in real application because the signals are easy to be contaminated by the noise and artifacts. Thus, the quality of ECG signals is essential for the accurate analysis.
The objective of this project is to design a reliable automated ECG signal quality indicator based on the supervised learning algorithm, which intends to estimate the quality of the signals and distinguish them. The methodology of this project is creating a classication model to indicate the quality of ECG signals based on the machine learning algorithm. The model is trained by the extracted features based on the Fourier transform, Wavelet transform, Autocorrelation function and Principal component analysis of ECG signals. Subsequently, the feature selection techniques are proposed to remove the irrelevant and redundant features and then the selected features are fed to classi- cation algorithms. The classier was then trained and tested on the expert-labeled data from the collected ECG signals. Particularly, we focus on the performance of classier and use the best training model to predict the quality of new ECG signals.
MSc BME Thesis Presentation
- Friday, 16 November 2018
- 14:30-15:15
- 3ME room J
The effect of dopamine release on electrical neural activity in the prefrontal cortex
Jack Tchimino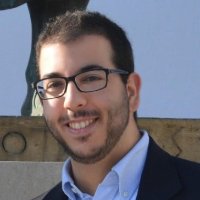
How can certain oscillations be detected from the measured brain signals?
Additional information ...
- Friday, 9 November 2018
- 11:00-12:00
- HB 09.150 (Dijkstrazaal)
Graph signal processing: Analyzing and filtering data over networks
Elvin Isufi
Nowadays, we are surrounded by massive data that reside on irregular structures. Examples include data generated by biological, financial, and sensor networks. Graphs indeed offer the ability to model the interactions between them. Current efforts in signal processing are being focused on providing analysis and processing tools for these data such that the underlying structure is taken into account. These include the development of a Fourier transform and filtering operations for graph data. The field that gathers these tools is called graph signal processing (GSP).
This talk will be focused on the fundamentals of GSP spanning concepts like the signal variation over a graph, the graph Fourier transform, the graph filter, and its distributed implementation. The talk will be concluded with some illustrative examples and future work directions.
Additional information ...
Signal Processing Seminar
- Thursday, 25 October 2018
- 13:30-14:30
- HB 17.150
Tensor-based blind source separation in epileptic EEG and fMRI
Borbála Hunyadi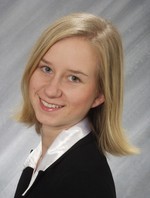
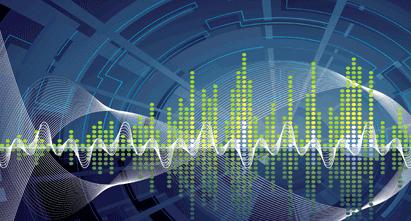
- Wednesday, 24 October 2018
- 10:00-10:30
- HB 17.150
Joint optimization of milk intake and sleep time for babies under one month old
Elena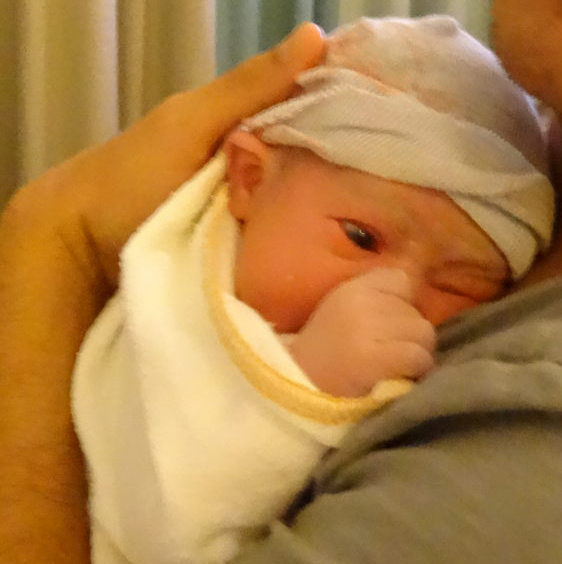
(daughter of Jorge)
Signal Processing Seminar
- Thursday, 18 October 2018
- 13:00-14:15
- HB 17.150
Biomedical signal processing/wavefield imaging
Patrick Fuchs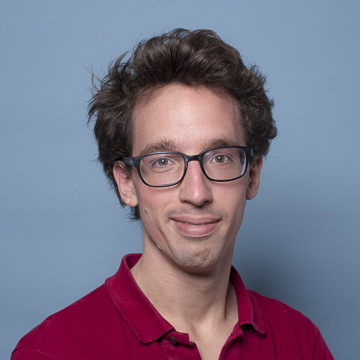
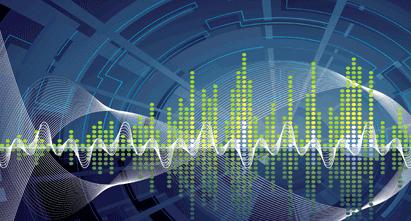
Signal Processing Seminar
- Tuesday, 16 October 2018
- 13:30-16:30
- EWI LB 1.010 Snijderszaal
Signal Processing Mini-Symposium
Hagit Messer, KVS Hari, Andrea Simonetto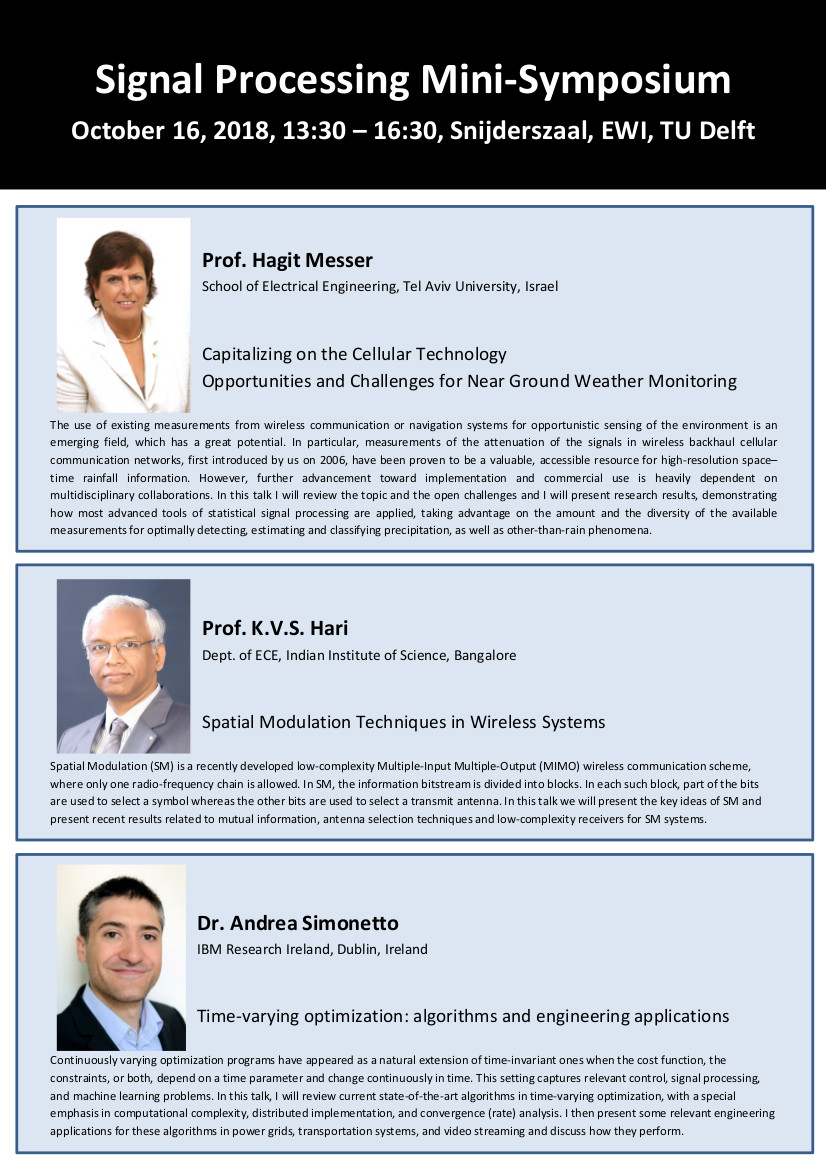
Talk 1: Capitalizing on the Cellular Technology Opportunities and Challenges for Near Ground Weather Monitoring
Prof. Hagit MesserSchool of Electrical Engineering, Tel Aviv University, Israel
Talk 2: Spatial Modulation Techniques in Wireless Systems
Prof. K.V.S. HariDept. of ECE, Indian Institute of Science, Bangalore
Talk 3: Time-varying optimization: algorithms and engineering applications
Dr. Andrea SimonettoIBM Research Ireland, Dublin, IrelandAdditional information ...
PhD Thesis Defence
- Monday, 15 October 2018
- 12:30-13:30
- Aula Senaatszaal
Spatio-temporal environment monitoring leveraging sparsity
Venkat Roy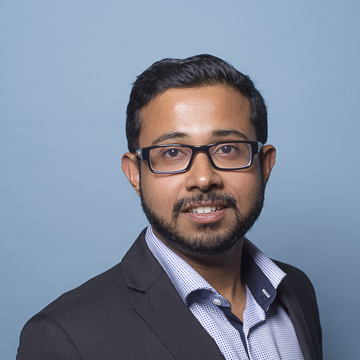
Linking sensor measurements to unknown field intensities, with application to rainfall monitoring from cellular networks
Additional information ...
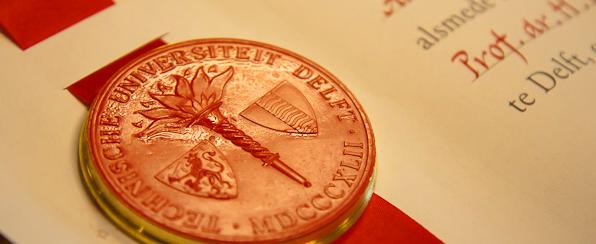
Signal Processing Seminar
- Thursday, 11 October 2018
- 13:30-14:30
- HB 17.150
manufacturing defect detection
Aydin Rajabzadeh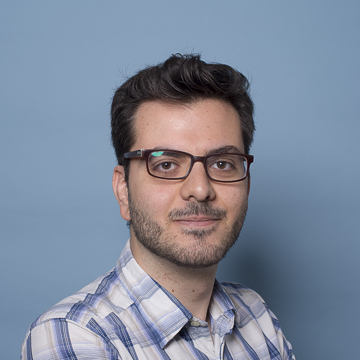
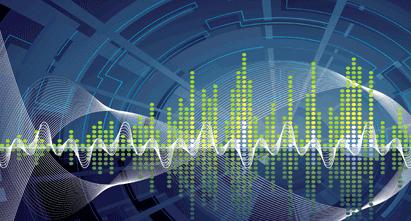
Signal Processing Seminar
- Thursday, 4 October 2018
- 13:30-14:30
- HB 17.150
Machine learning in physical sciences
Peter GerstoftUC San Diego
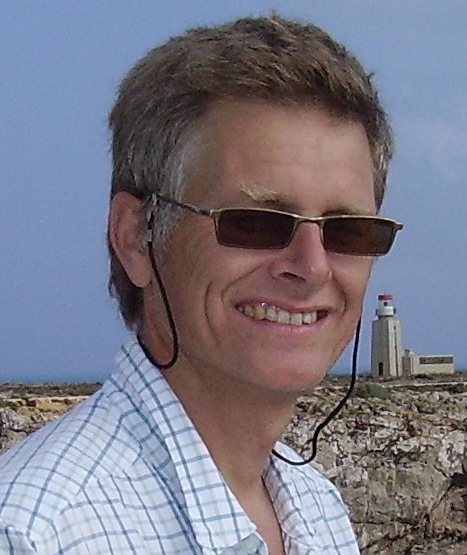
Machine learning (ML) is booming thanks to efforts promoted by Google. However, ML also has use in physical sciences. I start with a general overview of ML for supervised/unsupervised learning. Then I will focus on my applications of ML in array processing in seismology and ocean acoustics. This will include source localization using neural networks or graph processing. Final example is using ML-based tomography to obtain high-resolution subsurface geophysical structure in Long Beach, CA, from seismic noise recorded on a 5200-element array. This method exploits the dense sampling obtained by ambient noise processing on large arrays by learning a dictionary of local, or small-scale, geophysical features directly from the data.
Additional information ...
MSc ME Thesis Presentation
- Friday, 28 September 2018
- 13:00-13:30
- EWI HB 17.150
Design Space Exploration of a Neuromorphic ECG Classification System using a Spiking Self-Organizing Map
Johan Mes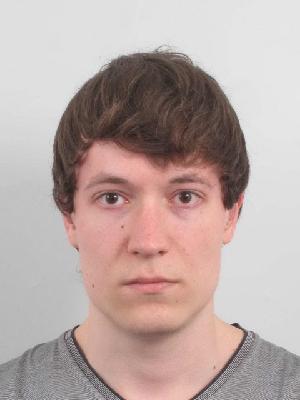
The Self-Organizing Map (SOM) is an unsupervised neural network topology that incorporates competitive learning for the classification of data. In this thesis we investigate the design space of a system incorporating such a topology based on Spiking Neural Networks (SNNs), and apply it to classifying Electrocardiogram (ECG) beats. We present novel insights into the characterization of the SOM and its encapsulating system by exploring configuration parameters such as learning rate, neuron models, potentiation and depression ratios, and synaptic conductivity parameters by performing high-level architectural simulations of the system whose SNN is developed with the aim of being implemented using power efficient neuromorphic hardware.
Due to the amount of manual work needed to monitor and analyze ECG signals when diagnosing cardiovascular problems, and because it is the leading cause of death in the world, an automated, realtime, and low power detection & classification system is essential. Unsupervised and in realtime, this system performs beat detection with an average TPR of 99.10% and a PPV of 99.58% and classification of 500 detected beats with an EMDS of 0.0169 and a beat recognition percentage of 100%.
Signal Processing Seminar
- Thursday, 27 September 2018
- 13:30-14:30
- HB 17.150
Signal processing algorithms for acoustic vector sensors
Krishnaprasad Nambur Ramamohan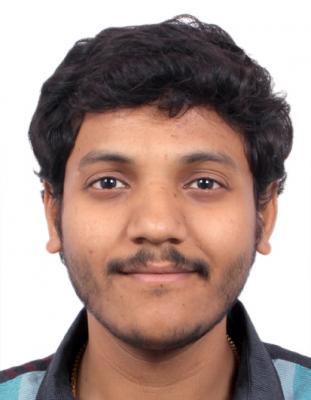
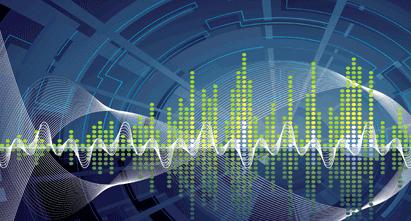
Symposium MRI for Low-Resource Setting
- Thursday, 27 September 2018
- 13:30-15:00
- Science Center, Mekelzaal 2, Mijnbouwstraat 120, 2628 RX Delft
Sustainable Low-Field MRI Technology for Point of Care Diagnostics in Low-Income Countries
Steven Schiff, Johnes ObungolochPenn State University (USA) and Mbarara University (Uganda)
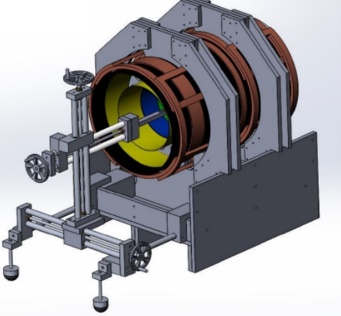
MRIs are expensive and require sophisticated facilities to use them, which is why many people worldwide do not have access to this diagnostic service. Luckily an interdisciplinary team of scientists and medical professionals from the Netherlands (TU Delft and LUMC), the US (Penn State) and Uganda (MUST) is working on creating an affordable and simple MRI scanner. Want to know more? Come to the symposium!
In the talk, Steven Schiff of Penn State University (USA) and Johnes Obungoloch of Mbarara University (Uganda) will explain how their Ultra-low field MRI technology is a response to the need for appropriate medical technologies in countries like those in Sub-Saharan Africa. Together with TU Delft and LUMC they intend to use simple non-cooled magnets that can do the job by combining them with algorithms and a reference set of advanced MRI images. The ultimate goal is to create an MRI scanner that is inexpensive and that can be assembled, operated and maintained in developing countries. Their initial focus is hydrocephalus – the most common condition in children worldwide that requires brain imaging and neurosurgical treatment.
Additional information ...
MSc SS Thesis Presentation
- Thursday, 27 September 2018
- 15:00-15:30
- EWI HB 17.150
Phase estimation of recurring patterns in nonstationary signals
Rik van der Vlist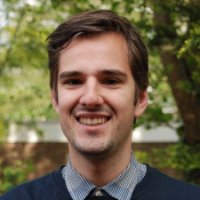
A phase estimation algorithm is presented to estimate the phase of a recurring pattern in a nonstationary signal. The signal is modelled by a template signal that represents one revolution of the recurring pattern, and that the frequency of this pattern can change at any time with no assumptions about local stationarity. The algorithm uses a constraint maximum likelihood estimator (MLE) to estimate the phase of the recurring pattern in the time series. Using the dynamic programming techniques from the dynamic time warping (DTW) algorithm, the solution is found in an efficient manner. The algorithm is applied to the digitization of meter readings from analog consumption meters.
As of today, analog consumption meters are still widely used to measure the consumption of gas, electricity and water. Often, smart home appliance use a simple reflective photosensor located on a rotating part of the meter to obtain information about the state of the consumption meter. The algorithm presented in this thesis accurately estimates the phase of the repeating pattern that occurs in the sensor observation when the meter rotates. Using this estimate, the signal of the photosensor can be converted to an estimate of the total resource consumption and consumption rate.
The algorithm improves in accuracy over conventional methods based on peak detection, and is shown to work in cases where the peak detection methods fails. Examples of this are signals where there is no distinctive peak in the signal or a signal where the recurring pattern is reversed. Furthermore, a template compression scheme is proposed that is used to decrease the computational complexity of the algorithm. Different time series compression methods are applied to the algorithm and evaluated on their performance.
Additional information ...
MSc SS Thesis Presentation
- Thursday, 27 September 2018
- 10:00-10:30
- HB 17.150
Snoring Sound Production and Modelling; Acoustic Tube Modelling
Mert Ergin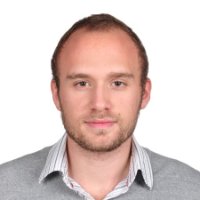
The thesis project is aimed at designing an unobtrusive method to determine from snoring sounds the obstruction location and severity for persons that have benign snoring and that are residing in their natural sleep environment.
Similar to speech generation, which is enabled by opening and closing of the vocal cords, the sound of snoring consists of a series of impulses caused by the rapid obstruction and reopening of parts of the upper airway. By exploiting this similarity, we try to explain snoring sound production using analysis and synthesis techniques that have been applied to speech. In particular, 1-D tube models and linear prediction have been studied and employed.
From a trial on anti-snoring device efficacy, audio recordings were available. These contained snoring sounds of multiple participants over multiple nights and were used for analysis. Similar to speech analysis, the Iterative Adaptive Inverse Filtering (IAIF) method has been used to find excitation flow and airway tract transfer functions from snoring sounds during the inhalation period. It has been found that the linear prediction part in IAIF is not suitable to directly determine the cross-sectional area of the upper airway from snoring sounds.
A model has been built using acoustic tube theory with the purpose of reflecting the physical realities of the upper airways. The tube model allows modeling flow obstructions at arbitrary positions in the upper airways. The transfer spectra from the acoustic tube having obstructions at various positions can be compared to the tube model resulting from IAIF using a gain-independent Itakura Spectral Distance Measure and the best match can be determined. This alternative approach was found to indicate obstruction positions which vary over persons. Further research is required to establish if the thus determined locations are adequately matching the true snoring cause and constitute a viable way for generating advice for anti-snoring devices and measures.
Additional information ...
Microelectronics Colloquium
- Tuesday, 25 September 2018
- 03:00-05:00
- EWI, Snijderszaal
Tenure track colloquium
Sten Vollebregt, Massimo Mastrangeli, Daniele CavalloWideband phased arrays for future wireless communication terminals, Daniele Cavallo (TS group)
Wireless data traffic will grow exponentially in the next years, due to the proliferation of user terminals and bandwidth-greedy applications. To address this demand, the next generations of mobile communication (5G and beyond) will have to shift the operation to higher frequencies, especially to millimetre-wave (mmWave) spectrum (30-300 GHz), that can provide extremely high-speed data links. To enable mm-wave wireless communication, mobile terminals such as smartphones will need phased arrays antennas, able to radiate or receive greater power in specific directions that can be dynamically steered electronically. However, to cover the different 5G mm-wave bands simultaneously (28, 39, 60 GHz, …) and to achieve total angular coverage, too many of such antennas should be on the same device: the main bottleneck is the insufficient space available to place all antenna modules. Therefore, I propose to investigate novel phased array antenna solutions with very large angular coverage and ultra-wide frequency bandwidth, to massively reduce the overall space occupation of handset antennas and overcome the current limitations of mobile terminal antenna development.
Towards smart organs-on-chip, Massimo Mastrangeli (ECTM Group)
Organs-on-chip are microfluidic systems that enable dynamic tissue co-cultures under physiologically realistic conditions. OOCs are helping innovating the drug screening process and gaining new fundamental insights in human physiology. In this talk, after a summary of my past research journey, I will describe how the ECTM group at TU Delft is envisioning the use microfabrication and materials science to embed real-time sensing and actuation in innovative and scalable OOC platforms.
Emerging electronic materials: from lab to fab, Sten Vollebregt (ECTM group)
Due to their nm-size features and often unique physical properties nanomaterials, like nanotubes and 2D materials, can potentially outperform classical materials or even provide functionality which cannot be obtained otherwise. Because of this, these nanomaterials hold many promises for the next generation of devices for sensing & communication and health & wellbeing.
Unfortunately, many promising applications of nanomaterials never reach sufficient maturity to be implemented in actual products. This is mostly because the interest in the academic community reduces once the initial properties have been demonstrated, while the risk for industrialization is still too high for most companies to start their own R&D activities. My goal is to bridge these two worlds by investigating the integration of novel nanomaterials in semiconductor technology and demonstrating the scalability of novel sensing devices. In this talk, I will give examples on how carbon nanotubes, graphene and other emerging nanomaterials can be used in the next generation of sensing devices.
Signal Processing Seminar
- Thursday, 20 September 2018
- 13:30-14:30
- HB 17.150
Tutorial on: Sum-of-squares Representation in Optimization and Applications in Signal Processing
Tuomas Aittomäki
Signal Processing Seminar
- Thursday, 13 September 2018
- 13:30-14:30
- HB 17.150
Low-cost sparse sensing designed for specific tasks
Pim van der Meulen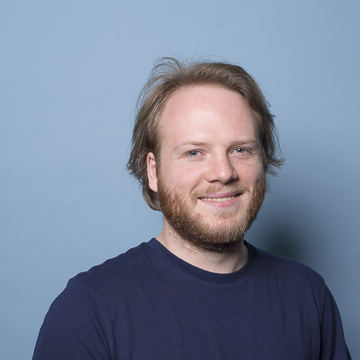
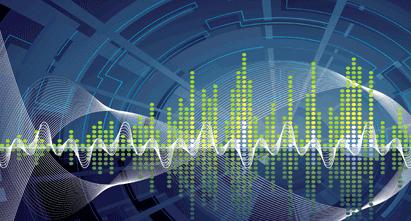
MSc TC Thesis Presentation
- Thursday, 13 September 2018
- 10:00-10:45
- HB 17.150
Bluetooth Direction Finding
Lichen Yao
This thesis project focuses on the algorithm developement and practical considerations for Indoor Direction Finding feature that will be incorporated in the next generation Bluetooth standard.
MSc SS Thesis Presentation
- Thursday, 30 August 2018
- 10:00-10:45
- HB 01.230
Vessel Layer Separation of X-ray Angiographic Images using Deep Learning Methods
Haidong Hao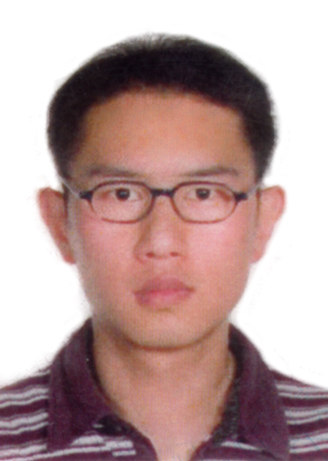
Percutaneous coronary intervention is a minimally-invasive procedure to treat coronary artery disease. In such procedures, X-ray angiography, a real-time imaging technique, is commonly used for image guidance to identify lesion sites and navigate catheters and guide-wires within coronary arteries. Due to the physical nature of X-ray imaging, photon energy undergoes absorption when penetrating tissues, rendering a 2D projection image of a 3D scene, in which semi-transparent structures overlap with each other. The overlapping structures make robust information processing of X-ray images challenging. To tackle this issue, layer separation techniques for X-ray images were proposed to separate those structures into different image layers based on structure appearance or motion information. These techniques have been proven effective for vessel enhancement in X-ray angiograms. However, layer separation approaches still suffer either from non-robust separation or long processing time, which prevent their application in clinics.
The purposes of this work are to investigate whether vessel layer separation from X-ray angiography images is possible via deep learning methods and further to what extent vessel layer separation can be achieved with deep learning methods.
To this end, several deep learning based methods were developed and evaluated to extract the vessel layer. In particular, all the proposed methods utilize a fully convolutional network (FCN) with two different architectures, which was trained by two different strategies: conventional losses and an adversarial loss.
The results of all the methods show good vessel layer separation on 42 clinical sequences. Compared to the previous state-of-the-art, the proposed methods have similar performance but runs much faster, which makes it a potential real-time clinical application. In addition, the proposed methods were assessed for low-contrast / low-dose scenarios with synthetic X-ray angiography data, and the results showed robust performance.
MSc SS Thesis Presentation
- Thursday, 30 August 2018
- 10:00-10:45
- HB 17.150
Automatic Interferer Selection for Binaural Beamforming
Costas Kokke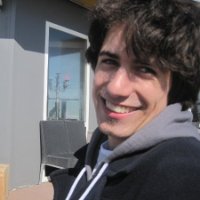
Spatial cues allow a listener to determine the direction sound is coming from. In addition, recognising spatially separated sound sources facilitate the listener to focus on specific sound sources. Because of this, preservation of spatial cues in multi-microphone hearing assistive devices is important to the listening experience and safety of the user. A number of linearly-constrained-minimum-variance-based methods exist for this purpose. Most of these are limited in the number of interfering sources for which they can preserve the spatial cues. In this thesis, a method of selecting the most important interfering point sources using convex optimisation is proposed.
The method is presented based on two different convex relaxations, which are compared, using simulation experiments, to existing, exhaustive search and randomised methods in terms of noise suppression and localisation errors.
Both methods are shown to improve the performance of the joint binaural linearly constrained minimum variance beamformer, an existing method for simultaneous noise reduction and spatial cue preservation, by giving it more degrees of freedom for noise reduction and allowing it to handle a larger number of (virtual) sources present in the scene.
Additional information ...
MSc SS Thesis Presentation
- Wednesday, 29 August 2018
- 13:00-13:45
- HB 17.150
Audio-visual authentication for mobile devices
Lucas Montesinos Garcia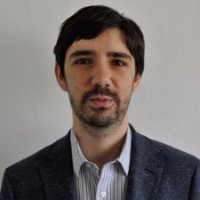
Authentication is becoming an increasingly important application in the connected world and is driven by the growing use of mobile and IoT devices that use an increasing number of applications that require transactions of sensitive data. Security usually relies on passwords and/or two-factor authentication which are too intrusive for daily use. Biometric solutions such as fingerprints, voice, iris and retina are a good alternative to overcome previous problems.
In this project an audio-visual identity verification is presented, where the use of multiple modes that can already be captured from most IoT devices (microphone and camera) make authentication robust to adverse conditions. End-factor analysis (i-vectors) with cosine distance is implemented as the main classification algorithm which takes into account variations within and between speakers. Mel Frequencies Cepstrum Coefficients (MFCC) are used as audio features, 2D-DCT coefficients of a single snapshot and Motion Vectors (MV) of the lips are extracted for visual features. Improvements combining different modes are shown using VidTimit dataset where the proposed algorithm achieves 0.7% of Half Total Error (HTER) in the test set outperforming single modes audio and visual by 9.5% and 6.4%, respectively.
MSc SS Thesis Presentation
- Wednesday, 29 August 2018
- 13:30-14:15
- LB01.010 Snijderszaal
Automatic Initialization for 3D Ultrasound CT Registration During Liver Tumor Ablations
Dirk Schut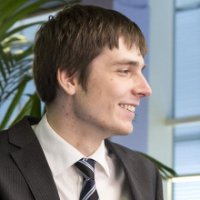
Ablation is a medical procedure to treat liver cancer where a needle-like catheter has to be inserted into a tumor, which will then be heated or frozen to destroy the tumor tissue. To guide the catheter, Ultrasound(US) imaging is used which shows the catheter position in real time. However, some tumors are not visible on US images. To make these tumors visible, image fusion can be used between the inter-operative US image and a pre-operative contrast enhanced CT(CECT) scan, on which the tumors are visible. Several methods exist for tracking the motions of the US transducer relative to the CECT scan, but they all require a manual initialization or external tracking hardware to align the coordinate systems of both scans. In this thesis we present a technique for finding an initialization using only the image data. To achieve this, deep learning is used to segment liver vessels and the boundary of the liver in 3D US images. To find the rigid transformation parameters, the SaDE evolutionary algorithm was used to optimize the alignment between the blood vessels and the liver boundary between both scans.
Additional information ...
MSc TC Thesis Presentation
- Friday, 24 August 2018
- 14:00-14:45
- HB 17.150
Phase Domain Ranging for Narrowband ISM Radio Bands
Aulia Recky Soepeno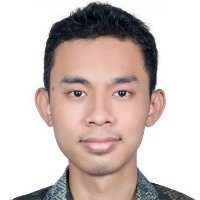
In this thesis, we study ranging algorithms in an indoor environment using narrow-band industrial, scientific, and medical (ISM) radio bands at 2.4 GHz. Previously, a phase difference approach has been implemented for this problem. However, the distance estimation is rather inaccurate for indoor ranging, mainly due to multipath and noise. This thesis studies several direction of arrival (DOA) techniques such as matched filter (MF), minimum variance distortionless response (MVDR), and multiple signal classification (MUSIC) to reduce the impact of indoor multipath. Forward-backward smoothing as well as the Akaike information criterion (AIC) and the minimum descriptive length (MDL) are also proposed to diminish the multipath effect further and estimate the number of separable multipath in the channel. Besides, a MUSIC-like method is discussed to prevent incorrect estimation of the number of sources. We test the proposed algorithm under different channel parameter values, compensate the bias, and show the related performance improvement as the absolute bias value is reduced up an order of magnitude.
MSc SS Thesis Presentation
- Thursday, 23 August 2018
- 14:00-15:00
- LB 01.170 Timmanzaal
Multidomain Graph Signal Processing: Learning and Sampling
Guillermo Ortiz Jiménez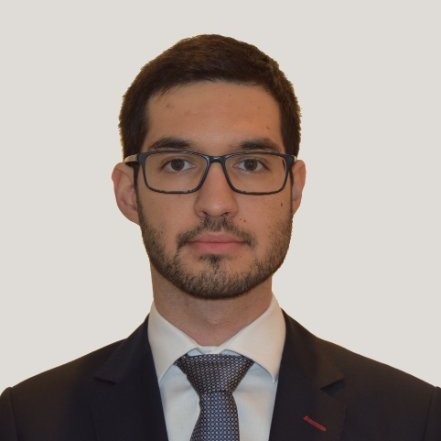
In this era of data deluge, we are overwhelmed with massive volumes of extremely complex datasets. Data generated today is complex because it lacks a clear geometric structure, comes in great volumes, and it often contains information from multiple domains. In this thesis, we address these issues and propose two theoretical frameworks to handle such multidomain datasets. To begin with, we extend the recently developed geometric deep learning framework to multidomain graph signals, e.g., time-varying signals, defining a new type of convolutional layer that will allow us to deal with graph signals defined on top of several domains, e.g., electroencephalograms or traffic networks. After discussing its properties and motivating its use, we show how this operation can be efficiently implemented to run on a GPU and demonstrate its generalization abilities on a synthetic dataset. Next, we consider the problem of designing sparse sampling strategies for multidomain signals, which can be represented using tensors. To keep the framework general, we do not restrict ourselves to multidomain signals defined on irregular domains. Nonetheless, this particularization to multidomain graph signals is also presented. To do so, we leverage the multidomain structure of tensor signals and propose to acquire samples using a Kronecker-structured sensing function, thereby circumventing the curse of dimensionality. For designing such sensing functions, we develop several low-complexity greedy algorithms based on submodular optimization methods that compute near-optimal sampling sets. To validate the developed theory, we present several numerical examples, ranging from multi-antenna communications to graph signal processing.
Additional information ...
MSc SS Thesis Presentation
- Monday, 16 July 2018
- 14:00-15:00
- HB 17.150
Impedance-based bioassay for characterization of single malignant melanoma cancer cells usinG CMOS-MEA systems
Makrina Sekeri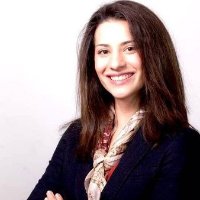
Malignant Melanoma (MM) is the most aggressive type of skin-cancer. Current diagnostic tools for the detection of malignancies of the skin (MM cancer) include histological, optical, ultrasound, and impedance-based techniques. The inadequacies of the first three practices are overwhelmed by the Electrical Impedance Spectroscopy (EIS) method. EIS overcomes reported spatiotemporal tradeoffs as a label-free and optics-free analytical method. Yet, MM’s enhanced heterogeneity and metastatic potential still results in misdiagnosis, or late diagnosis leading to stages characterized by high mortality rates. Important biological information and processing ability on single-cell level is missing. Single-cell dynamics recorded with a high-throughput system, contain important biological information on the heterogeneous subpopulations which are responsible for the MM aggressiveness.
This project aims to investigate experimentally the possibility and capabilities of such a bioassay development, create working protocols and generate a fundamental basis for analysis and interpretation of the big-data-sets which derive from Impedance monitoring from a high-throughput transducer.
Experiments were performed, employing two diverse, human-derived, MM cancer cell-lines, and using a high-throughput HD-MEA system with a 1024-channel impedance readout unit developed at IMEC, in Belgium. The measurements were realized at 1kHz aiming to extract Rseal information. The main proposal presents an experimental protocol of mid-term and long-term experiments Temporal and spatial resolutions were enhanced (Control System Automation), allowing for implementation of an experimental set to test the assay’s capabilities and determine any necessary additions to make the assay more robust for research (i.e. Z-Map, templates and scripts for OriginLab and Matlab, statistical methods for validation of findings on the big-data sets, optimizations in the experimental process, etc).
Additional information ...
Symposium
- Tuesday, 3 July 2018
- 13:00-19:00
- Room "Kubus" in buidling 26 (Van den Burghweg, south of Civil Engineering)
Waves, model reduction and imaging
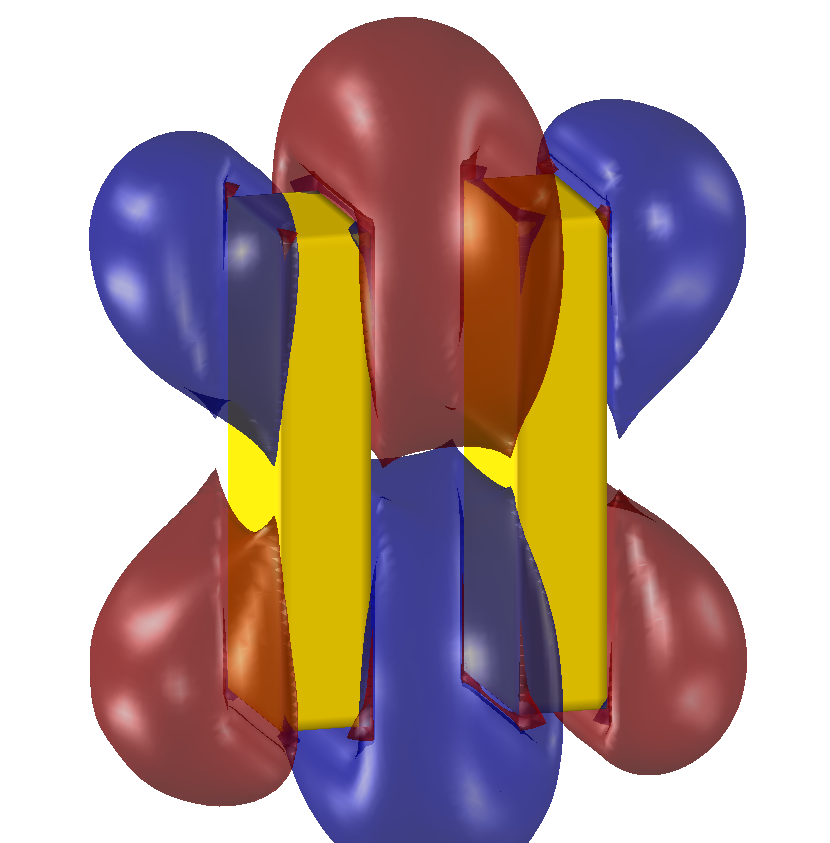
Symposium in context of the PhD defense of Jorn Zimmerling on 2 July. Click here for the program.
Additional information ...
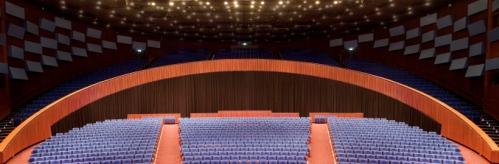
PhD Thesis Defence
- Monday, 2 July 2018
- 12:00-14:30
- Aula Senaatszaal
Model Reduction of Wave Equations
Jörn Zimmerling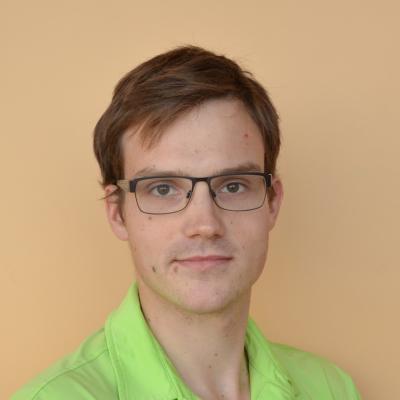
How do you look inside a box without opening it? How can we know whether or not a heart valve is functioning correctly without cutting a person open?
Imaging – the art of seeing the unseeable. A CT-scan at the doctor’s office, crack detection in the wing of an airplane, or medical ultrasound are all examples of imaging techniques that allow us to inspect the interior of an object or person and enable us to observe features that are not directly visible to the naked eye. Science continuously improves upon existing imaging methods and occasionally invents new ones leading to improved image quality and faster image acquisition.
Many imaging applications rely on acoustic, electromagnetic, or elastodynamic waves for imaging. These waves illuminate a penetrable object and an image is formed of its interior from measurements of the transmitted or scattered waves. Being able to efficiently compute wavefields in complex geometries is key in such wavefield imaging problems. To keep up with the developments within the imaging industry to move to larger domains, higher resolution, and larger data sets, new mathematical methods and algorithms need to be developed, since advancements in the computer industry cannot keep up with these demands.
This thesis is about reduced-order modeling of the equations that describe the dynamics of wave propagation. In reduced-order modeling, the aim is to systematically develop a small model that describes a complex system without losing information that is valuable for a specific application. Evaluating such a model is computationally much more efficient than direct evaluation of the unreduced system and in the context of imaging it can lighten the computational burden associated with imaging algorithms. The central question is, of course: How does one construct a model that describes the wave dynamics relevant for a particular application?
Wave equations are partial differential equations that interrelate the spatial and temporal variations of some physical wavefield quantity. When we discretize such equations in space, sparse systems of equations with hundreds of thousands or even millions of unknowns are obtained. Via projection onto a small subspace such a large-scale system can be reduced to a much smaller reduced system. The solution of this small system is called a reduced-order model. A properly constructed reduced-order model can be easily evaluated and gives an accurate wavefield description over a certain time or frequency interval or parameter range of interest.
In this thesis, we discuss different choices for the subspaces that are used for projection in model-order reduction. In particular, we show what types of subspaces are effective for wavefields that are localized and highly resonant and how to efficiently generate such subspaces by exploiting certain symmetry properties of the wave equations. We illustrate the effectiveness of the resulting reduced-order models by computing optical wavefield responses in three-dimensional metallic nano-resonators.
Not all wavefields are determined by a few resonances, of course. Waves can also travel over long distances without losing information; a property that is used by mobile phones every day. The reduction methods developed for resonating fields are not efficient for these types of propagation problems and require a different approach. In this thesis, we present a so-called phase-preconditioning reduction method, in which a specific subspace is generated that explicitly takes the large travel times of the waves into account. We demonstrate the effectiveness of this reduction approach using examples from geophysics, where waves with long travel times are frequently encountered or used to probe the subsurface of the Earth.
Finally, we show how reduced-order modeling techniques can be incorporated in advanced nonlinear imaging algorithms. Here, we focus on an imaging application in geophysics, where the goal is to retrieve the conductivity tensor of a bounded anomaly located in the subsurface of the Earth, based on measured electromagnetic field data that is collected on a borehole axis. We demonstrate that the use of reduced-order models in a nonlinear optimization framework that attempts to solve this imaging problem indeed leads to significant computational savings without sacrificing the quality of the imaging results. To illustrate the wide applicability of model-order reduction techniques in imaging, an additional example from nuclear geophysical imaging is presented as well.
Additional information ...
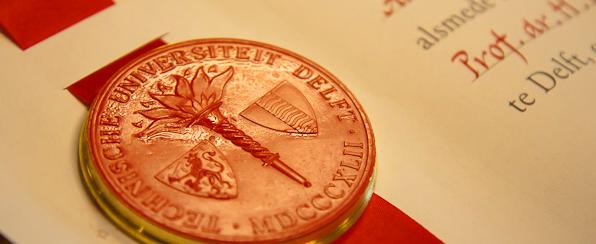
5G Phased Arrays
- Monday, 25 -- Friday, 29 June 2018
- TU Delft
International Summer School on 5G Phased Arrays
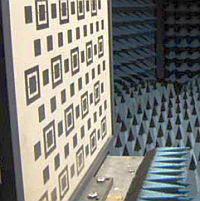
Understanding of phased array operation requires multi- disciplinary approach, which is based on the antenna array, microwave circuit and signal processing theories. By bringing these three areas together, the school provides integral approach to phased array front-ends for 5G communication systems.
At the school the phased array foundations will be considered from antenna, RF technology and signal processing points of view. Realization of 5G capabilities such as high data-rate communication link to moving objects will be discussed. The education will be concluded by a design project.
The summer school is open for all young specialists and researchers from both industry and academia. The attendees should have basic knowledge about EM, electrical circuits and signal processing (graduate courses on electromagnetic waves, electrical circuits including microwave (RF) circuits, and signal processing).
Topics:
- Foundations of antenna arrays
- Antenna array topologies for 5G applications
- Analog and digital beamforming in antenna arrays
- Front-end architecture and performance
- 5G applications and system requirements
Additional information ...
Conferences
- Thursday, 7 -- Friday, 8 June 2018
- 10:30-17:30
- University of Twente, Eschede
PRORISC 2018 Conference
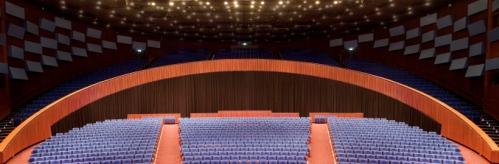
Annual conference on Integrated Circuit (IC) design, organized within the three technical Dutch universities Twente, Delft and Eindhoven
Additional information ...
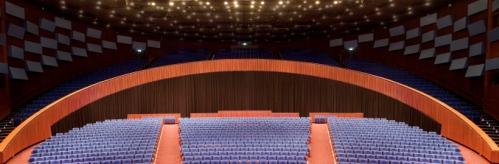
Conferences
- Thursday, 7 -- Friday, 8 June 2018
- 10:30-17:30
SAFE 2018 Conference
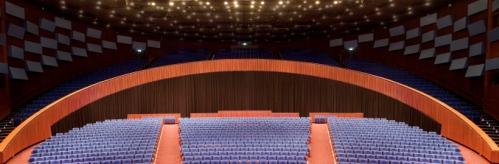
Annual conference on Micro-systems, Materials, Technology and RF-devices, organized within the three technical Dutch universities of Twente, Delft and Eindhoven.
Additional information ...
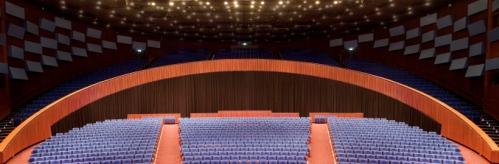
MSc SS Thesis Presentation
- Tuesday, 22 May 2018
- 11:00-11:40
- HB 17.150
Direction-of-Arrival Estimation using an Unsynchronized Array of Acoustic Vector Sensors
Bart Coonen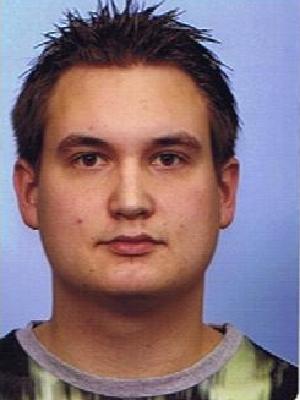
Direction-Of-Arrival (DOA) estimation of acoustic signals is of great interest in various applications including battlefield acoustic and noise localization. Acoustic sensors are employed in an array configuration to estimate DOAs based on the time differences of arrival DOAs. However, the acoustic sensors in the network have all their own Data AcQuisition (DAQ) unit with independent clocks than, it might not be possible to perfectly synchronize the network which affects the performance of the time differences of arrival reliably.
In this thesis we consider the issue of clock synchronization errors in a network where Acoustic Vector Sensors (AVSs) are used. AVSs are shown to be advantageous in terms of direction finding compared to conventional Acoustic Pressure Sensors (APSs) due to their directional particle velocity measurement capability. Initiallity the measurement model for AVSs is presented. After that the behavior of the clocks is incorporated in the measurement model of the full array setup. Subsequently, the effects of the clocks on the MVDR DOA estimation method is discussed.
The model with clock errors is used in the development of three new DOA-estimation methods. The first two techniques are eigenstructure methods that are capable of finding the DOAs regardless of the accuracy of the synchronization. However, to find the DOAs with high accuracy in a real-time application these methods are not due to their high computational cost. Alternatively, the third proposed algorithm takes the DOA estimate from previous methods with low accuracy as its input. The algorithm estimates the DOA in an iterative fashion with high accuracy based on these estimates with low accuracy.
Finally, measurements are conducted in a controlled environment in order to show that these methods are usable in practical situations.
Additional information ...
The possibilities of swarm robotics research
- Thursday, 3 May 2018
- 01:30-02:30
- HB17.150
The possibilities of swarm robotics research
Matthijs Otten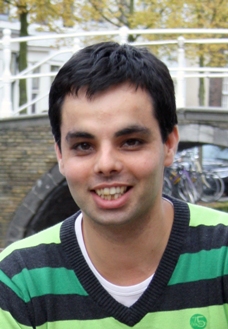
During this presentation, I would like to introduce the topic of swarm robotics and the vision on the development of this technology that the Zebro Team (18th floor, under Chris Verhoeven) has. At Zebro we develop both the swarming technology, as well as the robots that eventually execute the swarming algorithms. We use an integrated approach, letting research and engineering go hand in hand. I would like to show the robotic platform (Zebro) that we have developed to test the swarm, alongside with the technology (Swarming algorithms, ad-hoc communication protocols and localization methods). At the end of my short presentation (+- 10 – 15min) I would like to have a brainstorm session to discuss the possibilities for cooperation. We would like the Zebro Swarming Platform to be useful to the TU Delft, and allow researchers to use the platform for their own research. Research topics for which swarm robotics could include (but are not limited to): • Indoor / Outdoor (Mobile) Localization • Signal processing in sensor networks • Sensor systems for self-deploying sensor networks • Distributed Algorithms • Ad-Hoc Mass Communication • Potential field sensing
Electronic Instrumentation Colloquium
- Monday, 30 April 2018
- 11:00-12:00
- Snijderszaal (LB01.010)
Reducing Switching Artifacts in Chopper Amplifiers
Yoshinori Kusuda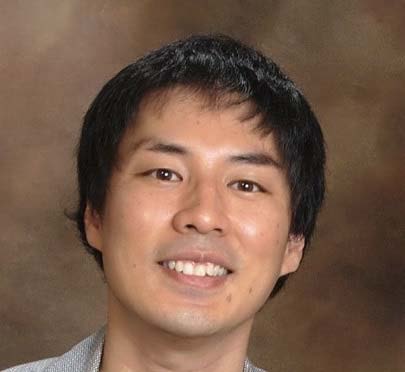
Abstract
Chopping is a technique with which amplifier offset can be reduced to sub-μV levels, at the expense of reduced signal bandwidth due to chopping artifacts such as up-modulated ripple and glitches. In this talk, some circuit techniques to reduce such artifacts are proposed.These circuit techniques have been used in three commercially-available operational amplifiers, whose design and measured performance will be discussed. Lastly, some of the challenges associated in testing low-offset amplifiers in mass-production will be discussed..
BiographyYoshinori Kusuda received the B.S. degree in electrical and electronic engineering in 2002, and M.S. degree in PhysicalElectronics in 2004, both from Tokyo Institute of Technology. Upon his graduation in 2004, he joined the Japan DesignCenter of Analog Devices (ADI) as an IC design engineer. He is currently based in San Jose, CA, U.S.A., working for the Linear and Precision Technology Group of ADI. The focus of his work is on precision CMOS analog designs, including stand-alone amplifiers and application specific mixed-signal products. This has resulted in presentations and papers at IEEE conferences and journals, as well as nine issued U.S. patents. Since August2015, he has been a guest researcher at the ElectronicInstrumentation Laboratory of the TU Delft.
Predicting the intelligibility of speech
- Thursday, 26 April 2018
- 03:45-17:00
- HB17.150
Predicting the intelligibility of speech
Steven van Kuykvisitor New Zealand
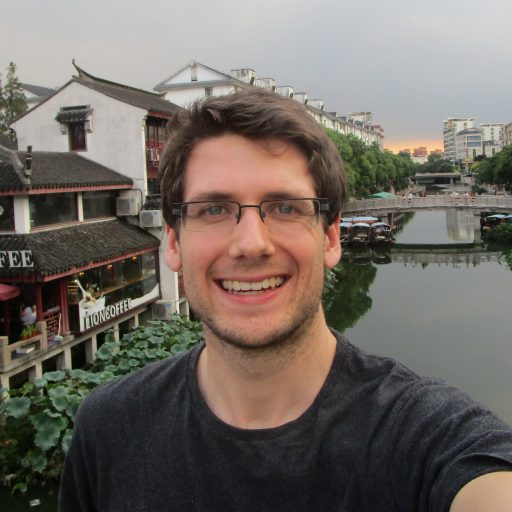
When designing a speech-based communication system it is important to understand how the system will affect intelligibility (i.e., the proportion of correctly identified words). Although formal listening tests can provide valid data, such tests are laborious to conduct. For this reason, algorithms that predict the intelligibility of communication systems have been proposed. This talk will describe a recently proposed algorithm called SIIB, which is based on information theoretic principles. In addition, we will analyze the results of a recent evaluation that investigates the accuracy of popular intelligibility metrics.
Active Implantable Biomedical Microsystems Course
- Tuesday, 24 April 2018
- 13:45-15:30
- DW IZ 1 (140 1verd)
Active Implantable Biomedical Microsystems Course
Vasiliki Giagka, Virgilio Valente, Christos Strydis, Wouter SerdijnDelft University of Technology and Erasmus Medical Center
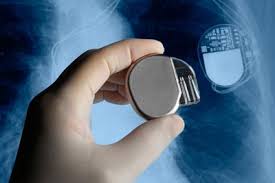
Course on the understanding, design and future developments of active implantable biomedical microsystems, such as cochlear implants, cardiac pacemakers, spinal cord implants, neurostimulators and bioelectronic medicine.
Additional information ...
Signal Processing Seminar
- Thursday, 12 April 2018
- 13:00-15:00
- HB 17.150
Compressive Covariance Sensing
Geert Leus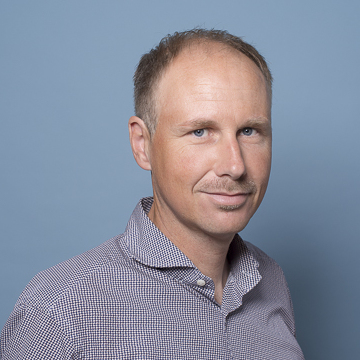
There are many engineering applications that rely on frequency or angular spectrum sensing, such as cognitive radio, radio astronomy, radar, seismic acquisition, and so on. Many of these applications do not require the reconstruction of the full signal, and can perfectly rely on an estimate of the power spectral density (PSD), or in other words, the second-order statistics of the signal. However, the large bandwidths of the involved signals lead to high sampling rates and thus high sampling costs, which can be prevented by a direct compression step carried out in the analog domain (e.g., by means of an analog-to-information converter, multi-coset sampling, analog beamforming, antenna selection, etc.). This leads to the problem of sensing the PSD or covariance using compressive observations, labeled as compressive covariance sensing (CCS). In this tutorial we will give an overview of the state-of-the-art in CCS and present its connections to compressive sensing (CS). We focus on the design constraints of the compression matrices, which are completely different as in classical CS, and elaborate on the estimation/detection techniques to sense the covariance using compressive measurements. In this context, both non-uniform and random sampling are discussed. We further elaborate on distributed CCS, where compressive measurements in one domain are fused in the dual domain, i.e., temporal compressive measurements are gathered at different spatial sensors or spatial compressive measurements from different time slots are combined. Finally, connections to super resolution techniques such as atomic norm minimization are discussed. We end this tutorial by sketching some open issues and presenting the concluding remarks.
Additional information ...
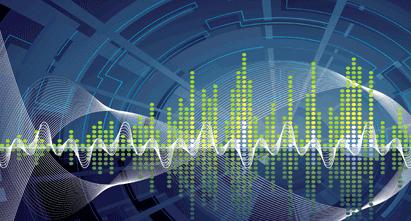
PhD Thesis Defence
- Tuesday, 3 April 2018
- 12:00-14:00
- Aula Senaatszaal
Front-End ASICs for 3-D Ultrasound: From Beamforming to Digitization
Chao Chen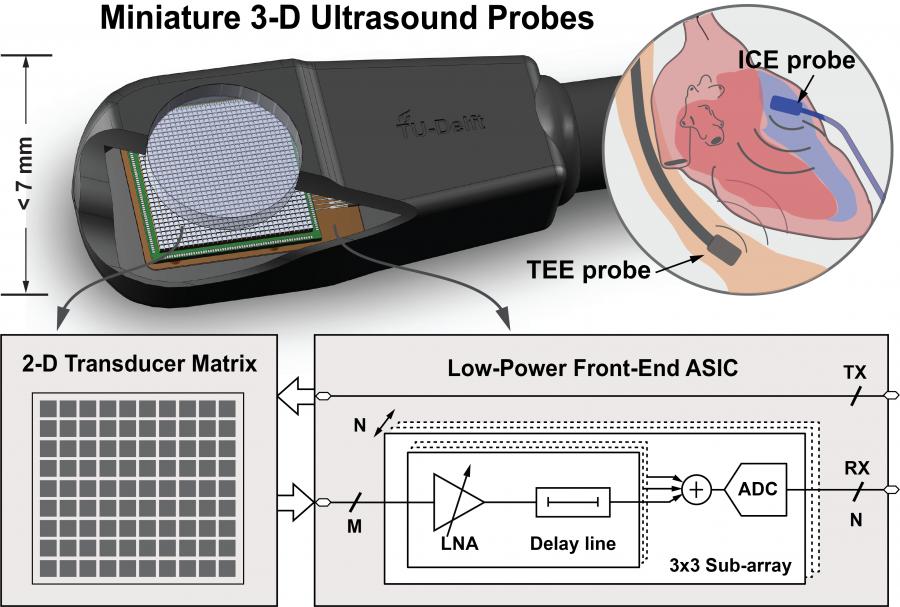
Program:
12:00 - 12:15 Introductory presentation
12:30 - 13:30 Public defense
13:45 - 14:00 Diploma ceremony
Address: Senaatszaal of the Aula Congress Center
SUMMARY
This thesis describes the analysis, design and evaluation of front-end application-specific integrated circuits (ASICs) for 3-D medical ultrasound imaging, with the focus on the receive electronics. They are specifically designed for next-generation miniature 3-D ultrasound devices, such as transesophageal echocardiography (TEE), intracardiac echocardiography (ICE) and intravascular ultrasound (IVUS) probes. These probes, equipped with 2-D array transducers and thus the capability of volumetric visualization, are crucial for both accurate diagnosis and therapy guidance of cardiovascular diseases. However, their stringent size constraints, as well as the limited power budget, increase the difficulty in integrating in-probe electronics. The mismatch between the increasing number of transducer elements and the limited cable count that can be accommodated, also makes it challenging to acquire data from these probes. Front-end ASICs that are optimized in both system architecture and circuit-level implementation are proposed in this thesis to tackle these problems.
The techniques described in this thesis have been applied in several prototype realizations, including one LNA test chip, one PVDF readout IC, two analog beamforming ASICs and one ASIC with on-chip digitization and datalinks. All prototypes have been evaluated both electrically and acoustically. The LNA test chip achieved a noise-efficiency factor (NEF) that is 2.5 × better than the state-of-the-art. One of the analog beamforming ASIC achieved a 0.27 mW/element power efficiency with a compact layout matched to a 150 µm element pitch. This is the highest power-efficiency and smallest pitch to date, in comparison with state-of-the-art ultrasound front-end ASICs. The ASIC with integrated beamforming ADC consumed only 0.91 mW/element within the same element area. A comparison with previous digitization solutions for 3-D ultrasound shows that this work achieved a 10 × improvement in power-efficiency, as well as a 3.3 × improvement in integration density.
The dissertation can be found in the TU Delft repository: http://doi.org/10.4233/uuid:a5002bb0-4701-4e33-aef6-3c78d0c9fd70
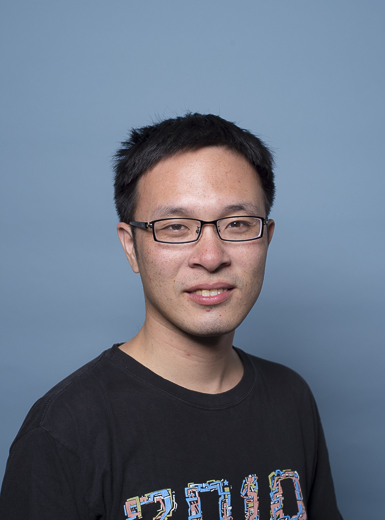
Signal Processing Seminar
- Thursday, 8 March 2018
- 13:00-15:00
- HB 17.150
Time-Varying System Theory - Can it be connected to Graph Signal Processing?
Alle-Jan van der Veen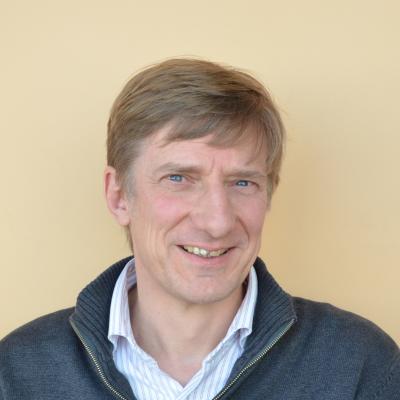
Long time ago, I was developing time-varying system theory. The rows of an "arbitrary" matrix can be viewed as impulse responses of a time-varying system. Next, there is a notion of causality, which relates to upper triangular matrices. And there is a "shift operator" which provides connections between the rows. From these ingredients, it turned out that we can develop a state-space theory where the matrix is implemented by a series of "nodes" that communicate to each other via "states". The ARMA graph filtering work of Elvin e.a. results in similar expressions, where the shift operator is the Laplacian. An open question is if this can be connected to the TV system theory? If so, we know how to do realization theory (given the responses, find a minimal realization, i.e. minimize the number of communication links) and approximation theory (given a realization, find one of lower complexity that has approximately the same response). The talk won't give the answers, but I hope it can start a discussion.
Additional information ...
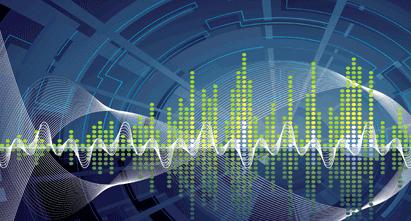
Dutch Ultra Low Power Conference
- Wednesday, 7 March 2018
- 10:00-17:00
- Novio Tech Campus, Nijmegen, NL
The medicine of the future you’ll need to take only once, and it’s a bioelectronic one
Wouter Serdijn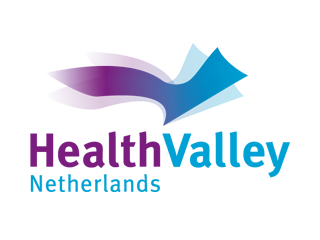
The Dutch Ultra Low Power Conference brings together Belgian and Dutch professionals and companies involved in the development and application of devices with ultra low power technologies. It targets engineers, designers and technical managers in the advanced field of energy harvesting and ultra low power and energy-efficient designs. The keynote will be given by Wouter Serdijn, professor of bioelectronics at Delft University of Technology.
Additional information ...
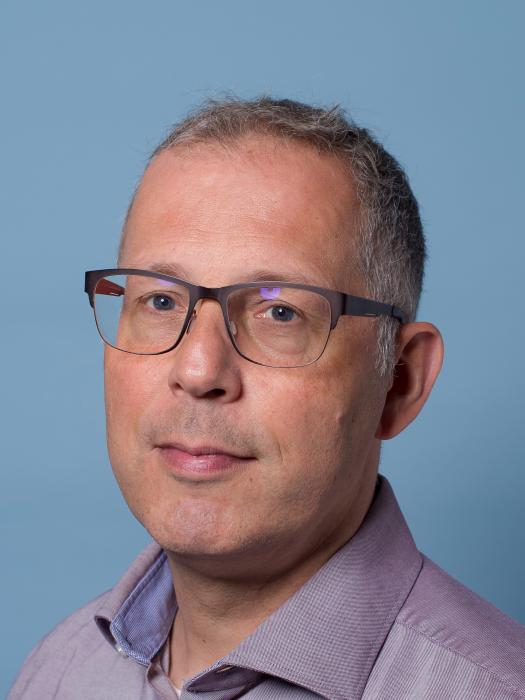
MSc CE Thesis Presentation
- Friday, 23 February 2018
- 16:00-16:40
- EWI HB 17.150
Energy Efficient Feature Extraction for Single-Lead ECG Classification Based On Spiking Neural Networks
Eralp Kolagasioglu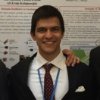
Cardiovascular diseases are the leading cause of death in the developed world. Preventing these deaths, require long term monitoring and manual inspection of ECG signals, which is a very time consuming process. Consequently, a wearable system that can automatically categorize beats is essential.
Neuromorphic machines have been introduced relatively recently in the science community. The aim of these machines is to emulate the brain. Their low power design makes them an optimal choice for a low power wearable ECG classifier.
As features are crucial in any machine learning system, this thesis aims at proposing an energy efficient feature extraction algorithm for ECG arrhythmia classification using neuromorphic machines. The feature extraction algorithm proposed in this thesis consists of the merger of a low power feature detection and a feature selection algorithm. Also, different network configurations have been investigated to achieve classification using an LSM architecture. The resulting system can accurately cluster seven beat types, has an overall classification rate of 95.5%, and consumes an estimate of 803.62 nW.
MEST Symposium
- Friday, 16 February 2018
- Aula Lecture Hall C
Mini Symposium on Hardware Security

Three talks from leading companies in the industry: Brighsight, Intrinsic ID and Riscure with the following topics:
- “Past , Present and Future of Hardware Attacks on Smart Cards and SOCs” by Gerard van Battum, Sr. Security Evaluator at Brightsight;
- “Removing the barriers of securing a broad range of IoT devices” by Dr. Georgios Selimis, Senior Security Engineer, Intrinsic ID;
- “How to use Deep Learning for hardware security testing?” by Marc Witteman (MSc), Chief Executive Officer, Riscure.
Free but required registration at the link below.
Additional information ...
MSc SS Thesis Presentation
- Friday, 16 February 2018
- 10:45-11:30
- EWI Room Electron, (HB first floor, behind the kitchen)
The cocktail party problem: GSVD-beamformers in reverberant environments
Derk-Jan Hulsinga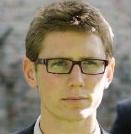
Hearing aids as a form of audio preprocessing is increasingly common in everyday life. The goal of this thesis is to implement a blind approach to the cocktail party problem and challenge some of the regular assumptions made in literature. We approach the problem as wideband FD-BSS. From this field of research, the common assumption of continuous activity is dropped. Instead a number of users detection is implemented as a preprocessing step and ensure the appropriate number of demixing vectors for each time frequency bin. The validity of the standard mixing model used for STFT’s is challenged by looking at the response of a linear array.
Source separation is achieved by demixing vectors based on the GSVD, derived in a model-based approach. While most permutation solvers offer an a posteriori solution for all users, we looked at finding local solutions for a single user. Combining this with the user identification called the alignment step, we conclude that the permutation problem can be reduced to selecting a demixing vector for each discrete time-frequency instance. The correlation coefficient proves to be a sufficient metric to couple reconstructions to the original data as it selects most of the active time-frequency bins.
In simulations, our demixing vectors achieve comparable inteligibility, measured by STOI, as the compared techniques and it is more robust against smaller sample sizes than the theoretically SINR optimal MVDR.
Additional information ...
MSc TC Thesis Presentation
- Wednesday, 14 February 2018
- 14:00-15:00
- EWI HB 17.150
Blind Signal Identification
Dennis van der Geest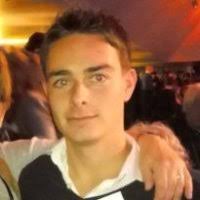
The capability to efficiently find signals of interest in a very dense electromagnetic spectrum is becoming increasingly important with the continuous increase in spectrum usage. In this research project, methods are developed to identify communication signals by estimating signal features (symbol rate, modulation scheme, etc.) in the absence of a-priori knowledge, i.e. blind. By modelling the received communication signal both as a stationary and a cyclostationary process, various feature estimation methods are evaluated based on their computational complexity, their estimation accuracy and their robustness in the presence of signal contamination, such as frequency offsets. By efficiently combining various estimation methods, a signal classification algorithm is derived which is aimed to provide an optimal tradeoff between computational complexity and classification performance.
Additional information ...
Signal Processing Seminar
- Thursday, 8 February 2018
- 13:00-15:00
- HB 17.150
An introduction to distributed signal processing
Richard Heusdens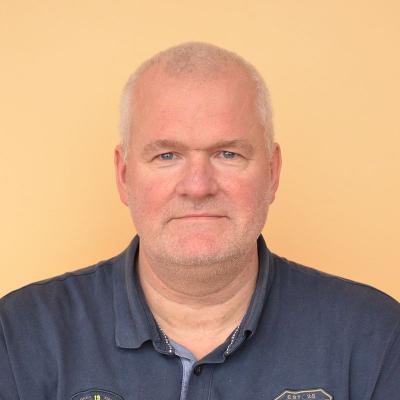
Due to the explosion in size and complexity of modern data sets, it is increasingly important to be able to solve problems with a very large number of features or training examples. In industry, this trend has been referred to as ‘Big Data’, and it has had a significant impact in areas as varied as artificial intelligence, internet applications, computational biology, medicine, finance, marketing, journalism, network analysis, weather forecast, telecommunication, and logistics. As a result, both the decentralized collection or storage of these data sets as well as accompanying distributed solution methods are either necessary or at least highly desirable. In this talk, we will give an introduction to the design of distributed algorithms. We will discuss the basic requirements of these algorithms, like being simple, resource efficient, scalable, robust against changes in network topology, asynchronous, etc. We will demonstrate the design of such algorithm by considering the example of distributed averaging in a sensor network.
Additional information ...
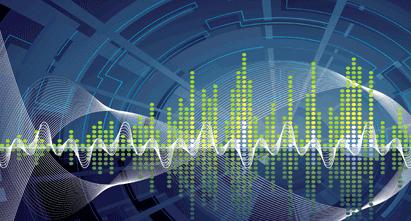
Signal Processing Seminar
- Thursday, 1 February 2018
- 13:30-14:30
- LB 01.010 Snijderszaal
Synchronization for underwater communications based on dual Zadoff-Chu sequences
Yiyin Wang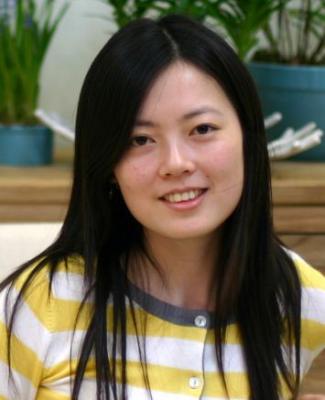
Abstract: Underwater acoustic channels are not only characterized by multipath propagation but also by Doppler scaling effects. These characteristics challenge the preliminary tasks of an acoustic receiver, such as timing and frequency synchronization, and Doppler scale and channel estimation. In this talk, we propose a novel preamble design based on a dual Zadoff-Chu (ZC) sequence. With the help of the well design preamble, a cyclic feature based detector is developed to bypass the requirement of channel statistic information. The Doppler scale estimation is simplified as the frequency estimation adopting the ESPRIT type algorithm. Furthermore, the special structure of the preamble facilitates the estimation of the residual carrier frequency offset (CFO), and the good correlation properties of the preamble enable a low-cost channel estimation. Therefore, with a single preamble, multiple preliminary tasks of the receiver are accomplished. Simulation results indicate the superior performance of the proposed methods.
Additional information ...
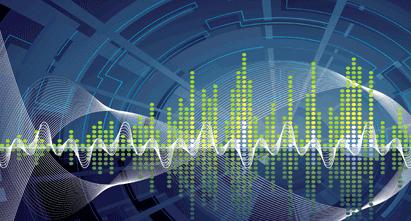
Conferences
- Wednesday, 24 January 2018
- 10:00-17:00
- TU Eindhoven (Zwarte Doos)
WIC Midwinter Meeting on Deep Learning
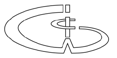
Organized by Werkgemeenschap voor Informatie- en Communicatietheorie, and IEEE Benelux Chapter on Information Theory
Additional information ...
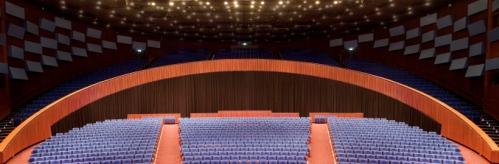
PhD Thesis Defence
- Wednesday, 24 January 2018
- 15:00
- TUD Aula, senaatszaal
Efficient computational methods in magnetic resonance imaging
Jeroen van GemertTechnical University Delft
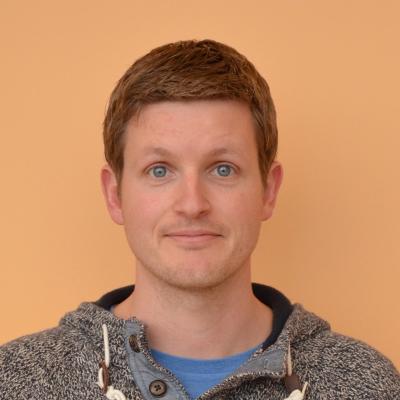
- Wednesday, 20 December 2017
- 16:30-21:00
- PSOR CAFE, building CiTG 23
CAS Christmas dinner
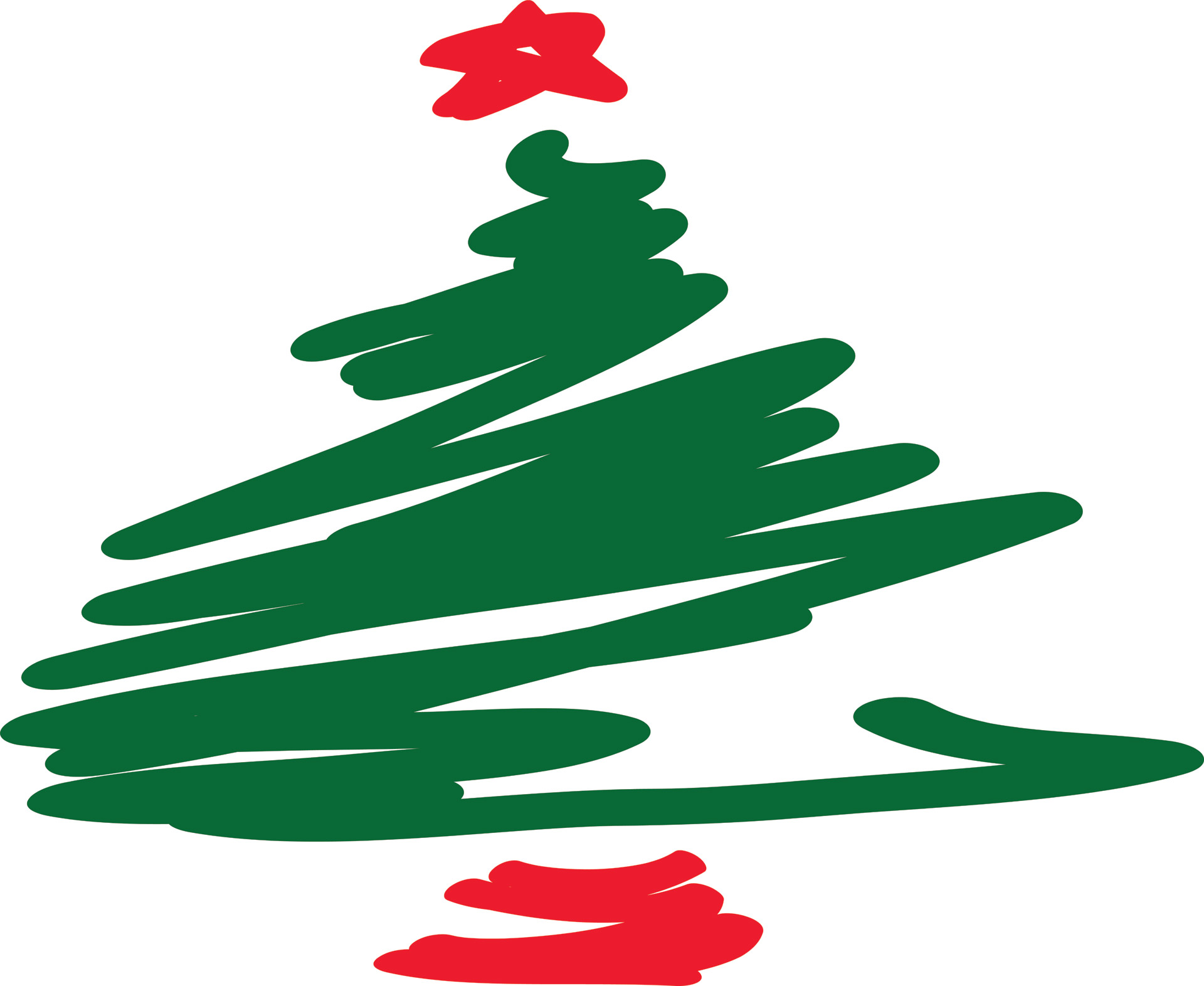
Christmas celebration with food and drinks from all corners of the world.
MSc Thesis Presentation
- Wednesday, 20 December 2017
- 09:30-10:30
Blind Graph Topology Change Detection: A Graph Signal Processing Approach
Ashvant Mahabir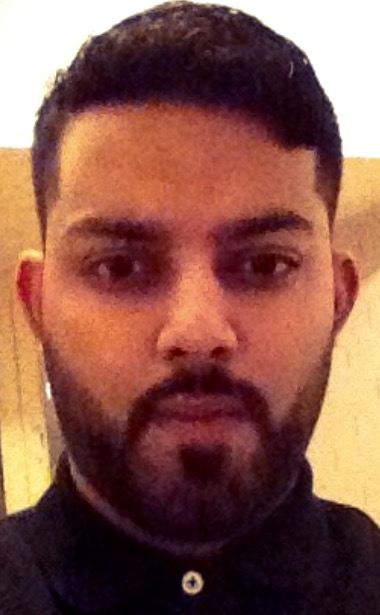
Graphs are used to model irregular data structures and serve as models to represent/capture the interrelationships between data. The data in graphs are also referred as graph signals. Graph signal processing (GSP) can then be applied which basically extends classical signal processing to solve problems. Anomaly detection is an example of such a problem. Two hypothetical situations are given, and a detector has to be designed to distinguish between these. Under the null hypothesis, graph structures are considered to be untouched. Under the alternative hypothesis, (unknown) topological changes might have occurred. Now by incorporating a priori knowledge about the graphs, the decision making process should improve. In most works, a priori knowledge of the graphs under the null and alternative hypothesis was incorpo- rated. This means that detectors were designed which were able to anticipate on possible topological changes. In this thesis, the problem is considered where only a priori knowledge of the graph under the null hypothesis is exploited. This means that detectors are not able to anticipate on potential changes and this where blind detection comes into play. Blind detection is important because it considers a more realistic scenario. In this work, the blind topology change detector (BTCD) and the constrained blind topology change detector (CTCD) are derived which exploit different properties of the data re- lated to the known graph structure. For the BTCD, the bandlimitedness of graph signals was exploited and for the CTCD, the graph signal smoothness. The main question in this work, was to find out what the potentials are with the blind detection principle for graph change detection. Different test scenarios are used to evaluate the detectors on both synthetic and real data. For the BTCD, the obtained results compare well when information about the alternative graph is available. For this detector, the potential of blind detection was highly visible. For bandlimited graph signals, the BTCD as good as detectors using full information. For the CTCD, comparable results (with detectors using full information) are attained for just a few test scenarios. For small changes, the graph signal smoothness seems to be less powerful as to the graph signal bandlimitedness. This study showed that graph change detection is still possible without having full information. Some graph signal properties are more powerful w.r.t. others.
Signal Processing Seminar
- Tuesday, 19 December 2017
- 10:30-11:30
- HB 17.150
When is Network Lasso Accurate: The Vector Case
Nguyen TranA recently proposed learning algorithm for massive network-structured data sets (big data over networks) is the network Lasso (nLasso), which extends the well- known Lasso estimator from sparse models to network-structured datasets. Efficient implementations of the nLasso have been presented using modern convex optimization methods. In this paper, we provide sufficient conditions on the network structure and available label information such that nLasso accurately learns a vector-valued graph signal (representing label information) from the information provided by the labels of a few data points.
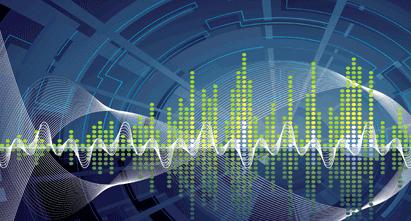
Signal Processing Seminar
- Thursday, 7 December 2017
- 11:00-12:00
- HB 17.150
Semi-supervised learning for likelihood-based classifiers
Marco LoogBioinformatis/Pattern Recognition group
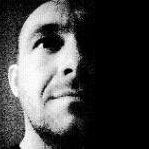
Bio: Marco Loog received an M.Sc. degree in mathematics from Utrecht University and in 2004 a Ph.D. degree from the Image Sciences Institute for the development and improvement of contextual statistical pattern recognition methods and their use in the processing and analysis of images. After this joyful event, he moved to Copenhagen where he acted as assistant and, eventually, associate professor next to which he worked as a research scientist at Nordic Bioscience. In 2008, after several splendid years in Denmark, Marco moved to Delft University of Technology where he now works as an assistant professor in the Pattern Recognition Laboratory. He currently is associate editor of Pattern Recognition and honorary full professor in pattern recognition at the University of Copenhagen. Marco's research interests primarily include all types of variations to supervised learning.
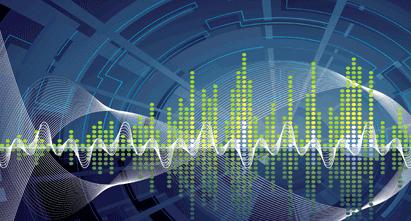
Signal Processing Seminar
- Thursday, 30 November 2017
- 13:30-14:30
- HB 17.150
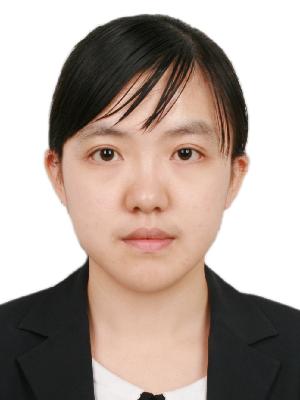
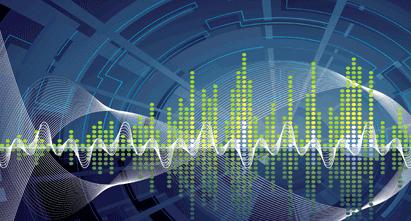
Signal Processing Seminar
- Wednesday, 29 November 2017
- 11:00-12:00
- Lecture Hall H
The Quest for Fast Learning from Few Examples
Andreas Loukas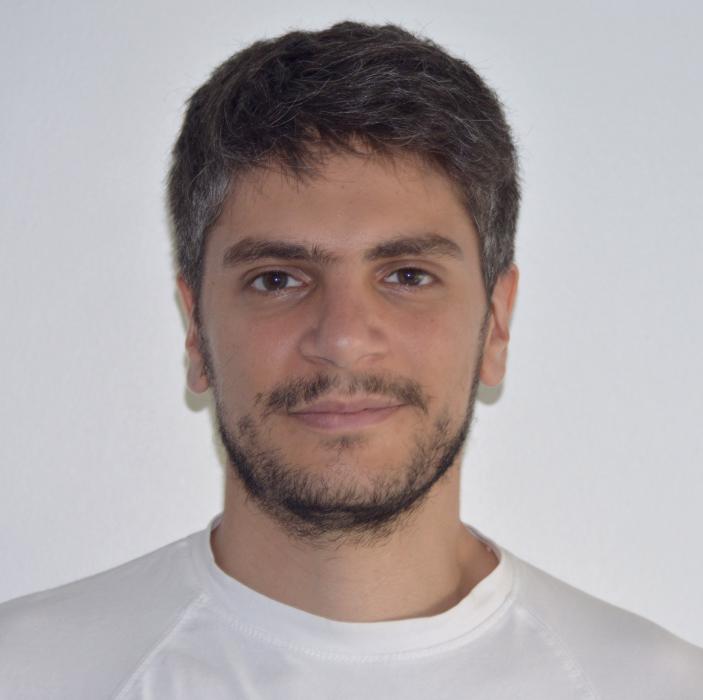
Though the data in our disposal are numerous and diverse, deriving meaning from them is often non trivial. This talk centers on two key challenges of data analysis, relating to the sample complexity (how many examples suffice to learn something with statistical significance) and computational complexity (how long does the computation take) of learning algorithms. In particular, we are going to consider two famous unsupervised algorithms, principal component analysis and spectral clustering, and ask what can they learn when given very few examples or a fraction of the computation time.
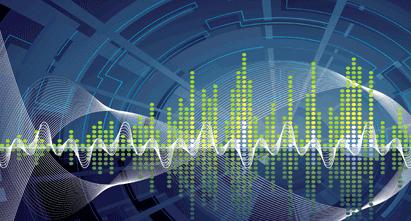
MSc ME Thesis Presentation
- Tuesday, 28 November 2017
- 10:00-11:30
- HB 17.150
FPGA based real time detection and signal, processing of electric nanosecond Partial Discharge (PD) pulses to extract parameters facilitating PD classication.
Ayush Joshi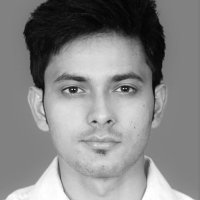
5G Multi-Beam Antenna Topologies
- Friday, 24 November 2017
- 14:30-15:30
- HB17.150
5G Multi-Beam Antenna Topologies
Yanki Aslan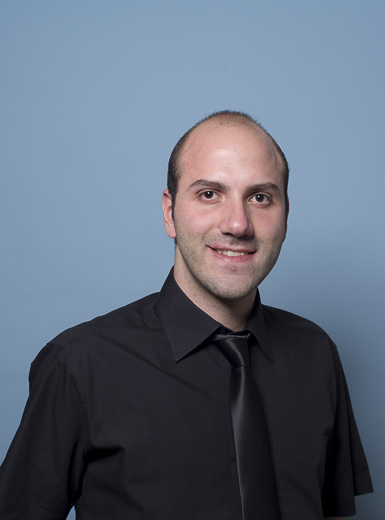
Description: Using the concept of beam-division multiple access, a base station can communicate with multiple users sharing the same time and frequency resources. In this seminar, I will talk about possible ways to design low-cost 5G phased array base station antenna systems at mm-waves for multiple beam forming with enhanced spatial multiplexing, limited interference, acceptable power consumption, passive cooling and suitable processing complexity and speed.
Additional information ...
Signal Processing Seminar
- Thursday, 23 November 2017
- 13:30-14:30
- HB 17.150
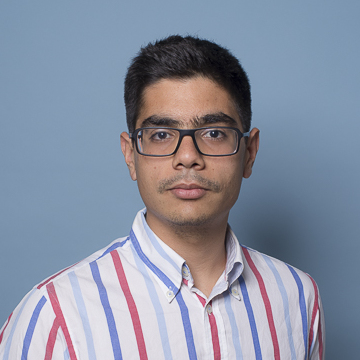
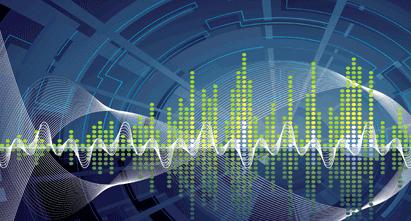
MSc Thesis Presentation
- Wednesday, 22 November 2017
- 10:00-11:30
- HB 17.150
Semi-Controllable Compression Schemes for Ultrasound Imaging
Xuyang Li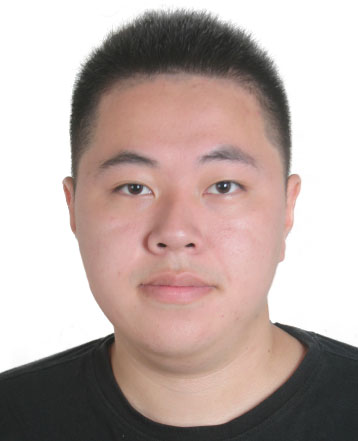
Signal Processing Seminar
- Thursday, 16 November 2017
- 13:30-14:30
- HB 17.150
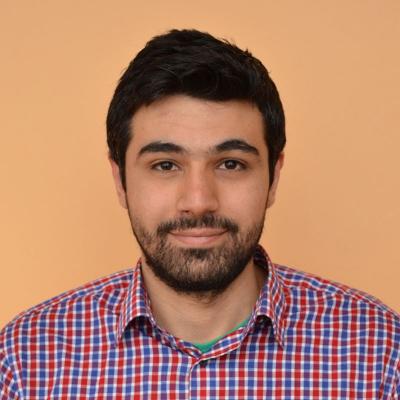
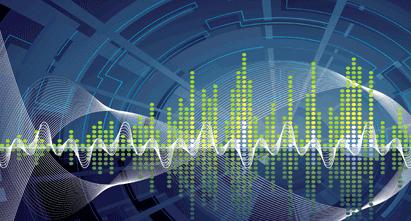
Signal Processing Seminar
- Thursday, 9 November 2017
- 13:30-14:30
- HB 17.150
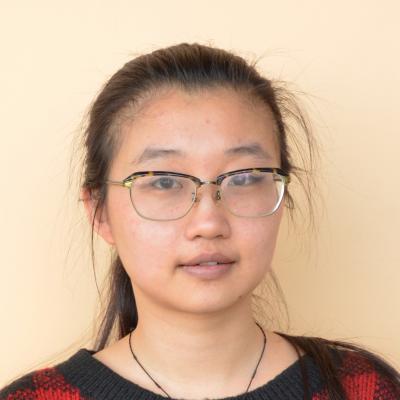
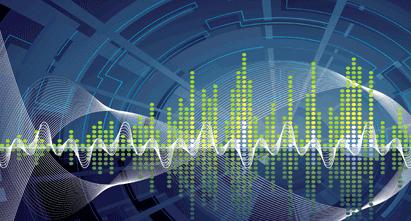
Signal Processing Seminar
- Thursday, 2 November 2017
- 13:30-14:30
- HB 17.150
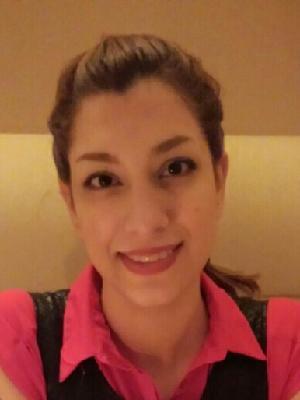
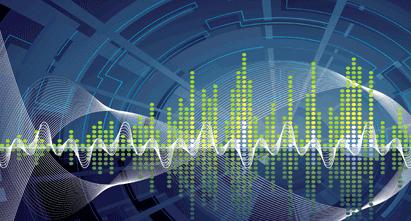
MSc BME thesis presentation
- Wednesday, 25 October 2017
- 10:00-11:00
- Van der Poel-zaal (EWI LB01.220)
System Building Blocks for Mathematical Operators Using Stochastic Resonance -- Application in an Action Potential Detection System
Insani Abdi Bangsa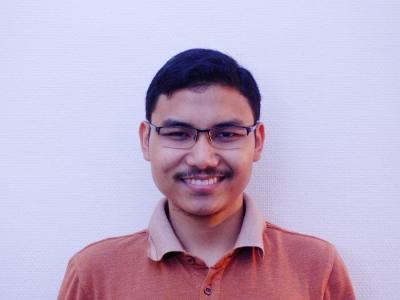
MSc thesis presentation on Stochastic Resonance Systems for Biomedical Applications
PhD Thesis Defence
- Tuesday, 24 October 2017
- 10:00-11:30
- Aula Senaatszaal
Signal Strength Based Localization and Path-Loss Exponent Self-Estimation in Wireless Networks
Yongchang Hu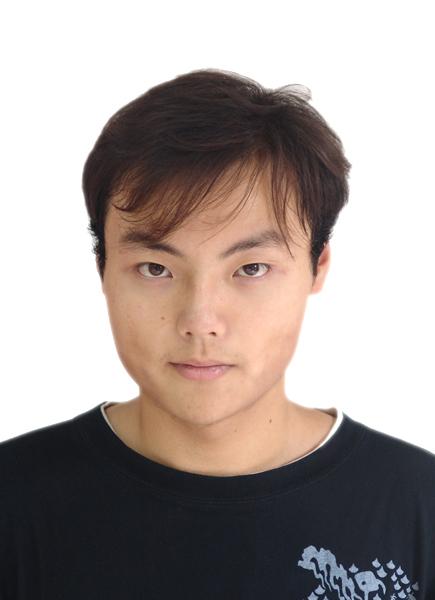
In wireless communications, received signal strength (SS) measurements are easy and convenient to gather. SS-based techniques can be incorporated into any device that is equipped with a wireless chip.
This thesis studies SS-based localization and path-loss exponent (PLE) self-estimation. Although these two research lines might seem unrelated, they are actually marching towards the same goal. The former can easily enable a very simple wireless chip to infer its location. But to solve that localization problem, the PLE is required, which is one of the key parameters in wireless propagation channels that decides the SS level. This makes the PLE very crucial to SS-based localization, although it is often unknown. Therefore, we need to develop accurate and robust PLE self-estimation approaches,which will eventually contribute to the improvement of the localization performance.
We start with the first research line, where we try to cope with all possible issues that we encounter in solving the localization problem. To eliminate the unknown transmit power issue, we adopt differential received signal strength (DRSS) measurements. Colored noise, non-linearity and non-convexity are the next three major issues. To deal with the first two, we introduce a whitened linear data model for DRSSbased localization. Based on that and assuming the PLE is known, three different approaches are respectively proposed to tackle the non-convexity issue: an advanced best linear unbiased estimator (A-BLUE), a Lagrangian estimator (LE) and a robust semidefinite programming (SDP)-based estimator (RSDPE). To cope with an unknown PLE, we propose a robust SDP-based block coordinate descent estimator (RSDP-BCDE) that jointly estimates the PLE and the target location. Its performance iteratively converges to that of the RSDPE with a known PLE.
As mentioned earlier, while generating DRSS measurements, we eliminate the unknown transmit power. This is very similar to the way time-difference-of-arrival (TDOA) methods cope with an unknown transmit time. Both of them use a differencing process to cope with an unknown linear nuisance parameter. Our DRSS study shows the differencing process does not cause any information loss and hence the selection of the reference is not important. However, this apparently contradicts what is commonly known in TDOA-based localization, where selecting a good reference is very crucial. To resolve this conflict, we introduce a unified framework for linear nuisance parameters such that all our conclusions apply to any kind of problem that can be written into this form. Three methods that can cope with linear nuisance parameters are considered by investigating their best linear unbiased estimators (BLUEs): joint estimation, orthogonal subspace projection (OSP) method and differential method. The results coincide with those obtained in our DRSS study. For TDOA-based localization, it is actually the modelling process that causes a reference dependent information loss, not the differencing process. Many other interesting conclusions are also drawn here.
Next, we turn our attention to the second research line. Undoubtedly, knowledge of the PLE is decisive to SS-based localization and hence accurately estimating the PLE will lead to a better localization performance. However, estimating the PLE also has benefits for other applications. If each node can self-estimate the PLE in a distributed fashion without any external assistance or information, it might be very helpful for efficiently designing some wireless communication and networking systems, since the PLE yields a multi-faceted influence therein. Driven by this idea, we propose two closedform (weighted) total least squares (TLS) methods for self-estimating the PLE, which are merely based on the locally collected SS measurements. To solve the unknown nodal distance issue, we particularly extract information fromthe random placement of neighbours in order to facilitate the derivations. We also elaborate on many possible applications thereafter, since this kind of PLE self-estimation has never been introduced before.
Although the previous two methods estimate the PLE by minimizing some residue, we also want to introduce Bayesian methods, such as maximizing the likelihood. Some obstacles related to such approaches are the totally unknown distribution for the SS measurements and the mathematical difficulties of computing it, since the SS is subject to not only the wireless channel effects but also the geometric dynamics (the random node placement). To deal with that, we start with a simple case that only considers the geometric path-loss for wireless channels. We are the first to discover that in this case the SS measurements in random networks are Pareto distributed. Based on that, we derive the CRLB and introduce two maximum likelihood (ML) estimators for PLE selfestimation. Although we considered a simplified setting, finding the general SS distribution would still be very useful for studying wireless communications and networking.
Additional information ...
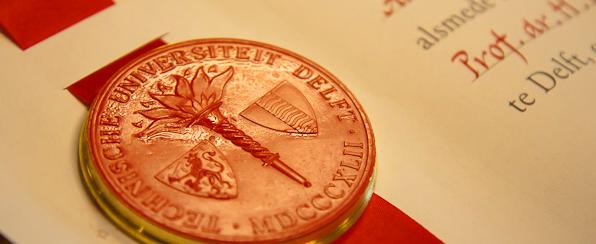
- Friday, 20 October 2017
- 14:00-15:00
- EWI, Snijderszaal (LB 01.010)
Distributed and MIMO radar
Marc LesturgieONERA, France
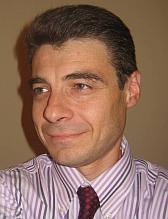
MIMO (multiple-input multiple-output) radar refers to the use of multiple transmitters and receivers, for sensing the environment and the targets present in this environment. Basically MIMO radar uses multiple antennas that transmit correlated or uncorrelated waveforms. For the last ten years MIMO has led to extensive research and publications, both in communications and Radar domains. Why such interest for MIMO in radar? Beside the prolific amount of publications, how to assess the interest of MIMO to overcome the current limitations of conventional radar?
Additional information ...
Signal Processing Seminar
- Thursday, 19 October 2017
- 13:30-14:30
- HB 17.150
Blind calibration of radio astronomical phased arrays
Stefan WijnholdsASTRON

Radio astronomical phased arrays are usually calibrated under the assumption that the observed scene is known. That assumption may not hold if a new frequency window is opened (for example in the case of the currently planned antenna arrays in space observing below 10 MHz) or when there are unexpected source signals such as transients or radio frequency interference (RFI). In this talk, I present recent work on blind calibration of radio astronomical phased assuming that the observed scene is sparse. The resulting method applies sparse reconstruction techniques to the measured array covariance matrices instead of time series data. I discuss the computation speed-up provided by this shift from signal domain to power domain and explain how phase transition diagrams need to be reinterpreted in this context
Additional information ...
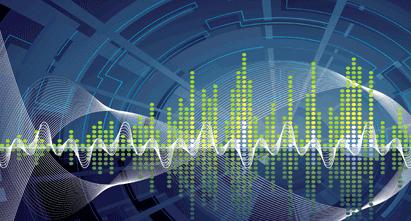
Signal Processing Seminar
- Thursday, 12 October 2017
- 13:30-14:30
- HB 17.150
With the increasing demand for wireless communication with high data rates, more and more spectral resources are allocated for communication systems. This has lead to the risk of decreasing spectrum allocated exclusively for radars. As the use of radars is likely to increase in future, it is necesary to look at how radar and communication systems could co-exist. This talk is an overview of developments in shared use of spectral and hardware resources between these systems.
Additional information ...
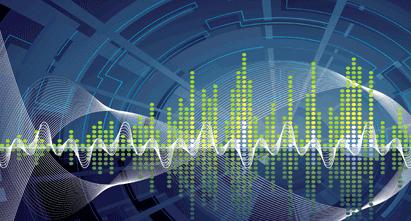
Signal Processing Seminar
- Thursday, 5 October 2017
- 13:30-14:30
- HB 17.150
Accurate Calculation of the Mean Strain of Non-uniform Strain Fields Using a Conventional FBG Sensor
Aydin Rajabzadeh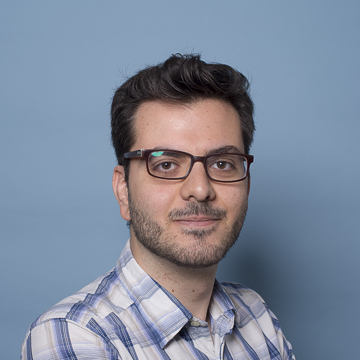
In the past few decades fibre Bragg grating (FBG) sensors have gained a lot of attention in the field of distributed point strain measurement. One of the most interesting properties of these sensors is the presumed linear relationship between the strain and the peak wavelength shift of FBG reflection spectra. However, subjecting sensors to a non-uniform stress field will in general result in a strain estimation error when using this linear relationship, which is due to the difference between the average strain value over the length of the sensor and the point strain value based on the peak wavelength shift of the FBG reflected spectra. In this presentation, we will first introduce a new formulation for analysis of FBG reflected spectra under an arbitrary strain distribution. The presented method is an approximation of the classic transfer matrix model, and will be called the approximated transfer matrix model or ATMM. Using the properties of this new formulation, a new method will be presented that compensates for the mean strain estimation error, and it will be validated using simulations and experimental FBG measurements.
Additional information ...
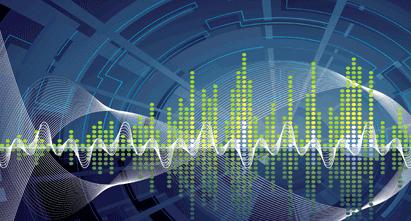
Signal Processing Seminar
- Thursday, 28 September 2017
- 13:30-14:30
- HB 17.150
Graph Sampling for Covariance Estimation
Geert Leus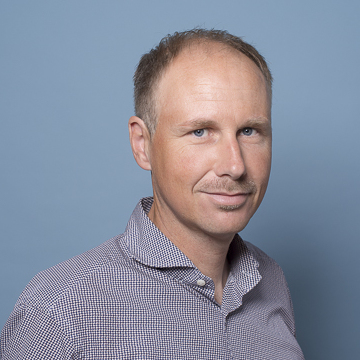
In this talk, the focus is on subsampling as well as reconstructing the second-order statistics of signals residing on nodes of arbitrary undirected graphs. Second-order stationary graph signals may be obtained by graph filtering zero-mean white noise and they admit a well-defined power spectrum whose shape is determined by the frequency response of the graph filter. Estimating the graph power spectrum forms an important component of stationary graph signal processing and related inference tasks such as Wiener prediction or inpainting on graphs. The central result is that by sampling a significantly smaller subset of vertices and using simple least squares, we can reconstruct the second-order statistics of the graph signal from the subsampled observations, and more importantly, without any spectral priors. To this end, both a nonparametric approach as well as parametric approaches are considered. The results specialize for undirected circulant graphs in that the graph nodes leading to the best compression rates are given by the so-called minimal sparse rulers.
Additional information ...
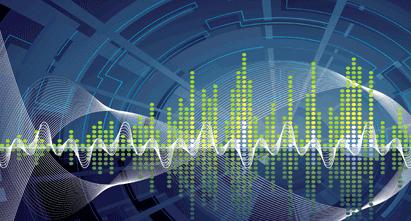
MSc SS Thesis Presentation
- Tuesday, 26 September 2017
- 14:00-14:45
- EWI HB 17.150
Multiway Component Analysis for the Removal of Far Ventricular Signal in Unipolar Epicardial Electrograms of Patients with Atrial Fibrillation
Jelimo Maswan
Atrial fibrillation (AF) is one of the more common clinical arrhythmias with a high morbidity and mortality. Despite this, the electrophysiological and pathological mechanisms associated with AF largely remain a mystery, encouraging the use of ever more sophisticated techniques to extract vital information for diagnostic and therapeutic purposes. Contamination by signals of ventricular origin is considered the main artifact present in high-resolution epicardial electrograms (EGMs) that hinders the accurate and efficient analysis of AF EGM datasets. Furthermore, the complexity and dynamism of AF signals calls for robust data analysis tools that can effectively reduce or remove ventricular activity (VA) while preserving the texture and morphology of atrial activity (AA).
Multiway component analysis, specifically block term decomposition (BTD), proves useful for the decontamination of epicardial EGMs as demonstrated in this project by enabling the automatic estimation of VA on an electrode-by-electrode basis, which is thereafter temporally and/or power spectrally subtracted thus retaining AA at a relatively high accuracy.
The performance of BTD compared to average beat subtraction (ABS) and the more restrictive canonical polyadic decomposition (CPD) is visually verified and numerically confirmed based on a set of key performance indices. Additionally, the technique is entirely data-driven i.e., does not depend on any statistical properties, but if/when available, can contribute to enhanced performance via the imposition of appropriate constraints in the tensor decomposition.
Additional information ...
Signal Processing Seminar
- Thursday, 21 September 2017
- 13:30-14:30
- HB 17.150
Rethinking Sketching as Sampling: A Graph Signal Processing Approach
Fernando GamaSampling of bandlimited graph signals has well-documented merits for dimensionality reduction, affordable storage, and online processing of streaming network data. Most existing sampling methods are designed to minimize the error incurred when reconstructing the original signal from its samples. Oftentimes these parsimonious signals serve as inputs to computationally-intensive linear operator (e.g., graph filters and transforms). Hence, interest shifts from reconstructing the signal itself towards instead approximating the output of the prescribed linear operator efficiently.
In this context, we propose a novel sampling scheme that leverages the bandlimitedness of the input as well as the transformation whose output we wish to approximate. We formulate problems to jointly optimize sample selection and a sketch of the target linear transformation, so when the latter is affordably applied to the sampled input signal the result is close to the desired output. These designs are carried out off line, and several heuristic (sub)optimal solvers are proposed to accommodate high-dimensional problems, especially when computational resources are at a premium.
Similar sketching as sampling ideas are also shown effective in the context of linear inverse problems. The developed sampling plus reduced-complexity processing pipeline is particularly useful for streaming data, where the linear transform has to be applied fast and repeatedly to successive inputs or response signals.
Numerical tests show the effectiveness of the proposed algorithms in classifying handwritten digits from as few as 20 out of 784 pixels in the input images, as well as in accurately estimating the frequency components of bandlimited graph signals sampled at few nodes.
Additional information ...
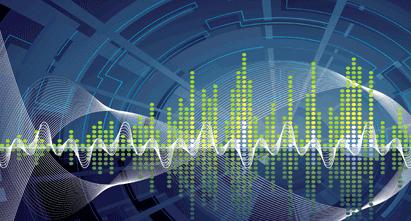
- Friday, 15 September 2017
- 13:30-14:30
- HB17.150
Quantized Distributed Optimization Schemes; a monotone operator approach
Jake Jonkman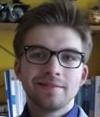
Recently, the effects of quantization on the Primal-Dual Method of Multipliers were studied. In this thesis, we have used this method as an example to further investigate the effects of quantization on distributed optimization schemes in a much broader sense. Using monotone operator theory, the effect of quantization on all distributed optimization algorithms that can be cast as a monotone operator was researched for two different problem subclasses. The averaging problem was used as an example of a quadratic problem, while the Gaussian channel capacity problem was an example of the non-linear problem subclass. A fixed bit rate quantizer was used in combination with a dynamic cell width, to analyse the robustness of distributed optimization schemes against quantization effects. In particular, we have shown that for practical implementations it is possible to incorporate fixed bit rate quantization with dynamic cell width in a distributed optimization algorithm without loss of performance for both problem classes.
Additional information ...
Signal Processing Seminar
- Thursday, 14 September 2017
- 13:30-14:30
- HB 17.150
Signal Processing on Kernel-based Random Graphs
Matthew Morency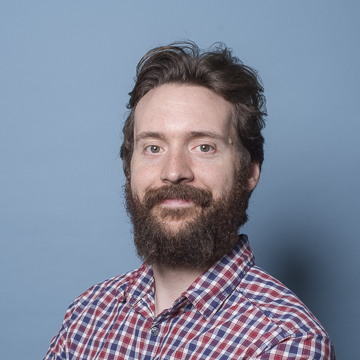
We present the theory of sequences of random graphs and their convergence to limit objects. Sequences of random dense graphs are shown to converge to their limit objects in both their structural properties and their spectra. The limit objects are bounded symmetric functions on $[0,1]^2$. The kernel functions define an equivalence class and thus identify collections of large random graphs who are spectrally and structurally equivalent. As the spectrum of the graph shift operator defines the graph Fourier transform (GFT), the behavior of the spectrum of the underlying graph has a great impact on the design and implementation of graph signal processing operators such as filters. The spectra of several graph limits are derived analytically and verified with numerical examples.
Additional information ...
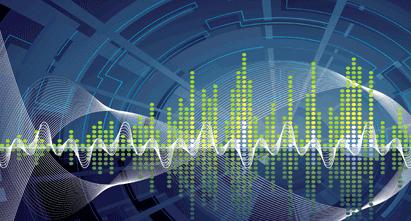
Signal Processing Seminar
- Thursday, 7 September 2017
- 13:30-14:30
- HB 17.150
Electromagnetic 3D anisotropic imaging in the model reduction framework
Jörn ZimmerlingT.U. Delft
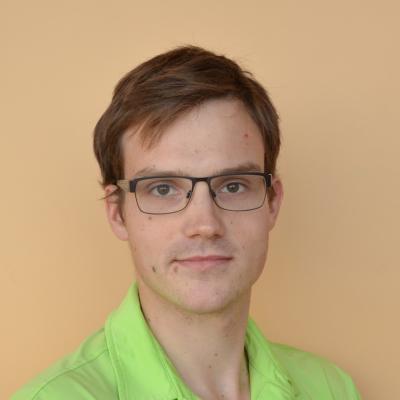
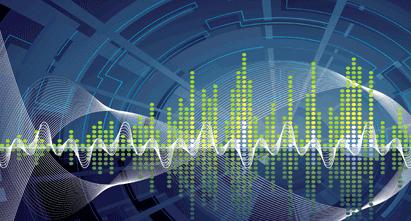
Msc Thesis Presentation
- Thursday, 31 August 2017
- 13:30-14:30
- HB 17.150
Multi-FPGA Interconnection Simulation
He Zhang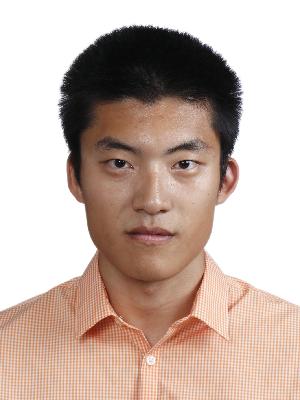
The scalable simulation of neuron communication needs a large amount of computing resources. The high throughput of data cause the high requirement of interconnection network. This thesis is aimed at the finding the proper multi-FPGA connection for the neuron network. First describe the characteristics of the network in terms of the topology, routing and flow control. To find the proper connection, analysis of the throughput for the different network with different traffic pattern by considering the hopcount and bandwidth are made. It shows that the multicast is a good solution. Based on the interconnection router architecture, a simulator is built to make a cycle accurate simulation in systemC and test different traffic pattern by unicast and multicast routing. To break the limitation of FPGA ports, the source synchronous serdes connection is built by using the primitive in the Xilinx FPGA. With the requirement of bandwidth, the possible solution of number of channels and the overhead are anaylsed.
Msc Thesis Presentation
- Wednesday, 30 August 2017
- 13:30-14:30
- HB 17.150
Full-Custom Multi-Compartment Synaptic Circuits in Neuromorphic Structures
Xuefei You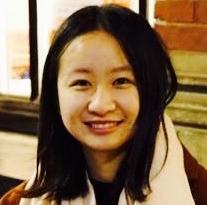
Neuromorphic engineering, aiming at emulating neuro-biological architectures in efficient ways, has been widely studied both on component and VLSI system level. The design space of neuromorphic neuron, the basic unit to conduct signal processing and transmission in nervous system, has been widely explored while that of synapse, the specialized functional unit connecting neurons, is less investigated. In this thesis, a current-based phenomenological synapse model with power-efficient structures, consisting of efficient synaptic learning algor ithms and multi-compartment synapses, has been proposed. A vertical insight is given into the design space of spike-based learning rules in regards to design complexity and biological fidelity. Due to various biological conducting mechanisms, the receptors, namely AMPA, NMDA and GABAa, demonstrate different kinetics in response to stimulus. The designed circuit offers distinctive features of receptors as well as the joint synaptic function. A better computation ability is demonstrated through a cross-correlation detection experiment with a recurrent network of synapse clusters. The analog multi-compartment synapse structure is able to detect and amplify the temporal synchrony embedded in the synaptic noise. The maximum amplification level is 2 times larger than that of single-receptor configurations The final design implemented in UMC65nm technology consumes 1.92, 3.36, 1.11 and 35.22pJ per spike event of energy for AMPA, NMDA, GABAa receptors and the advanced learning circuit, respectively.
Additional information ...
Msc Thesis Presentation
- Tuesday, 29 August 2017
- 13:00-14:00
- HB 17.150
A 32 x 32 Spiking Neural Network System On Chip
Ester Stienstra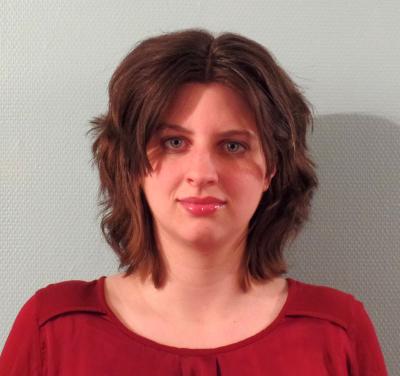
In this thesis a prototyping system on chip of a 32 x 32 spiking neural network is presented. This network has been designed in UMC 65. In order to determine which neuron model to use three different analog CMOS neuron models are studied. One of these models is used in the network. The network consists of arrays of synapses and neurons, 32 synapses for each neuron. In order to be able to control all the synaptic inputs and read all the neural outputs, logic is presented that minimizes the number of pads needed, while maintaining controllability and keeping all the important information in the neural signal. Simulations are performed to determine the influence of several behaviors of the neuron and the synapse on the output of the network. Also a floorplan and place and route design for the chip are presented.
Msc Thesis Presentation
- Monday, 28 August 2017
- 10:00-11:00
- HB 17.150
Source-Synchronous Interface with All-Digital Data Recovery
Shizhao ZhangThis thesis proposes a low-cost high-efficiency source-synchronous interface for high-speed inter-chip communication. The interface is composed of LVDS transceivers as external I/O buffers, and an alldigital data recovery, which can calibrate the received data phase to be aligned to the 90◦ phase of the received half-rate reference clock, for error free data sampling. The proposed data recovery adopts a fulldigital scheme, which uses time-to-digital converters (TDC) as phase acquisition, a digitally-controlled delay line (DCDL) to calibrate the phase, and a finite-state machine (FSM) as the control unit. Reference clock generated from phase-locked loops (PLL) or delay-locked loops (DLL) is not needed for the proposed data recovery. The interface is implemented in UMC 65 nm Low-leakage technology, with circuits designed at both transistor-level and RTL-level. The postlayout simulation shows the proposed interface works properly with data rates from 412.4Mbps to 1.25Gbps in all process corners. The total layout area is 688 ñm × 87 ñm, and the total power consumption is 16.74 mW.
- Tuesday, 22 August 2017
- 14:00-15:00
- Dijkstrazaal, HB09.150
CSI-EPT: Towards Practical Implementation
Jiying Dai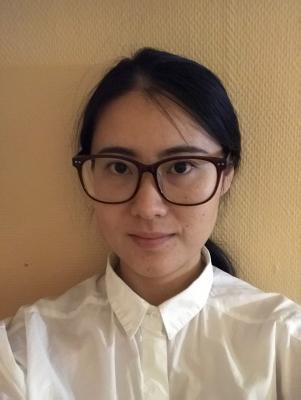
The information of electrical properties of biological tissues within human body can be useful information for diagnosis, tissue characterization, hyperthermia treatment planning and MR safety control. Contrast Source Inversion Electrical Properties Tomography (CSI-EPT) is an MR base imaging modality, which reconstructs the electrical properties of the object. However, this method has not been implemented in practice due to the following issues: 1) The exact transmit phase, which is a necessary input of regular CSI-EPT algorithm, is not available from MR acquisitions. 2) The incident field, another input of CSI-EPT reconstruction, cannot be measured directly. 3) The reconstruction loses sensitivity at those regions with low electrical fields. 4) The sufficiency of the two-dimensional configuration, which has been used in most published results as an approximation
Signal Processing Seminar
- Thursday, 27 July 2017
- 13:30-14:30
- HB 17.150
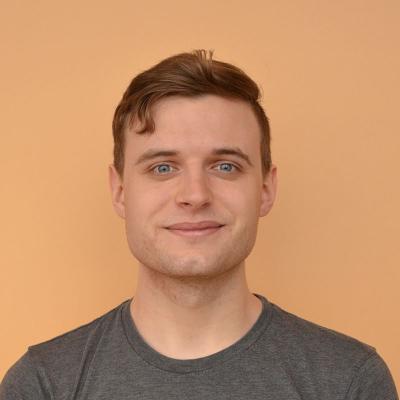
Thomas is going to present his recent research.
Additional information ...
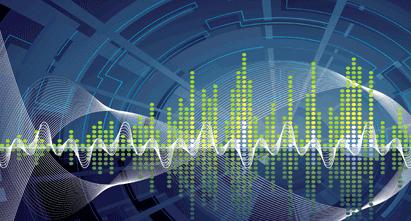
Network topology inference from graph stationary signals
- Friday, 21 July 2017
- 10:00-11:00
- HB17.150
Network topology inference from graph stationary signals
Antonio Garcia Marques (King Juan Carlos University)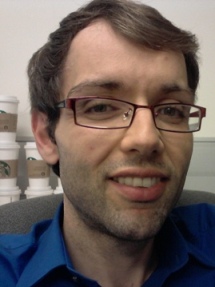
We address the problem of identifying a graph structure from the observation of signals defined on its nodes. Fundamentally, the unknown graph encodes direct relationships between signal elements, which we aim to recover from observable indirect relationships generated by a diffusion process on the graph. The fresh look advocated here permeates benefits from convex optimization and stationarity of graph signals, in order to identify the graph shift operator (a matrix representation of the graph) given only its eigenvectors. These spectral templates can be obtained, e.g., from the sample covariance of independent graph signals diffused on the sought network. The novel idea is to find a graph shift that, while being consistent with the provided spectral information, endows the network with certain desired properties such as sparsity. To that end we develop efficient inference algorithms stemming from provably-tight convex relaxations of natural nonconvex criteria, particularizing the results for two shifts: the adjacency matrix and the normalized Laplacian. Algorithms and theoretical recovery conditions are developed not only when the templates are perfectly known, but also when the eigenvectors are noisy or when only a subset of them are given. Numerical tests showcase the effectiveness of the proposed algorithms in recovering social, brain, and amino-acid networks.
Additional information ...
MSc Defense of Boliang Xu
- Friday, 21 July 2017
- HB17.150
Packet loss concealment for speech transmissions in real-time wireless applications
Boliang Xu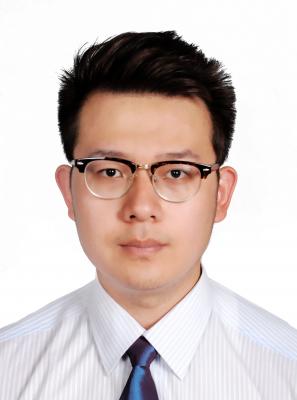
Packet communication applications cannot guarantee correct delivery of every packet. Congestions and interferences in the network lead to lost packets. However, real-time applications require timely delivery of data or information and always tolerate packet loss to achieve this aim. When some speech packets are lost, packet loss concealment (PLC) is used to replace the missing speech.
In this thesis, after investigating packet loss characteristics in realistic wireless networks and problems in existing PLC algorithms, we propose a new PLC scheme named adaptive PLC, which is composed of three algorithms: odd-even interpolation, waveform similarity matching and silence substitution. Adaptive PLC adjusts the algorithm to use depending on loss situations. Odd-even interpolation recovers the loss by interpolating odd or even samples in a packet. Waveform similarity matching estimates waveform segments from correctly received or already recovered packets. Silence substitution just fills in the missing part by zeros.
The adaptive PLC achieves improvements in speech quality relative to each single PLC algorithm and other existing PLC algorithms.
Signal Processing Seminar
- Thursday, 20 July 2017
- 13:30-14:30
- HB 17.150
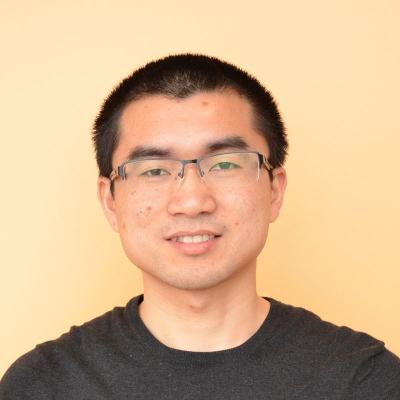
Jie is going to present his recent research.
Additional information ...
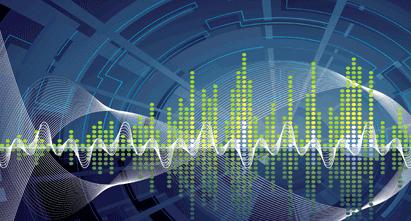
Signal Processing Seminar
- Thursday, 13 July 2017
- 13:30-14:30
- HB 17.150
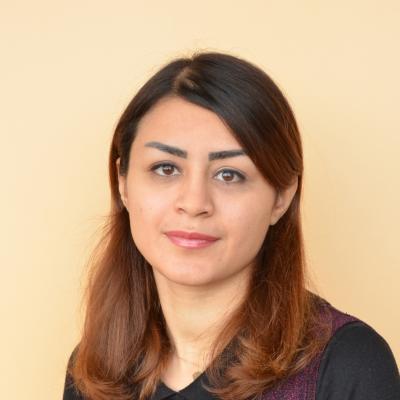
Bahareh is going to present her recent research.
Additional information ...
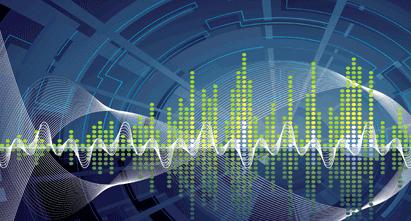
MSc CE Thesis Presentation
- Wednesday, 12 July 2017
- 13:30-14:15
- EWI HB 17.150
An Accurate System-Level Device Aging Assessment and Mitigation Simulation Framework
Evelyn Rashmi Jeyachandra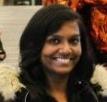
As technology scaling enters the nanometer regime, device aging effects cause quality and reliability issues in CMOS Integrated Circuits (ICs), which in turn shorten its lifetime. Evaluating system aging through circuit simulations is very complex and time consuming. In this thesis, a framework is proposed, which allows for the evaluation of long-term aging effects of ICs and the corresponding measures to counteract premature failure. The focus of this work lies in the abstraction of low-level aging models to system-level models, in order to facilitate swift high-level simulation, without any knowledge of underlying circuit dynamics.
Two major aging mechanisms, namely Negative Bias Temperature Instability (NBTI) and Channel Hot Carrier (CHC) degradation are considered for analysis. System-level aging management is performed with the prototype of a System-on-Chip (SoC) including a Management Unit (MU), which counteracts aging by employing Dynamic Voltage Scaling (DVS), Dynamic Frequency Scaling (DFS), and Adaptive Body Biasing (ABB). The simulation platform prototype is based on System-C and a 65-nm technology library. This SoC simulation computes path delay using characterized models, which represent the aged behaviour of individual circuit elements. Results show that the obtained values are within 2% of circuitlevel simulation values.
Furthermore, the System-C implementation has a shorter execution time with an approximate speedup of 15 times over conventional circuit simulators (e.g. Cadence NCSim).
Additional information ...
Microelectronics IoT Pitch
- Wednesday, 5 July 2017
- 15:00-18:00
- New Sports Cafe - TU Deft
and Summer Drink
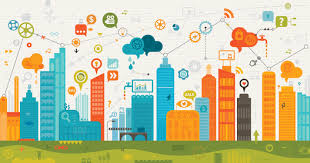
All ME-MSc’s and ME-employees are cordially invited to make a 2-minute pitch for an interesting and unexpected IoT application.
The format is free like the level of seriousness and feasibility are but there is a meaningful purpose as the event is meant to inspire the definition of technology integrating projects in the field of IoT. The pitches will be ‘graded’ by measuring the intensity of the applause.
The pitch wil be followed by the yearly Summer Drink of the Microelectronics Department.
Signal Processing Seminar
- Tuesday, 4 July 2017
- 13:15-14:15
- Katwijkzaal HB 3.250
Image domain gridding for radio astronomy
Bas van der TolASTRON
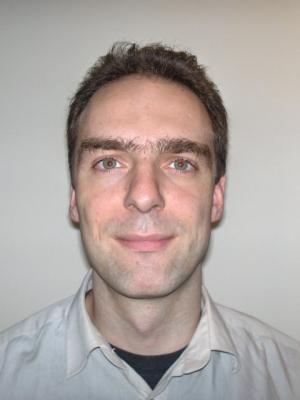
Fast implementations of a "non-uniform FFT" operation
Additional information ...
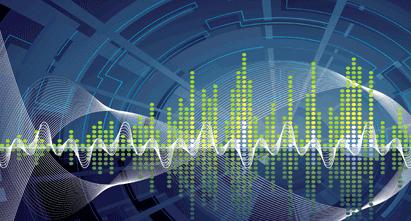
Signal Processing Seminar
- Thursday, 29 June 2017
- 13:30-14:30
- HB 17.150
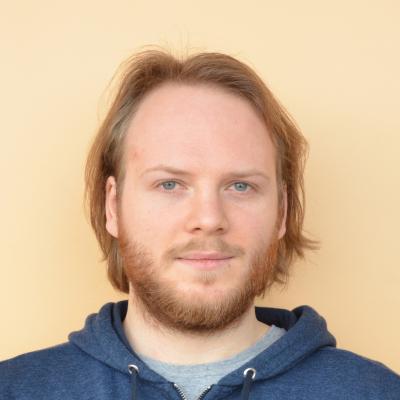
Pim is going to present his recent research.
Additional information ...
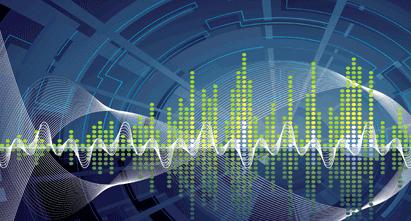
Signal Processing Seminar
- Thursday, 22 June 2017
- 13:30-14:30
- HB 17.150
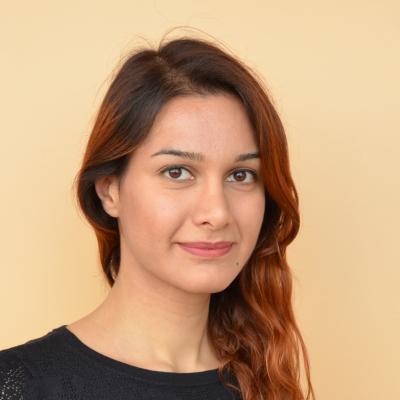
Shahrzad is going to present her recent research.
Additional information ...
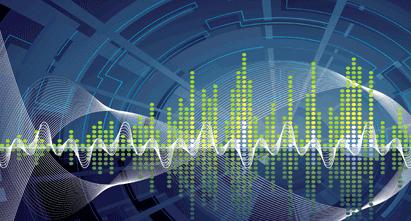
Signal Processing Seminar
- Friday, 16 June 2017
- 13:30-14:30
- HB 17.150
3D direction of arrival estimation of multiple audio sources with spherical microphone arrays
Despoina PavlidiUniversity of Crete, Department of Computer Science, Heraklion, Crete, Greece
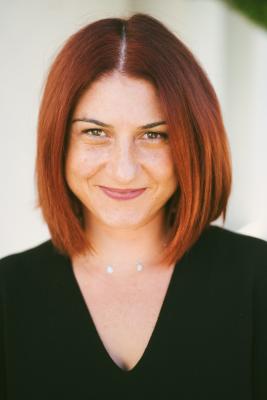
Abstract: Direction of arrival estimation plays a central role in numerous signal-processing applications, such as smart home automation, surveillance systems, etc. Until recently the research community was mainly interested in single-dimensional direction of arrival (DOA) estimation by deploying linear or planar microphone arrays. Nowadays the focus has turned also towards spherical microphone arrays, which enable the more accurate capturing of the acoustic wavefield, hence enabling two-dimensional DOA estimation, i.e., the azimuth and elevation of an active audio source. In this talk we will present our proposed methodologies for DOA estimation in the 3D space. Our first proposed method relies on energetic analysis. We estimate the sound intensity vector on selected time-frequency elements of the spectrum and post-process the estimates utilizing 2D histogram representations. We enhance our approach by applying beamforming around local intensity vector directions. We call our hybrid approach spatially constrained beamforming (SCB). Our second proposed method improves the performance of two grid-based approaches, namely the steered response power (SRP) and the multiple signal classification (MUSIC) algorithm, both formulated in the spherical harmonic domain. We propose to derive local DOA estimates from the power map for SRP and the pseudospectrum for MUSIC. From these local DOA estimates we form a 2D histogram that we process to derive the final multiple sources directions.
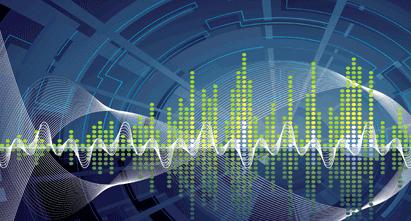
Signal Processing Seminar
- Thursday, 15 June 2017
- 13:30-14:30
- HB 17.150
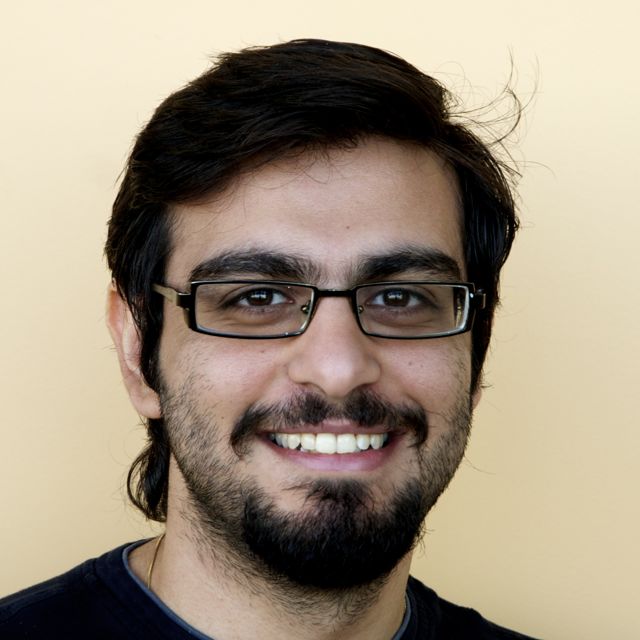
Millad is going to present his recent research.
Additional information ...
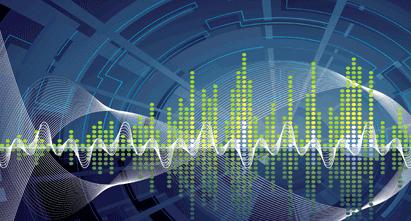
Signal Processing Seminar
- Thursday, 8 June 2017
- 13:30-14:30
- HB 17.150
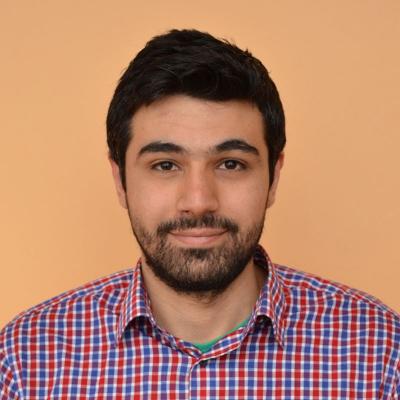
Jamal is going to present his recent research.
Additional information ...
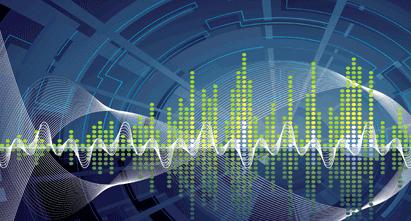
Signal Processing Seminar
- Thursday, 1 June 2017
- 13:30-14:30
- HB 17.150
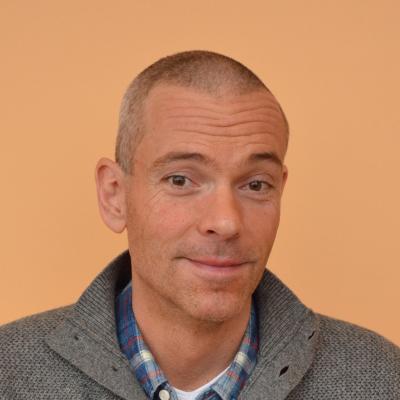
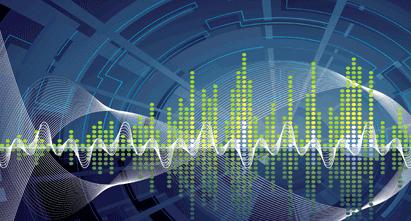
DCSE/SIAM Seminar
- Wednesday, 24 May 2017
- 11:00-12:00
- EWI Lipkenszaal
Untangling nonlinearity in inverse scattering with data-driven reduced order models
Vladimir DruskinSchlumberger-Doll, Boston
We consider an inverse problem for the acoustic wave equation, where an array of sensors probes an unknown medium with pulses and measures the scattered waves. The goal is to determine from these measurements the structure of the scattering medium, modeled by a spatially varying acoustic impedance function.
Many inversion algorithms assume that the mapping from the unknown impedance to the scattered waves is approximately linear. The linearization, known as the Born approximation, is not accurate in strongly scattering media, where the waves undergo multiple reflections before they reach the sensors in the array. Thus, the reconstructions of the impedance have numerous artifacts. In this talk we show that it is possible to remove the multiple scattering effects from the data registered at the array, using a reduced order model (ROM). The ROM is defined by an orthogonal projection of the wave propagator operator on the subspace spanned by the time snapshots of the solution of the wave equation. The snapshots are known only at the sensor locations, which is enough information to construct the ROM. The main result of the paper is a novel algorithm that uses the ROM to map the data to its Born approximation. We develop the algorithm from first principles and demonstrate its accuracy with numerical simulations.
MSc thesis presentation
- Monday, 22 May 2017
- 14:00-14:45
- t.b.d.
A Low-Complexity CMOS Receiver for UWB siqnals
Ernesto Huaman
Ernesto's MSc thesis presentation on localization using UWB and its implementation in CMOS
MSc CE Thesis Presentation
- Friday, 19 May 2017
- 13:30-14:15
- HB 17.150
Digital Neuron Cells for Highly Parallel Cognitive Systems
Haipeng Lin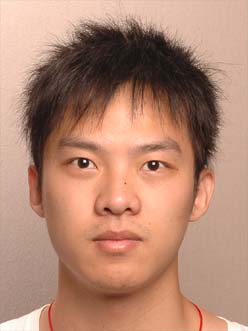
The biophysically-meaningful neuron models can be used to simulate human brain behavior. The understanding of neuron behaviours is expected to have prominent role in the fields such as artificial intelligence, treatments of damaged brain, etc. Several neuron models exist, which vary in a level of accuracy complexity, speed, etc. In this thesis, a general simulator is presented, which can implement Hodgkin-Huxley(HH) model, Integrate and Fire model and Izhikevich model in the same architecture in a real-time. The different neuron models can be selected in the simulator to evaluate various network configurations, such as the amount of the neuron cells in the network, properties of the neuron models and so on. The simulator communication cost grows approximately linearly with the number of the neuron cells. Similarly, implementation over multiple Field Programmable Gate Array(FPGA) devices is possible. At last, this simulator is synthesised and validated on a FPGA device. The pipeline is added to reduce resource cost and latency.
Signal Processing Seminar
- Thursday, 18 May 2017
- 13:30-14:30
- HB 17.150
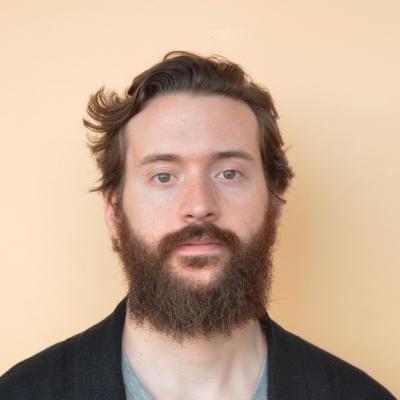
Matthew will present his recent research findings.
Additional information ...
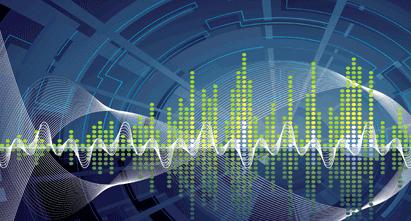
Conferences
- Thursday, 11 -- Friday, 12 May 2017
- TU Delft Science Center
2017 Symposium on Information Theory and Signal Processing in the Benelux
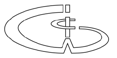
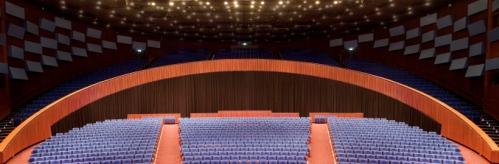
Signal Processing Seminar
- Thursday, 4 May 2017
- 13:30-14:30
- HB 17.150
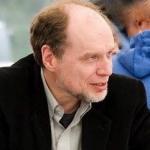
Bastiaan is going to present his recent research.
Additional information ...
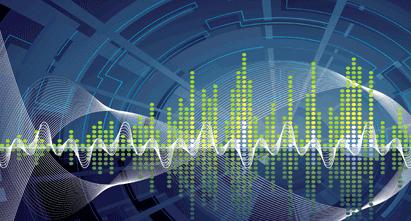
Signal Processing Seminar
- Thursday, 20 April 2017
- 13:30-14:30
- LB01.170 (Timmanzaal)
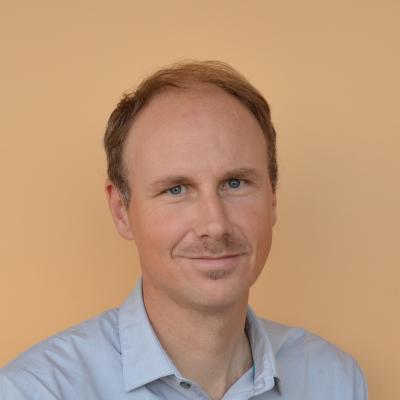
Geert is going to present his recent research.
Additional information ...
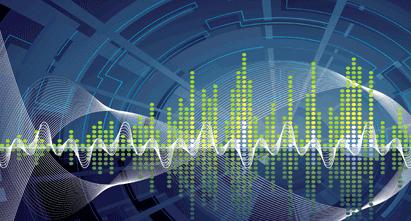
Conferences
- Friday, 14 April 2017
- 10:00-12:00
Eurosensors dead-line
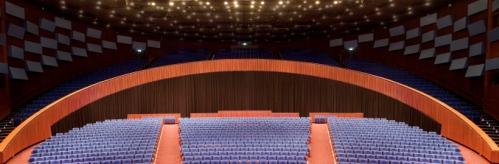
Signal Processing Seminar
- Thursday, 13 April 2017
- 13:30-14:30
- LB01.010 (Snijderszaal)
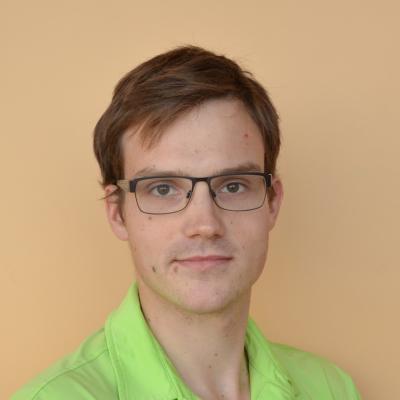
Jorn is going to present his recent research.
Additional information ...
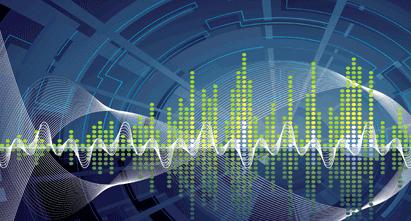
Signal Processing Seminar
- Thursday, 6 April 2017
- 13:30-14:30
- HB 17.150
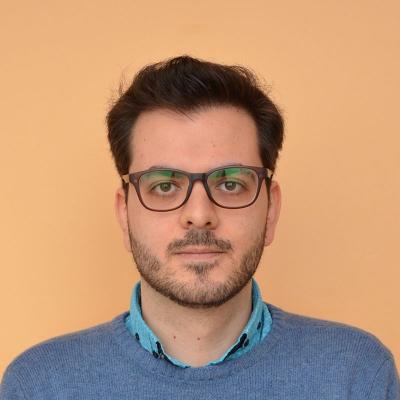
Aydin is going to present his recent research.
Additional information ...
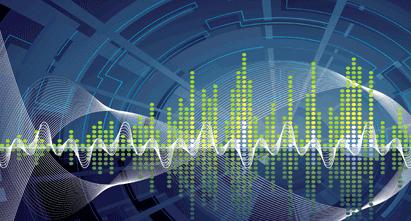
Signal Processing Seminar
- Thursday, 30 March 2017
- 13:30-14:30
- HB 17.150
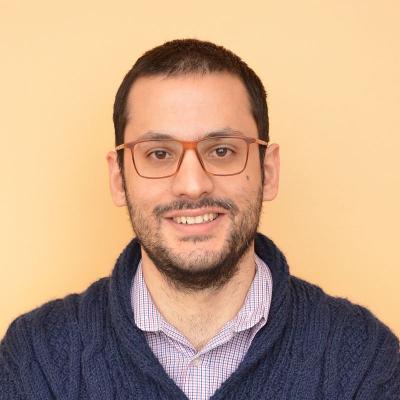
Andreas is going to present his recent research.
Additional information ...
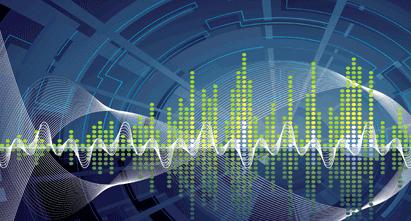
Professoren in de Arena
- Tuesday, 28 March 2017
- 20:00-21:30
- Theater de Veste
Professoren in de Arena: De bionische mens, van protheses naar upgrades
Wouter Serdijn, Just Herder, Harrie Weinans, Project March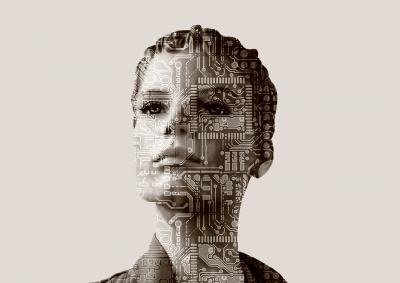
Op 28 maart gaan drie hoogleraren, waaronder Wouter Serdijn, met elkaar in debat over 'de bionische mens'. Wat is er mogelijk en hoe ver kun, wil en mag je gaan? In drie korte minicolleges praten de heren u bij en worden ze vervolgens stevig aan de tand gevoeld door cabaretier, columnist en TU-docent Jasper van Kuijk. In de discussie die daarop volgt, wordt het publiek van harte uitgenodigd mee te doen.
De sprekers van deze avond zijn:
Just Herder - Professor of Interactive Mechanisms and Mechatronics
Harrie Weinans - Professor of Tissue Biomechanics and Implants
Wouter Serdijn - Professor in Bio-Electronics
Project March
Deze editie van ‘Professoren in de Theaterarena’ wordt georganiseerd i.s.m. het ‘Explore your Brain’ evenement van de TU Delft Library in het kader van het 175 jarig bestaan van de TU Delft.
Over Professoren in de Arena
In nauwe samenwerking met de TU Delft en de universiteiten van Leiden en Rotterdam zetten wij in een theatrale setting steeds drie spraakmakende hoogleraren op het podium rondom een actueel thema. Deze onderwerpen worden van verschillende kanten belicht, vanuit de harde wetenschap en/of maatschappelijke en ethische hoek. In een magazine-achtig format met korte colleges, stand-up colums wordt u bijgepraat en doet u mee in de discussie.
Locatie: Theatercafé, Theater de Veste
Signal Processing Seminar
- Thursday, 23 March 2017
- 13:30-14:30
- HB 17.150
Complex factor analysis and extensions
Alle-Jan van der Veen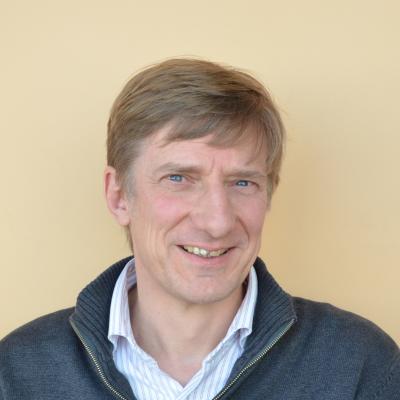
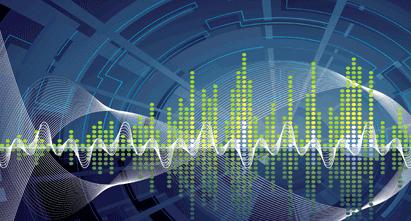
Signal Processing Seminar
- Thursday, 16 March 2017
- 13:30-14:30
- HB 17.150
Reconstruction of a Novel Wheel-Rail Contact Signal for the Wheel Defect Detection
Alireza Alemi3ME
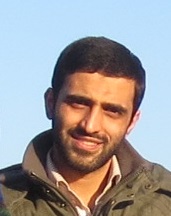
Wheel Impact Load Detectors measure the rail response to monitor the railway wheel condition. Due to the wheel dimension, the mounted sensor on the rail makes a partial observation of the wheel. Therefore, using multiple sensors is common to cover the wheel circumference. To combine the partial information collected by multiple sensors, a fusion method is required. Normally, the features of the measured data are extracted and then the feature-level fusion is applied. This research proposes a fusion method at the data-level to reconstruct a new wheel-rail contact signal. This method transfers the data from the time domain to the space domain using the space relation of the sensor intervals, and the wheel circumference. The signal reconstructed is used for the wheel defect detection.
Microelectronics Colloquium
- Wednesday, 15 March 2017
- 15:00-17:00
- EWI: Snijderszaal
Microelectronics Department Colloquium
Daniele Cavallo, Vasiliki Giagka, Fabio Sebastiano, Rob Remis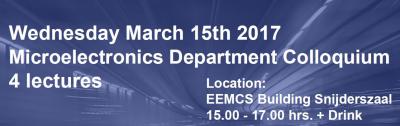
On Wednesday March 15 the next Microelectronics colloquium wil take place, including four lectures by staff members.
Please register online by completing the form.
- Vasso Giagka
Flexible bioelectronic medicines
Abstract: Bioelectronic medicines are the next generation of neuromodulation devices: small active three-dimensional neural interfaces able to modulate nerve activity by targeting a specific neural region. They aim to treat a number of conditions, such as diabetes and asthma, in a tailored (per individual) and reversible fashion, avoiding the side effects of conventional drug-based interventions (pharmaceuticals). They achieve so by recording signals from the respective nerves, extracting information and using it as feedback to electrically stimulate the neural region in a closed-loop manner.
Current technologies for active implants have not yet managed to achieve the miniaturisation and integration levels required for the development of bioelectronic medicines. For such breakthrough devices, novel concepts need to be explored, developed, and tested.
In this talk I will present my current activities as well as my vision on realizing the first flexible three-dimensional graphene active implant, for safe chronic neural stimulation and recording from the peripheral nerves.
-
Fabio Sebastiano
Cryo-CMOS for Quantum Computing: does it work?
Quantum computing holds the promise to change our lives by efficiently solving computing problems that are intractable today, such as simulation of quantum systems for synthesis of materials and drugs. A quantum computer comprises both a quantum processor and a classical electronic controller to operate and read out the quantum devices. The quantum processor must be cooled at cryogenic temperature in order to show quantum behavior, thus making it unfeasible to wire thousands of signals from the cryogenic quantum devices to a room-temperature controller.
While this issue can be solved by placing also the electronic controller at cryogen¬ic temperature, which electronic technology is the best choice for its implementation? This talk will address the challenges of building such electronic controller, and answer whether a standard CMOS technology can be employed for the required analog and digital circuits operating at 4 K and below.
-
Daniele Cavallo
Advanced Antenna Arrays for Modern Radar and Communication Systems
Abstract: Several of today’s radar and wireless communication applications are shifting their operation to higher frequency to fulfil more demanding requirements on resolution, compactness and data rates. For this reason, there is a growing need to develop low-cost integrated circuit transceivers working at millimeter and sub-millimeter waves.
However, on-chip antennas are currently characterized by very poor radiation efficiency and extremely narrow bandwidth. My approach of combining the concepts of connected arrays with artificial dielectrics will solve the inefficiency problem and enable high-efficiency on-chip antenna designs.
Similar concepts can be also realized at microwave frequencies in printed circuit board, allowing for low-cost phased array antennas with state-of-the-art performance in terms of scan range, bandwidth and polarization purity.
-
Rob Remis
Imaging with Waves
We present an overview of our current wave field imaging and inversion research. Effective inversion strategies for important applications in Magnetic Resonance Imaging (MRI), nano-optics, and subsurface monitoring will be discussed. In particular, dielectric shimming (shaping of the radio frequency field in MRI) as well as inversion algorithms that determine the dielectric properties of various tissue types based on measured MRI data will be considered, and state-of-the-art model-order reduction techniques for large-scale wave propagation problems will be discussed as well.
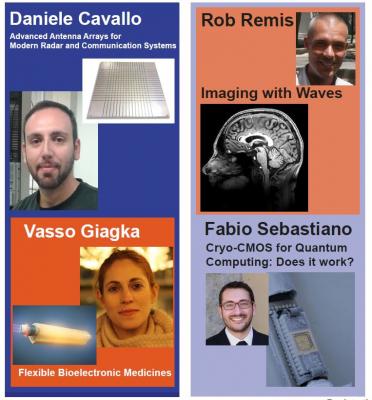
Signal Processing Seminar
- Thursday, 2 March 2017
- 13:30-14:30
- HB 17.150
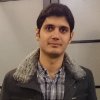
Ehsan is going to present his recent research.
Additional information ...
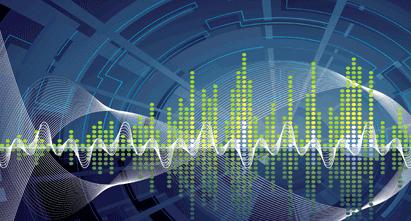
Signal Processing Seminar
- Thursday, 23 February 2017
- 13:30-14:30
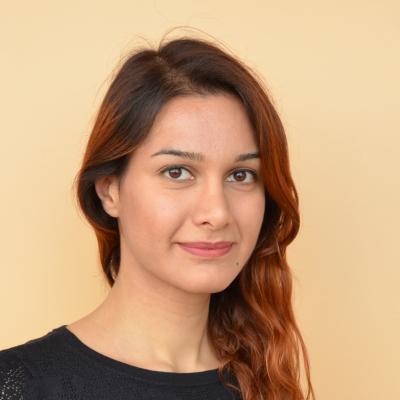
Signal Processing Seminar
- Thursday, 23 February 2017
- 13:30-14:30
- HB 17.150
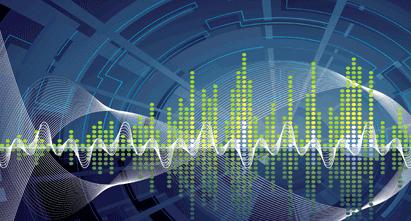
Signal Processing Seminar
- Thursday, 16 February 2017
- 13:30-14:30
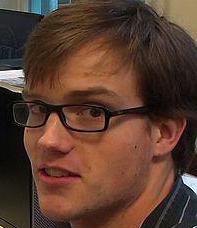
MS3 seminar
- Wednesday, 15 February 2017
- 16:00-17:00
- EEMCS, Snijderszaal
Capabilities and Research Activities at the University of Oklahoma Advanced Radar Research Center
Prof. Nathan A. GoodmanThe Advanced Radar Research Center (ARRC) at the University of Oklahoma

The Advanced Radar Research Center (ARRC) at the University of Oklahoma consists of a vibrant group of faculty and students from both engineering and meteorology, focused on solving challenging radar problems and preparing the next generation of students. Through the collaborative nature instilled in its members, the ARRC has proven effective at developing synergy between science and engineering in the field of radar. The ARRC resides in state-of-art Radar Innovations Laboratory, a one-of-a-kind and unrivalled facility for radar research, development, and education. This 35,000-sqft facility includes microwave labs, advanced fabrication capability, and two anechoic chambers.
Bio Prof. Goodman: Nathan A. Goodman received the B.S., M.S., and Ph.D. degrees in electrical engineering from the University of Kansas, Lawrence, in 1995, 1997, and 2002, respectively. From 1996 to 1998, he was an RF systems engineer for Texas Instruments, Dallas, TX., and from 2002 to 2011, he was a faculty member in the ECE Department of the University of Arizona, Tucson. He is now a Professor in the School of Electrical and Computer Engineering and Director of Research for the Advanced Radar Research Center at the University of Oklahoma, Norman.
MS3 seminar
- Monday, 13 February 2017
- 15:30-18:00
- EWI Snijderszaal
MS3 Master Event
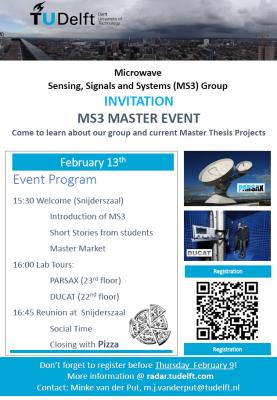
Come to learn about our group and current Master Thesis Projects...
Additional information ...
Signal Processing Seminar
- Thursday, 9 February 2017
- 13:30-14:30
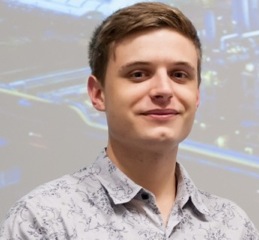
MSc SS Thesis Presentation
- Monday, 6 February 2017
- 14:00-14:45
- EWI Snijderszaal (LB 01.010)
Enhancement of the Spatial Resolution for the Temperature Sensing System of the 7 Tesla Magnetic Resonance Imaging Scanner
Tariq SaboeraliThe MRI scanner with an ultrahigh magnetic field of 7T not only increases the image resolution but it also increases the Specific Absorption Rate (SAR) of the patient. In other words, the body temperature of the patient increases due to the absorption of heat produced by the 7T MRI scanner. This is dangerous for the health of the patient. In order to ensure that the SAR level of the patient does not exceed the acceptable limit, the body temperature of the patient should be monitored during the scan with a spatial resolution as small as possible. This way safety measures can be taken immediately if the body temperature increases. In order to monitor the temperature during the MRI scan, fiber optic sensors (FOS) can be used. The fiber optic sensors (FOS) are immune from electromagnetic interference and there is no electrical connection to the patient and thus it is safe to monitor the temperature during an MRI scan by using FOS [1]. However, the FOS may have a spatial resolution which is not acceptable for medical purposes. This study focuses on methods to increase the spatial resolution of an existing fiber optic temperature sensing system of a 7T MRI scanner. To increase the spatial resolution of the existing temperature sensing system two methods are evaluated, namely the total variation deconvolution method and the blind deconvolution method. This study shows that the total variation deconvolution method gives the best results for the input temperature estimate. The blind deconvolution method strongly depends on the initial guess of the impulse response of the temperature sensing system, which is difficult to find. Therefore the results of the input temperature and the impulse response are less reliable when using the blind deconvolution method. Also it is shown that the machine resolution gets worse when increasing the spatial resolution by interpolating the input temperature in the Fourier domain.
Signal Processing Seminar
- Thursday, 26 January 2017
- 13:30-14:30
- van der Poelzaal
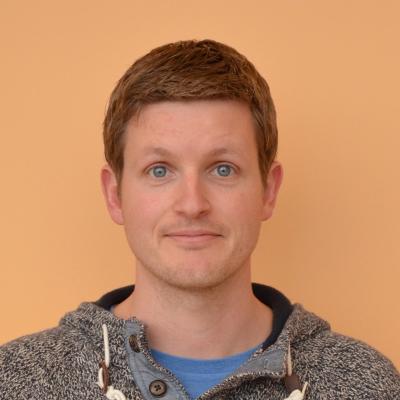
Strong magnetic background fields are of great interest in Magnetic Resonance Imaging (MRI), since images with high spatial resolution can be obtained at reduced scanning times. Strong background fields may cause RF interference effects, however, and these effects can severely degrade the quality of an MR image. This problem can be partly resolved using various advanced and mostly expensive techniques, but there is also a cheap and practical solution, namely, dielectric pads. The design of such a pad is not trivial. Normally, finding the “optimal” pad for a specific region of interest involves evaluating many different pad designs using electromagnetic field simulations. This is a very time-consuming approach taking hours to days of computation time. We propose a nonlinear optimization method based on model order reduction that allows us to design high-permittivity pads in less than 30 seconds.
Additional information ...
Signal Processing Seminar
- Thursday, 19 January 2017
- 13:30-14:30
Digital Active Noise Cancellation
Additional information ...
MS3 seminar
- Friday, 13 January 2017
- 11:00-11:45
- EWI, Snijderszaal (LB 1.010)
Dynamic rainfall monitoring using microwave links
Venkat Roy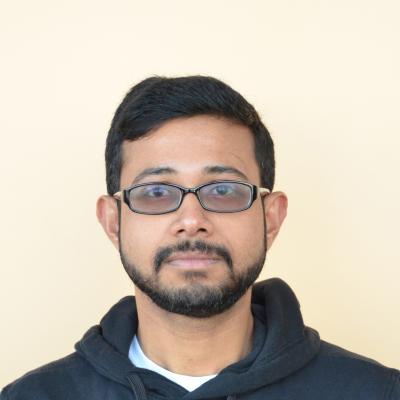
We propose a sparsity-exploiting dynamic rainfall monitoring methodology using rain-induced attenuation measurements from microwave links. To estimate the rainfall intensity dynamically from a limited number of non-linear measurements, we exploit the physical properties of rainfall such as spatial sparsity and non-negativity along with the dynamics of the rainfall. We develop a dynamic state estimation algorithm, where the aforementioned spatial properties are utilized as prior information. To exploit spatial sparsity, we use a basis function to tailor the sparse representation of the rainfall intensity. The developed methodology is applied to dynamically monitor the rainfall field intensity in an area with a specified spatial resolution using less number of simulated non-linear measurements than pixels. The proposed methodology can be generalized for any dynamic field reconstruction, where the limited number of non-linear measurements are field intensities integrated over a linear path.
Additional information ...
Signal Processing Seminar
- Thursday, 12 January 2017
- 13:30-14:30
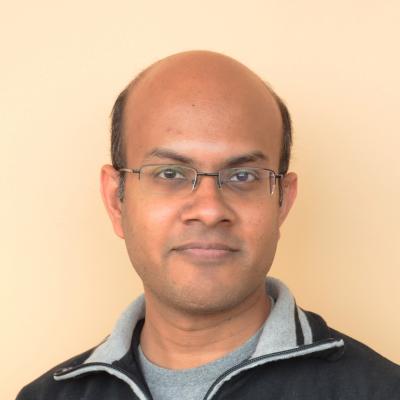
Signal Processing Seminar
- Thursday, 15 December 2016
- 10:30-11:30
- LB 01.170 (Timmanzaal)
Transmitter and Receiver Optimization for MIMO Radar Systems
Tuomas AittomakiAalto University, Finland
The spatial and waveform diversity achieved in MIMO radars can be beneficial for target detection and target parameter estimation, especially for low-observability targets. Taking advantage of the diversity requires optimization of both the transmitter and the receiver end. For the transmitter, it is necessary to encode the transmitted waveforms to have minimal sidelobe and cross-correlation levels. Also by appropriate spatial coding, the channel estimation error can be reduced. Furthermore, the transmit power allocation can be optimized. For the receivers, mismatched filters can be optimized to reduce jamming and clutter as well as the sidelobe and the cross-correlation levels for any Doppler frequency.
MSc CE Thesis Presentation
- Tuesday, 13 December 2016
- 13:30-14:15
- HB 17.150
A Real-Time Hybrid Neuron Network for Highly Parallel Cognitive Systems
G.J. Christiaanse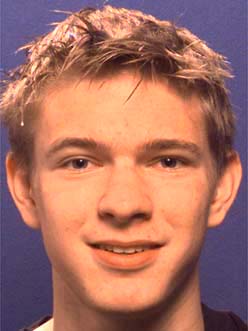
For comprehensive understanding of how neurons communicate with each other, new tools need to be developed that can accurately reproduce and mimic the behaviour of such neurons in real-time. By using current complex mathematical models, simulated neurons are able to accurately approximate the behaviour of biological neural tissue. This comes at the price of computing complexity, resulting in responses that lag behind, and thus cannot interface with biological neurons.
The proposed design in this thesis, models an Inferior Olivary Nucleus network on an FPGA device, with a maximised amount of simulated neurons for the given FPGA family type. To achieve both accuracy and real-time speed, a complex biophysically meaningful mathematical model has been analysed and scheduled on a highly pipelined, and parallel running architecture design, specified within a SystemC specification. This has contributed to the creation of hybrid neuron network that executes optimally scheduled floating-point operations that, together with open source IP, has resulted in cost-effective solutions, capable of simulating responses faster or on par with their biological counterparts.
Microelectronics Introduction Colloquium
- Monday, 12 December 2016
- Theatre - Culture Building 38, Mekelweg 10
Introduction 3 new Tenure Trackers
Masoud Babaie, Morteza Alavi, Faruk Uysal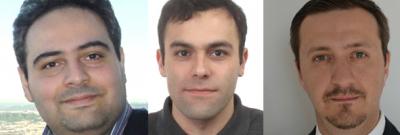
On December 12 we organize the next Microelectronics Colloquium to introduce three new Assistant Professors (Tenure Trackers) of the Microelectronics department. They are happy to present a lecture about their research.
The colloquium start at 15.00 hrs. there will be a drink afterwards in the foyer.
Location: Theatre of Culture Builing (38) Mekelweg 10.
Please
register online if you want to attend, latest December 5.
- Masoud Babaie: Pushing The Limits of CMOS Circuits for Emerging Technologies
Within the next few years, quantum processors, Fifth Generation (5G) cellular systems and the wireless Internet-of-Things (IoT) are expected to see significant deployment to realize more integration between the physical and digital worlds, promising enormous computation power, high data rate communications and enabling more objects to be remotely sensed and controlled.
This talk will address some of the main challenges in the design and implementation of IoT devices, mm-wave 5G transceivers, and cryogenic CMOS controller for quantum computers. An overview of my past and ongoing research activities will be also presented, with emphasis on novel solutions to improve power efficiency and spectral purity of RF/mm-wave transceivers.
- Morteza Alavi: Universal Transmitters for 5G
Today, our daily activities are intertwined with the Internet. The ever-growing demand to swiftly get access to the data-cloud systems leads to huge data traffic. In order to seamlessly transmit and receive these gigantic data, _ 40 GB, agile radio-frequency (RF) transceivers are inevitable.
These radios must be capable of supporting the current and future communication standards such as 5th generation of wireless mobile communications. The ultimate goal is that they can be implemented as universal radios whose modes of operation can be defined by their clients. To address these demands, RF transmitters are currently reinvented and are directed towards digital-intensive architecture. In this short presentation, we will briefly describe the strengths,possibilities, and challenges that exist for these advanced transmitters. First and foremost, the concept of RF-DAC based transmitters will be introduced. Next, the talk will review various RF-DAC based transmitters that have already been implemented at ELCA. Eventually, the presentation will concisely unveil the future directions of the research of these software-defined transmitters at ELCA.
- Faruk Uysal: Distributed Radar Networks: Beyond a single radar
The number of operational radar is rapidly increasing due to the growing demand of the remote sensing. Software defined radio and emerging single-chip radar technology make use of radars in every aspect of life such as autonomous driving, safety and security applications. With the increase of active transmitters, spectrum management and coexistence started to become a concern for some radar systems. In this talk, the previous applications of waveform, frequency agility will be reviewed to bring multi-functionality to the modern radar system. Finally, we will discuss the future research for distributed radar networks and how to fuse data from various radars to acquire different aspects of a target to be viewed simultaneously.
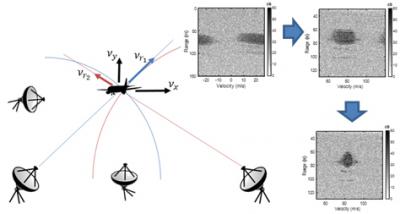
Signal Processing Seminar
- Thursday, 8 December 2016
- 13:30-13:30
- HB 17.150
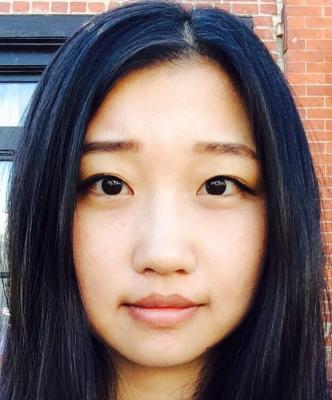
Signal Processing Seminar
- Thursday, 1 December 2016
- 13:30-14:30
- HB 17.150
Accurate ranging and localization capabilities of narrowband ISM band radios
Jac Romme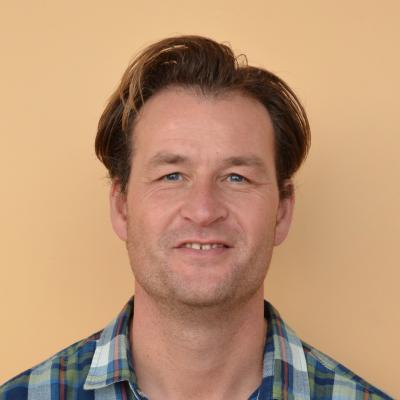
In this presentation, the results are presented of a feasibility study on the ranging and localization capabilities of narrowband radio. Starting point of the study was the phase-difference (PD) principle used by Atmel’s AVR2151 chipset. To quantify the ranging performance in indoor environment, multi-channel VNA-based channel measurements have been conducted. The analyses revealed that PD principle is sensitive to multipath, even in the presence of a line-of-sight. To improve the accuracy, two super-resolution-based ranging algorithms (using Matrix-Pencil and Music) have been evaluated, which are shown to be considerably more robust against multipath. Additionally, the channel measurements have been used to quantify the benefit of antenna/polarization diversity for ranging. The diversity gave an additional, significant improvement and resulted in ranging with 0.5 meter accuracy in combination with the super-resolution algorithms using only the 2.4 GHz ISM band.
Additional information ...
PhD Thesis Defence
- Tuesday, 22 November 2016
- 10:00-11:30
- Frans van Hasseltroom of the Aula
Gigabit Wireless Transmission in Dispersive Environments
Seyran Khademi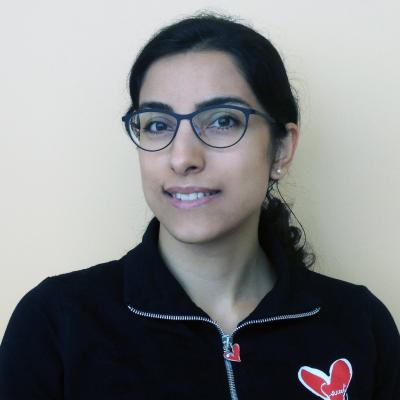
The advent of the digital era has revolutionized many aspect of our society and has significantly improved the quality of our lives. Consequently, signal processing has gained a considerable attention as the science behind the digital life. Among different applications for signal processing theory and algorithms, wireless communications remains one of the attractive and popular ones due to the widespread use of mobile devices.
This thesis is dedicated to develop signal processing algorithms to design highspeed wireless transceivers that can perform in highly reflective and harsh environments. The start of this research work initiated as a collaboration between TU Delft and an industrial partner, on a research aimed at short range gigabit wireless link within a lithography machine. The underlying unique wireless environment, together with the challenging specifications of the communication link for mechatronic systems, made this a compelling research project.
The first part of this research work focuses on constructing a reliable propagation model for dispersive environments, based on actual measurements. In our opinion it is crucial to have decent models to build effective theory and applications upon it. We developed a statistical channel model for the 60 GHz band for the extreme case of a confined metal enclosure in order to evaluate and test the existing signal processing algorithms under such pessimistic ambient conditions. This unique experiment opened up new research challenges to look back to popular design paradigms and reevaluate them with respect to the proposed channel model with a delay spread in the order of miliseconds. The concept of orthogonal frequency division multiplexing (OFDM) transmission was revisited and a customized OFDM system was designed which meets the data rate requirements of the mechatronic system of interest. The effectiveness of the proposed OFDM design was examined via Matlab simulations using the measured and modeled channels. Interestingly, the performance of the OFDM system is not heavily affected by the frequency selectivity of the extreme propagation environment. The loss is mainly due to the time guard that is dedicated to avoid interference between consecutive OFDM blocks, suggesting the use of longer OFDM blocks to minimize the bandwidth loss.
The second part of this thesis is dedicated to multiple-input multiple-output (MIMO) systems versus the single-input single-output (SISO) system which was studied in the first part. The emphasis is on general challenges in high speed (wideband) communication systems rather than the specific wireless link within a mechatronic machine. Challenging research questions are posed regarding the design and implementation of MIMO systems. This part starts with a brief introduction to such systems and redefining our system model with respect to the MIMO setting and it continues by revisiting the timely problem of peak-to-average power-ratio (PAPR) reduction in OFDMsystems, which deals with stochastic (data-dependent) OFDM waveforms, and the proposal of an effective algorithm to handle this challenge within the MIMO context . The hard problem of antenna selection for MIMO system was considered at the end by investigating different linear precoding designs subject to the realistic hardware constraints including per antenna power constraints (rather than conventional total power constraint) and limited number of RF chains.
Additional information ...
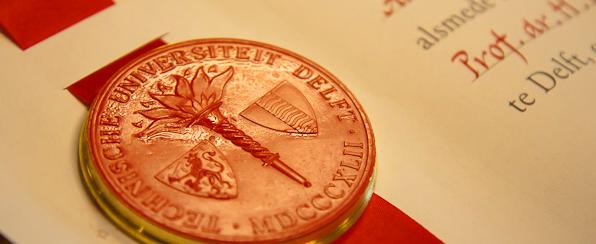
Signal Processing Seminar
- Monday, 21 November 2016
- 16:00-17:00
- HB 17.150
Bilinear Inverse Problems: Bad News and Good News
Urbashi MitraUniversity of Southern California, USA
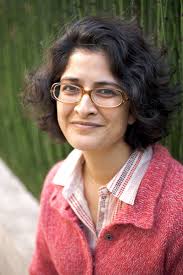
A number of important inverse problems in signal processing, such as blind deconvolution, matrix factorization, dictionary learning and blind source separation share the common characteristic of being bilinear inverse problems. In such problems, the observation model is a function of two inputs and conditioned on one input being known, the observation is a linear function of the other. We will review important applications and challenges.
A key question is that of identifiability: can one unambiguously recover the pair of inputs from the output? We shall consider both deterministic conditions for identifiability as well as probabilistic statements that result in new scaling laws under cone constraints. We provide additional results specific to blind deconvolution and show, surprisingly, that adding the sparsity structural constraint is insufficient for signal identifiability suggesting that other strategies such as coding are necessary to achieve identifiability. However, there is hope that additional structure can help in certain cases. To this end, we discuss a novel strategy that exploits low rank matrix factorization to estimate parameters of a time-varying wireless channel.
Biography
Urbashi Mitra received the B.S. and the M.S. degrees from the University of California at Berkeley and his Ph.D. from Princeton University. Dr. Mitra is currently a Dean’s Professor of Electrical Engineering at the University of Southern California.Dr. Mitra is a Fellow of the IEEE. She is the inaugural Editor-in-Chief for the IEEE Transactions on Molecular, Biological and Multi-scale Communications. She is a member of the IEEE Information Theory Society's Board of Governors (2002-2007, 2012-2017) and the IEEE Signal Processing Society’s Technical Committee on Signal Processing for Communications and Networks (2012-2016).
Her research interests are in: wireless communications, communication and sensor networks, biological communication systems, detection and estimation and the interface of communication, sensing and control.
Additional information ...
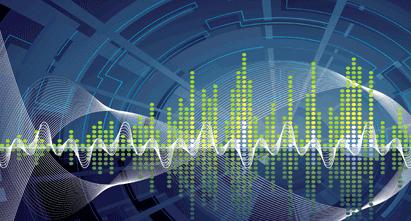
- Monday, 14 November 2016
- 15:00-15:45
- HB 17.150
Performance evaluation of a promising candidate waveform for 5G networks (GFDM)
Amirhossein Mohammadian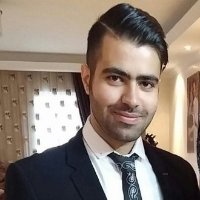
The advent of next generation wireless communication system (5G), arises new requirements that clearly go beyond higher data rates. Orthogonal Frequency Division Multiplexing (OFDM) is a widely adopted solution, but has some major drawbacks, including high out-of-band (OOB) emissions, high power consumption and Low spectral efficiency. As a result of OFDM limitation in addressing requirements of scenarios presumed for 5G, investigation of new waveforms is necessitated. Generalized Frequency Division Multiplexing (GFDM) is a flexible candidate of non-orthogonal waveform for next generation wireless networks. GFDM has attractive properties and as a result has recently received a great deal of attention.
In this presentation, after introducing the GFDM structure, I am going to discuss the following titles which are related to its performance evaluation.
- Derivation of PAPR distribution for GFDM modulated signal
- Spectral analysis of GFDM modulated signal under nonlinear behavior of power amplifier (PA)
- Rate optimization of GFDM-based cognitive radio networks
One of the most critical elements in all communication systems is PA. Nonlinear behavior of PA causes the expansions of spectral contents, called spectral regrowth. The power leakage of GFDM on the adjacent channels, caused by nonlinear PA is investigated by spectrum analyzing.
Due to the limited spectral resources in wireless communication systems, cognitive radio (CR) appears to be a promising approach. A rate optimization problem for GFDM under interference constraints is formulated and solved.
Signal Processing Seminar
- Thursday, 10 November 2016
- 13:30-14:30
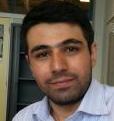
Signal Processing Seminar
- Thursday, 3 November 2016
- 13:30-14:30
- HB 17.150
Swarm Exploration under Sparsity Constraints
Christoph ManssGerman Aerospace Center Institute for Communications and Navigation (DLR)
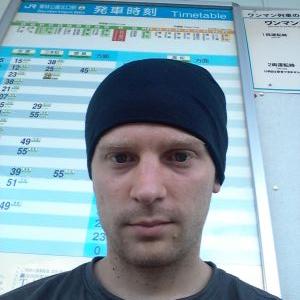
Robotic exploration aims at reconstructing (or understanding) an unknown physical process autonomously and as efficiently as possible. The Curiosity Rover on Mars exemplifies well a typical application of a robotic platform for extraterrestrial exploration. In many situations, however, the exploration domain is either vast and a full coverage of it is time consuming for one agent. Another issue is the robustness of the exploration mission, where a single robot represents a single point of failure. This is the reason why our group focuses on multi-agent exploration systems, called swarms.
The multi-agent systems coordinate their actions by cooperatively collecting measurements and jointly processing the acquired data, thus they split the computational complexity of the estimation, and risk of whole system failure, among individual agents. This talk will address a specific class of models for exploration, where the exploration area of interest is considered to be sparse. Sparsity implies that the explored process can be accurately represented with only a few relevant components. This assumption leads to optimization algorithms employing specific non-smooth regularizations. With the help of compressive sensing methods such assumptions can significantly speed up estimation and exploration. Specifically, it will be shown how compressive sensing methods are used to estimate model parameters in a multi-agent setting and how optimal agent trajectories (with respect to the efficiency of the estimation of an unknown process) are computed.
MSc SS Thesis Presentation
- Tuesday, 1 November 2016
- 13:30-14:15
- LB 01.010 Snijderszaal
Three dimensional Contrast Source Inversion-Electrical Properties Tomography (3D CSI-EPT)
Reijer Leijsen
PhD Thesis Defence
- Friday, 28 October 2016
- 10:00-11:30
- Aula Senaatszaal
Relative Space-Time Kinematics Of an Anchorless Network
Raj Thilak Rajan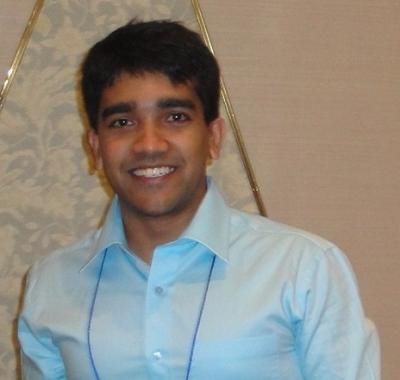
Space and time awareness has been an integral quest of human evolution, and more so in the currently burgeoning era of wireless sensor networks (WSN), internet of things (IoT) and big data. The rapid advances in technology in recent times has led to affordable, miniaturized and low-power sensor nodes, enabling the feasibility of networks with numerous nodes. These nodes are typically equipped with diverse portfolio of sensors to measure various physical phenomenon, which are cooperatively communicated and processed for appropriate statistical inference. To ensure coherent sampling, efficient communication and prudent inference, the knowledge of position and time of the sampled data is imperative, and consequently accurate space-time estimation of the nodes is as valuable as the sampled data itself.
In this dissertation we address the space-time estimation of a specific class of WSNs, namely an anchorless network of asynchronous mobile nodes. As the terminology suggests, we consider a network of mobile nodes under non-relativistic motion, whose space-time kinematics are to be estimated. In addition, the term anchorless indicates no apriori information on the absolute position or time of any node within the network. This approach is a stark contrast to conventional anchored scenarios, e.g., GPS-based localization, where absolute space-time reference is known. Anchorless networks arise naturally when deployed in inaccessible regions, where an absolute space-time reference is non-existent or only intermittently available. Moreover, when a swarm of nodes is considered, imparting the absolute reference to all the nodes could be limited by communication resources. A few application scenarios include, for example, indoor localization, underwater networks, drone swarms and space-based satellite arrays. In such anchorless networks, it is paramount to understand the relative space-time kinematics, which is the primary theme of this dissertation.
Unfortunately, our understanding of relative kinematics in Euclidean space is inherently dependent on an absolute reference. For instance, consider the first-order relative spatial kinematics, i.e., relative velocity, which is rightly defined as the vector difference between absolute velocities of the respective nodes. However, in the absence of apriori information on any absolute velocities, a natural question arises if these relative velocities can be estimated using only pairwise distance measurements between the nodes. In addition to relative spatial estimation, the asynchronous clocks on-board each of these nodes must also be synchronized, in the absence of a known absolute time-reference. These are some of the fundamental challenges which are addressed in this dissertation.
Additional information ...
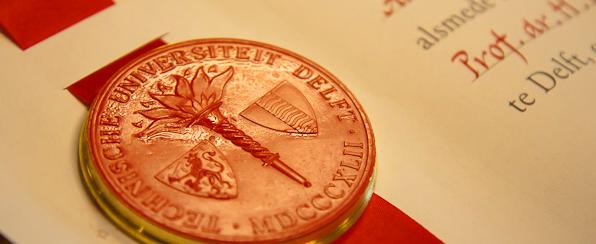
Signal Processing Seminar
- Friday, 28 October 2016
- 15:00-16:00
- HB 17.150
Three easy pieces in statistical signal processing
Amir LeshemBar-Ilan University, Israel
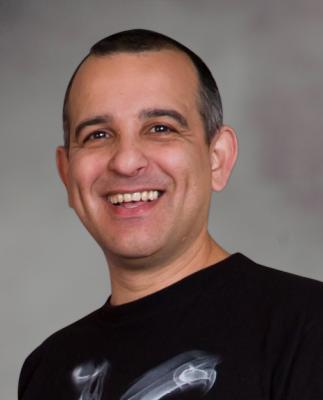
I will describe some new results from estimation theory and networks dynamics. All the results are very simple and deal with very well known concepts in statistical signal processinfg.
The first will be the non existence of unbiased estimators in many constrained estimation problems. The second will describe a novel herding phenomenon in consensus based on actions The last will be novel bounds on the performance of LS estimators with finitely many samples and non Gaussian noise.
I will assume knowledge of basic estimation theory and will describe all the necessary probabilistic tools. Interestingly in all results advanced probability and measure theory play an important role.
Additional information ...
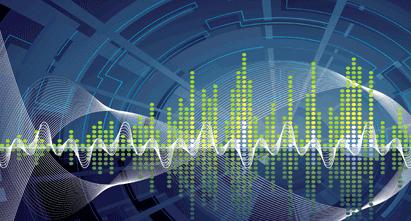
MSc CE Thesis Presentation
- Friday, 28 October 2016
- 10:30-11:15
- HB 17.150
Design and Characterization of the Time Division Multiplexing concept on a dual clock Imaging DSP
Ioanna Kyriakou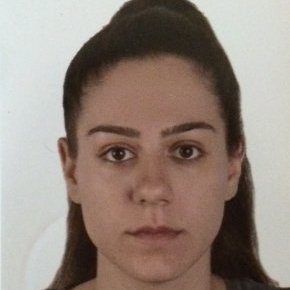
The market demand for quality imaging on portable devices has increased heavily in the last few years. This increase on the demands has a direct effect on the complexity of the imaging-dedicated devices, making the Digital Signal Processors (DSP) development a key factor in the race of imaging quality. More complex devices have been developed to cope with the technical challenges in terms of computational capability, power consumption and area footprint of the devices, especially in the portable market. This challenge is the key to the success of the imaging processor in the market, forcing companies to develop new and creative ways to reduce area and power while keeping performance.
Experimental results showed that by applying the Time Division Multiplexing (TDM) concept, as a clock domain crossing technique, in an Imaging DSP (IDSP), the area that it occupies as well as the power that it consumes can be reduced. In this thesis, four new implementations of the IDSP are proposed and their functionality was proven to be correct. All these designs were synthesized and results about area and power were extracted and analyzed.
Additional information ...
MRI based Electrical Properties Tomography: Electromagnetic inversion
- Thursday, 20 October 2016
- 13:30-14:30
- HB 17.150
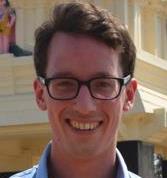
In magnetic resonance imaging (MRI) the interest in electric properties tomography (EPT) is growing. In current EPT applications the reconstruction is performed based on the Helmholtz equation which relies on the assumption of a homogeneous contrast. In this talk I will present new approaches to reconstruct the electrical properties that require less assumptions on the contrast. Two fundamentally new approaches are presented, one based on first order differentiation and one on the global integral field equations using a contrast-source variable. Reconstruction of both two- and three-dimensional simulations as well as the reconstruction of an in vivo measurement are performed to compare the five different methods. It can be concluded from this comparison that methods that are not based on the homogeneous contrast assumption perform much more accurate (overall) than the Helmholtz equation based method. These new methods provide new insight into the inversion problem in MRI, specifically for EPT and get us one step closer to accurate electric properties reconstruction from an MRI scan.
Additional information ...
DCSE and SIAM Seminar
- Tuesday, 18 October 2016
- 15:00-16:00
- LB 01.010 Snijderszaal
Finite Difference Interpretation of Reduced Order Models and Applications to Wave Propagation
Vladimir L. DruskinSchlumberger-Doll Research, Cambridge (USA)
In model order reduction, one approximates the response (transfer function) of a large scale dynamic system using a smaller system, known as reduced order model (ROM), that retains certain features of the larger problem.
For lossless wve propagation such a feature is energy conservation, that manifests in the Stieltjess-Markov property of (frequency-domain) transfer functions. In his seminal ork (1952) Mark Krein showed that the Stieltjes rational transfer functions can be equivalently presented by (Stieltjes) strings of point masses and weightless springs, i.e., described by a dynamic system with s.p.d. tri-diagonal matrix.
In turn, the Stieltjes strings give rise to interpretation of the ROMs via the second-order finite-difference approximation of the underlying PDE on judiciously chosen grids. A known application of such an approach is construction of optimal discrete perfectly matched layers (PMLs) for exterior wave problems.
In this talk I will present two more recent applications of the Stieltjes string technology. The first one is construction of so-called S-fraction multiscale reduced order model with sparse coarse cell approximation for hyperbolic problems, illustrated ith simulation examples for large scale 3D elastic anisotropic wave problems. The second application is direct nonlinear imaging algorithm via the data-driven discrete-time ROM with 2D examples from seismic exploration and ultrasonic medical imaging.
This is joint work with Alexander Mamonov, Andre Thaler and Mikhail Zaslavsky.
Biography
Vladimir L. Druskin is Scientific Advisor at Schlumberger-Doll Research and a SIAM Fellow. Throughout his career, Vladimir Druskin made fundamental contributions to inverse problems, scientific computing, and numerical analysis and their application to hydrocarbon exploration.
MSc SS Thesis Presentation
- Thursday, 13 October 2016
- 10:00-10:45
- EWI HB09.150 Dijkstrazaal
Accelerating Diffusion-Weighted Chemical Shift Imaging using Compressed Sensing with Parameter Mapping
Joost van der Kemp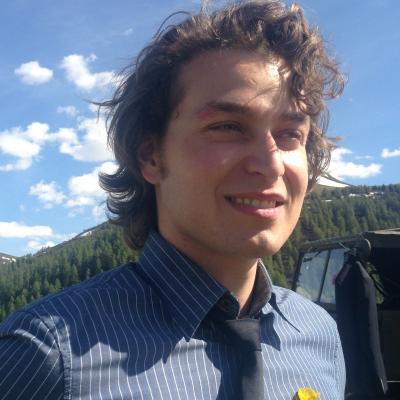
Diffusion-weighted chemical shift imaging (DW-CSI) is a recently developed MRI modality that enables radiologists to reveal the diffusion properties of small molecules that act in metabolic reactions in-vivo. In order to extract this diffusion information from a patient, DW-CSI requires approximately one hour of scan time. This extensive scan time makes DW-CSI currently inapplicable for the clinical setting. This thesis describes the closely intertwined implementation of compressed sensing with parameter mapping (CS-PM) in the DW-CSI processing pipeline to accelerate its acquisition. The CS-PM algorithm enables DW-CSI to acquire less measurements (sample under Nyquist) and subsequently reconstruct the missing samples with the use of a custom designed, model-based sparsifying dictionary. As proof of concept, CS-PM was evaluated on the water signal of a non-water-suppressed scan. The results of the integration of CS-PM in the DW-CSI processing pipeline already indicate a feasible acceleration factor of 1.5 along with valuable insight to further improve the performance of DW-CSI in combination with CS-PM.
Additional information ...
Comparisson of State-of-the-Art Consensus Algorithms
- Thursday, 13 October 2016
- 13:30-14:30
- HB 17.150
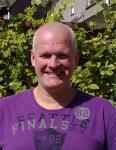
Abstract: Unlike the Telephone network or the Internet, many of the next generation networks are not engineered for the purpose of providing efficient communication between various networked entities. Examples of such networks are sensor networks, peer-to-peer networks, mobile networks of vehicles and social networks. These networks lack infrastructure; they exhibit unpredictable dynamics and they face stringent resource constraints. Therefore, algorithms operating within them need to be extremely simple, distributed, robust against networks dynamics, and efficient in resource utilization. Distributed consensus algorithms are built upon a gossip or rumor style unreliable, asynchronous information exchange protocol. Due to their immense simplicity and wide applicability, this class of algorithms is particularly suitable for the next generation networks. In this talk we will give an overview of state-of-the art consensus algorithms and compare their performance.
Slides
Here are the slides.Additional information ...
Signal Processing Seminar
- Thursday, 6 October 2016
- 13:30-14:30
- HB 17.150
An Ideal-Theoretic Criterion for Localization of an Unknown Number of Sources
Matthew Morency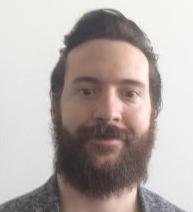
Source localization is among the most fundamental problems in statistical signal processing. Methods which rely on the orthogonality of the signal and noise subspaces, such as Pisarenko's method, MUSIC, and root-MUSIC are some of the most widely used algorithms to solve this problem. As a common feature, these methods require both a-priori knowledge of the number of sources, and an estimate of the noise subspace. In this paper, we propose a new localization criterion based on the algebraic structure of the noise subspace. An algorithm is proposed which adaptively learns the number of sources and estimates their locations.
Additional information ...
- Thursday, 29 September 2016
- 13:30-14:30
- HB 17.150
Optimizing Speech Intelligibility in Noisy Environments Using a Simple Model of Communication
Richard Hendriks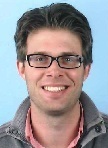
Modern communication technology allows a user to communicate from almost anywhere to almost anywhere. However, interfering sources in the environment of the talker at the far-end and the listener at the near-end often affect the ability of the parties to communicate. Conventional systems for noise reduction and speech intelligibility enhancement typically treat the processing at the far-end and the near-end as two independent problems. To quantify and optimize the intelligibility of noisy speech, we recently introduced a speech intelligibility model based on mutual information. This model takes the noise inherent in the speech production process into account at a fixed SNR (i.e., scaling independent). Such a constant production SNR has a significant effect on a power constrained communication system. That is, the usefulness of a particular communication channel ?saturates? near the production SNR. The resulting intelligibility predictor resembles heuristically derived classical measures of intelligibility such as the AI and the SII. In this presentation we will review our proposed mutual information-based speech intelligibility model and demonstrate its potential. We will relate it to classical measures of intelligibility and will show its potential for speech intelligibility prediction. Furthermore, we will show that this model can be used to derive a multi-microphone processor that is jointly optimal with respect to interfering sources at the far-end as well as at the near-end.
Additional information ...
MSc SS Thesis Presentation
- Monday, 19 September 2016
- 13:30-14:30
- HB 17.150
Informed phase restoration of amplitude spectra for audio signals
Iakov Chalegoua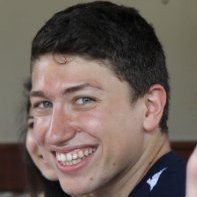
In transform coding for audio, is it sufficient to store only the magnitude information?
Additional information ...
- Monday, 19 September 2016
- 15:00-17:30
- Aula, committee room A3
Special Celebrative Seminar: New model of Sino-Dutch R&D cooperation
Since the establishment of TU Delft's Beijing Research Centre (BRC) in 2012, 10 PhD researchers have been enrolled for this unique program in close cooperation with our Chinese Academic Partners. We are very pleased that the first two BRC PhD candidates will have their PhD thesis defence on September 19 2016, in the Aula of Delft University of Technology.
To celebrate this important milestone, we would like to invite you to join a special seminar after the defences, about the New model of Sino-Dutch R&D cooperation, to share the experiences, look to the future and raise the glass together.
Additional information ...
- Thursday, 15 September 2016
- 14:00-14:45
- EWI lecture hall G
Multivariate signal processing for "big data" sensing
Gabri?l VasileGIPSA lab, Grenoble
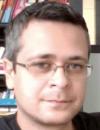
Big data?sensing brings a strong impact on many sensor-oriented application fields, such?as disaster control and monitor, healthcare services, and environment?protection. This presentation aims at providing an?overview on several application-driven?signal processing schemes, which have been carried out?in the field of environment protection at the?Grenoble-Image-sPeech-Signal-Automatics?Lab.
MSc SS Thesis Presentation
- Wednesday, 14 September 2016
- 12:00-12:45
- HB17.150
Ultrasound Imaging Using a Single Element Transducer
Andrejs Fedjajes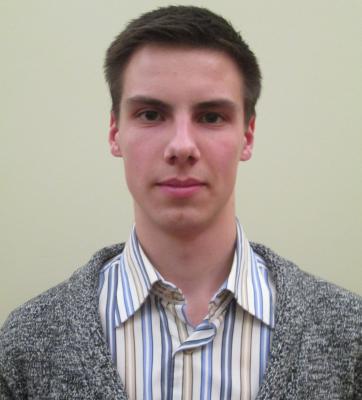
Most of nowadays ultrasound systems visualize 3D space in 2D images. State of the art matrix transducers are very expensive and have not achieved the same popularity yet. We prove the possibility to image a 3D volume with a single element transducer. The core idea is to deliberately change the transducer?s spatial field and collect the knowledge about that change into a system matrix A. This is done by means of a static aberration mask and a calibration procedure. Opposite to the conventional beamforming, we formulate the imaging task as a least squares inverse problem. This comes at the price of computational resources needed due to the problem dimensionality. The project potentially can lead to low-cost ultrasound imagers as a part of growing industry of body area sensors.
MSc SS Thesis Presentation
- Wednesday, 14 September 2016
- 15:00-15:45
- HB17.150
Regularized Least Squares Imaging for High Resolution Ultrasound
Pim van der Meulen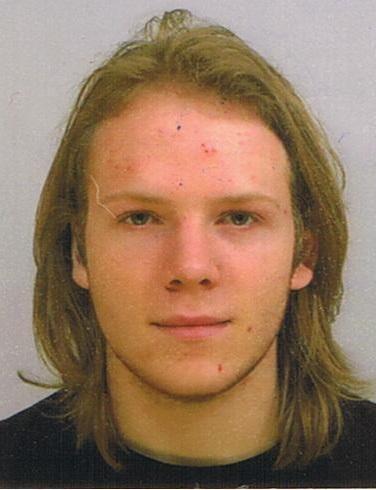
Conventional ultrasound imaging by delay-and-sum beamforming is based on geometrical operations on the ultrasound measurements. We think that it makes more sense to try and estimate the scattering composition that best explains the measurement. We have investigated this approach by formulating a linear mathematical model, which allows for a large and flexible variety of techniques to estimate the image, which have been well established and investigated in mathematics. Such a formulation also makes it easier to incorporate prior information about an experiment, such as the sparsity of an image, or its statistical properties. In our work, we have focused on using these techniques to attain high-resolution images, obtained by finding the image that minimizes the squared error between the formulated model and the measurement (i.e. tries to 'explain' the measurement), which vastly improves the common delay-and-sum technique. In the coming presentation some of these exciting results will be shown.
MSc SS Thesis Presentation
- Friday, 26 August 2016
- 14:00-14:45
- HB 17.150
Identification of room boundaries for sound field estimation
Mario Coutino Minguez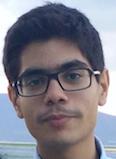
Echoes generated by the sound reflected off the walls of a room carry information about the geometry of the enclosure. Capitalization of this acoustic property could lead to improvements in current state-of- the-art methods for sound field estimation, where prior information can be used to improve the conditioning of the problem.
In this thesis, robust and computational efficient methods are developed for identifying first order reflections to estimate the room geometry using small microphone arrays.
Furthermore, as the estimation of such reflections becomes even more challenging in actual audio reproduction systems, this work aims to develop methods capable to deal with complications that might arise due to the employed drivers. This is done by considering the estimation problem in two different scenarios. Firstly, the first order reflections estimation problem is posed as a sorting problem. For this case, a set of echoes, received at different microphones, must be grouped accordingly to the wall which originated them. This problem is solved by using a greedy subspace-based algorithm. The proposed approach provides similar performance compared with the state-of-the-art method at a reduced computational cost. For the second scenario, instead of echoes, only raw microphones measurements are available. This instance of the problem is posed under an estimation theory framework, and solved by sequential minimization of a non-linear cost function based on the propagation of waves.
Experimental results, evaluated in simulated shoe-box shaped rooms, demonstrate the performance and applicability of the proposed methods for room geometry estimation.
MSc SS Thesis Presentation
- Friday, 29 July 2016
- 10:45-11:15
Dielectric Shimming
Michiel Gerlach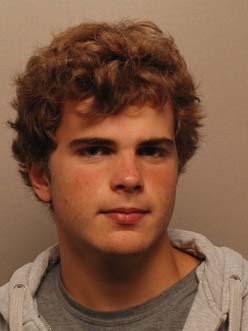
Dielectric shimming is proven to be very useful in increasing the homogeneity of the B1+ field in high field MRI. Current optimization and design techniques for dielectric pad parameters are slow. The goal of this thesis is to find a fast and accurate pad design and optimization technique. Two new techniques are proposed. The first, a method that simply uses inspection by solving the forward problem in a relatively fast way. The other proposed technique follows a more analytical approach to find the optimal permittivity and conductivity of a pad in a couple of iterative steps with a Gauss-Newton method. This last technique uses a new proposed approach to predict the phase of the B1+ field in a direct fashion.
These techniques provide fast and accurate simulation results for a two-dimensional abdominal body slice placed in a 3T MRI scanner for different pad scenarios. From these results it can be concluded that both proposed techniques generate comparable pads, which are able to increase the homogeneity of the B1+ field.
A comparison between the two techniques is made. The Gauss-Newton method provides a fast, robust and accurate optimization technique for large scale problems, but offers less flexibility and insight to the data compared to the method via inspection.
The flexibility of the method via inspection and the insight it provides is shown for different scenarios (pad location, multiple pads, pad shape, pad thickness), where the effect of the optimal permittivity and conductivity on the homogeneity of the resulting B1+ field is simulated. Even the maximum allowed SAR can be incorporated in this pad optimization technique.
- Wednesday, 6 July 2016
- 13:00-13:45
- EWI HB 17.150
Multiple user Access Technology in Optical OFDM-PON Upstream Transmission
Han DunAs bandwidth-hunger multimedia applications continue to fuel the rise in bandwidth demand in the multiple users access system, thus a PONs (passive optical networks)-based solution, which could provide high transmission capacity flexibly and cost-effectively, will be the core of the next-generation optical access network. Recently, Orthogonal Frequency Division Multiple (OFDM)-based PON has been highlighted as an attractive method to realize the next generation optical network due to its desirable feature such as higher spectral efficiency and resilience to linear dispersion.
This presentation will mainly focus on key technology on multi-user access OFDM (A)-PON specifically for the upstream transmission. Each ONU (optical network unit) shares a same ranging subcarrier, and the Gold sequences would be modulated in this subcarrier as synchronization codes (User ID). With Gold sequences modulated in a single carrier we could estimate the offsets both in time and frequency domain such as the transmission delay, different sampling clocks and optical wavelength drift. Each ONU would correct the time and frequency offsets with the estimated information, and keep the orthogonality between each subcarrier.
- Tuesday, 5 July 2016
- 11:00-11:45
- EWI LB01.220 (van der Poelzaal)
Energy harvesting wireless networks: A new frontier for communication and information theory
Aylin YenerPennsylvania State University

Wireless communication networks composed of devices that can harvest energy from nature will lead to the green future of wireless, as energy harvesting offers the possibility of perpetual network operation without adverse effects on the environment. By developing effective and robust communication techniques to be used under energy harvesting conditions, some of the communication devices in a heterogeneous network can even be taken off the grid. Energy harvesting brings new considerations to system level design of wireless communication networks, leading to new insights. These include randomness and intermittency of available energy, as well as additional system issues to be concerned about such as energy storage capacity and processing complexity. The goal of this talk is to furnish the audience with fundamental design principles of energy harvesting wireless communication networks which is an emerging area. The focus will be on identifying optimum transmission scheduling policies in various settings, and the ensuing algorithmic solutions. Time permitting we will also go into the information theory of energy harvesting communications, which brings in new challenges taking into account energy availability and storage at the channel use level.
Biography
Aylin Yener is a professor of Electrical Engineering at The Pennsylvania State University, University Park, PA since 2010, where she joined the faculty as an assistant professor in 2002. During the academic year 2008-2009, she was a Visiting Associate Professor with the Department of Electrical Engineering, Stanford University, CA. Her research interests are in information theory, communication theory and network science with recent emphasis on green communications, information security and networked systems.She received the NSF CAREER award in 2003, the best paper award in Communication Theory in the IEEE International Conference on Communications in 2010, the Penn State Engineering Alumni Society (PSEAS) Outstanding Research Award in 2010, the IEEE Marconi Prize paper award in 2014, the PSEAS Premier Research Award in 2014, and the Leonard A. Doggett Award for Outstanding Writing in Electrical Engineering at Penn State in 2014. She is a fellow of the IEEE. Dr. Yener is an elected member of the board of governors of the IEEE Information Theory Society for the term 2015-2017.
Additional information ...
MSc TC Thesis Presentation
- Monday, 4 July 2016
- 13:00-13:40
- EWI HB 17.150
Chinese Digital Radio Receiver Design and Development with FM Interference Cancellation
Yiling Zhang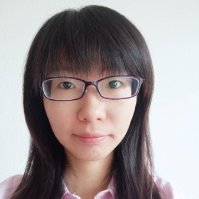
A Chinese Digital Radio (CDR) has been made and become effective in 2013 by SGAPPRFT (State General Administration of Press, Publication, Radio, Film and Television), which is an In-Band On-Channel (IBOC) digital audio broadcasting hybrided with analog FM signal in one FM channel. This project investigates the interferences to the CDR digital signals caused by both co-channel and adjacent-channel FM signals, and proposes solutions to combat such influences. A new acquisition algorithm is implemented to realize mode detection and synchronization for the hybrid signal. Two FM interference removal methods have been proposed and studied in simulation. An energy detector is introduced to select the best FM interference removal method in run time. The proposed solution has reached very good reception performance against co-channel FM interference in commonly used CDR modes. For the adjacent-channel FM interference, if we can detect and select the better removal method in real time, the performance degradation can be controlled to[LINK] a limited SNR increase under the AWGN channel.
Signal Processing Seminar
- Thursday, 30 June 2016
- 13:30-14:30
- LB01.220 (Van der Poelzaal)
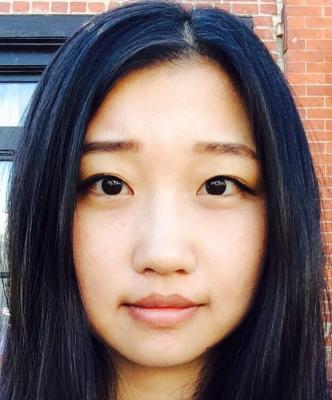
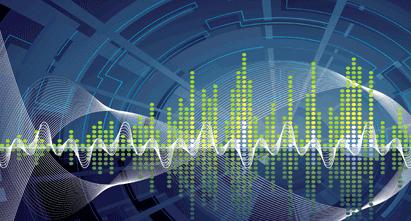
- Thursday, 30 June 2016
- 10:30-11:30
- EWI HB 03.240 (van Katwijkzaal)
Physical Foundations Underlying Green Information and Communication Technologies
Earl McCune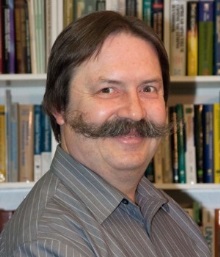
There are physical limitations on how much energy efficiency can be realized from any actual hardware used to implement any communications standard.? Experience shows that in most instances the signals adopted by the standard committee place an additional ceiling on the achievable energy efficiency using that hardware.? For example, there is hardware that is capable of providing more than 60% energy efficiency under ideal conditions, but for some standardized signals the maximum achievable efficiency drops to 7%.? This drop in achievable efficiency is predictable, and such analyses should become part of standards committee deliberations.? Such a low operating efficiency is not compatible with IoT, 5G, and other upcoming Standards objectives.
This presentation was originally given to the IEEE Green-ICT Initiative Steering Committee at the IEEE Board meeting series in New Jersey on June 16, 2016.? It establishes the reasons why such efficiency ceilings occur and shows how to predict them.? Further, recipes are provided on how it is physically possible to simultaneously achieve high bandwidth efficiency and optimum energy efficiency ? along with the PSD impacts that come with these more Green-optimized signal modulations.
Additional information ...
MSc SS Thesis Presentation
- Tuesday, 28 June 2016
- 10:45-11:15
- EWI Lecture Room Chip
Electrical properties tomography for MRI
Patrick Fuchs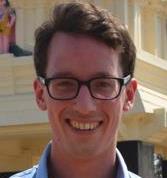
In Magnetic resonance imaging (MRI) an interest in electrical properties tomography (EPT) is growing. In current EPT the reconstruction is performed based on the Helmholtz equation which relies on the assumption of a homogeneous contrast. The goal of this thesis is to present new approaches to reconstruct the electrical properties that require less assumptions on the contrast. Two new approaches are presented, one based on first order differentiation and one on the global integral field equations using a contrast-source variable. In this thesis these methods are described alongside the existing Helmholz based approach, the contrast source inversion - EPT approach and the deconvolution approach. These last three approaches have already been published on, but are reviewed here for completeness.
Reconstruction of both two dimensional and three dimensional simulations as well as the reconstruction of an in-situ measurement are performed to compare the five different methods. It can be concluded from this comparison that all methods that are not based on the homogeneous contrast assumption perform much more accurate (overall) than the Helmholtz equation based method. Both contrast source inversion and the direct inversion method based on the global integral equations perform comparable, but the latter is a lot faster and offers almost the same range of flexibility regarding regularisation and preconditioning. The direct inversion method is a straight improvement on the deconvolution method, performing equally well regarding noise robustness, but offering better reconstructions in almost all cases due to the lack of apodisation step. The first order differential method provides a surprisingly robust, accurate and extremely fast way to get insight into the data, and shows that the inversion problem in MRI is actually very well behaved as far as inversion problems go. These new methods provide fresh insight into the inversion problem in MRI, specifically for EPT and get us one step closer to accurate electric properties reconstruction from an MRI scan.
Additional information ...
Signal Processing Seminar
- Thursday, 23 June 2016
- 13:30-14:30
- LB01.150 (Lipkenszaal)
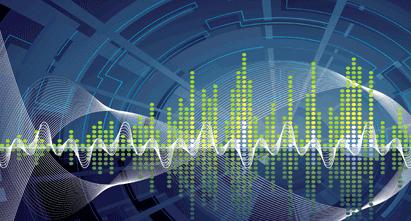
MSc SS Thesis Presentation
- Thursday, 23 June 2016
- 11:00-12:00
- LB 1.150 Lipkenszaal
Acoustic Vector Sensor Based Source Localization
Krishnaprasad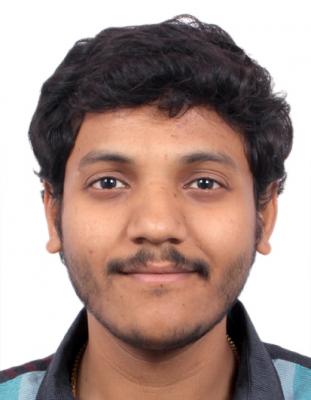
MSc work done at Microflown
Additional information ...
Signal Processing Seminar
- Thursday, 16 June 2016
- 13:30-14:30
- HB 17.150
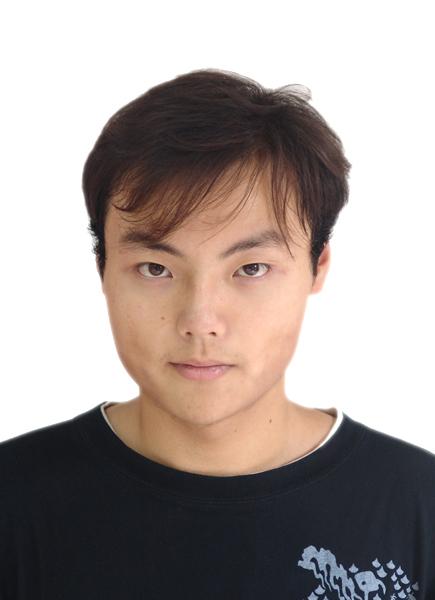
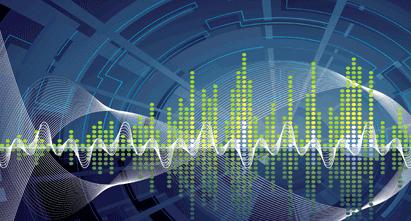
PhD Thesis Defence
- Wednesday, 15 June 2016
- 15:00-17:30
- Aula Senaatszaal
Underwater Acoustic Localization and Packet Scheduling
Hamid Ramezani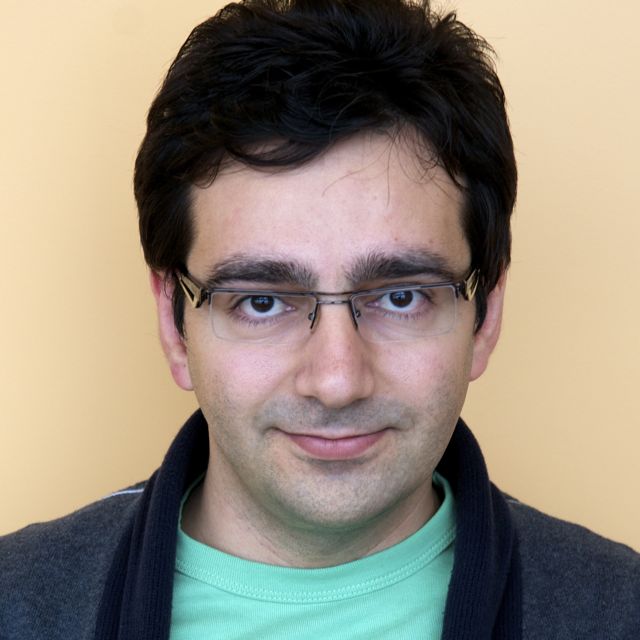
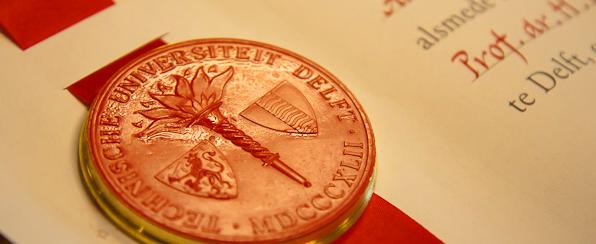
Computational neuroscience seminar
- Wednesday, 15 June 2016
- 10:00-11:00
- HB 17.150
Short presentations with update of MSc research projects status.
Additional information ...
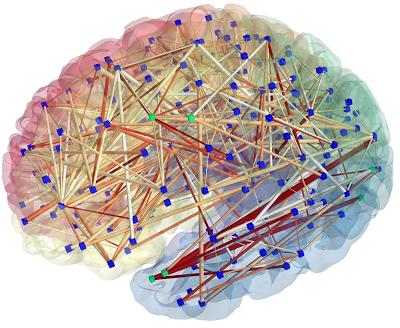
Signal Processing Seminar
- Tuesday, 14 June 2016
- 14:00-15:00
- HB 17.150
Fundamentals of Graph Signal Processing and Applications to Diffusion Processes
Prof. Antonio G. MarquesKing Juan Carlos University, Madrid
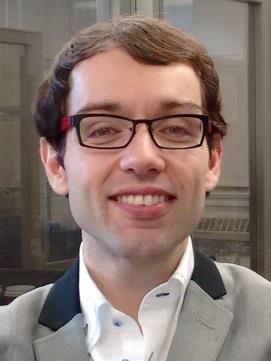
Coping with the challenges found at the intersection of Network Science and Big Data necessitates broadening the scope beyond classical temporal signal analysis and processing in order to also accommodate signals defined on graphs. Under the assumption that the signal properties are related to the topology of the graph where they are supported, the goal of graph signal processing (GSP) is to develop algorithms that fruitfully leverage this relational structure. Instrumental to that end is the so-termed graph-shift operator, a matrix capturing the graphs local topology and whose spectral decomposition is central to defining graph Fourier transforms.
In the last years a body of works has successfully implemented this approach, showing how several classical signal processing results can be gracefully generalized to the more irregular graph domain.
The talk consists of two parts. The first part introduces the field of GSP, motivates its usefulness via meaningful applications, and presents its foundational concepts, which have been derived over the past five years. The second part focuses on contemporary results, including optimal filter design, blind identification and network topology inference. For each of these results, the theoretical contribution will be first described and then the implications for distributed and dynamic processing will be discussed.
Biography
Antonio G. Marques received the Telecommunications Engineering degree and the Doctorate degree, both with highest honors, from the Carlos III University of Madrid, Spain, in 2003 and 2007, respectively. In 2007, he became a faculty of the Department of Signal Theory and Communications, King Juan Carlos University, Madrid, Spain, where he currently develops his research and teaching activities as an Associate Professor. From 2005 to 2015, he held different visiting positions at the University of Minnesota, Minneapolis. In 2015 and 2016 he was a Visiting Scholar at the University of Pennsylvania. His research interests lie in the areas of communication theory, signal processing, and networking. His current research focuses on stochastic resource allocation wireless networks and smart grids, nonlinear network optimization, and signal processing for graphs. Dr. Marques has served the IEEE and the EURASIP in a number of posts (currently, he is an Associate Editor of the IEEE Signal Process. Letters and of the EURASIP J. on Advances in Signal Process.), and his work has been awarded in several conferences and workshops.
Signal Processing Seminar
- Thursday, 9 June 2016
- 13:30-14:30
- HB 17.150
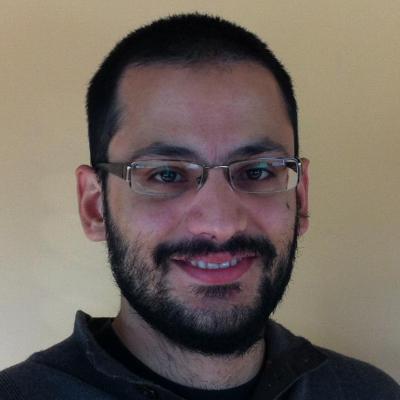
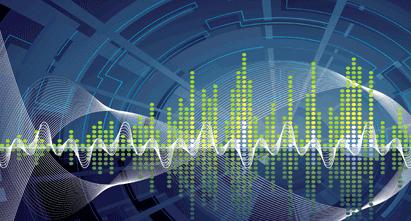
MSc Thesis Presentation
- Tuesday, 7 June 2016
- 10:00-10:40
- EWI lecture hall Data
Quantization effects in the primal-dual method of multipliers (PDMM)
Daan Schellekens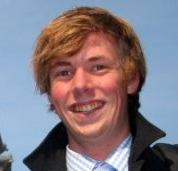
Nowadays, large-scale networks of computing units, often characterized by the absence of central control, have become more commonplace in many applications. To facilitate data processing in these large-scale networks distributed signal processing is required. The iterative behaviour of distributed processing algorithms combined with the limited energy, computational power, and bandwidth, limitations imposed by such networks, place tight constraints on the transmission capacities of the individual nodes. For this reason quantization in distributed algorithms has become an interesting and popular topic.
Already considerable research has been performed into quantization effects for various distributed algorithms, such as alternating-direction method of multipliers (ADMM). However, for the primal-dual method of multipliers (PDMM), a recently proposed promising distributed algorithm, no research into the effects of quantization exists.
In this thesis the effects of subtractively dithered uniform quantization in PDMM are investigated for the synchronous distributed averaging problem. This specific averaging problem, which is often considered as the canonical distributed problem, was selected to start the research from a natural point. As such, the theory developed in this thesis can form a foundation for further research into quantization effects in PDMM in general.
The quantization effects are discussed by considering the convergence rate of the algorithm. This is done by deriving expressions for the mean squared error (MSE) that include quantization noise. Also the required bitrate for quantized PDMM is considered. It was concluded that for practical applications quantization in PDMM can be applied with a near-fixed-rate quantizer, such that significant bitrate reduction can be achieved, without compromising the rate of convergence.
Additional information ...
PhD Thesis Defense
- Monday, 6 June 2016
- 12:00-14:30
- Aula Senaatszaal
Covariance matching techniques for radio astronomy calibration and imaging
Millad Sardarabadi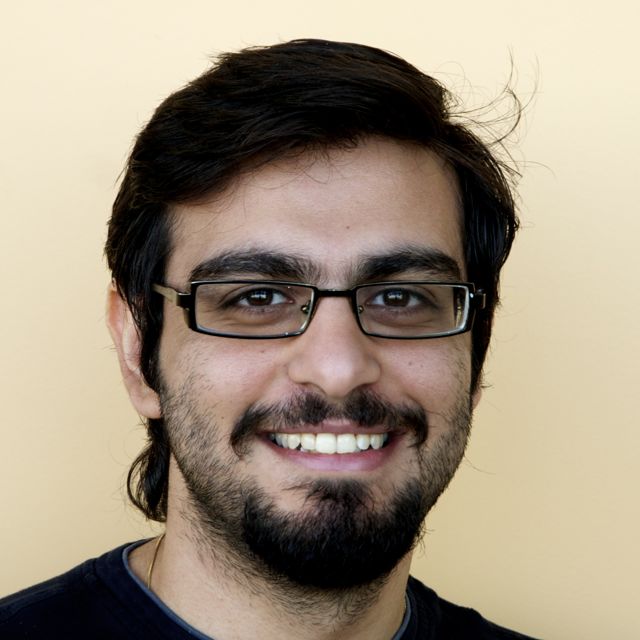
The search for the answer to one of the most fundamental scientific questions, ?How was the universe formed??, requires us to study very weak radio signals from the early universe. In the last eighty years, radio astronomers have been able to use radio frequency observations for significant discoveries such as quasars, supermassive Black Holes and the Cosmic Microwave Background radiation. Radio astronomers use a radio telescope to study the cosmos. A radio telescope usually consists of an array of radio receivers (antennas) and supporting hardware/software to produce synthesized images of the sky. While the earlier generation of the radio telescopes such as the Westerbork Synthesis Radio Telescope (WSRT), the Very Large Array (VLA) and the Giant Meter?wave Radio Telescope (GMRT) consisted of 14-45 receivers separated a few kilometers (3-25 km basedlines), the next generation of radio telescopes such as LOFAR and SKA have thousands of receivers which cover distances of over 1000 km. This massive increase in the number of receivers and the geometric dimensions is a consequence of the required (high) resolution and sensitivity for modern scientific studies and while it is necessary, it does not guarantee the desired results without the appropriate data and signal processing.
The main challenges in radio astronomy can be divided in three closely related problems: mitigation of man?made radio frequency interference, calibration and image formation. The main goal of this thesis is to investigate howthe signal processing formalism can be used to systematically model and analyze these three problems and what signal processing tools are needed for addressing them.
The number of RFI free bands is diminishing rapidly as a consequence of the increased number of wireless services and applications. The shift towardswideband digital systems has created new problems which are not sufficiently addressed by currently implemented RFI detection and mitigation systems. For this class of continuously present wide?band RFI, the use of array processing techniques such as spatial filtering could provide access to frequency bands otherwise unusable by astronomers. Such a spatial filtering can be achieved by estimating and removing the subspace that the interfering signal is occupying. Many signal processing algorithms use the eigenvalue decomposition (EVD) for estimating the signal subspace. However the use of EVD is limited to systems where the noise is white or known from calibration. This requirement is a limiting factor for applying these techniques to uncalibrated arrays with unknown noise models. In these situations a more generic approaches which allows for combined RFI filtering and noise power calibration is preferred. In this thesis factor analysis (FA) is proposed as suitable substitution for EVD.
FA is a technique that allows for the decomposition of the signal into a low?rank part corresponding to the signal and a diagonal part which represents the covariance of the noise on the receivers. Because the diagonal elements can be different this technique can be used when the noise is not white and forms a generalization of the EVD. In RFI mitigation applications the signal part of the data is dominated by RFI and changes more rapidly than the noise. Estimating the noise covariance which is shared by several measurements jointly allows for a more accurate estimation. As a result extensions to the classical FA are proposed to improve the estimates for the diagonal part of the decomposition in a joint fashion. Even a diagonal noise structure can be limiting in some applications. For example the contribution of theMilkyWay affects the short baselines which can be modeled by using a non?diagonal covariance matrix. The FA model can be extended for this type of signals. An extension to FA called Extended FA (EFA) is used to allow for capturing such structures into the model. Similar to JFA we can also estimate the parameters in EFA jointly, and the resulting method is denoted by Joint EFA (JEFA). Using nonlinear optimization techniques combined with Krylov subspace based solvers an scalable algorithm is developed. The statistical efficiency of this algorithm is shown by comparing its results to the Cram?r?Rao bound and its application in RFI mitigation has been demonstrated on measurements from the WSRT and LOFAR.
Antenna gain calibration is an essential step in producing accurate images. Using common array processing data models, the gain calibration is formulated as a nonlinear covariance matching problem. In this thesiswe showthat the matrices involve in this estimation problem are highly structured and that the systemof equations involving these matrices can be efficiently solved using Krylov subspace based solvers (similar to JEFA). The resulting calibration algorithm is scalable and requires a low number of iterations in order to converge which makes it an attractive alternative to currently available techniques.
Both classical and parametric based image formations consist of two steps. First a ?dirty? image is constructed from the measurements and then an improved estimate is found by performing a deconvolution step. When the number of pixels on the image becomes large, the deconvolution step becomes an ill?posed problem. In this thesis we showthat image values are bounded frombelowby a nonnegativity constraint and above by the dirty image. Using beamforming techniques, we show that tighter upper bounds can be constructed using the MVDR beamformer. These bounds allow us to regularize the deconvolution problem by a set of inequality constraints. Following a signal processing model, the image formation is then formulated as a parameter estimation problem with inequality constraints. This optimization problem can be solved using an active set algorithm. We show that, with the right initialization, the active set steps are very similar to sequential source removing techniques such as CLEAN. This connection between classical approaches and parametric imaging techniques provides the necessary theoretical basis for further analysis and allows for improving both methods.
Based on the results presented in this thesis we can conclude that signal processing methodologies can provide new solutions to the radio astronomical problems and also shed light on the inner working of the classical techniques. Hence, a signal processing approach is extremely beneficial in tackling the problems that the next generation of radio telescopes will face.
Additional information ...
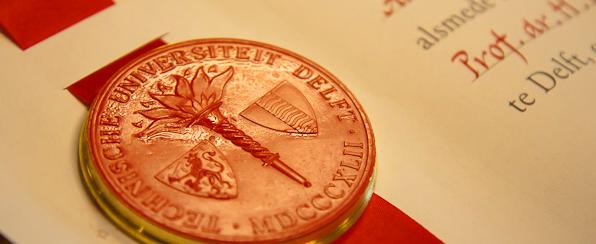
SP Seminar
- Monday, 6 June 2016
- 15:00-17:00
- HB 17.150
Signal Processing for Radio Astronomy
Yves Wiaux, Stefan Wijnholds, Amir Leshem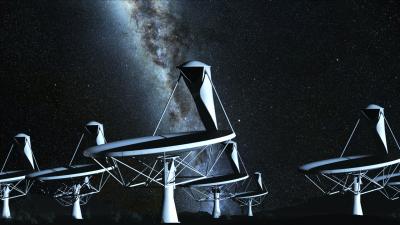
Yves Wiaux: "Astronomical imaging, in every sense of the word: scalable optimisation algorithms in radio-interferometry"
Amir Leshem: "Detection of transient sources"
Stefan Wijnholds: "Blind calibration of aperture arrays"
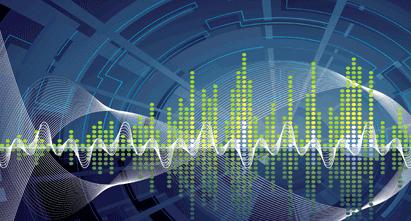
Signal Processing Seminar
- Thursday, 2 June 2016
- 13:30-14:30
- HB 17.150
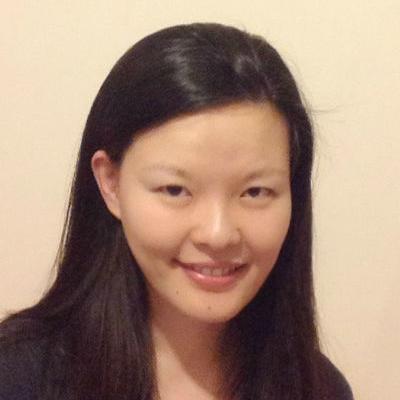
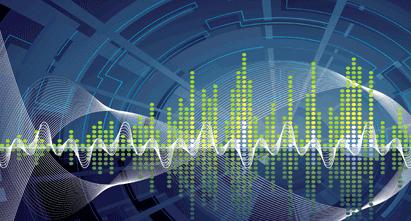
Computational neuroscience seminar
- Wednesday, 1 June 2016
- 10:00-11:00
- HB 17.150
Short presentations with update of MSc research projects status.
Additional information ...
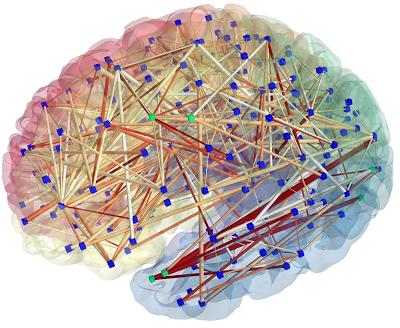
Signal Processing Seminar
- Thursday, 19 -- Thursday, 19 May 2016
- 13:30-14:30
- HB 17.150
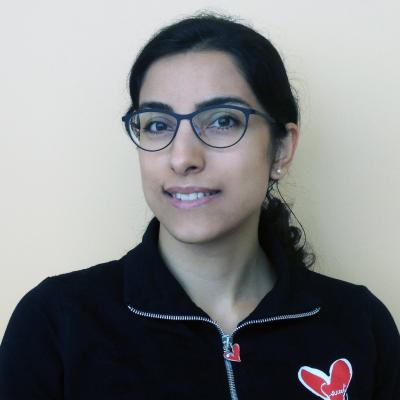
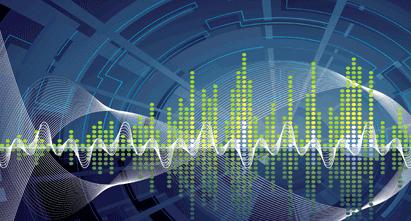
Computational neuroscience seminar
- Wednesday, 18 May 2016
- 10:00-11:00
- HB 17.150
Short presentations with update of MSc research projects status.
Additional information ...
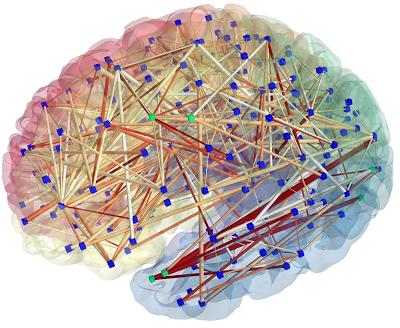
Signal Processing Seminar
- Thursday, 12 May 2016
- 13:30-14:30
- HB 17.150
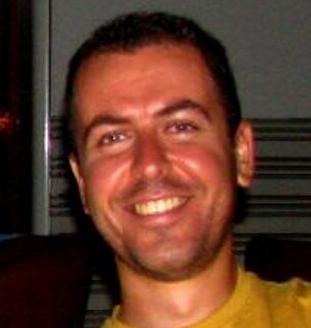
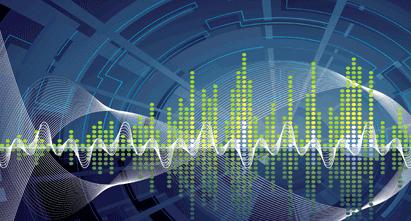
Signal Processing Seminar
- Thursday, 28 April 2016
- 13:30-14:30
- HB 17.150
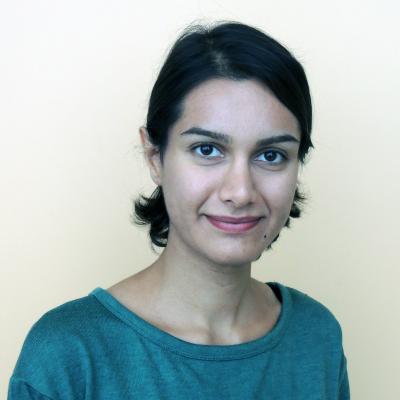
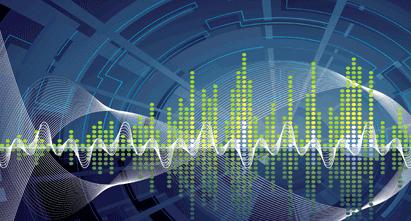
Signal Processing Seminar
- Thursday, 21 April 2016
- 13:30-14:30
- HB 17.150
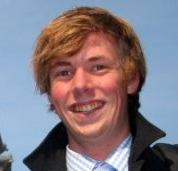
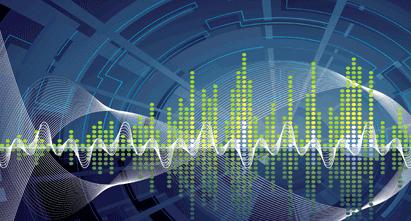
PhD Thesis Defence
- Tuesday, 19 April 2016
- 12:00-14:30
- Aula Senaatszaal
Cognitive Radio-based Home Area Networks
Mohd Adib Sarijari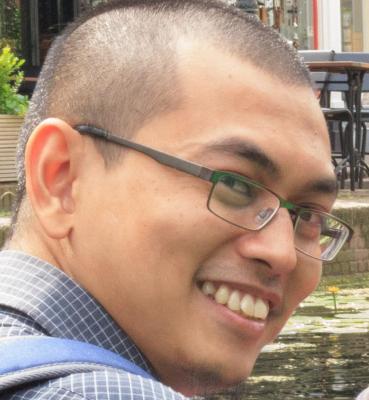
A future home area network (HAN) is envisaged to consist of a large number of devices that support various applications such as smart grid, security and safety systems, voice call, and video streaming. Most of these home devices are communicating based on various wireless networking technologies such as WiFi, ZigBee and Bluetooth which typically operate in the already congested ISM licensed-free frequency band. As these devices are located in a small physical space (i.e., limited by the size of the house), they might interfere with one another which causes a severe limitation to the quality-of-service (QoS) such as throughput. These issues are further aggravated in dense cities where the HAN also receives interference from neighboring HANs. Cognitive radio (CR) is seen as one of the most promising technologies to solve these problems and at the same time fulfill the HANs communication needs. CR technology enables the HAN devices to intelligently exploit idle spectrum including licensed spectrum for their communications, avoiding from being interfered as well as causing interference to others (in particular the incumbent user). We study these problems and the appropriateness of CR as a candidate solution.
We start by designing a new communication system for HAN based on CR technology and clustered network topology, called TD-CRHAN. TD-CRHAN aims at sustainably and efficiently supports the ever-rising throughput demand as well as solving the interference issue in HAN. In the TD-CRHAN, the achievable throughput is optimized to be just equal or slightly higher than the total network?s throughput demand, instead of being maximized. We then mathematically model the proposed TD-CRHAN where in the model, general expressions of the cooperative spectrum sensing performance parameters are considered. This allows us to analyze the performance of TD-CRHANfor amore realistic scenario where the incumbent user signal-to-noise-ratio (SNR) is not the same at different sensing devices. We illustrate promising results, numerically and through simulation, confirming the performances of the proposed design.
As a cognitive radio based network also imposes additional overhead in energy consumption due to the spectrum sensing, we then propose an energy efficient cooperative spectrum sensing (CSS) scheme. The scheme is designed based on the proposed TD-CRHAN. In this scheme we also ensure that the throughput demand is kept satisfied efficiently. From the difference in sensing devices? incumbent user SNR (that is previously considered), we select the optimal sensing devices for CSS with the corresponding sensing time and detection probability which can be varied from one sensing device to another. We then evaluate the proposed CSS scheme and exhibit the gains obtained in energy- and throughput-efficiency.
Finally, we present a sensing device grouping and scheduling scheme for multichannel CSS. In addition to the energy- and throughput-efficiency, this scheme addresses the fairness in spectrum sensing load distribution among the available sensing devices in a HAN. In this work, we consider the fairness objective as to maximize the lifetime of each sensing device to its expected lifetime. In the proposed scheme, we determine the optimal number of channels that should be used for the network and the selected channels. We also determine the optimal number of devices in each sensing group and which devices. Subsequently, we optimally schedule the formed sensing groups to sense the selected channels. The results from the performance analysis verify our claims.
Additional information ...
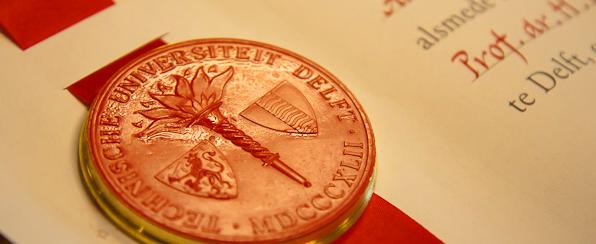
Signal Processing Seminar
- Thursday, 14 April 2016
- 13:30-14:30
- HB 17.150
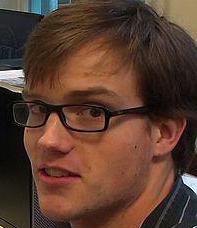
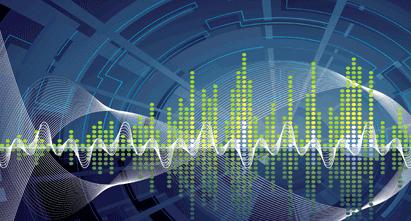
Signal Processing Seminar
- Tuesday, 5 April 2016
PhD Thesis Defence
- Thursday, 24 March 2016
- 12:30-14:30
- Aula Senaatszaal
Array of single-photon detectors applied to PET imaging
Chockalingam Veerappan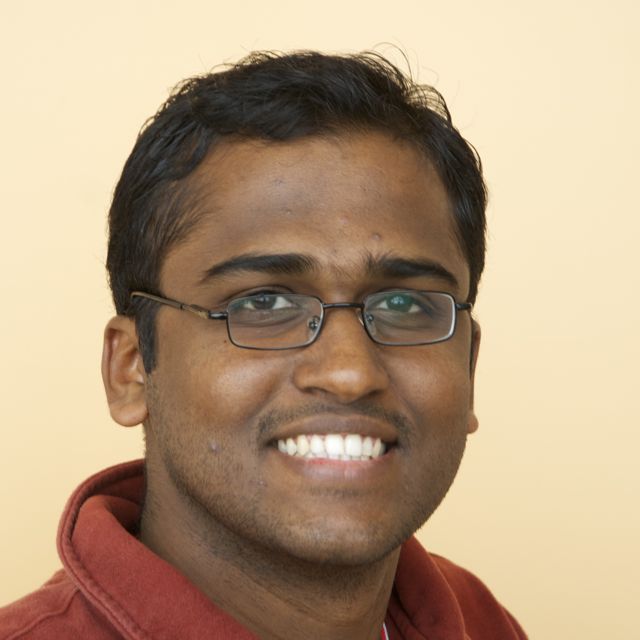
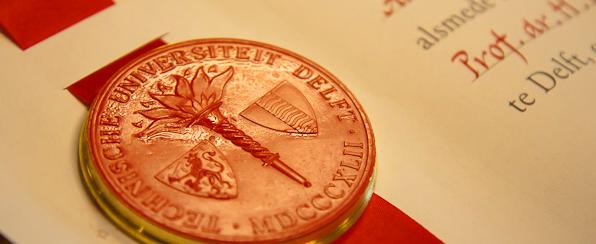
High-accuracy Positioning in Multipath Channels: Location-Awareness for 5G Networks
- Monday, 21 March 2016
- 15:45-16:45
- EWI, DI01.180
Graz University of Technology
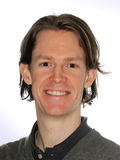
Location-awareness is the capability of a mobile network to employ position information for the sake of operating more efficiently. It is foreseen that fifth-generation (5G) wireless networks will be able to exploit much more accurate position information than any previous generation of wireless networks. This comes naturally due to the increased bandwidth and the application of multi-antenna techniques, which will eventually turn multipath propagation from an enemy to a friend.
This talk will first highlight the impact of multipath propagation on the accuracy of wireless range and position estimation. The important influence of bandwidth and the benefit of MIMO processing will be analyzed. Next it will be shown how one can make use of multipath to benefit from improved positioning accuracy, robustness, and a relaxed need for infrastructure nodes. Analytical performance bounds, their experimental validation, and algorithms derived thereof will be discussed. Finally, it will be shown that a multipath-enabled positioning system is a showcase example of a cognitive dynamic system that can optimize the information gained from each measurement, exploiting its memory of past measurements to plan future measurements. The environment map it collects can be used to predict the propagation characteristics, yielding location-awareness for positioning and communications. It is concluded that the use of position information may become as important to 5G networks as other new, disruptive technologies such as massive MIMO and mm-wave.
Biography
Klaus Witrisal received the Dipl.-Ing. degree in electrical engineering from Graz University of Technology, Graz, Austria, in 1997, the Ph.D. degree (cum laude) from Delft University of Technology, Delft, The Netherlands, in 2002, and the Habilitation from Graz University of Technology in 2009. He is currently an Associate Professor at the Signal Processing and Speech Communication Laboratory (SPSC) of Graz University of Technology, Graz, Austria, where he has been participating in various national and European research projects focused on UWB communications and positioning. He is co-chair of the Technical Working Group "Indoor'' of the COST Action IC1004 "Cooperative Smart Radio Communications for Green Smart Environments.'' His research interests are in signal processing for wideband and UWB wireless communications, propagation channel modeling, and positioning. Prof. Witrisal served as a leading chair for the IEEE Workshop on Advances in Network Localization and Navigation (ANLN) at the IEEE Intern. Conf. on Communications (ICC) 2013 - 2016, as a TPC (co)-chair of the Workshop on Positioning, Navigation and Communication (WPNC) 2011, 2014, and 2015, and as a co-organizer of the Workshop on Localization in UHF RFID at the IEEE 5th Annual Intern. Conf. on RFID, 2011. He is an associate editor of IEEE Communications Letters since 2013.
Signal Processing Seminar
- Thursday, 10 March 2016
- 13:30-14:30
- HB 17.150
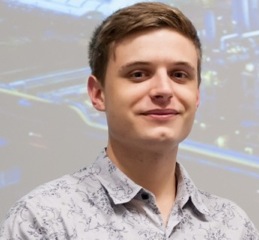
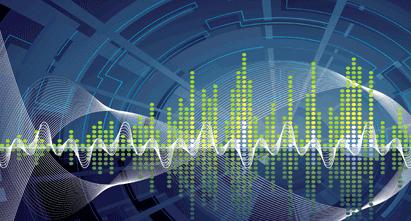
Signal Processing Seminar
- Thursday, 3 March 2016
- 13:30-14:30
- HB 17.150
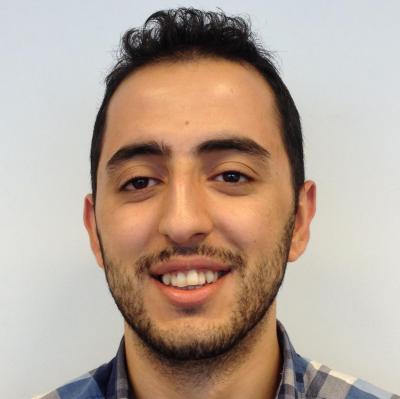
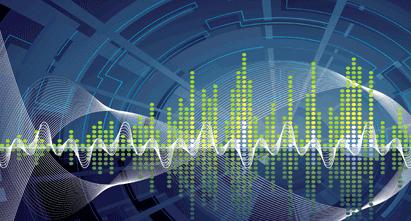
Signal Processing Seminar
- Thursday, 25 February 2016
- 13:30-14:30
- HB 17.150
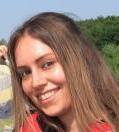
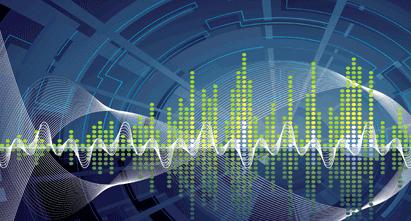
Signal Processing Seminar
- Thursday, 18 February 2016
- 13:30-14:30
- HB 17.150

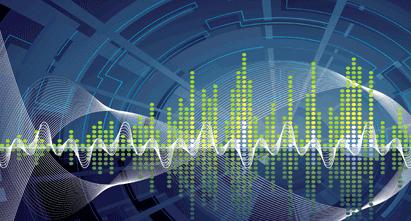
Signal Processing Seminar
- Thursday, 11 February 2016
- 13:30-14:30
- HB 17.150
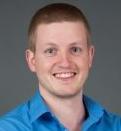
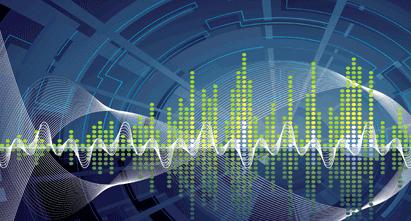
Signal Processing Seminar
- Thursday, 28 January 2016
- 14:00-15:00
- LB 01.170 Timmanzaal
An Algebraic Approach to Rank-Constrained Semidefinite Programs
Matthew Morency
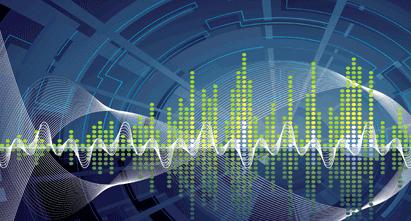
PhD Thesis Defence
- Monday, 25 January 2016
- 12:30-14:30
- Aula Senaatszaal
Sparse Sensing for Statistical Inference: Theory, Algorithms, and Applications
Sundeep Prabhakar Chepuri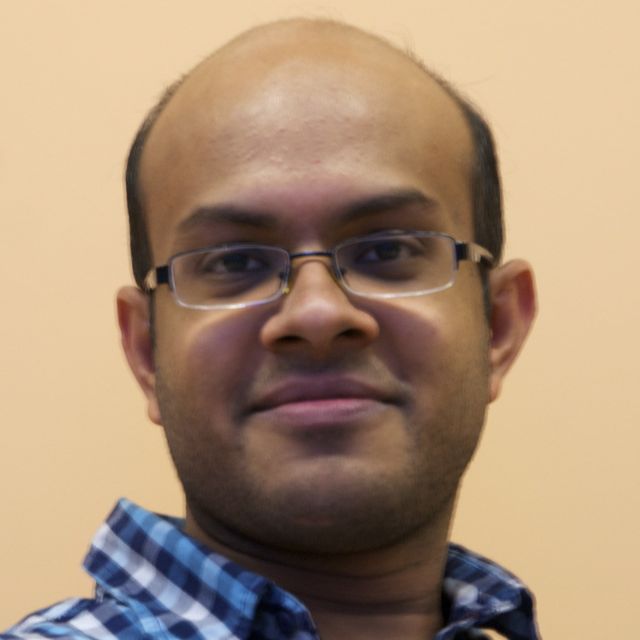
In today's society we are flooded with massive volumes of data of the order of a billion gigabytes on a daily basis from pervasive sensors. It is becoming increasingly challenging to locally store and transport the acquired data to a central location for signal/data processing (i.e., for inference). Consequently, most of the data is discarded blindly, causing serious performance loss. It is evident that there is an urgent need for developing unconventional sensing mechanisms to extract as much information as possible yet collecting fewer data. Thus, reducing the costs of sensing as well as the related memory and bandwidth requirements.
The first aim of this thesis is to develop theory and algorithms for data reduction. We develop a data reduction tool called sparse sensing, which consists of a deterministic and structured sensing function (guided by a sparse vector) that is optimally designed to achieve a desired inference performance with the reduced number of data samples. The first part of this thesis is dedicated to the development of sparse sensing models and convex programs to efficiently design sparse sensing functions.
Sparse sensing offers a number of advantages over compressed sensing (a state-of-the-art data reduction method for sparse signal recovery). One of the major differences is that in sparse sensing the underlying signals need not be sparse. This allows us to consider general signal processing tasks (not just sparse signal recovery) under the proposed sparse sensing framework. Specifically, we focus on fundamental statistical inference tasks, like estimation, filtering, and detection. In essence, we present topics that transform classical (e.g., random or uniform) sensing methods to low-cost data acquisition mechanisms tailored for specific inference tasks. The developed framework can be applied to sensor selection, sensor placement, or sensor scheduling, for example.
In the second part of this thesis, we focus on some applications related to distributed sampling using sensor networks. Recent advances in wireless sensor technology have enabled the usage of sensors to connect almost everything as a network. Sensor networks can be used as a spatial sampling device, that is, to faithfully represent distributed signals (e.g., a spatially varying phenomenon such as a temperature field). On top of that, the distributed signals can exist in space and time, where the temporal sampling is achieved using the sensor's analog-to-digital converters, for example. Each sensor has an independent sample clock, and its stability essentially determines the alignment of the temporal sampling grid across the sensors. Due to imperfection in the oscillators, the sample clocks drift from each other, resulting in the misalignment of the temporal sampling grids. To overcome this issue, we devise a mechanism to distribute the sample clock wirelessly. Specifically, we perform wireless clock synchronization based on the time-of-flight measurements of broadcast messages. In addition, clock synchronization also plays a central role in other time-based sensor network applications such as localization. Localization is increasingly gaining popularity in many applications, especially for monitoring environments beyond human reach, e.g., using robots or drones with several sensor units mounted on it. Consequently we now have to localize more than one sensor or even localize the whole sensing platform. Therefore, we extend the classical localization paradigm to localize a (rigid) sensing platform by exploiting the knowledge of the sensor placement on the platform. In particular, we develop algorithms for rigid body localization, i.e., for estimating the position and orientation of the rigid platform using distance measurements.
Additional information ...
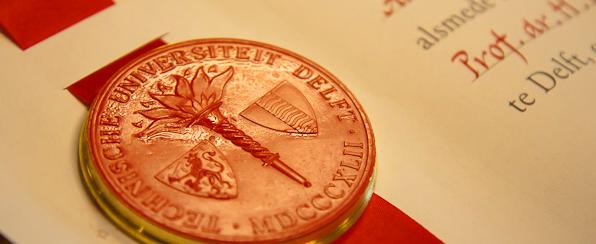
Signal Processing Seminar
- Friday, 22 January 2016
- 14:00-15:00
- Snijderszaal LB 01.010
Censor, Sketch, and Validate for Learning from Large-Scale Data
Georgios GiannakisUniv. of Minnesota
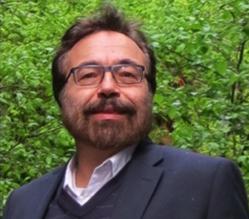
We live in an era of data deluge. Pervasive sensors collect massive amounts of information on every bit of our lives, churning out enormous streams of raw data in various formats. Mining information from unprecedented volumes of data promises to limit the spread of epidemics and diseases, identify trends in financial markets, learn the dynamics of emergent social-computational systems, and also protect critical infrastructure including the smart grid and the Internet?s backbone network.
While Big Data can be definitely perceived as a big blessing, big challenges also arise with large-scale datasets. This talk will put forth novel algorithms and present analysis of their performance in extracting computationally affordable yet informative subsets of massive datasets. Extraction will effected through innovative tools, namely adaptive censoring, random subset sampling (a.k.a. sketching), and validation. The impact of these tools will be demonstrated in machine learning tasks as fundamental as (non)linear regression, classification, and clustering of high-dimensional, large-scale, and dynamic datasets.
Biography
Georgios B. Giannakis received his Diploma in Electrical Engr. from the Ntl. Tech. Univ. of Athens, Greece, 1981. From 1982 to 1986 he was with the Univ. of Southern California (USC), where he received his MSc. in Electrical Engineering, 1983, MSc. in Mathematics, 1986, and Ph.D. in Electrical Engr., 1986. Since 1999 he has been a professor with the Univ. of Minnesota, where he now holds an ADC Chair in Wireless Telecommunications in the ECE Department, and serves as director of the Digital Technology Center.His general interests span the areas of communications, networking and statistical signal processing ? subjects on which he has published more than 380 journal papers, 650 conference papers, 20 book chapters, two edited books and two research monographs (h-index 115). Current research focuses on big data analytics, wireless cognitive radios, network science with applications to social, brain, and power networks with renewables.
He is the (co-) inventor of 22 patents issued, and the (co-) recipient of 8 best paper awards from the IEEE Signal Processing (SP) and Communications Societies, including the G. Marconi Prize Paper Award in Wireless Communications. He also received Technical Achievement Awards from the SP Society (2000), from EURASIP (2005), a Young Faculty Teaching Award, the G. W. Taylor Award for Distinguished Research from the U. of Minnesota, and the IEEE Fourier Technical Field Award (2015). He is a Fellow of IEEE and EURASIP, and has served the IEEE in a number of posts including that of a Distinguished Lecturer for the IEEE-SP Society.
Additional information ...
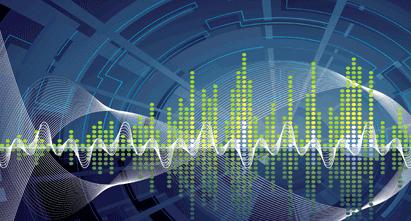
Signal Processing Seminar
- Wednesday, 20 January 2016
- 13:00-14:00
- Vassiliadis room HB10.230
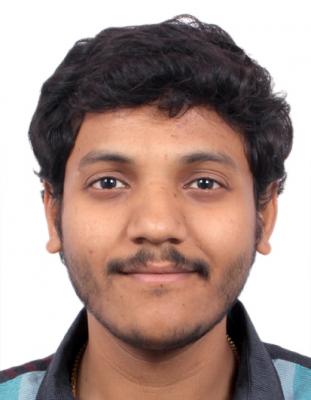
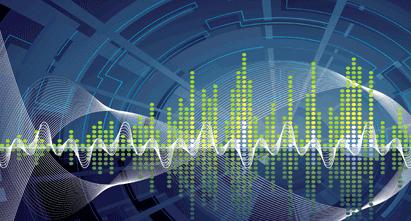
Signal Processing Seminar
- Thursday, 14 January 2016
- 13:30-14:30
- EWI - Snijderszaal
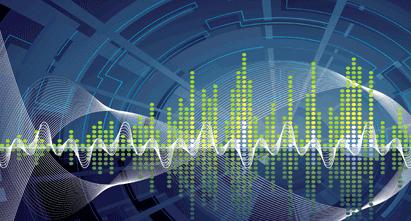
MSc Thesis Presentation
- Thursday, 14 January 2016
- 14:00-15:30
- HB17.150
Multi-Domain SystemC Model of a Neural Interface
Kiki Wirianto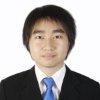
Neural networks have been investigated by researchers for several decades. Microelectrodes and neural interface are used to obtain the informations contained in the neuronal networks activity, which can be used to control neural prosthetic devices. This field has developed rapidly and the current research is focusing on multi-channel implementation of neural interface system to monitor the activity of a large number of neurons simultaneously.
Area and safety are two main constraints in the design of neural interface electronics. The chip area constrain is important to minimize the severity of the surgery and limit the displacement of the brain caused by the implanted device. The safety constrain is critical in avoiding the damage to the brain tissue. Both constrains create a limitation on the power consumption of the neural interface system. This limited power budget needs to be utilized carefully to implement a design with low noise and high data rate with as few computational resources as possible. An efficient design allows a large number of channels to be implemented within the allowed power budget.
This thesis proposes behavioral models of the electrode and the neural interface front-end, a part which precondition the neural signals before they are further processed and stored. The functionality of the proposed models are verified and, together with a power estimation model, they are used to perform a system study to investigate the trade-offs between neural interface design parameters.
Additional information ...
Signal Processing Seminar
- Thursday, 10 December 2015
- 13:30-14:30
- HB017.150, EWI
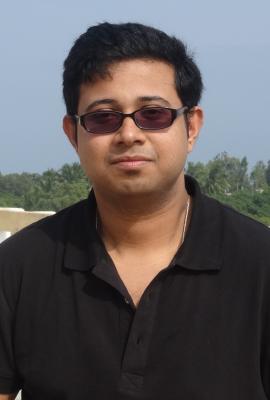
PhD Thesis Defence
- Friday, 4 December 2015
- 10:00-11:30
- Aula Senaatszaal
Blind Beamforming Techniques for Global Tracking Systems
Mu Zhou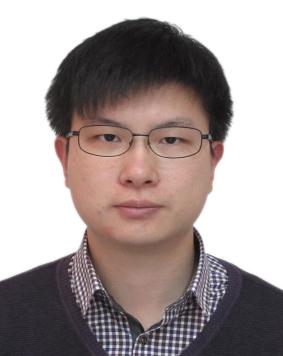
In lower frequency bands, existing communication systems face unprecedented demands to accommodate more users in new applications. These growing demands exceed the designed system capacity and thus call for innovative solutions while keeping compatibility to the current setup to reduce the cost of users. For example, in the automatic identification system (AIS), satellite receivers are being used for expanding the service coverage of ship tracking to the global range, and similarly in the automatic dependent surveillance-broadcast (ADS-B) system for aircraft tracking. These systems are narrowband and originally designed in the last century, but they will continue to run for at least another couples of years without major updating of the user-side equipment.
The new application of AIS considered in this thesis is Satellite AIS. The satellite runs in the low-earth orbit (LEO). On the satellite, receiving AIS signals becomes much more difficult than before: one has to combat in-cell and inter-cell interfering sources from the system itself. Interference suppression is the main topic of this thesis.
Narrowband spatial beamforming techniques for antenna arrays are candidate solutions to this challenge. This thesis tries to develop new beamforming techniques with a simple structure and a low computational complexity. With these techniques, this thesis establishes a framework of multiuser reception for Satellite AIS.
As a basic tool for the proposed algorithms in this thesis, a signed URV algorithm (SURV) is proposed for the basic problem of principal subspace computation and tracking as a replacement of the singular value decomposition (SVD). The updating and downdating of SURV is direct and simple. SURV has no issue of numerical stability unlike previous algorithms in linear algebra and shows consistent performance in both stationary and nonstationary cases.
New blind beamforming techniques are proposed for separating overlapping packets in nonstationary scenarios. The connections between subspace intersection, oblique projection, the generalized SVD (GSVD), the generalized eigenvalue decomposition (GEVD), and SURV are exposed. Simulation and experimental results of the proposed algorithms are shown.
In the remaining part of the thesis, the work developing the software simulation model and constructing the hardware platform is presented. The outputs of the work are used for the verification and validation of the proposed algorithms in this thesis.
Additional information ...
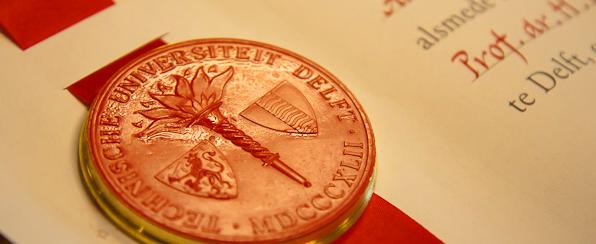
Signal Processing Seminar
- Friday, 4 December 2015
- 13:30-14:15
- HB 17.150
Efficient two-way relaying schemes for amplify and forward relays with multiple antennas
Martin HaardtTU Ilmenau, Germany
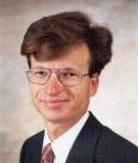
Biography
Martin Haardt is a Full Professor in the Department of Electrical Engineering and Information Technology and Head of the Communications Research Laboratory at Ilmenau University of Technology, Germany, since 2001.He received his Ph.D. degree from Munich University of Technology in 1996 after which he joined Siemens Mobile Networks.
Martin Haardt has received the 2009 Best Paper Award from the IEEE Signal Processing Society, the Vodafone (formerly Mannesmann Mobilfunk) Innovations-Award for outstanding research in mobile communications, the ITG best paper award from the Association of Electrical Engineering, Electronics, and Information Technology (VDE), and the Rohde & Schwarz Outstanding Dissertation Award.
His research interests include wireless communications, array signal processing, high-resolution parameter estimation, as well as numerical linear and multi-linear algebra.
Additional information ...
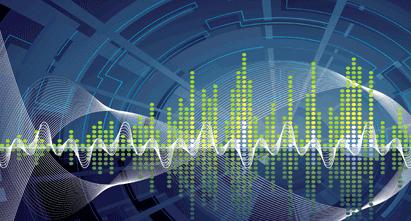
Signal Processing Seminar
- Thursday, 3 December 2015
- 16:30-17:00
- HB 17.150
From Tensor Decomposition to Coupled Matrix/Tensor Decompositions in Array Processing
Lieven DelathauwerKU Leuven
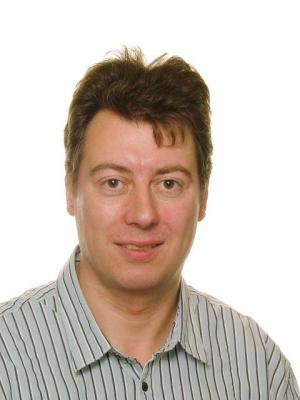
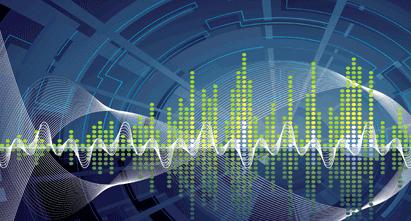
Signal Processing Seminar
- Thursday, 3 December 2015
- 16:00-16:30
- HB 17.150
Something on GSVD
Soren Holdt JensenAalborg University (Denmark)
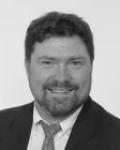
GSVD, oblique projections, and source separation
Additional information ...
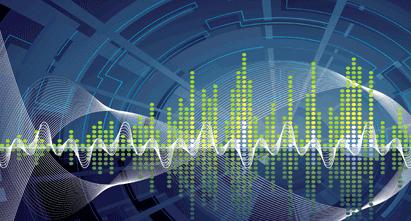
MSc ME Thesis Presentation
- Thursday, 26 November 2015
- 10:00
- HB17.150
Sensor Selection and Bit Allocation in WSNs with Realistic Digital Communication Channels
Hongrun Zhang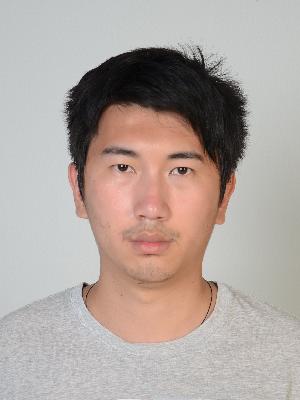
For energy management in wireless sensor networks, only the sensors with most informative measurements are activated to operate. How to select sensors that make good tradeoff between performance and energy consumption is what many researchers are focusing on. Existing solutions assume analog data model, i.e., the data from sensors collected by a center node, called fusion center, are analog measurements. In practical application, due to limitations of energy of sensors and bandwidth of wireless channel, original measurements are usually compressed before being transmitted to the fusion center. In addition, transmitted signals are usually distorted by wireless channel effects, therefore it is possible that the received data are corrupted with errors. In this thesis, we consider two compressive techniques: one-bit quantization and multi-bit quantization. In one-bit quantization, an indicator message is generated in a sensor according to whether the original measurement is larger than a threshold or not. In multi-bit quantization, the original measurements are quantized to multiple bits and only the most significant bits are reserved. The indicators or the most significant bits are then transmitted through realistic wireless channel to the fusion center for it to process. By these ways, the transmitted signals are digital, and they may flip into opposite values by the effects of wireless channels. For one-bit quantization case, we develop a sensor selection approach, based on convex programming. For multi-bit quantization, we extend the sensor selection to bit allocation and propose a novel algorithm to determine the number of bits to transmit for each sensors, which is also based on convex programming. In both cases we consider the effects of wireless channels, which are characterized as bit error rate. Particularly, for the multi-bit quantization, numerical results show that the bit allocation can further reduce the cost that we defined compared with existing solutions where transmitted data are assumed to be analog.
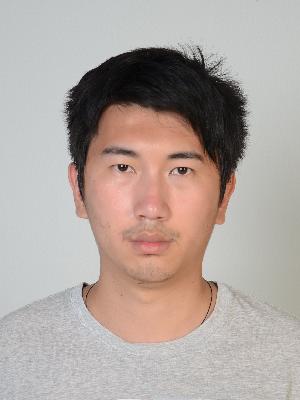
Signal Processing Seminar
- Thursday, 26 November 2015
- 13:30-14:30
- HB017.150, EWI
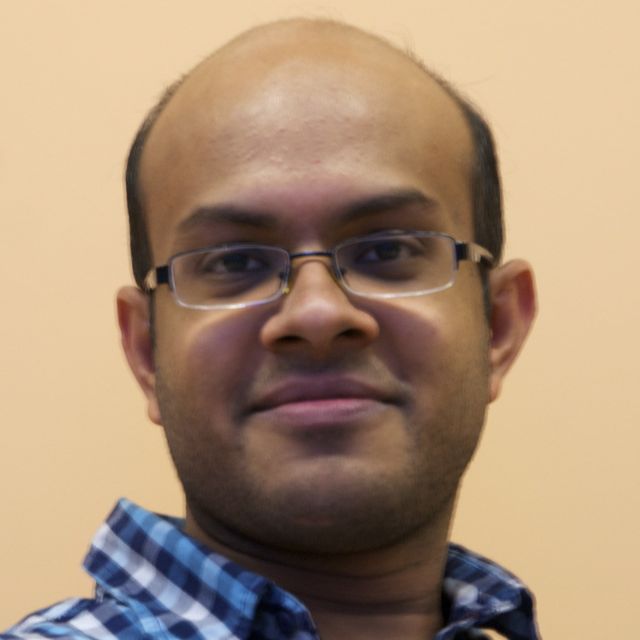
Data reduction tools like censoring or sketching for (large-scale) linear inverse problems in presence of outliers and/or bounded data uncertainties
Additional information ...
Signal Processing Seminar
- Thursday, 19 November 2015
- 13:30-14:30
- HB017.150, EWI
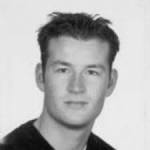
MSc SS Thesis Presentation
- Friday, 13 November 2015
- 10:00
- HB17.150
Speech Based Onset Estimation for Multisensor Localization
Rodolfo Solera Urcuyo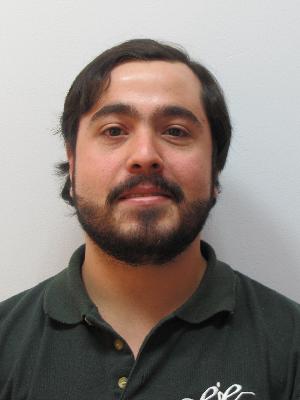
This work presents a study of a current problem in the field of audio processing: Source and receiver localization. Currently, this problem requires that either the onset time of the sources or the internal delay of the receivers are known. The algorithms studied here, take advantage of the structure of the time matrix, which contains the TOA of all the receivers with respect to all the sources, and finds the solution to the locations when the onset times are known. The problem here is then approached from a time difference of arrival (TDOA) perspective, which inherently cancels the onset times by subtracting the time of arrival (TOA) of a source at every receiver.
An alternative approach is also proposed, which uses speech signals as calibration signals in order to estimate the onset times. Such an approach is based on an algorithm which uses artificial calibration signals to calculate the onsets. Those signals are known a-priori, which implies that an additional device which produces those signals is needed. Once both internal delays and onset times are known, the locations of both sources and receivers can be estimated using a current algorithm which is also described here
MSc ME Thesis Presentation
- Friday, 13 November 2015
- 09:00
- Dijkstrazaal, HB09.150
A 1 GSa/s Deep Cryogenic, Reconfigurable Soft-core FPGA ADC for Quantum Computing Applications
Stefan Visser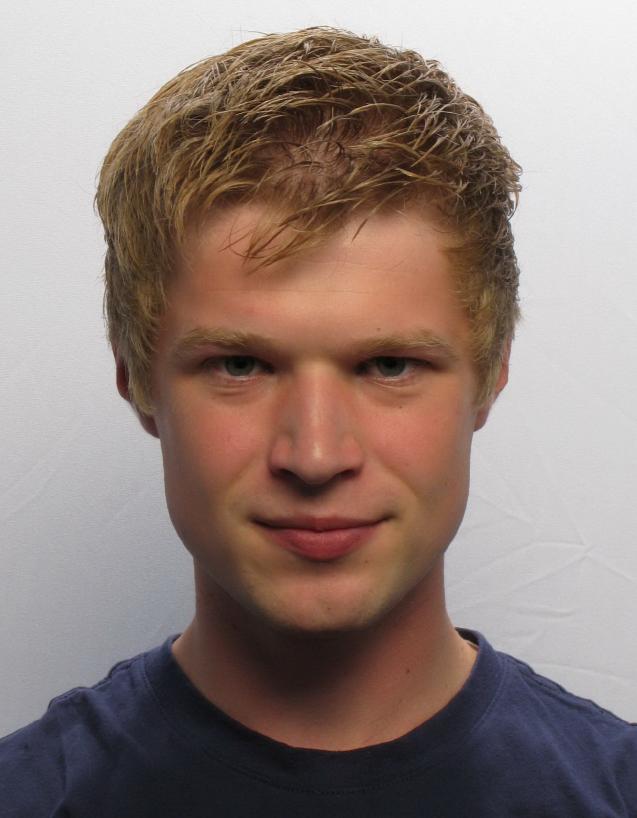
This project proposes a solution using a FPGA to create a soft-core ADC architecture. Except for some small resistors on the PCB, the ADC can be completely integrated into the reconfigurable hardware blocks of an FPGA. Therefore the ADC can be easily interfaced with the remainder of the digital circuitry, it can be scaled to the required sampling rate or resolution and it even allows ADCs with different specifications in one system.
This approach allows calibration to each new environment the system is operating in, i.e. changes in voltage, temperature or chip can be calibrated out. We aim to show the effectiveness of our calibration techniques by operating the ADC both at room temperature and in a deep cryogenic environment at 4 Kelvin.
Signal Processing Seminar
- Thursday, 12 November 2015
- 13:45-14:45
- HB 17.150
Schur Subspace Estimator
Alle-Jan van der Veen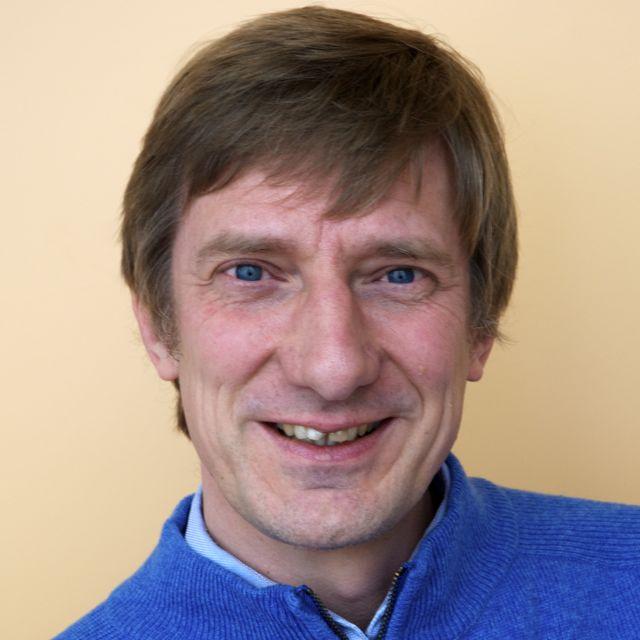
The ?Schur subspace estimator? (SSE) can replace the SVD and GSVD, and is easily updated allowing sliding window tracking of subspaces.
It is based on a generalization of the Schur algorithm. Recall that the Schur algorithm establishes the ?stability? of a polynomial (roots inside unit circle) without explicitly computing the roots. Likewise, the SSE partitions the space into a dominant and a minor subspace w.r.t. a threshold, without computing the SVD.
(Joint work with Mu Zhou, who will defend his PhD thesis on Dec. 4.)
Additional information ...
Signal Processing Seminar
- Tuesday, 27 October 2015
- 16:00-16:45
- HB 17.150
Compressive Sensing-based Video Coding and Range Imaging Platforms
George TzagkarakisEONOS Investment Technologies (Paris, Fr.) and ICS-FO.R.T.H (Crete, Greece)
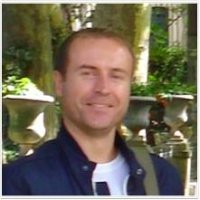
The framework of compressive sensing (CS), acting simultaneously as a sensing and compression protocol, has revolutionized the design of low-complexity onboard remote imaging devices with reduced power and processing requirements. This is achieved by reducing radically the sampling rates dictated by the well-established Shannon-Nyquist theory.
In this presentation, the core properties of CS will be exploited towards improving the performance of existing solutions in two distinct application areas of high industrial interest.
First, we will focus on the design of efficient lightweight video codecs to cope with the growing compression ratios required by modern remote imaging applications. To this end, appropriate CS modules are embedded in the typical MPEGx and M/JPEG systems to exploit frame sparsity in an appropriate residual and/or transform domain, with the main computational burden being put at the decoder, where increased computational and power resources are usually available.
The second application concerns the design of a CS-based methodology for range imaging using time-of-flight cameras, which aims at reducing dramatically the number of necessary frames for reconstructing the depth map of a scene. The use of CS in this case is motivated naturally by the inherent spatial sparsity of objects located in space.
For both application scenarios, we illustrate the effectiveness of CS in improving significantly the performance of previous approaches, thus making it a very promising solution for future high-performance systems.
About the presenter
Dr. G. Tzagkarakis received the Ph.D. and M.Sc. degrees (first in class, Honors) from the Computer Science Department ‐ University of Crete (UoC), Greece, and a B.Sc. degree in Mathematics from the Department of Mathematics (UoC) (first in class, Honors).Since 2000 he has been also collaborating with the Wave Propagation Group of the Institute of Applied and Computational Mathematics (IACM)‐FO.R.T.H., while in 2002‐2010 he was affiliated as a research assistant in the Telecommunications and Networks Lab (TNL) of the Institute of Computer Science (ICS)‐FO.R.T.H. In the period 2010‐2012 he was a member of the Cosmology and Statistics Lab of CEA/Saclay as a Marie Curie post‐doctoral researcher focusing on the design and implementation of compressive sensing algorithms for remote imaging in areal and terrestrial surveillance systems.
From 2012, he holds a research associate position in EONOS Investment Technologies, working on the development of statistical signal processing algorithms with applications in computational finance and econometrics, while in parallel he is a research collaborator with the Signal Processing Lab (SPL) at ICS‐FO.R.T.H.
His research interests lie in the fields of statistical signal and image processing, with emphasis in non‐Gaussian heavy‐tailed modeling, compressive sensing and sparse representations, with applications in signal processing, image and video processing, and computational finance. Among his topics of interest are also distributed signal processing for sensor networks, information theory, image classification and retrieval, and inverse problems in underwater acoustics.
Additional information ...
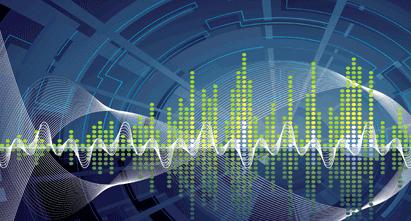
MSc SS Thesis Presentation
- Tuesday, 27 October 2015
- 14:00-14:40
- HB 17.150
Automated Detection of Central Apnea in Preterm Infants
Marina Nano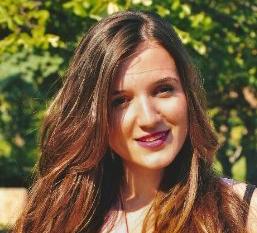
In 2010, an estimated 14.9 million babies were born preterm, which amounted to 11.1% of?all?livebirths worldwide, ranging from about 5% in several European countries to 18% in?some?African countries. The rate of preterm births has increased remarkably. Prematurity of birth can?predispose neonates to undesirable cessations of breathing, a condition?termed as Apnea of?Prematurity. The prevalence of this condition poses problems, because?when untreated or?inadequately treated Apnea of Prematurity, may impair development.?
This thesis investigates the?automated central apnea detection in preterm infants based on?raw waveform analysis of one-lead ECG and chest impedance signals. For this purpose,?18 novel features and 34 features of?existing research that characterize different aspects?of chest impedance and ECG signals were?extracted for automated apnea classification.?Features aim to extract information regarding?respiratory and cardiac regularity, estimated?from chest impedance and ECG signals. These?features are indicators of some properties?of cardio-respiratory physiology, which is not?independent of the presence of apnea and?thus can be in turn used to classify apnea.
The?objective is to find the most discriminative subset of features from one-lead ECG and chest?impedance signals that can be used?by a machine-learning approach to study and accurately?detect central apnea. This was?achieved by applying feature selection algorithms in order to?remove redundant or irrelevant features without incurring much loss of information.
In this?thesis, nine hours of continuously recorded data of ten very low-birth-weight infants (birth weight?< 1,500 gr) undergoing continuous cardiopulmonary monitoring in the NICU at Maxima Medisch?Centrum?from 2008 were included in the analysis. The dataset was annotated by two?neonatologists.?Results from this work indicate that the analysis of chest impedance and ECG?signals with?a support vector machine can automatically detect Apnea of Prematurity.
Additional information ...
MSc SS Thesis Presentation
- Monday, 26 October 2015
- 14:00-14:40
- HB 17.150
Blind Segmentation of Time-Series
Vana Panagiotou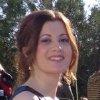
Change-point detection is an indispensable tool for a wide variety of applications which?has been?extensively studied in the literature over the years. However, the development of?wireless devices and?miniature sensors that allows continuous recording of data poses new?challenges that cannot be?adequately addressed by the vast majority of existing methods.
?In this work, we aim to balance?statistical accuracy with computational efficiency, by developing a hierarchical two-level algorithm?that can significantly reduce the computational?burden in the expense of a negligible loss of detection?accuracy. Our choice is motivated?by the idea that if a simple test was used to quickly select some?potential change-points?in the first level, then the second level which consists of a computationally?more expensive algorithm, would be applied only to a subset of data, leading to a significant run-time?improvement. In addition, in order to alleviate the difficulties arising in high-dimensional?data, we use a?data selection technique which gives more importance to data that are more?useful for detecting?changes than to others.
Using these ideas, we compute a detection?measure which is given as the?weighted sum of individual dissimilarity measures and we?present techniques that can speed up some?standard change-point detection methods.?Experimental results on both artificial and real-world data?demonstrate the effectiveness?of developed approaches and provide a useful insight about the?suitability of some of the?state-of-the-art methods for detecting changes in many different scenarios.
Additional information ...
Distinguished Lecture IEEE COMSOC
- Monday, 19 October 2015
- 14:00-15:00
- HB 17.150
A Stochastic Analysis of Network MIMO Systems
Wei YuUniversity of Toronto

Network MIMO, where base-stations (BSs) cooperatively transmit and receive to/from the users, promises to significantly alleviate the inter-cell interference problem in wireless cellular networks; but its analytical performance characterization is still a difficult open problem.
In this talk, we describe a stochastic geometry analysis of a network MIMO system, where the multiple-antenna BSs are distributed according to a Poisson point process and cooperate using zero-forcing beamforming to serve multiple users. We obtain tractable and accurate approximations of the signal power and inter-cluster interference power distributions, and derive a computationally efficient expression for the achievable per-BS ergodic sum rate. The analysis enables us to obtain the optimal number of users to schedule. Further, it allows us to quantify the performance improvement of network MIMO systems as a function of the cooperating cluster size.
In particular, due to the zero-forcing penalty across a distributed set of BSs and the inevitable out-of-cluster interference that always exists, the per-BS ergodic sum rate of a network MIMO system does not approach that of an isolated cell even at unrealistically large cluster sizes.
Finally, we illustrate the benefit of user-centric clustering for cell-edge users, and remark on a comparison between massive MIMO and network MIMO systems.
About the presenter
Professor Wei Yu (S?97-M?02-SM?08-F?14) received the B.A.Sc. degree in Computer Engineering and Mathematics from the University of Waterloo, Waterloo, Ontario, Canada in 1997 and M.S. and Ph.D. degrees in Electrical Engineering from Stanford University, Stanford, CA, in 1998 and 2002, respectively. Since 2002, he has been with the Electrical and Computer Engineering Department at the University of Toronto, Toronto, Ontario, Canada, where he is now Professor and holds a Canada Research Chair (Tier 1) in Information Theory and Wireless Communications. His main research interests include information theory, optimization, wireless communications and broadband access networks.Additional information ...
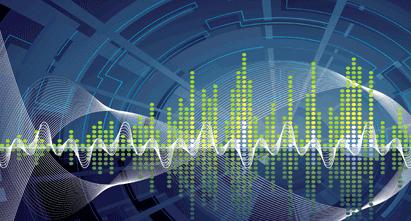
- Thursday, 8 October 2015
- 12:00-13:00
- LB 1.01 Snijderszaal
CAS lunch
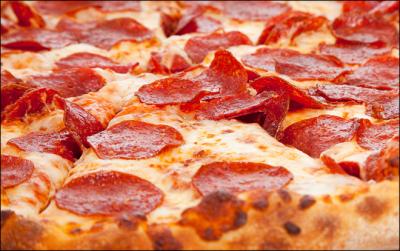
Let's introduce several new members of the CAS group!
MSc ME Thesis Presentation
- Tuesday, 22 September 2015
- 14:00-14:40
- HB 17.150
Highly Accurate Synchronization Over Ethernet
Jeroen Somers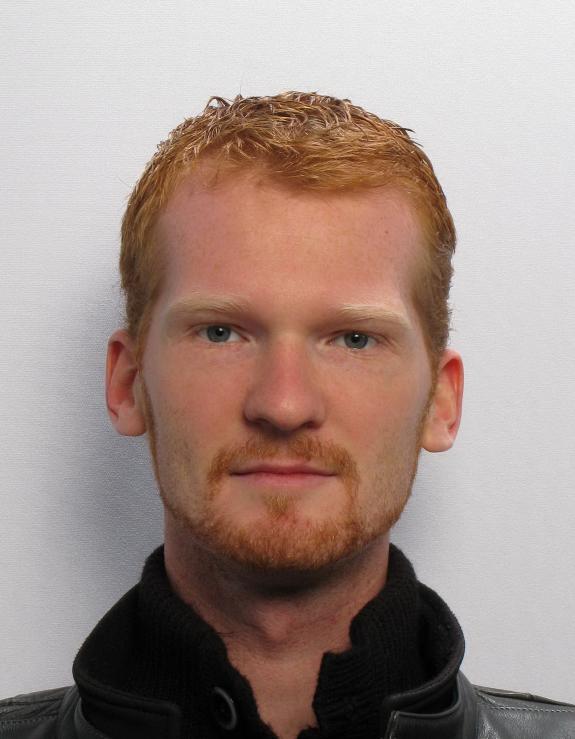
Audio Signal Processing Seminar
- Wednesday, 16 September 2015
Audio Signal Processing Seminar
- Wednesday, 16 September 2015
Audio Signal Processing Seminar
- Wednesday, 16 September 2015
Audio Signal Processing Seminar
- Wednesday, 16 September 2015
PhD Thesis Defence
- Tuesday, 15 September 2015
- 12:30-14:00
- Aula Senaatszaal
Thermal-Aware Design and Runtime Management of 3D Stacked Multiprocessors
Sumeet Kumar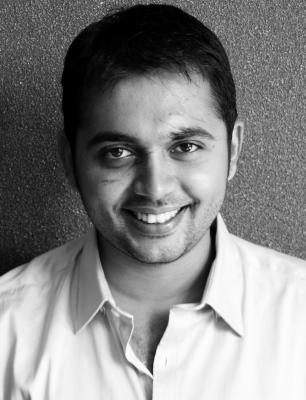
This dissertation presents architectural techniques to enable the realization of efficient, high-performance chip multiprocessors, and facilitate runtime temperature management to ensure their dependable operation. Most importantly, it provides new insights into the complex thermal behaviour of 3D ICs, and illustrates how the design space of stacked die architectures can be effectively explored in order to maximize performance in the dark silicon era. This dissertation consists of two main themes, architecture and temperature.
The thesis addresses the following questions.
- How can the performance and efficiency of on-chip memory operations in multiprocessors be improved?
- How do the physical design parameters in Nagata?s equation affect the thermal behavior of 3D Integrated Circuits?
- How can the knowledge of thermal behaviour be effectively leveraged in the design of 3D stacked multiprocessors?
- How can the architecture and operating parameters be efficiently adapted at runtime to mitigate the severity of thermal issues, and improve execution performance?
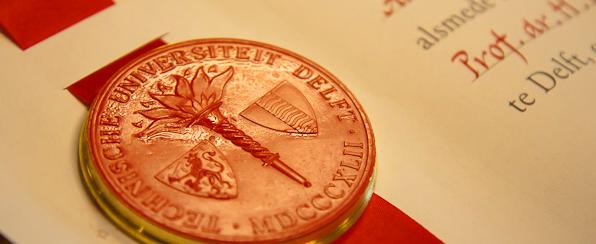
Audio Signal Processing Seminar
- Tuesday, 15 September 2015
- 10:00-11:00
- HB 17.150
Fast room-mode reproduction in box-shaped rooms: the rigid walls case.
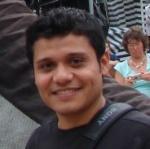
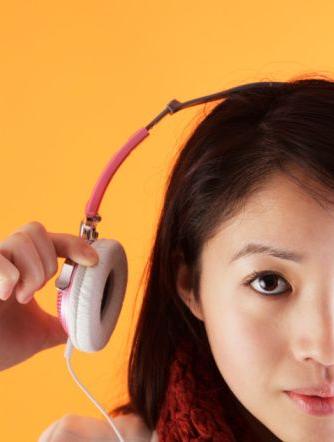
MSc TC Thesis Presentation
- Thursday, 27 August 2015
- 10:00-10:45
- HB 17.150
GSVD based blind-beamforming technique for suppression of partially overlapping Bluetooth data packets from WiFi signals
Shailja Shukla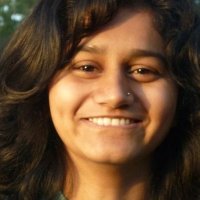
WLAN 802.11 (WiFi) and WPAN 802.15 (Bluetooth) operate in the same 2.4GHz ISM band. OFDM WLANs are designed to provide very high data rates, resilience to multipath and extended operating range. One of the main barriers to actually achieving the high data rates is the interference from the Bluetooth systems which is one of the main sources of interference in the 2.4GHz unlicensed band. This thesis investigates the interference problem and proposes a novel subspace-based method to mitigate the Bluetooth interference inWiFi signals using spatial filtering with an array of antennas. We assume continuous transmission of WLAN packets and partially overlapping, unsynchronous and intermittent Bluetooth interfering packets. The proposed method estimates the target (WiFi) and interfering (Bluetooth) signal subspaces and uses this subspace information to estimate beamformers for interference suppression.
Results show that through the proposed subspace based algorithm the interference of Bluetooth transmission for 802.11 as target model can be reduced and the throughput of 802.11 can be significantly improved at the expense of additional computational complexity.
Additional information ...
MSc TC Thesis Presentation
- Wednesday, 22 July 2015
- 10:00-11:00
- HB 17.150
Compressive Power Spectrum Estimation
Ruijie Zhang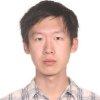
Power spectrum estimation of a wide-sense stationary signal using multi-coset (non-uniform) sampling
MSc TC Thesis Presentation
- Thursday, 16 July 2015
- 14:00-15:00
- HB 17.150
Sparse Arrays: Vector Sensors and Design Algorithms
Shilpa Rao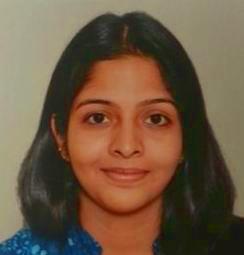
Direction-of-arrival (DOA) estimation of acoustic sources is of great interest in a number of applications. Acoustic vector sensors (AVSs) provide an edge over traditional scalar sensors since they measure the acoustic velocity field in addition to the acoustic pressure. It is known that a uniform linear array (ULA) of M conventional scalar sensors can identify up to M-1 DOAs. However, using second-order statistics, the class of sparse scalar sensor arrays have been shown to identify more source DOAs than the number of sensors. In this thesis, we extend these results using sparse AVS arrays. We first assume that the sources are quasi-stationary and use the Khatri-Rao subspace approach to estimate the source DOAs. In addition, a spatial-velocity smoothing technique is proposed to estimate the DOAs of stationary sources. For both scenarios, we show that the number of source DOAs that can be identified is significantly greater than the number of physical vector sensors. The second problem considered in this thesis is sensor selection for non-linear models. It is often necessary to guarantee a certain estimation accuracy by choosing the best subset of the available set of sensors. A non-linear measurement model in additive Gaussian noise is considered. To solve the sensor selection problem, which is inherently combinatorial, a greedy algorithm based on submodular cost functions is developed. The proposed low-complexity greedy algorithm is computationally attractive as compared to existing sensor selection solvers for non-linear models. The submodular cost ensures optimality of the greedy algorithm. Such a sensor selection can be applied, for example, to design sparse AVS arrays that also ensure a certain quality of the DOA estimates next to their identifiability.
Additional information ...
- Wednesday, 15 July 2015
- 14:00-15:00
- Seminar Room 17th floor
Doppler-resilient Orthogonal Signal Division Multiplexing for Underwater Acoustic Communication
Tadashi EbiharaUniversity of Tsukuba, Japan
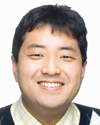
D-OSDM multiplexes several data vectors in addition to a pilot vector, and preserves orthogonality among them even after propagation through doubly spread channels, under the assumption that the channel can be modeled by a basis expansion model (BEM). We describe the signal processing steps at the transmitter and the receiver for D-OSDM, and evaluate its performance by both simulations and experiments. To generate a doubly spread channel, a test-tank with a wave generator is employed. The obtained results suggest that D-OSDM can provide low-power and high-quality UWA communications in channels with large delay and Doppler spreads. Overall, it was found that D-OSDM can become a powerful communication tool for underwater operations.
Additional information ...
MSc ME Thesis Presentation
- Thursday, 9 July 2015
- 09:30-10:30
- HB 9.150 (Dijkstrazaal)
Implementation of in-situ monitoring techniques for power reduction in smart sensors
Guozhi Xu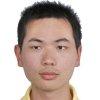
Nowadays, smart sensors are widely used in a variety of application domains, such as telecommunication, health care, cars, mobile phones, smart cities. Because of limited battery capacity, low-power design is required for smart sensors. Low-voltage operation is a key leverage to reduce power consumption in smart sensors. However, uncertainties due to process, voltage and temperature variations or random fluctuations gain in relevance when operating in the near-threshold range. Hence, monitoring of the actual silicon behavior is crucial to lowering supply voltage while preserving reliable operation. An interrupt-based in-situ monitoring approach is proposed in this thesis. This approach uses an interrupt service routine to stimulate the critical paths on the chip. By monitoring the timing of the exercised paths, a warning signal is generated to steer the control of reliable supply voltage levels. This approach is developed, validated and applied on an ARM-based processor. Finally, a 10 mV supply voltage margin is achieved based on measurements in the near-threshold range. In addition, reliable operation is verified by running different self-checking codes over multiple dies while varying the environmental conditions.
MSc TC Thesis Presentation
- Wednesday, 8 July 2015
- 14:00-14:40
- HB 14.240
Software Defined Radio Receiver Design Developement for China Digital Radio
Yun Wang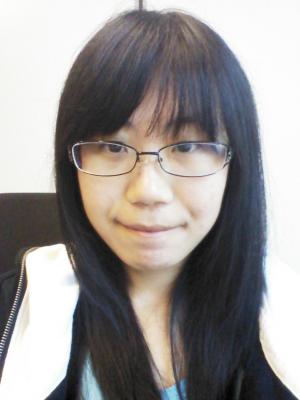
(Thesis work done at NXP, Eindhoven)
MSc SS Thesis Presentation
- Monday, 6 July 2015
- 14:00-15:00
- HB14.240
Distributed Convex Optimization
He Ming Zhang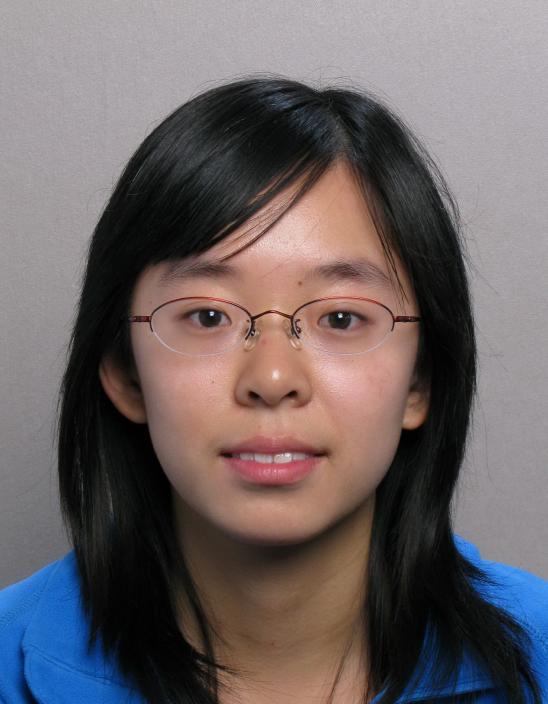
The Primal-Dual Method of Multipliers (PDMM) is a new algorithm that solves convex optimization problems in a distributed manner.?This study focuses on the convergence behavior of the PDMM. For a deeper understanding, the PDMM algorithm was applied to distributed averaging and distributed dictionary learning problems. The results were compared to those of other state-of-the-art algorithms. The experiments show that the PDMM algorithm not only has a fast convergence rate but also robust performance against transmission failures in the network.?Furthermore, on the basis of these experiments, the convergence rate of the PDMM was analyzed. Different attempts at proving the linear convergence rate were carried out. As a result, the linear convergence rate has been proven under certain conditions.
MSc SS Thesis Presentation
- Friday, 3 July 2015
- 11:00-11:45
- HB16.240
Heart Rate Variability Analysis based on Instantaneous Frequency Estimation
Di Feng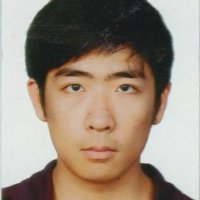
MSc ME Thesis Presentation
- Monday, 29 June 2015
- 13:30-14:15
- HB14.240
Low power digital baseband architecture for wireless sensor nodes
Yuteng Hao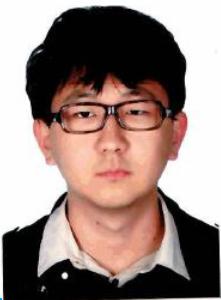
This thesis presents a digital baseband design for an upcoming wireless standards: IEEE 802.11ah. It is a branch of Wi-Fi (IEEE 802.11) standards. Compared with the previous Wi-Fi standards, this new standard has larger coverage range and consumes less energy. It is particularly suited for energy-constrained sensor applications.? In contrast to the Digital Baseband (DBB)s of other Wi-Fi standards, this design consumes much less power. The basic modulation method of the system is Orthogonal Frequency Division Multiplexing (OFDM) and the detailed algorithms are explored. To prove the robustness of the system, some error tests for the system are performed. A gate-level hardware design and the synthesis netlist are also presented to prove the low-power design. Based on the synthesis results, a series of optimization is done to lower the power consumption.? The DBB has been implemented in 40nm Low-power CMOS process to prove the concept.? It includes the key blocks of this system. Measurement results show that the DBB for IEEE 802.11ah is suitable for low power applications. The power consumption of this DBB is around 200 - 400 uW, which is hundreds times less than that of the traditional 802.11 baseband.
Thesis work performed at Holst Centre
Additional information ...
3rd International Workshop on Compressed Sensing Theory and its Applications to Radar, Sonar and Remote Sensing
- Monday, 22 -- Wednesday, 24 June 2015
- Pisa, Italy

Following the success of the previous two editions of the workshop on compressive sensing applied to radar, we are pleased to announce the third one in this series. The 3rd Int. Workshop on Compressed Sensing Theory and its Applications to Radar, Sonar and Remote Sensing (CoSeRa 2015) will be held in Pisa (Italy) on 22-24 June 2015. The aim of CoSeRa is to bring experts of Compressive Sensing (CS) and radar/sonar/EO/IR signal processing and remote sensing together to explore the state-of-the-art in development of CS techniques for different areas of applications and to turn out its advantages or possible drawbacks compared to classical solutions.
Topics include but are not limited to:
-
Compressive sensing theory
-
Mathematical aspects of Compressive sensing in imaging systems
-
Sparsity of Radar/SAR/Sonar/IR signals
-
Applications of sparse sensing in Radar/SAR/Sonar/IR signal processing
-
Compressive sensing for SAR tomography (TomoSAR)
-
Compressive sensing for SAR Interferometry (InSAR)
-
Compressive sensing for Inverse SAR (ISAR)
-
Target detection based on compressive sensing
-
Compressive sensing for slow GMTI
-
Co-prime sampling in radar/sonar/EO/IR systems
-
Co-prime array processing in radar/sonar/EO/IR systems
-
Nested sampling in radar/sonar/EO/IR systems
-
Netsted array processing in radar/sonar/EO/IR systems
-
Sparse sensing in synthetic aperture imaging systems
Important Dates
- Full five-page paper submission: February 2, 2015
- Notification of acceptance: March 16, 2015
- Final camera-ready papers and author registration: April 20, 2015
All accepted and presented papers will be referenced by IEEEXplore
Additional information ...
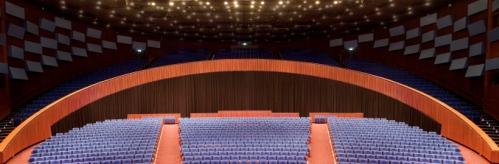
Signal Processing Seminar
- Friday, 19 June 2015
- 10:00-10:45
- Lipkenszaal LB01.150
Speaker Tracking Using Recursive EM Algorithms
Sharon GannotBar Ilan University (Israel)
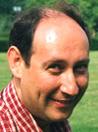
The problem of localizing and tracking a known number of concurrent speakers in noisy and reverberant enclosures is addressed in this talk. We formulate the localization task as a maximum likelihood (ML) parameter estimation problem, and solve it by utilizing the expectation-maximization (EM) procedure.
For the tracking scenario, we propose to adapt two recursive EM (REM) variants. The first, based on Titterington's scheme, is a Newton-based recursion. In this work we also extend Titterington's method to deal with constrained maximization, encountered in the problem at hand. The second is based on Cappe and Moulines' scheme. We discuss the similarities and dissimilarities of these two variants. The applicability of the proposed methods to localization and tracking problems is demonstrated using both simulated data and recordings from our acoustic lab.
Additional information ...
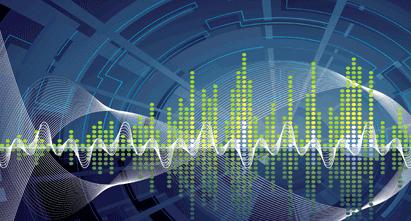
Signal Processing Seminar
- Friday, 19 June 2015
- 10:45-11:30
- Lipkenszaal LB01.150
A Simple Model of Speech Communication and its Application to Intelligibility Enhancement
Bastiaan Kleijn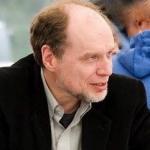
Modern technology allows speech communication from anywhere to anywhere. With phone booths a relic of the past, speech intelligibility has become a common problem, particularly when the listener side is noisy. We will show that it is possible to enhance intelligibility in a noisy listener environment using a formal, information-theory based approach. The new paradigm leads to a family of intelligibility-enhancement algorithms, some of which resemble existing heuristically-derived methods.
Additional information ...
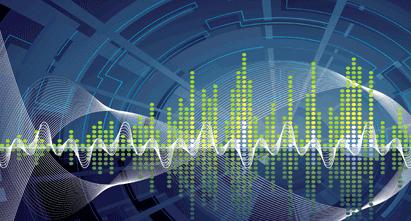
PhD Thesis Defence
- Thursday, 18 June 2015
- 15:00-16:30
- Aula Senaatszaal
Distributed Speech Enhancement in Wireless Acoustic Sensor Networks
Yuan Zeng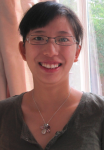
In digital speech communication applications like hands-free mobile telephony, hearing aids and human-to-computer communication systems, the recorded speech signals are typically corrupted by background noise. As a result, their quality and intelligibility can get severely degraded. Traditional noise reduction approaches process signals recorded by microphone arrays using centralized beamforming technologies. Recent advances in micro-electromechanical systems and wireless communications enable the development of wireless sensor networks (WSNs), where low-cost, low-power and multi-functional wireless sensing devices are connected via wireless links. Compared with conventional localized and regularly arranged microphone arrays, wireless sensor nodes can be randomly placed in environments and thus cover a larger spatial field and yield more information on the observed signals. This thesis explores some problems on multi-microphone speech enhancement for wireless acoustic sensor networks (WASNs), such as distributed noise reduction processing, clock synchronization and privacy preservation.
First, we develop a distributed delay-and-sum beamformer (DDSB) for speech enhancement in WASNs. Due to limited power of each wireless device, signal processing algorithms with low computational complexity and low communication cost are preferred in WASNs. Distributed signal processing allows that each node only communicates with its neighboring nodes and perform local processing, where communication load and computational complexity are distributed over all nodes in the network. Without central processor and network topology constraint, the DDSB algorithm estimates the desired speech signal via local processing and local communication. The DDSB algorithm is based on an iterative scheme. More specifically, in each iteration, pairs of neighboring nodes update their estimates according to the principle of traditional delay-and-sum (DSB) beamformer. The estimation of the DDSB converges asymptotically to the optimal solution of the centralized beamformer. However, experimental study indicates that the noise reduction performance of the DDSB is at the expense of a higher communication cost, which can be a serious drawback in practical applications.
Therefore, in the second part of this thesis, a clique-based distributed beamformer (CbDB) has been proposed to reduce communication costs of the original DDSB algorithm. In the CbDB, nodes in two neighboring non-overlapping cliques update their estimates simultaneously per iteration. Since each non-overlapping clique consists of multiple nodes, the CbDB allows more nodes to update their estimates and leads to lower communication costs than the original DDSB algorithm. Furthermore, theoretical and experimental studies have shown that the CbDB converges to the centralized beamformer and is more robust for sensor nodes failures in WASNs.
In the third part of this thesis, we propose a privacy preserving minimum variance distortionless response (MVDR) beamformer for speech enhancement in WASNs. Different wireless devices in WASNs generally belong to different users. We consider a scenario where a user joins the WASN and estimates his desired source via the WASN, but wants to keep his source of interest private. To introduce a distributed MVDR beamformer in such scenario, a distributed approach is first proposed for recursively estimation of the inverse of the correlation matrix in randomly connected WASNs. This distributed approach is based on the fact that using the Sherman- Morrison formula, estimation of the inverse of the correlation matrix can be seen as a consensus problem. By hiding the steering vector, the privacy preserving MVDR beamformer can reach the same noise reduction performance as its centralized version.
In the final part of this thesis, we investigate clock synchronization problems for multi-microphone speech enhancement in WASNs. Each wireless device in WASNs is equipped with an independent clock oscillator, and therefore clock differences are inevitable. However, clock differences between capturing devices will cause signal drift and lead to severe performance degradation of multi-microphone noise reduction algorithms. We provide theoretical analysis of the effect of clock synchronization problems on beamforming technologies and evaluate the use of three different clock synchronization algorithms in the context of multi-microphone noise reduction. Our experimental study shows that the achieved accuracy of the three clock synchronization algorithms enables sufficient accuracy of clock synchronization for the MVDR beamformer in ideal scenarios. However, in practical scenarios with measurement uncertainty or noise, the output of the MVDR beamformer with time-stamp based clock synchronization algorithms gets degraded, while the accuracy of signal based clock synchronization algorithms is still enough for the MVDR beamformer, albeit at a much higher communication cost.
Additional information ...
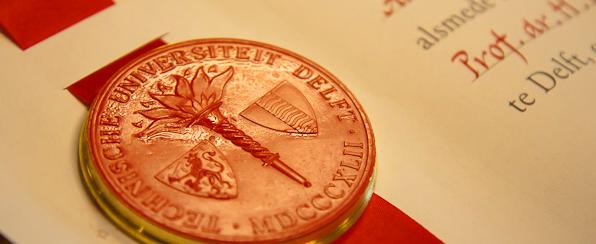
Signal Processing Seminar
- Thursday, 4 June 2015
- 13:30-14:30
- HB 14.240
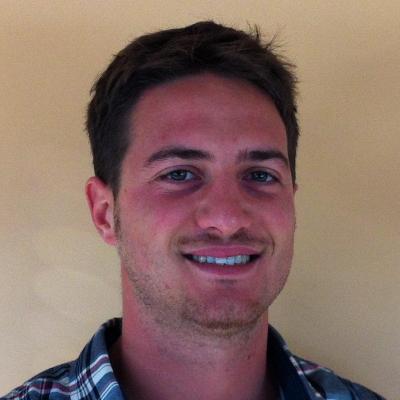
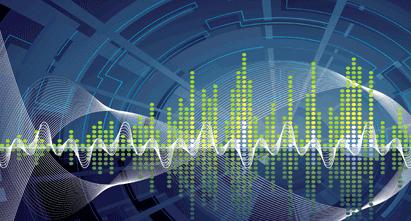
Signal Processing Seminar
- Thursday, 28 May 2015
- 13:30-14:30
- HB 14.240
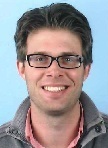
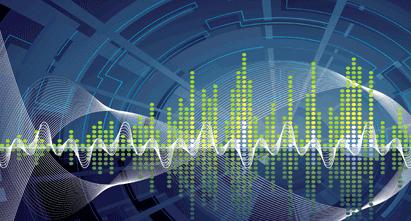
Signal Processing Seminar
- Thursday, 21 May 2015
- 13:00-13:45
- HB 14.240
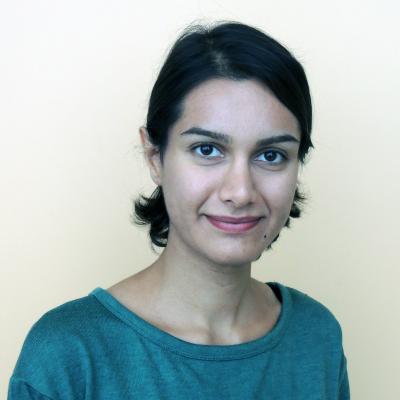
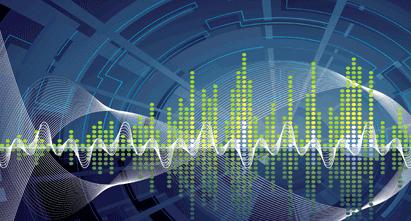
Signal Processing Seminar
- Thursday, 7 May 2015
- 13:30-14:30
- HB 14.240
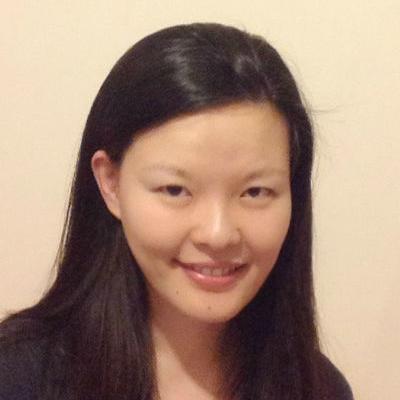
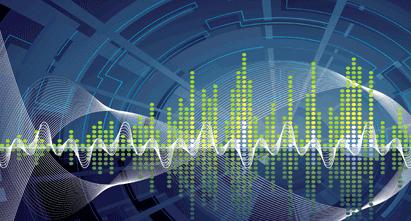
Signal Processing Seminar
- Thursday, 23 April 2015
- 13:30-14:30
- HB 14.240
Maximum Likelihood Self-Estimation for Path-Loss Exponent in Wireless Networks
Yongchang Hu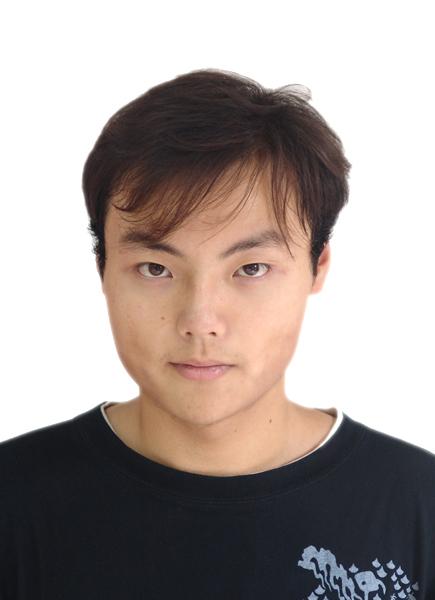
Signal Processing Seminar
- Thursday, 16 April 2015
- 13:30-14:30
- HB 14.240
Additional information ...
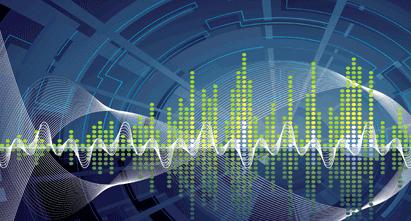
Signal Processing Seminar
- Thursday, 9 April 2015
- 13:30-14:30
- HB 14.240
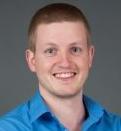
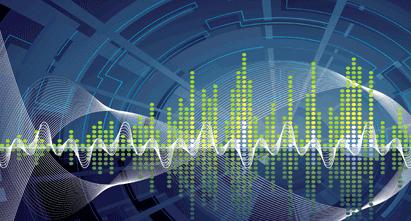
Signal Processing Seminar
- Thursday, 2 April 2015
- 13:30-14:30
- HB 14.240
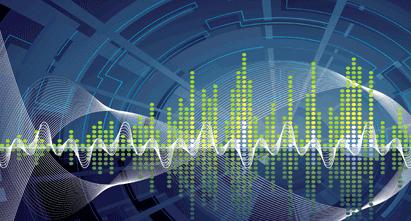
QuTech Seminar
- Tuesday, 24 March 2015
- 16:00-17:00
- EWI Snijderszaal (LB 01.010)
Designs for quantum information hybrid devices and systems
Prof. Kae NemotoNational Institute of Informatics, Quantum Information Sciences, Tokyo, Japan
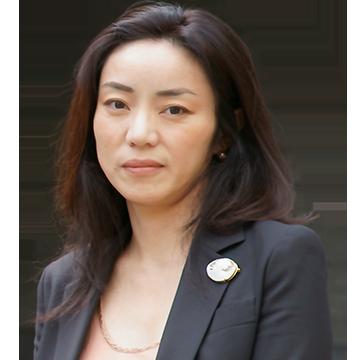
There have been many architectures for quantum computer and quantum information devices proposed, yet we face a gap between these proof-of-principle idea and feasible quantum devices. We focus on an integrated cavity device based on a single diamond NV center to identify the problems and obstacles by integrating necessary elements to perform computational tasks.
Additional information ...
PhD Thesis Defence
- Thursday, 19 March 2015
- 15:00-16:30
- Aula Senaatszaal
Compressive Power Spectral Analysis
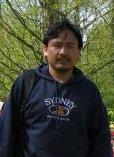
The main focus of this thesis is on the reconstruction of the second-order statistics (including correlations and power spectrum) from digital samples produced by compressive sampling a.k.a. sub-Nyquist-rate sampling. Note that it has been known that compressive sampling offers substantial assistance in sampling rate reduction, which is important when we deal with signals having a very large bandwidth.
What interests us is that there are applications where the sampling still needs to be done at sub-Nyquist rate (due to the high bandwidth of the signal of interest) but where the second-order statistics (instead of the original signal) are of interest. One application is, for instance, spectrum sensing for a cognitive radio network, which is a network where unlicensed radio systems opportunistically search for a currently unoccupied frequency band in the licensed spectrum and then borrow these discovered ?white spaces? to establish a communication link. This spectrum sensing is continuously performed by these unlicensed systems since they have to monitor when the actual owners of the borrowed bands (called licensed users) become suddenly active, in which case the unlicensed radios have to vacate the spectrum. In this application, sampling the signal at sub-Nyquist rate is of interest since the spectral range that has to be sensed is generally very wide. However, note that the unlicensed radio systems are never interested in the original signal of the licensed users occupying the bands to be monitored. This implies that a power spectrum plot describing which frequency bands are occupied together with the amount of power in the occupied bands is more than enough and any efforts to reconstruct the original signal in this application will be overkill. We show that power spectrum reconstruction of WSS signals below the Nyquist rate is possible without any additional constraints on the original signal or the power spectrum.
Other applications are the estimation of directions-of-arrival from more sources than antennas.
Additional information ...
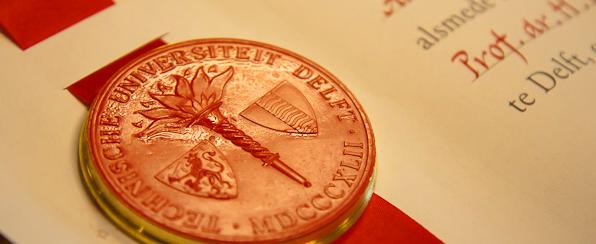
Signal processing mini-symposium
- Thursday, 19 March 2015
- 10:00-12:15
- LB 01.150 (Lipkenszaal)
University of Vigo, Vigo, Spain. Aalto University, Helsinki, Finland. Technion, Haifa, Israel.
Facilitator: Prof. dr. ir. Geert Leus, Circuits and Systems group
1. Carving the Multicarrier Spectrum
Roberto L?pez Valcarce University of Vigo, Vigo, Spain
Multicarrier modulation has become the format of choice in modern high-speed wireless and wireline systems, due to its many well-known qualities. Nevertheless, the large IFFT sidelobes result in substantial leakage across subcarriers with the ensuing adjacent channel interference. The usual approach of deactivating a number of guard subcarriers at the edges of the signal spectrum is very inefficient in terms of data rate. In order for OFDM to be adopted by future high-performance systems, e.g., 5G, a number of enhancements will become necessary to overcome this and other drawbacks. The leakage problem is also of concern in wideband OFDM-based cognitive systems, in which deep notches must be sculpted in the spectrum to avoid interfering to narrowband licensed users. Judiciously modulating (a few) cancellation subcarriers in order to reduce leakage is an appealing alternative, which originally incurred in high online implementation complexity. We will review this Active Interference Cancellation approach and present efficient designs recently developed in our group, with extensions to linear symbol precoding.
Roberto Lopez-Valcarce received the Ph.D. degree in electrical engineering from the University of Iowa, Iowa City, in 2000. He was a Postdoctoral Fellow of the Spanish Ministry of Science and Technology from 2001 to 2006, with the Signal Theory and Communications Department, University of Vigo, Spain, where he currently is an Associate Professor. His main research interests lie in the areas of adaptive signal processing, digital communications, and sensor networks, having coauthored over 50 papers in leading international journals. He holds several patents in collaboration with industry. Roberto was the recipient of a 2005 Best Paper Award of the IEEE Signal Processing Society. He served as an Associate Editor of the IEEE TRANSACTIONS ON SIGNAL PROCESSING from 2008 to 2011, and as a member of the IEEE Signal Processing for Communications and Networking Technical Committee from 2011 to 2013.
2. Optimal Array Signal Processing in the Face of Non-Idealities
Visa Koivunen Aalto University, Helsinki, Finland
In this talk we describe techniques that facilitate applying high performance array processing algorithms using real-world sensor arrays with nonidealities. The theoretical background is in wavefield modeling that allows one to develop computationally-efficient and asymptotically-optimal array processing methods regardless of the array geometry (conformal arrays). Wavefield modeling also facilitates incorporating array nonidealities into array processing methods and performance bounds. Parameter estimation and beamforming in the azimuth-elevation-polarimetric domain will be addressed. We acquire a realistic array steering vector model by taking into account array nonidealities such as mutual coupling, mounting platform reflections, cross-polarization effects, errors in element positions as well as individual directional beampatterns. This facilitates achieving optimal or close-to-optimal performance and retaining high-resolution capability despite the nonidealities. Moreover, tighter performance bounds may be established for parameter estimation. We describe how the various approaches can be applied in practice in the context of high-resolution direction finding as well as beamforming so that problems related to beamsteering, SOI and interference cancellation are mitigated. This is joint work with Dr. Mario Costa.
Visa Koivunen received his Ph.D in electrical engineering from the University of Oulu, Finland. He was visiting researcher at the Univ of Pennsylvania in 1992-1995. Since 1999 he has been full professor of signal processing at Aalto University (Helsinki Univ of Technology), Finland where he currently holds the Academy Professor position. He has been an adjunct professor at Penn and visiting fellow at Nokia Research Center. He has spent multiple research visits and sabbaticals terms at Princeton University. His research interests include statistical, communication and array signal processing. Dr. Koivunen is an IEEE Fellow and 2015 IEEE SPS Distinguished Lecturer. He received the 2007 IEEE Signal Processing Society best paper award.
3. Sub-Nyquist Sampling: Bounds, Algorithms and Hardware
Yonina Eldar Technion, Haifa, Israel
The famous Shannon-Nyquist theorem has become a landmark in the development of digital signal processing. However, in many modern applications, the signal bandwidths have increased tremendously, while the acquisition capabilities have not scaled sufficiently fast. Consequently, conversion to digital has become a serious bottleneck. Furthermore, the resulting high rate digital data requires storage, communication and processing at very high rates which is computationally expensive and requires large amounts of power. In this talk, we present a framework for sampling and processing a wide class of wideband analog signals at rates far below Nyquist. We refer to this methodology as sampling:combination of compression and sampling, performed simultaneously.
Using the Cramer-Rao bound we develop a generic low-rate sampling architecture that is optimal in a mean-squared error sense, and can be applied to a wide variety of wideband inputs. The resulting system can be readily implemented in hardware, and is easily modified to incorporate correlations between signals. We consider application of these ideas to a variety of problems including low rate ultrasound imaging, radar detection, ultra wideband communication, and cognitive radio, and show several demos of real-time sub-Nyquist prototypes.
Yonina Eldar received the B.Sc. degree in physics and the B.Sc. degree in electrical engineering both from Tel-Aviv University (TAU), Tel-Aviv, Israel, in 1995 and 1996, respectively, and the Ph.D. degree in electrical engineering and computer science from the Massachusetts Institute of Technology (MIT), Cambridge, in 2002. She is currently a Professor in the Department of Electrical Engineering at the Technion?Israel Institute of Technology, Haifa. She is also a Research Affiliate with the Research Laboratory of Electronics at MIT and a Visiting Professor at Stanford University, Stanford. Dr. Eldar was a Horev Fellow of the Leaders in Science and Technology program at the Technion and an Alon Fellow. In 2004, she was awarded the Wolf Foundation Krill Prize for Excellence in Scientific Research, in 2005 the Andre and Bella Meyer lectureship, in 2007 the Henry Taub Prize for Excellence in Research, in 2008 the Hershel Rich Innovation Award, the Award for Women with Distinguished Contributions, the Muriel & David Jacknow Award for Excellence in Teaching, and the Technion Outstanding Lecture Award, in 2009 the Technion's Award for Excellence in Teaching, in 2010 the Michael Bruno Memorial Award from the Rothschild Foundation, and in 2011 the Weizmann Prize for Exact Sciences. In 2012 she was elected to the Young Israel Academy of Science and to the Israel Committee for Higher Education, and elected an IEEE Fellow. In 2013 she received the Technion's Award for Excellence in Teaching, the Hershel Rich Innovation Award, and the IEEE Signal Processing Technical Achievement Award. In 2014 she was awarded the IEEE/AESS Fred Nathanson Memorial Radar Award. She received several best paper awards together with her research students and colleagues. She is the Editor in Chief of Foundations and Trends in Signal Processing. In the past, she was a Signal Processing Society Distinguished Lecturer, a member of several Signal Processing technical committees, and an associate editor for several IEEE and SIAM journals.
Additional information ...
MEST Symposium
- Tuesday, 17 March 2015
- 09:00-16:00
- Aula conference center
THE SILICON CRYSTAL BALL

Symposium on silicon technology -?where speakers from industry, academia and from leading research?centers?within?Netherlands and from abroad will cover the latest advancements and challenges in silicon technology.
Speakers
- P. de Jager( ASML) Lithography beyond EUV
- E. Vreugdenhil (ASML) 3D-NAND Flash: vertical stacking of new thin-film gate-all-around transistors
- M. Pelgrom (PelgromConsulting) Statistical design has the future
- Z. Tokei (IMEC) Wiring in 3D
- F. Rosenboom (TU Eindhoven) Plasma etching for continued semiconductor scaling
- S. Hamdioui (TU Delft) Computing for Data-Intensive Applications: Beyond CMOS and beyond Von Neumann
- J. Dorgelo (Marvell) Terabit NAND Flash comes with advanced error correction
Open to all
It is FREE for all?Msc, PhD, PD and Professors in Micro-electronics, Computer engineering and Telecommunications. Don't forget to REGISTER?at?www.mest-delft.nlAdditional information ...
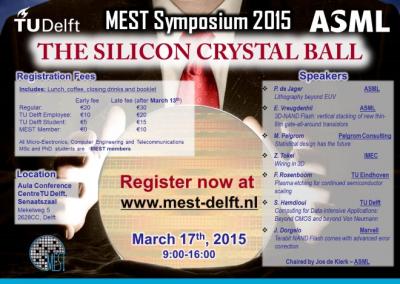
Signal Processing Seminar
- Thursday, 12 March 2015
- 13:30-14:30
- HB 14.240
Sparse covariance sensing using vector-sensor array
Shilpa Rao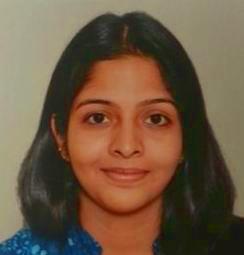
In directional-of-arrival (DOA) estimation of sources, vector sensors provide an edge over scalar sensors since they measure directional information in addition to the acoustic pressure. We study the covariance sensing problem for a sparse vector-sensor array using two techniques: using a quasi-stationary assumption on the sources, and using spatial smoothing in the correlation domain.
Additional information ...
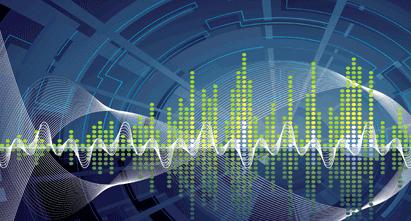
Sensor Data Fusion @ Fraunhofer FKIE: Surveillance and Protection for Defence and Security Applications
- Friday, 6 March 2015
- 09:00-10:00
- TBD
Fraunhofer FKIE, Bonn, Germany
Advanced algorithms to effectively exploit data streams from heterogeneous sources and optimally manage available sensor and unmanned platforms are of crucial importance. The talk will provide an overview of both, methodological work and advanced applications at Fraunhofer FKIE. We will place emphasis on exact track-to-track fusion, multistatic exploration and passive surveillance, aspects of resources management, and fusion tasks with unmanned aerial vehicles.
Signal Processing Seminar
- Thursday, 5 March 2015
- 13:30-14:30
- HB 14.240
A Krylov Subspace Approach to Modeling Wave Propagation in Open Domain
Jörn Zimmerling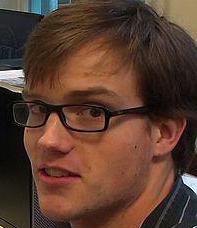
Simulating electromagnetic or acoustic wave propagation in complex open structures is extremely important in many areas of science and engineering. In a wide range of applications, ranging from photonics and plasmonics to seismic exploration, efficient wave field solvers are required in various design and optimization frameworks. In this talk, a Krylov subspace projection methodology is presented to efficiently solve wave propagation problems on unbounded domains. To model the extension of the computational domain to infinity, an optimal, frequency independent complex scaling method is introduced, that allows us to simulate wave propagation on unbounded domains provided we compute the propagating waves via a stability-corrected wave function. In our Krylov subspace framework, this wave function is approximated by polynomial or rational functions, which are obtained via Krylov subspace projection on Polynomial, Extended and Rational Krylov subspaces. In this talk we compare the convergence within these three Krylov subspaces. Further we show how symmetry relations in the finite difference approximation of wave equations can be used to efficiently construct Polynomial and Extended Krylov subspaces. Numerical examples illustrate the performance of the method and show that our Krylov resonance expansions significantly outperform conventional solution methods.
Additional information ...
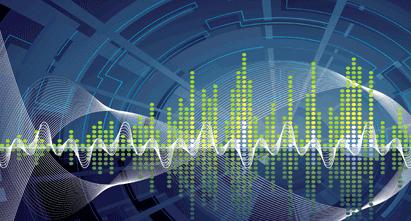
PhD Thesis Defence
- Thursday, 5 March 2015
- 12:00-11:30
- Aula Senaatszaal
Sensor management for surveillance and tracking. An operational perspective. March 5, 12.00 Aula, Senaatszaal. Promotor A. Yarovoy, co- promotor, H. Driessen
Fotios Katsilieris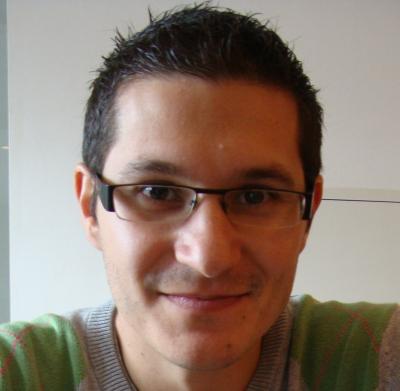
Defence, March 5, 12.00 Aula, Senaatszaal. Sensor management for surveillance and tracking. An operational perspective. In the literature, several approaches to sensor (including radar) management can be found. These can be roughly grouped into: a) rule-based or heuristics; b) task-based; c) information-driven; and d) risk/threat-based. These approaches are compared in this dissertation and it is found that there is not a single approach that is both Bayes-optimal and takes into account explicitly the user requirements in different operational contexts. In order to overcome the challenges with the existing approaches, this dissertation proposes managing the uncertainty in higher-level quantities (as per the JDL model) that are directly of interest to an operator and directly related to the operational goal of a radar system. The proposed approach is motivated by the threat assessment process, which is an integral part of defence missions. Accordingly, a prominent example of a commonly used higher-level quantity is the threat-level of a target. The key advantage of the proposed approach is that it results in Bayes-optimal sensor control that also takes into account the operational context in a model-based manner. In other words: a) a radar operator can select the aspects of threat that are relevant to the operational context at hand; and b) external information about the arrival of targets and other scenario parameters can be included when defining the models used in the signal processing algorithms, leading to context-adaptive sensor management.
Additional information ...
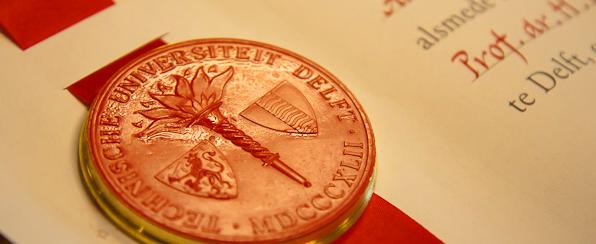
Signal Processing Seminar
- Thursday, 26 February 2015
- 13:30-14:30
- HB 14.240
Novel Symbol- and Frame-level Precoding Algorithms and Connections to Multicasting
Symeon ChatzinotasInterdisciplinary Centre for Security, Reliability and Trust - University of Luxembourg
Abstract: This seminar focuses on the latest advancements in MU-MISO downlink precoding. Two recent applications which target novel precoding scenarios are reviewed and connection to PHY-layer multicasting are revealed. More specifically, the first part addresses the concept of frame-based precoding which originates in practical standards dictating the grouping of multiple users in a single Forward Error Corrected frame. The second part reviews the field of constructive interference precoding, where the precoding takes place on a symbol-by-symbol basis taking into consideration both the user channel vector and its desired symbol. Finally, a number of promising but challenging open research topics are proposed.
Bio: Dr Symeon Chatzinotas (MEng, MSc, PhD, SMIEEE) received the M.Eng. in Telecommunications from Aristotle University of Thessaloniki, Greece and the M.Sc. and Ph.D. in Electronic Engineering from University of Surrey, UK in 2003, 2006 and 2009 respectively. He is currently a Research Scientist with the research group SIGCOM in the Interdisciplinary Centre for Security, Reliability and Trust, University of Luxembourg, managing H2020, ESA and FNR projects. In the past, he has worked in numerous R&D projects for the Institute of Informatics & Telecommunications, National Center for Scientific Research ?Demokritos,? the Institute of Telematics and Informatics, Center of Research and Technology Hellas and Mobile Communications Research Group, Center of Communication Systems Research, University of Surrey. He has authored more than 120 technical papers in refereed international journals, conferences and scientific books. His research interests are on multiuser information theory, cooperative/cognitive communications and wireless networks optimization. Dr Chatzinotas is the co-recipient of the 2014 Distinguished Contributions to Satellite Communications Award, Satellite and Space Communications Technical Committee, IEEE Communications Society. He is currently co-editing a book on "Cooperative and Cognitive Satellite Systems" to appear in 2015 by Elsevier and he is co-organizing the First International Workshop on ?Cognitive Radios and Networks for Spectrum Coexistence of Satellite and Terrestrial Systems? (CogRaN-Sat) in conjunction with the IEEE ICC 2015, 8-12 June 2015, London, UK.
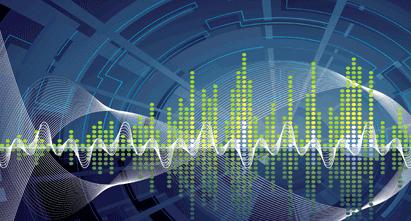
Signal Processing Seminar
- Thursday, 19 February 2015
- 13:30-14:30
- HB 14.240
A fast speech analysis method performing simultaneous high resolution voiced unvoiced detection and glottal closure/opening instant estimation.
Andreas Koutrouvelis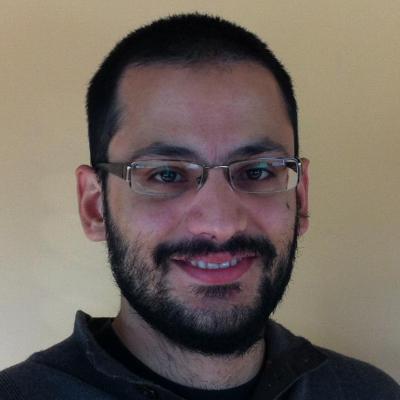
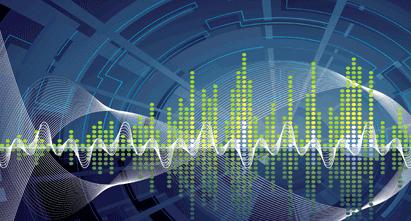
MEST event
- Tuesday, 17 February 2015
- 09:00-13:15
- Room J, 3ME Faculty
TU Delft in ISSCC 2015
Program:
9:00 | Prof. Kofi Makinwa | Welcome |
9:10 | A. Carimatto | A 67,392 SPAD PVTB-Compensated Multi-Channel Digital SiPMwith 432 column-Parallel 48ps 17b TDCs for Endoscopic Time-of-Flight PET |
9:50 | M. Shahmohammadi | A 1/f Noise Up-conversion Reduction Technique Applied to Class-D and Class-F Oscillators |
10:15 | R. Quan | A 4600um2 1.5oC (3s) 0.9kS/s Thermal-Diffusivity Temperature Sensor with VCO-Based Readout |
10:40 | Break | |
10:55 | L. Xu | A 110dB SNR ADC with +/-30V Input Common-Mode Range and 8uV offset for Current Sensing Applications |
11:35 | Y. He | A 0.05-mm2 1-V Capacitance-to-Digital Converter Based on Period Modulation |
12:00 | H.Jiang | A 30-ppm <80-nJ Ring-Down-Based Readout Circuit for Resonant Sensors |
There will be free pizza from 12:45 to 13:15
Signal Processing Seminar
- Thursday, 12 February 2015
- 13:00-14:00
- HB 14.240
Signal Processing Tools for Radio Astronomy
Millad Sardarabadi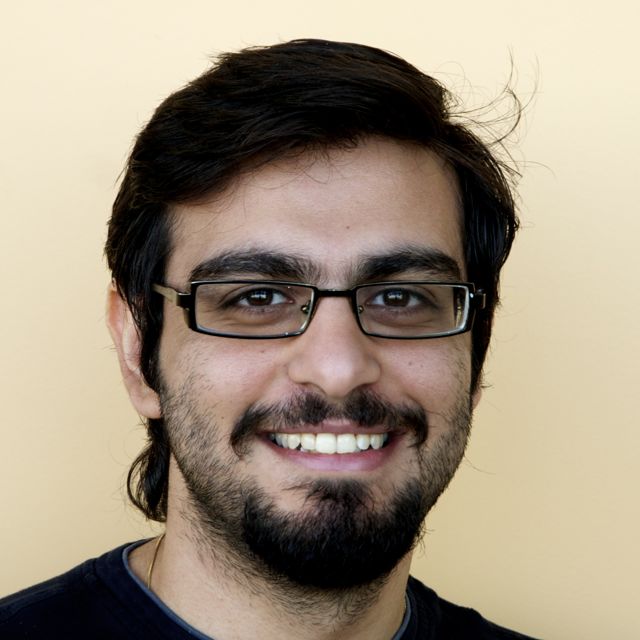
Millad will give an overview of "Signal Processing Tools for Radio Astronomy" that he have developed at Delft, he will cover the problems that arose and remaining challenges to be solved
Additional information ...
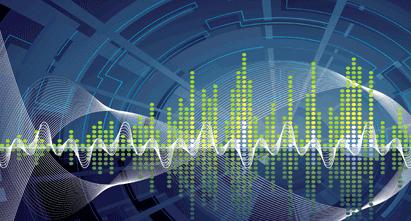
Signal Processing Seminar
- Thursday, 5 February 2015
- 13:30-14:30
- HB 14.240
Dynamic rainfall monitoring using attenuation measurements from telecommunication links.
Venkat Roy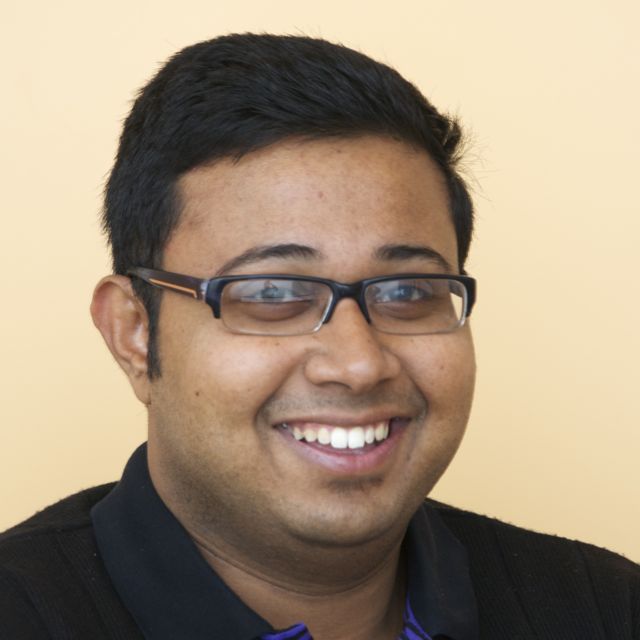
Venkat will present his work in this round of the Signal Processing Seminar.
Additional information ...
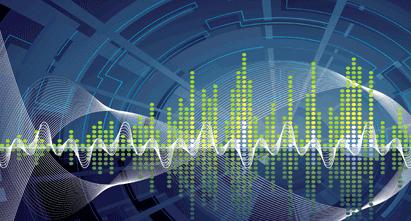
Signal Processing Seminar
- Thursday, 11 December 2014
- 15:45-16:15
- HB 14.230
Acoustic localization and ad-hoc beamforming
Nikolay Gaubitch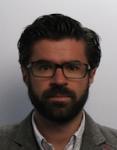
A demonstration of a combined acoustic localization and beamforming technology for multi-microphone speech enhancement applications will be given. A short discussion on the theory behind the technology will follow.
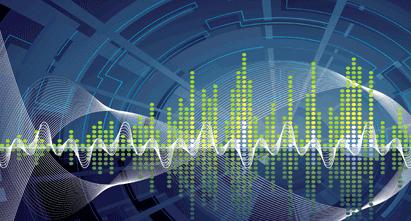
Signal Processing Seminar
- Thursday, 11 December 2014
- 14:35-15:05
- HB 14.230
Computational challenges in Mass Spectral Imaging
Raf Van de Plas3ME DCSC
Mass spectral imaging (MSI) is a novel imaging modality capable of concurrently measuring the spatial distribution of thousands of molecular species throughout an organic tissue section. This technique, also known as imaging mass spectrometry, has been gaining considerable attention in recent years with applications ranging from medicine to plant science and from material science to forensics. In this talk, we introduce the nature of MSI data with a specific focus on its high-dimensional aspects. We illustrate some of the low-level signal processing challenges using wavelet analysis and matrix factorization examples. High-level biological interpretation challenges are demonstrated using recent work on the automated anatomical interpretation of ion images.
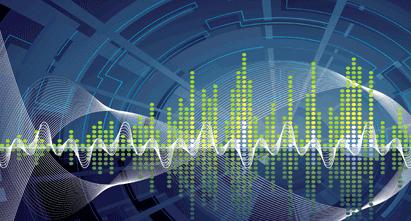
Signal Processing Seminar
- Thursday, 11 December 2014
- 15:05-15:35
- HB 14.230
Fiber-Optic Communication using Nonlinear Fourier Transforms
Sander Wahls3ME DCSC
When a signal travels through optical fiber, it evolves in a complicated way that is approximately described by the nonlinear Schroedinger equation. However, it turns out that the evolution of a solution to the nonlinear Schroedinger equation becomes simple when it is considered in the so-called nonlinear Fourier domain. This fact has recently started to attract attention in fiber-optic communications, where the idea has risen to encode information in the nonlinear Fourier domain instead of the time or the conventional Fourier domain. In this talk, the advantages of this concept will be explained. Recent results as well as open questions will also be discussed.
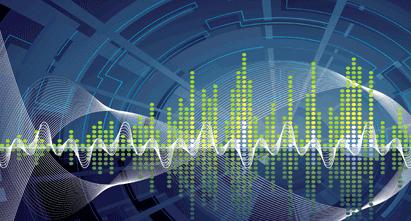
Signal Processing Seminar
- Thursday, 11 December 2014
- 14:00-14:35
- HB 14.230
Compressive and Sparse Sensing for Statistical Inference
Geert Leus, Alle-Jan van der Veen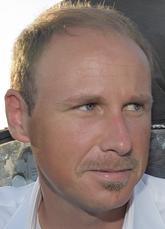
Geert Leus with an introduction by Alle-Jan van der Veen. Geert will talk about relevant research topics within our group.
Additional information ...
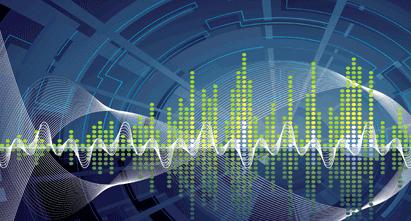
Signal Processing Seminar
- Thursday, 4 December 2014
- 13:30-14:30
- HB 14.230
Andrea's Talk
Andrea Pizzo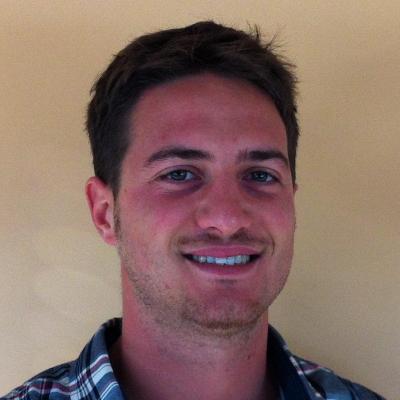
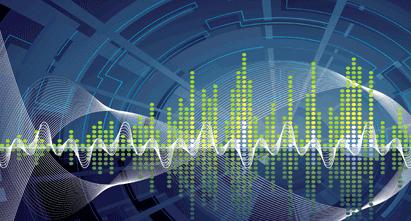
MSc CE Thesis Presentation
- Friday, 28 November 2014
- 10:00-11:00
- HB 14.230
Profiling of Polyhedral Process Networks
Wouter van Teijlingen
High-level synthesis (HLS) is a design method to raise the level of abstraction in the design of digital circuits. We use HLS to map sequential C-code to Polyhedral Process Networks (PPN), which are implemented in hardware. Designers need feedback on performance limitations as soon as possible, as going through the complete design flow to derive PPNs is time-consuming. The result is that only a few design points can be evaluated in a given amount of time.
In this work, we leverage previous research, and present cprof. Additionally, it estimates the performance of sequential C-code, when implemented as a PPN in hardware. Cprof estimates the execution finish time and the degree of parallelism of a PPN. Cprof provides assistance in Design Space Exploration (DSE), and Hierarchical Program Analysis (HPA) is used to profile programs with inter-procedural behavior.
We verified that cprof is capable of profiling and optimizing sequential C programs, which are realized in hardware as PPNs. We have also shown that on average, cprof overestimates the execution finish time of PolyBench/C benchmarks implemented in hardware by 0.44%. Cprof helps increasing engineering productivity by assisting in DSE, and risk is reduced by making design limitations explicit at an early stage in the design process. The result is that the hardware design flow looks like a regular software design flow, and no special hardware skills are required to analyze and optimize a design that is implemented as a PPN in hardware.
Additional information ...
Signal Processing Seminar
- Thursday, 27 November 2014
- 13:00-14:00
- HB 14.230
MCMC methods: Brief review and application to constrained blind deconvolution
Georg Kail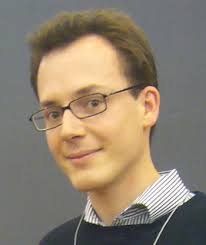
A brief and basic review of Markov chain Monte Carlo (MCMC) methods and an application where I have used them
Additional information ...
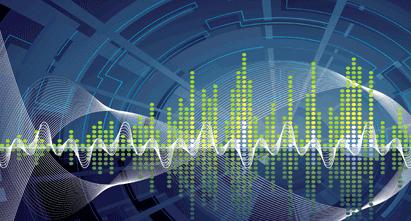
PhD Thesis Defence
- Monday, 24 November 2014
- 10:00-11:30
- Aula Senaatszaal
A GPS inspired terrain referenced navigation algorithm
Daniela Vaman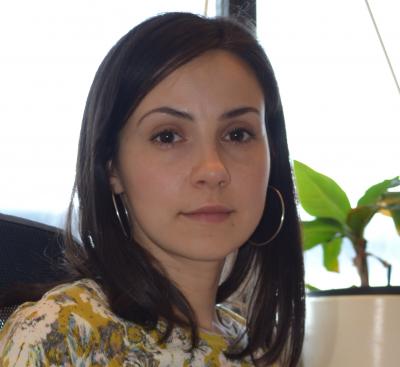
Terrain Referenced Navigation (TRN) refers to a form of localization in which measurements of distances to the terrain surface are matched with a digital elevation map allowing a vehicle to estimate its own position within the map. The main goal of this dissertation is to improve TRN performance through better signal processing. More specifically, the project aims to explore opportunities in the field of TRN by using digital signal processing techniques that were originally developed for the acquisition and tracking of GPS signals.
A typical TRN system uses speed, heading and time to establish the relative horizontal position between subsequent elevation measurements. Thus, any error in speed, heading or time will cause an error in the resulting relative position. If the speed or heading error contains a bias, this will cause a gradual reduction in the correlation. To prevent that a reduction in correlation causes the estimated position to drift away, the idea behind the research described in this thesis is the use of arrays of terrain elevation measurements with intentional (positive and negative) offsets in speed and heading in a tracking-loop configuration. It is well known that such a concept works well for optimized signals such as the ones used in GPS.
To further explore the viability of this idea for a signal defined by a series of terrain elevation measurements, an analysis of similarities and differences with the GPS signal is performed. In accordance to the GPS receiver approach, a novel correlation algorithm for TRN is proposed and implemented. The basic rationale for the algorithm is to use terrain correlation to ?acquire and track? the speed and heading of the host vehicle, while the position advances are calculated using these estimates together with the previously determined position. The novelty of the approach consists in the implementation of a tracking scheme based on the DLL concept. To answer feasibility-related questions, the algorithm is first evaluated in a purely theoretical framework. Based on this analysis it is concluded that the concept seems feasible and promising, but additional considerations in the design are required to compensate for the differences between the GPS and TRN signals. Enhancements are brought to the initial design resulting in the development of an adaptive tracking scheme, in which the tracking loops are configured based on an analysis of the terrain signal.
Next, an in-depth sensitivity analysis is carried out to understand how sensor measurement errors (in speed, heading and terrain height) impact the algorithm performance. The analysis is performed using exclusively simulated data. It is shown that sensitivity to speed and heading errors is dependent on terrain features and it is possible to assess the degree of sensitivity by analysing the terrain signal. By combining this information with the expected error characteristic of the navigation sensors, the performance of the algorithm can be predicted. The sensitivity to terrain measurement errors depends on the ratio between the terrain signal strength and the measurement errors. It is shown that this ratio can be predicted up to a certain extent and a method to improve the ratio is proposed and discussed.
The developed capabilities are validated with recorded sensor data from flight tests. Two different types of recorded sensor data are used: radar and lidar based datasets.
Additional information ...
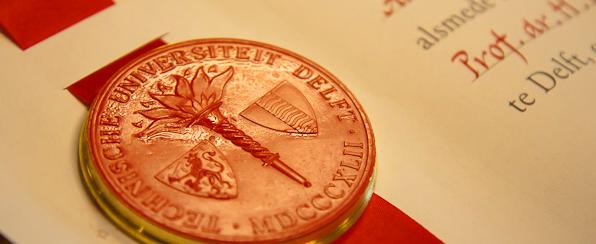
Signal Processing Seminar
- Thursday, 20 November 2014
- 13:30-14:30
- HB 14.230
An experimental setup for UWB indoor positioning system
Yan Xie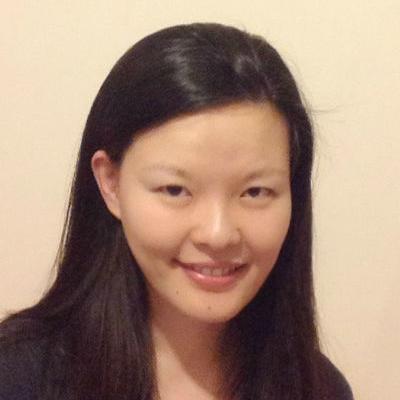
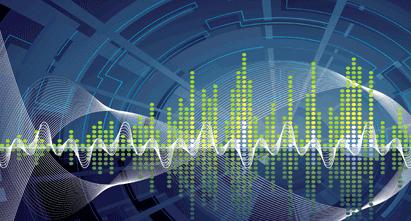
TU Delft best graduate ceremony
- Thursday, 13 November 2014
- 15:30-17:30
- Aula auditorium
Final competition
Jörn Zimmerling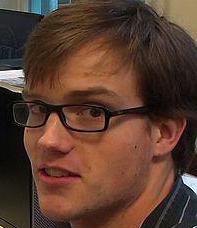
The best graduates from each faculty (for EWI: Jorn Zimmerling, member of CAS) will try to convince the jury that they are the Best Graduate 2014 by shortly presenting their graduation project. Together with a professional film crew, the study associations of the faculties made short movies about why their each lecturer deserves the ?Best Lecturer TU Delft 2014? title. Once again, it promises to be a festive celebration of the people who make our education special. The best graduates and best lecturers count on your support!
Additional information ...
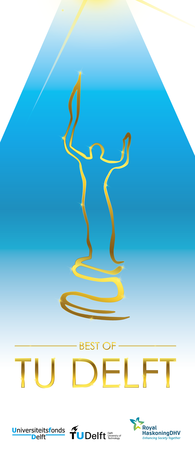
Signal Processing Seminar
- Thursday, 6 November 2014
- 13:30-14:30
- HB 17.150
Near-field beamforming for ad-hoc microphone arrays
Nikolay Gaubitch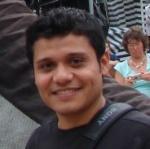
We will give a short talk and demonstration of an autolocalizing beamformer for ad-hoc networks that we developed.
Additional information ...
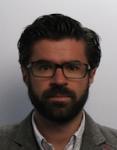
Signal Processing Seminar
- Thursday, 30 October 2014
- 13:30-14:30
- HB 14.230
Differential Received Signal Strength-Based Localization with Unknown Path-Loss Model
Yongchang Hu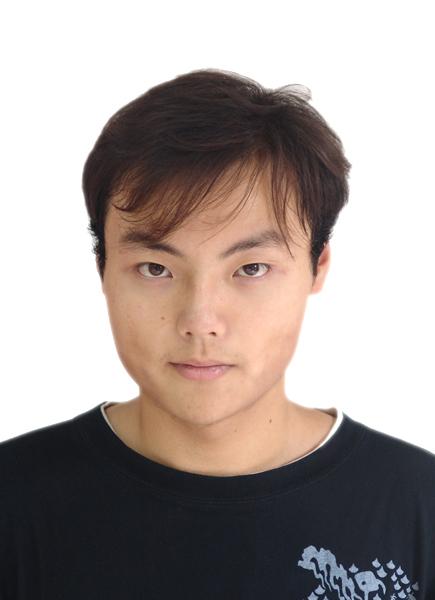
We present and investigate a new decorrelated model for the DRSS-based localization, on which all the proposed estimators in this paper are based. Then when assuming that the PLE is known, three different kinds of estimators for the DRSS-based localization are introduced: the advanced best linear unbiased estimator (A-BLUE), the semidefinite programing (SDP)-based estimators (SDPE) and the exact estimators (EE). The simulation results show that all the proposed DRSS-based estimators are able to outperform a jointly least squares RSS-based estimator (JLS-RSS) which estimates the unknown transmit power and the target location altogether. Furthermore, they are also shown having a better performance than a recent weighted least squares DRSS-based estimator (WLS) which particularly requires a perfect knowledge of the variance of the shadowing effect. Besides besting the existing methods, the three proposed estimators have their own advantages from different perspectives: the A-BLUE has the lowest computational complexity; the EE holds the best accuracy in a small shadowing environment; the SDPE yields the best performance to endure a large shadowing effect and possesses a very good tolerance, e.g., of using an imperfect PLE. Some more theoretical analyses and comparative discussions are also presented. Finally, based on the studies above, we propose a SDP-based modified block coordinate descent estimator (SDP-MBCDE) to deal with the case when the PLE also becomes unknown. The SDP-MBCDE jointly estimates the unknown PLE and the target location iteratively and, with an increasing iteration number, its performance approaches that of the SDPE using a perfectly-known PLE. In a nutshell, we present a thorough study on the DRSS-based localization based on our proposed decorrelated model. To meet different practical demands when encountering different situations, different proposed estimators are provided as options. Additionally, all the proposed estimators and the corresponding analyses are expected of any help, not only to the DRSS-based localization, but also to the source localization in general or some similar optimization problems.
Additional information ...
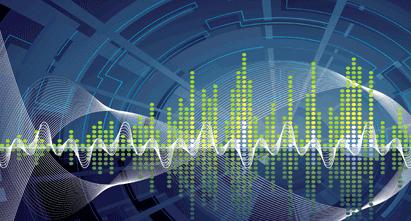
Signal Processing Seminar
- Thursday, 23 October 2014
- 12:30-13:30
- HB 14.230
Like-Tex : Simplify the writing of your PhD thesis (and all your other scientific documents)
Seyran Khademi, Andrea Simonetto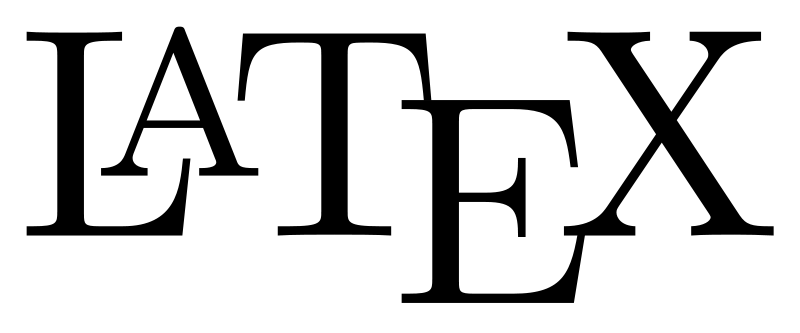
During her PhD the candidate is required to write high-quality scientific documents. To achieve this, Latex and its ecosystem of tools constitute a very powerful yet difficult to learn tool.
In this special session of the SP Seminar we will cover the use of Latex and some of its related tools. Getting to know these tools will allow the user to considerably simplify the workload inherent in writing high-quality scientific documents. In this session we will focus on writing the PhD thesis, but the tips and ideas we give can of course be used for writing documents at any level. Anyone interested is therefore welcome.
The presentation will be given during lunch time, including pizza and mexican burritos. So if you are interested please fill in your name in this doodle so that we can estimate how much food we need to order:
http://doodle.com/sdyswx7idgktuydd
See you all there!
Seyran, Andrea and Jorge.
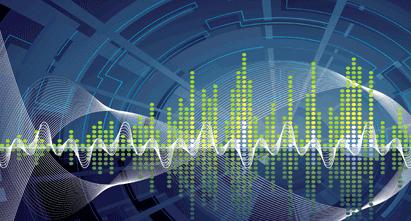
SP mini symposium
- Thursday, 16 October 2014
- 15:00-16:00
- van der Poelzaal (LB 01.220)
Distributed time-varying optimization
Andrea Simonetto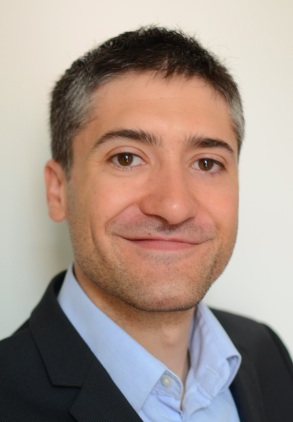
Abstract: We devise a distributed asynchronous stochastic epsilon-gradient-based algorithm to enable a network of computing and communicating nodes to solve a constrained discrete-time time-varying stochastic convex optimization problem.
Additional information ...
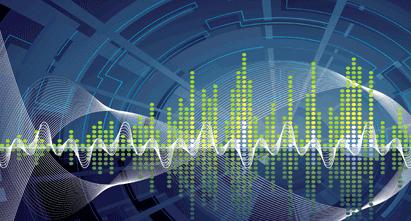
SP Mini symposium
- Thursday, 16 October 2014
- 14:00-15:00
- van der Poelzaal (LB 01.220)
Where am I? An experiment in Indoor Localization
Prof. K.V.S. HariDepartment of ECE, Indian Institute of Science, Bangalore
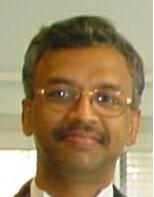
Abstract: Indoor Localization of people (or objects), where GPS is not available, is an interesting problem with several applications. Several solutions exist, of which, positioning based on WiFi, Video-Tag identification, Ultra Wideband signals and Inertial sensors, are a few examples. In this talk, we consider a scenario where a First-Responder team enters a building after a disaster and the position of each member of the team needs to be known to the control center outside the disaster-affected building. Specifically, we will discuss how an Inertial Navigation System (INS) embedded in a shoe can be designed, to address this problem.
Brief Bio: K.V.S. Hari received the B.E., M.Tech and PhD(1990) degrees from Osmania University, IIT Delhi, University of California at San Diego, respectively. Since 1992, he has been a Faculty Member at the Department of ECE, Indian Institute of Science (IISc), Bangalore, where he is currently a Professor and coordinates the activities of the Statistical Signal Processing Lab in the department. Currently, he is also an Affiliated Professor in the Department of Signal Processing, KTH-Royal Institute of Technology, Stockholm. His current research and development interests include MIMO Wireless Communication, Sparse signal Processing, Indoor Localization and Assistive technologies for the Elderly.
International Radar Conference 2014, Lille, France
- Monday, 13 -- Friday, 17 October 2014
- Lille, France
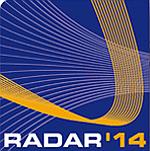
The French SEE Society (Soci?t? de l'Electricit?, de l'Electronique, et des Technologies de l'Information et de la Communication) organises RADAR 2014 in Lille, from 13 to 17 of October 2014. The conference will be organized in the frame of the international relations set up between the Institution of Engineering and Technology (IET), the Institute of Electrical and Electronics Engineers (IEEE), the Chinese Institute of Electronics (CIE), the Institution of Engineers Australia (IEAust) and the SEE.
Additional information ...
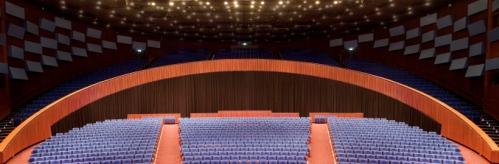
Signal Processing Seminar
- Thursday, 9 October 2014
- 14:00-15:00
- LB01.170 Timmanzaal
Joint antenna selection and precoding with quadratic sparsity inducing regularizer for multi-user MIMO systems
Seyran Khademi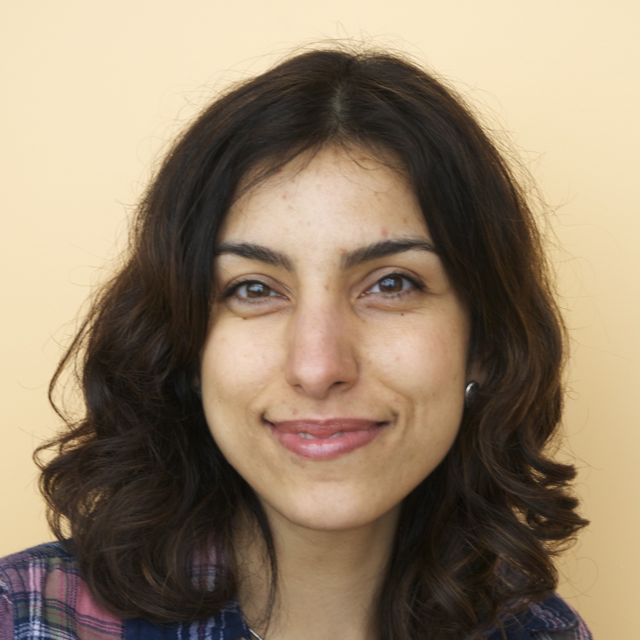
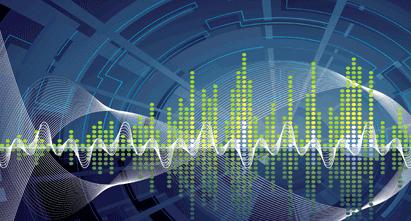
MEST Colloquium
- Thursday, 9 October 2014
- 12:30-13:30
- EWI Chip
Electronics in Nano-Era: Are we Facing a Reliability Wall?
Said Hamdioui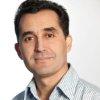
The talk will address technology scaling and its impact on different aspects of IC and electronics, and in particular the emerging reliability bottlenecks. First the basics of scaling will be covered, together with its impact on integration density, performance and power. The technology outlook will be analyzed in order to extract the challenges with respect to design, test and reliability both for near and long terms. IC realization process will be (re) defined while considering the technology trends and business pressure. Possible ways for the realization of future systems will be discussed.
MSc ME Thesis Presentation Menno Vastenholt
- Monday, 29 September 2014
- 10:00-11:00
- EWI Building, LB01.150 (Lipkenszaal)
A Sub-GHz UWB Correlation Receiver for Wireless Biomedical Communication
Menno Vastenholt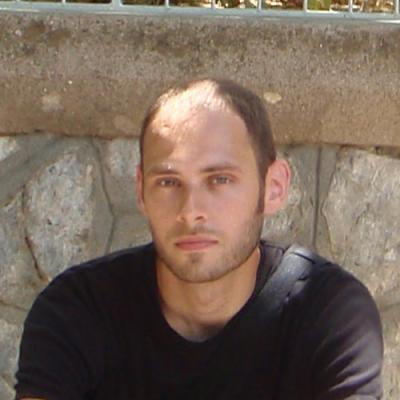
Receivers Topology Optimization of the Combined Active and WiFi-based Passive Radar Network
- Friday, 26 September 2014
- 10:00-10:30
- Snijderszaal (LB 01.010)
Presentation for the EuRAD?14 conference
Inna Ivashko
This paper focuses on the accuracy analysis of the combined active and WiFi-based passive radar network. The Cramer-Rao Lower Bound is used as an accuracy metric. It is shown that localization performance of the active radar network can be improved with exploitation of the signals from passive bistatic WiFi radars. This makes reasonable to use information from passive and active radars simultaneously in order to enhance system localization capability. Sparsity-based algorithm is applied to find optimum geometry of the WiFi receivers at the fixed positions of the WiFi access points and active radars.
Additional information ...
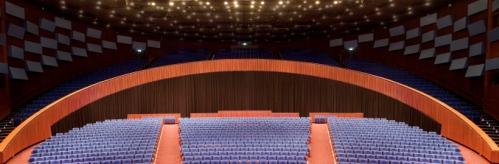
Signal Processing Seminar
- Thursday, 25 September 2014
- 11:00-12:00
- HB 17.150
Indoor granular presence sensing with an ultrasonic circular array sensor
Shahrzad Naghibzadeh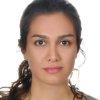
Shahrzad will talk about a paper she is preparing based on her Msc. work.
Additional information ...
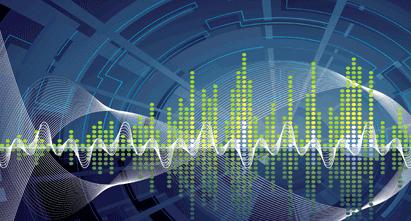
MSc CE Thesis Presentation
- Thursday, 25 September 2014
- 14:00-14:45
- HB 17.150
Guaranteed Quality ECG Signal Compression Algorithm
Dongni FanThe aim of the project is to develop an ECG signal compression algorithm that has a high compression ratio while guaranteeing signal quality.
An electrocardiography (ECG) signal is a representation of cardiac activity and has an need to be compressed to reduce data storage requirements. Previous ECG signal compression techniques have shown steady improvement on compression ratio. However, these techniques generally lack quality considerations, so their applications are limited. We present a discrete cosine transform (DCT) based compression scheme and use beat detection which considerably improve the compression ratio. The quality of the compressed signal is configurable, and the accuracy of the signal is maintained given a signal quality requirement.
The algorithm is implemented in a software/hardware solution. Some parts need to be done in the software. As a proof of concept, we have chosen the filter to be implemented in hardware. Mathworks HDL coder was used for generating RTL code and testbenches. Results show that our algorithm is capable of maintaining the specified quality, has a better compression ratio compared to previous work and is also capable to compress noisy ECG signals.
Additional information ...
EI Colloquium
- Monday, 22 September 2014
- 11:00-12:00
- EWI Snijderszaal
Analog-to-digital converters
Jesper SteensgaardLinear Technology, Milpitas, CA
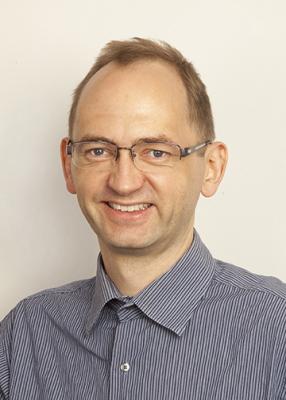
Analog-to-digital converters have traditionally been a weak link in mixed-mode signal chains. As such, logarithmic and programmable-gain amplifiers have been used to effectively increase their overall dynamic range. In recent years, however, ADC performance has dramatically improved, making it difficult to design amplifiers and references capable of matching their performance. This talk will discuss the challenges of designing a circuit capable of driving a 20-bit SAR ADC with better than 1-part-per-million accuracy.
Biography
Jesper Steensgaard, obtained his MSEE and then his PhD from the Technical University of Denmark in 1999. He has 20+ years of experience in the design of high-resolution data converters. His early work focused on delta-sigma data converters, including mismatch-shaping binary-weighted-element DACs and continuous-time delta-sigma ADCs. Recently, Jesper developed a family of high-resolution low-power SAR ADCs, including the LTC2378-20, which combine the best features of delta-sigma ADCs (precision, low noise) and SAR ADCs (speed, low power, ease of use).
MSc CE Thesis Presentation
- Monday, 22 September 2014
- 14:00-15:00
- HB 17.150
CacheBalancer: A communication latency and utilization aware resource manager
Jurrien de KlerkAs the number of processors increases in today's many-core processors, new issues regarding memory management arises. Performance of many-core processors, including large numbers of processors, is often limited by the communication latency due to transfer of data from one node to another. Conventional dynamic memory allocators are unaware of the communication costs, and do not consider what data is send between nodes due to memory allocation. Existing proposals that address this issue result in a limited number of utilized memory resources, potentially leading to over utilized memory resources.
This work introduces a technique for dynamic memory allocation, where state-of-the-art is improved to overcome the limited utilization of the memory resources. The proposed memory allocation method measures the utilization of the different memory resources and uses this information to determine which memory section should be assigned to a requesting task.
This work demonstrates that the proposed memory allocation scheme can reduce memory access latency up to 63.4%, by avoiding the allocation of memory that maps to over utilized resources. The memory allocation scheme is implemented in a run-time manager called Cache Balancer. In addition, the Cache Balancer includes a task mapping algorithm that combines information on the tasks themselves with information communication costs, to map task such that memory throughput is improved. The task mapping algorithm showed a further reduction of memory access latency of 14.5%.
Additional information ...
Quantum imaging seminar
- Thursday, 18 September 2014
- 13:30-15:00
- HB 17.150
Quantum Nonlinear Optics in Integrated Devices
Marco LiscidiniUniversity of Pavia, Italy
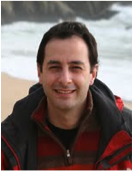
There is a burgeoning interest in the study of parametric fluorescence in integrated devices to obtain compact and efficient sources of non-classical states of light, which are necessary toward the full integration of quantum optical devices. As in the case of quantum linear optics, the use of microstructures gives the opportunity for investigating new phenomena that would be hardly observable in larger bulk-crystal sources. Yet, integrated sources present also several challenges, such as their efficient and fast characterization, which is typically quite demanding because of their low external brightness. In this talk, I will review all these points, starting with the demonstration of silicon microring resonators as CMOS compatible room-temperature sources of time-energy entangled photon pairs.
About the speaker
Marco Liscidini received the Ph.D degree in physics from the University of Pavia (Italy) 2006, working in the group of Prof. Lucio Andreani, with a dissertation entitled "Nonlinear optical properties of planar microcavities and photonic crystal slabs". From 2007 to 2009, he was Post-Doctoral Fellow in the group of Prof. John E. Sipe at the Department of Physics of the University of Toronto, Canada. From 2009 to 2013 is research scientist at the University of Pavia. He is currently tenure-track Assistant Professor at the Department of Physics of the University of Pavia. Since October 2011 is professor of Photonics at the Department of Physics of the University of Pavia. His research activity is focused on the theoretical study and modeling of light-matter interaction in micro- and nano?structures. He works in several areas of photonics, including classical and quantum nonlinear optics, spontaneous emission, plasmon and QW-exciton polaritons, optical sensing and bio-sensing, and photovoltaic effects. He is coauthor of more than 50 papers in peer-reviewed journals. His theoretical research activity is in strong collaboration with experimental groups and in the framework of national, European, and Canadian research programs.
Signal Processing Seminar
- Thursday, 18 September 2014
- 16:00-17:00
- HB 17.150
Sparse Sensing for Statistical Inference Tasks
Sundeep Prabhakar Chepuri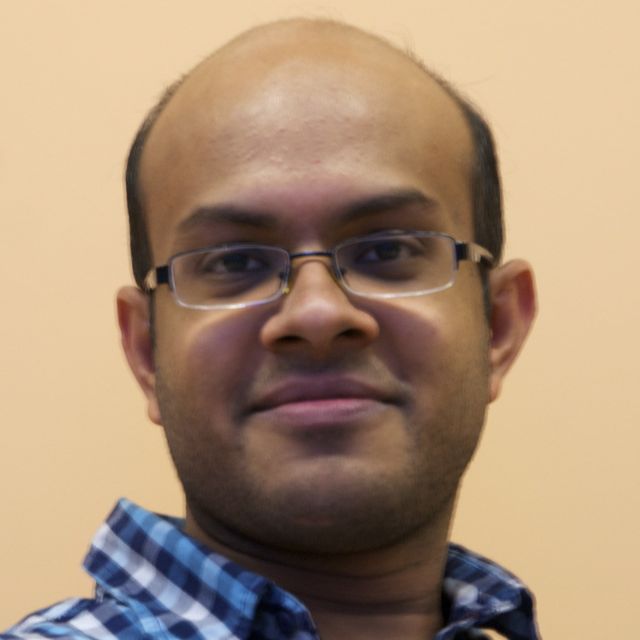
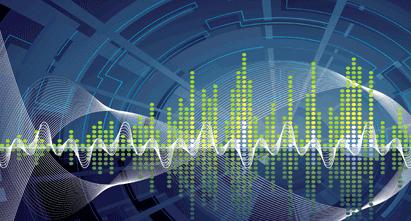
Signal Processing Seminar
- Thursday, 11 September 2014
- 16:00-17:00
- HB 17.150
Recent advances
Amir LeshemBar-Ilan University (Israel)
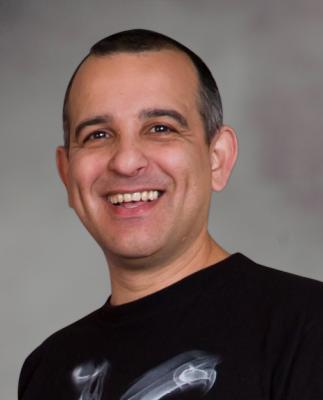
Amir is visiting Delft this week and will talk about his research progress. Amir has been a postdoc and visiting professor at CAS in the past, for in total several years.
Additional information ...
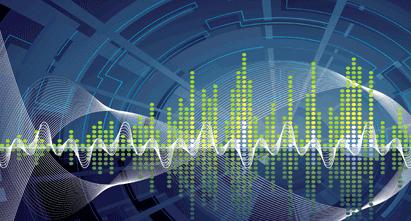
DIMES colloquium
- Wednesday, 10 September 2014
- 14:00-16:00
- Dimes colloquiumroom
Probabilistic Design for Reliability in Electronics and Photonics: Role, Attributes, Challenges
Prof.dr. Ephraim SuhirPortland State University, USA
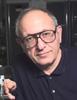
The recently suggested probabilistic design for reliability (PDfR) concept is based on:?1) highly focused and highly cost-effective failure oriented accelerated testing (FOAT),??aimed at understanding the physics of the anticipated failures and at quantifying, on the probabilistic basis, the outcome of FOATs conducted for the most vulnerable element(s) of the product of interest and the most likely and meaningful combination of possible stressors (the principle of superposition does not work in reliability engineering), and? 2) simple and physically meaningful predictive modeling (PM), both analytical and computer-aided, aimed at bridging the gap between what one "sees" as a result of FOAT and what he/she will supposedly "get" in the field. FOAT and PM based sensitivity analysis (SA) algorithms are developed as by-products.
The PDfR concept is based on the recognition of the fact that nobody and nothing is perfect, and that the difference between a highly reliable and insufficiently reliable product is ?merely? in the level of its probability of failure. If this probability (evaluated for the anticipated loading conditions and the given time in operation) is not acceptable, then such a SA can be effectively employed to determine what could be possibly changed, in terms of materials, geometries, application restrictions, etc., to improve the situation.
The PDfR analysis enables one also to check if the product is not "over-engineered", i.e., is not superfluously robust: if it is, it might be too costly: although the operational reliability cannot be low, it does not have to be higher than necessary either, but has to be adequate for the given product and application. This means that when both reliability and cost-effectiveness are imperative, ability to quantify reliability is a must. In this seminar the major PDfR concepts will be illustrated by case studies and practical examples. Although some advanced and subtle PDfR predictive modeling techniques have been recently developed for quantifying and assuring reliability of electronic and photonic products, especially those intended for aerospace applications, the practical examples addressed employ more or less elementary analytical models.
Biography
Prof. Dr. E. Suhir is Fellow of ASME, IEEE, American Physical Society (APS), Institute of Physics (UK), Society of Optical Engineers (SPIE), International Microelectronics and Packaging Society (IMAPS), Society of Plastics Engineers (SPE), Foreign Full Member (Academician) of the NAE, Ukraine, and Fulbright Scholar in Information Technologies. He has authored above 300 publications (patents, books, book chapters, papers) and received numerous professional awards, including 2004 ASME Worcester Read Warner Medal for outstanding contributions to the permanent literature of engineering and laying a foundation of a new discipline ?Structural Analysis in Electronics and Photonics Systems?. Dr. Suhir is the third Russian American, after Steven Timoshenko and Igor Sikorsky, who received this prestigious award. Dr. Suhir is co-founder of the ASME Journal of Electronic Packaging and served as its Technical Editor for eight years (1994-2002).Additional information ...
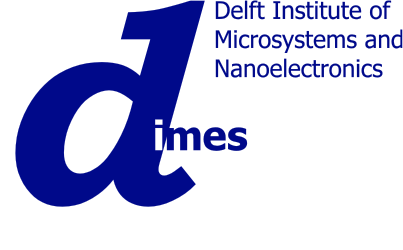
- Wednesday, 10 September 2014
- 17:00-19:00
- /pub (EWI basement)
MEST welcome drink

Meet and greet your friends and colleagues with a FREE Drink to say Hallo !!!
Organized by MEST student association
Additional information ...
MSc CE Thesis Presentation
- Friday, 29 August 2014
- 14:00-15:00
- HB 17.150
Multi-chip dataflow architecture for massive scale biophysically accurate neuron simulation
Jacco HofmannThe ability to simulate brain neurons in real-time using biophysically-meaningful models is a critical pre-requisite grasping human brain behavior. By simulating neurons behavior, it is possible, for example, to reduce the need for in-vivo experimentation, to improve artificial intelligence and to replace damaged brain parts in patients.
A biophysically accurate but complex neuron model, which can be used for such applications, is the Hodgkin-Huxley (HH) model. State of the art simulators are capable of simulating, in real-time, tens of neurons, at most. The currently most advanced simulator is able to simulate 96 HH neurons in real-time. This simulator is limited by its exponential growth in communication costs.
To overcome this problem, in this thesis, we propose a new system architecture, which massively increases the amount of neurons which is possible to simulate. By localizing communications, the communication cost is reduced from an exponential to a linear growth with the number of simulated neurons As a result, the proposed system allows the simulation of over 3000 to 19200 cells (depending on the connectivity scheme). To further increase the number of simulated neurons, the proposed system is designed in such a way that it is possible to implement it over multiple chips. Experimental results have shown that it is possible to use up to 8 chips and still keeping the communication costs linear with the number of simulated neurons. The systems is very flexible and allows to tune, during run-time, various parameters, including the presence of connections between neurons, eliminating (or reducing) resynthesis costs, which turn into much faster experimentation cycles. All parts of the system are generated automatically, based on the neuron connectivity scheme.
A powerful simulator that incorporates latencies for on and off chip communication, as well as calculation latencies, can be used to find the right configuration for a particular task. As a result, the resulting highly adaptive and configurable system allows for biophysically accurate simulation of massive amounts of cells.
MSc ME Thesis Presentation
- Friday, 29 August 2014
- 11:00-12:00
- HB 17.150
Long-range 3D Range Detector Based on Time-correlated Single-photon Counting
Dali Zhang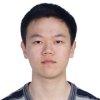
Three-dimensional (3D) range detectors enabling 3D computer vision is now popular in automotive industry. With their participation, automobile safety has been further enhanced, autonomous driving has become realizable. Time-correlated single-photon counting (TCSPC) technique utilizing complementary metal-oxide semiconductor (CMOS) single photon detectors (SPDs) and time-to-digital converters (TDCs) embodies the proper participant of automotive 3D vision, with low power consumption, low cost, high speed, high robustness, small size, and portability.
In this thesis, a TCSPC 3D range detector for automotive application was studied and modeled. The model covered all main components of a TCSPC system, including the TCSPC range detection process, the signal, and the noise. It was designed to predict the behavior of TCSPC systems and help future designers optimize the performance in accordance with the targeted application.
To verify the model, a experimental setup was designed, implemented, and characterized. The setup consists of a data acquisition system, data processing procedures, and an optical-mechanical system. Measurements performed using the setup have confirmed that the model was designed correctly. For further exploration, range detection from 0.2 m to 60 m were carried out.
- Wednesday, 27 August 2014
- 14:00-15:00
- HB 17.150
CMOS-based implantable electronics for bioscientific and medical applications
Takashi TokudaNAIST, Japan
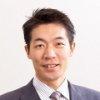
CMOS-based implantable device technology is attracting a lot of interest because of its potential for next-generation bioscientific and medical applications. In this presentation, circuit design, device packaging, and functional demonstration of some CMOS-based implantable devices are presented. An implantable imaging device for in vivo (in a living body) optical brain imaging, implementation of light source for neural stimulation in optogenetics, and flexible neural stimulator for retinal prosthesis will be mainly described.
Additional information ...
MSc SS Thesis Presentation
- Thursday, 17 July 2014
- 14:00-15:00
- LB 01.220 (vdPoelzaal)
Speech Production Modelling and Analysis
Andreas Koutrouvelis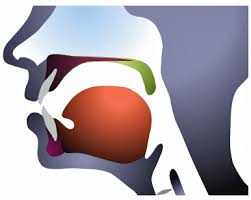
The first part of the present thesis reviews the speech production mechanism and several models of the glottal flow derivative waveform and of the vocal tract filter. The source filter model is investigated in depth, since it is the most important "ingredient" of linear prediction analysis. We also review seven linear prediction (LP) methods based on the same general LP optimization framework. Moreover, we examine the importance of pre-emphasis and glottal-cancellation prior to LP.
The second part of the thesis, provides an experimental evaluation of the LP methods combined with several pre-emphasis and glottal-cancellation techniques in the context of two general application areas. The first area consists of applications which aim to estimate the true glottal flow or glottal flow derivative signal. The second area consists of applications which aim to find a sparse residual. In particular, five factors are investigated: the sparsity of the residual using the Gini index, the estimation accuracy of the glottal flow derivative using the signal to noise ratio (SNR), the estimation accuracy of the vocal tract spectral magnitude using the log spectral distortion distance (LSD) metric, and the probability of obtaining a stable linear prediction filter. All these factors are evaluated for clean and reverberated speech signals. The sparse linear prediction methods and the iteratively reweighted least squares method combined with the second order pre-emphasis filter give the most accurate glottal flow derivative estimates, the most accurate vocal tract estimates and the sparsest residuals in most cases. Finally, we compare several linear prediction methods in the context of the speech dereverberation method proposed in [1, 2]. This method enhances the reverberated residual obtained via the autocorrelation method. In the context of this application, we show that the sparse linear prediction method and the weighted linear prediction method combined with a second-order pre-emphasis filter perform better than the autocorrelation method.
MSc TC Thesis Presentation
- Thursday, 17 July 2014
- 16:00-16:45
- HB 17.150
Network Coding in Underwater Communications
Elvin Isufi
MSc TC Thesis Presentation
- Wednesday, 16 July 2014
- 14:00-14:45
- HB 17.150
Indoor Granularity Presence Sensing and Control Messaging with an Ultrasonic Circular Array
Shahrzad Naghibzadeh
MSc ME Thesis Presentation
- Friday, 4 July 2014
- 13:00-13:45
- Lipkenszaal
Development of a Multichannel TCSPC System in a Spartan 6 FPGA
Harald HomulleFor the master project work was carried out for the development of a fluorescence lifetime imaging probe for fluorescence guided surgery. For this project a prototype was designed. The work on the prototype was divided into three main parts, hardware, firmware / software, and system / optics. In this thesis the firmware / software of the system are described. An overview of the system is given and the performance is evaluated.
Additional information ...
PhD Thesis Defence
- Tuesday, 1 July 2014
- 10:00-11:30
- Aula Senaatszaal
Multichannel Digital Silicon Photomultipliers for Time-of-Flight PET
Shingo Mandai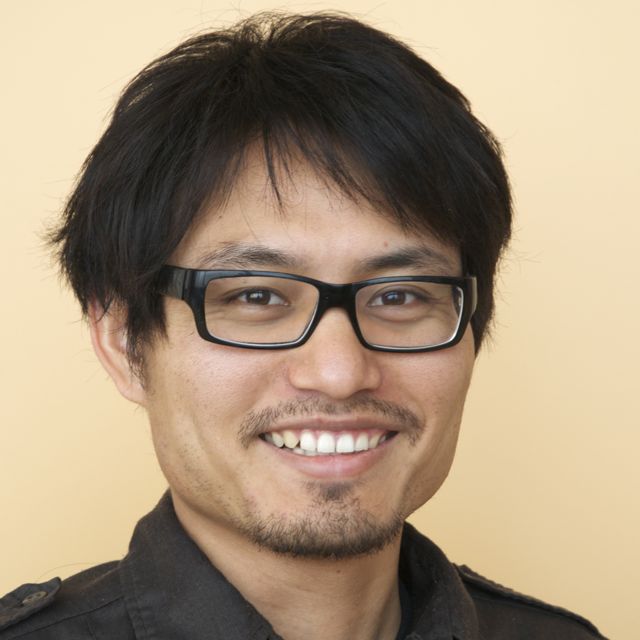
This thesis discusses the potential of CMOS based SiPMs, especially for TOF PET applications, in a systematic and comprehensive fashion. CMOS based SPADs are still need to be designed carefully to improve fill factor, TDCs be improved from the point of the area and power consumption, and the necessity of high voltage for SPADs be handled efficiently. Thus, this thesis also aims to design and integrate various circuits in the SiPM to realize the high integrations utilizing the biggest advantage of the CMOS technology.
Additional information ...
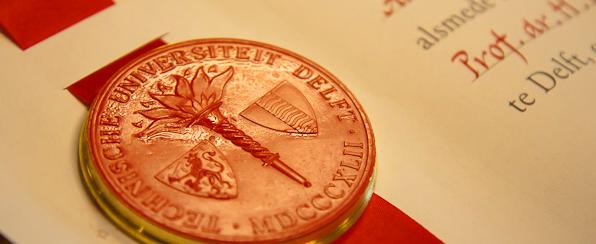
PhD Thesis Defence
- Thursday, 19 June 2014
- 15:00-17:30
- Aula Senaatszaal
Compressive Sampling for Wireless Communications
Shahzad Gishkori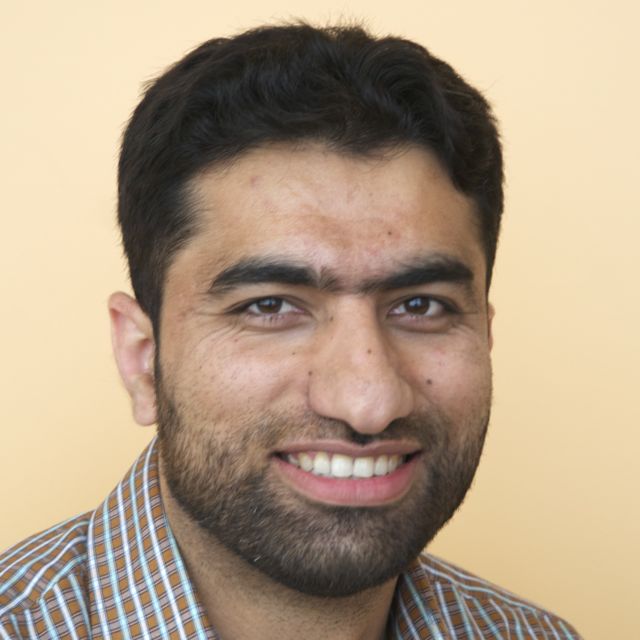
Wireless communications is undergoing massive development in all forms of its manifestations. In the field of short-range communications, technologies like ultra-wideband (UWB) systems are promising very high data rates, fine timing resolution and coexistence with other physical layer standards. Along with these benefits, the promise of low cost and low complexity devices makes UWB systems a highly sought-after option. The main reason for these benefits is the utilization of a very large bandwidth. However, these benefits come at a price, that is the high sampling rate required to receive such signals. According to the Nyquist sampling theorem, a signal can be fully determined if sampled at twice its maximum frequency. This means that the UWB signals may require a sampling rate in the order of Giga samples per second. At the receiver, the sampling is carried out by an analog-to-digital converter (ADC). The power consumption of an ADC is proportional to its sampling rate. A very high sampling rate means stressing the ADC in terms of power consumption. This can put the whole idea of low cost and low complexity UWB systems in jeopardy. Therefore, using subsampling methods is indispensable. In this regard, we propose the utilization of compressive sampling (CS) for UWB systems. CS promises a reasonable reconstruction performance of the complete signal from very few compressed samples, given the sparsity of the signal. In this thesis, we concentrate on impulse radio (IR) UWB systems. IR-UWB systems are known to be sparse, meaning, a large part of the received signal has zero or insignificant components. We exploit this time domain sparsity and reduce the sampling rate much below the Nyquist rate but still develop efficient detectors.
We propose CS based energy detectors for IR-UWB pulse position modulation (PPM) systems in multipath fading environments. We use the principles of generalized maximum likelihood to propose detectors which require the reconstruction of the original signal from compressed samples and detectors which skip this reconstruction step and carry out detection on the compressed samples directly, thereby further reducing the complexity. We provide exact theoretical expressions for the bit error probability (BEP) to assess the performance of our proposed detectors. These expressions are further verified by numerical simulations.
We also propose CS based differential detectors for IR-UWB signals. These detectors work on consecutive symbols. We develop detectors with separate reconstruction and detection stages as well as detectors that perform these steps jointly. We further present detectors which do not need reconstruction at all and can work directly on the compressed samples. However, this can put some limitations on the overall flexibility of the detector in terms of the measurement process. To assess the performance of all these detectors, we also provide maximum a posteriori (MAP) based detectors. We provide numerical simulations to display the detection results.
We extend the CS based classical differential detectors to the case of multiple symbol differential detectors. To keep the implementation complexity at its minimum, we work only with compressed samples directly. We use the principles of the generalized likelihood ratio test (GLRT) to eliminate the limitations on such detectors, in terms of the measurement process. Apart from focusing on compressed detectors which contain full timing information, we also propose detectors which need such information at symbol level only. This effectively results in low cost and low complexity detectors.
Finally, we present some work on the theoretical aspects of CS. We develop algorithms which exploit the block sparse structure of the signal. This block sparsity is combined with varying block sizes and signal coefficients having smooth transitions. Such signals are often encountered in a wide range of engineering and biological fields.
Additional information ...
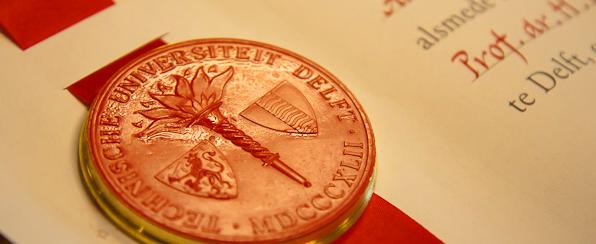
Signal processing seminar
- Thursday, 19 June 2014
- 10:15-11:00
Relaying Techniques for Multi-Hop Noncoherent UWB Communications
Dr. Vincenzo LotticiUniversity of Pisa
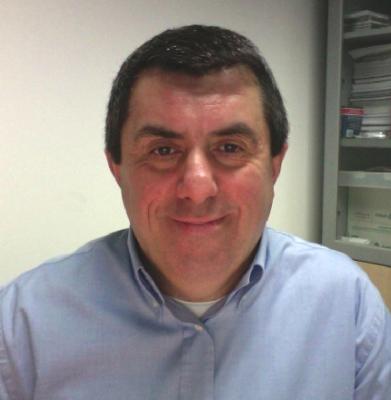
Ultra-wideband impulse radio has been attracting an interest as a strong candidate for short-range high-rate indoor connectivity, low-rate communications with high-resolution ranging, and location-aware wireless sensor networks. On top of this communication format, multi-hop relaying techniques can effectively contribute to extend the coverage and boost the system performance, especially when the energy available to each relay node of the network is a critical issue. This talk discusses a few novel cooperative approaches for both amplify-and-forward (AF) and decode and forward (DF) relaying. Firstly, we focus on a non-coherent setup employing a double-differential encoding scheme at the source node and a single differential demodulation at the relay and destination. The log-likelihood ratio based decision rule is derived at the destination node, and a semi-analytical power allocation strategy is presented by evaluating a closed-form expression for the effective signal to noise ratio (SNR) at the destination. Secondly, we focus on developing a single differential encoded DF non-cooperative relaying scheme. To favor simple receiver structures, differential noncoherent detection is employed which enables effective energy capture without any channel estimation. Putting emphasis on the general case of multi-hop relaying, we illustrate an original algorithm for the joint power allocation and path selection (JPAPS), minimizing an approximate expression of the overall bit error rate (BER). After deriving a closed-form power allocation strategy, the optimal path selection is reduced to a shortest path problem on a connected graph, which can be solved without any topology information with complexity O(N3), N being the number of available relays of the network. An approximate scheme is also presented, which reduces the complexity to O(N2), while showing a negligible performance loss. For benchmarking purposes, an exhaustive-search based multi-hop DF cooperative strategy is taken into consideration. Simulation results for various network setups corroborate the effectiveness of the proposed low-complexity JPAPS algorithm, which favorably compares to existing AF and DF relaying methods. CV. Since 1993, Vincenzo Lottici has been with the Department of Information Engineering of the University of Pisa, where he is currently Professor in Communication Systems. He participated in several international and national research projects, and as TPC member, in numerous IEEE conferences in wireless communications and signal processing, He recently joined the Editorial Board of EURASIP Advances on Signal Processing. His research interests include the broad area of signal processing for communications, with emphasis on synchronization, channel estimation, dynamic resource allocation, cognitive radio and compressive sensing.
Additional information ...
Signal Processing Seminar
- Thursday, 19 June 2014
- 09:30-10:15
- HB 17.150
Noncoherent Decision-Feedback Equalization in Massive MIMO Systems
Dr. Robert Fischer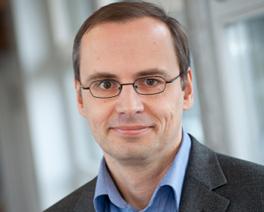
We discuss noncoherent receivers in multi-user massive MIMO uplink systems. On the one hand, sorted decision-feedback differential detection (DFDD) is attractive for the detection of a particular user over a transmission burst. On the other hand, we present a noncoherent approach to decision-feedback equalization (DFE) over the users. Thereby, the contradicting principles of DFE, where interference of already detected symbols is canceled using actual channel knowledge, and noncoherent reception, where the symbols are detected without any channel-state information, are combined. Based on an analysis of the statistics of the interference terms in autocorrelation-based noncoherent receivers, DFE is proposed and optimized. Moreover, we discuss a joint user/temporal detection with optimized sorting and an iterative scheme. Numerical results quantifying the performance of the noncoherent schemes relative to coherent BLAST, taking the non-perfect channel knowledge due to finite-length training sequences into account, are presented. CV. Since 2011 Dr. Robert Fischer has been full professor for communications and signal theory at the University of Ulm, Germany. Currently, he teaches undergraduate and graduate courses on signals and systems and on digital communications. His research concentrates on fast digital transmission including single- and multicarrier modulation techniques. Current interests are information theory, coded modulation, digital communications and signal processing, and especially precoding and shaping techniques for high-rate transmission schemes.
Additional information ...
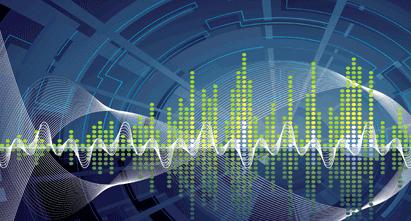
Signal Processing Seminar
- Thursday, 19 June 2014
- 11:00-11:30
- HB 17.150
Channel Estimation and Hybrid Precoding for Millimeter Wave Cellular Systems
Geert Leus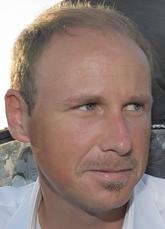
Millimeter wave (mmWave) cellular systems will enable gigabit-per-second data rates thanks to the large bandwidth available at mmWave frequencies. To realize sufficient link margin, mmWave systems will employ directional beamforming with large antenna arrays at both the transmitter and receiver. Due to the high cost and power consumption of gigasample mixed-signal devices, mmWave precoding will likely be divided among the analog and digital domains. The large number of antennas and the presence of analog beamforming requires the development of mmWave-specific channel estimation and precoding algorithms. This talk discusses an adaptive algorithm to estimate the mmWave channel parameters that exploits the poor scattering nature of the channel. To enable the efficient operation of this algorithm, a novel hierarchical multi-resolution codebook is designed to construct training beamforming vectors with different beamwidths. Using the estimated channel, a new hybrid analog/digital precoding algorithm that is proposed that overcomes the hardware constraints on the analog-only beamforming, and approaches the performance of digital solutions. Simulation results show that the proposed low-complexity channel estimation algorithm achieves comparable precoding gains compared to exhaustive channel training algorithms. The results also illustrate that the proposed channel estimation and precoding algorithms can approach the coverage probability achieved by perfect channel knowledge even in the presence of interference.
Additional information ...
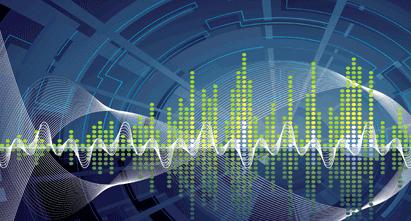
Signal Processing Seminar
- Thursday, 12 June 2014
- 13:00-14:00
- HB 17.150
Sparsity-Aware Sensor Selection: Centralized and Distributed Algorithms
Hadi Jamali-RadAdditional information ...
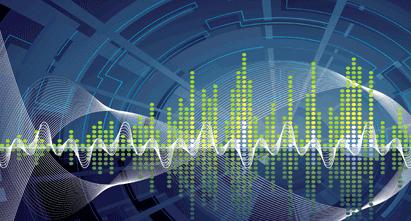
MSc TC Thesis Presentation
- Friday, 6 June 2014
- 14:00-15:00
- EWI Snijderszaal
Modeling of wave propagation in open domains: A Krylov subspace approach
Jorn Zimmerling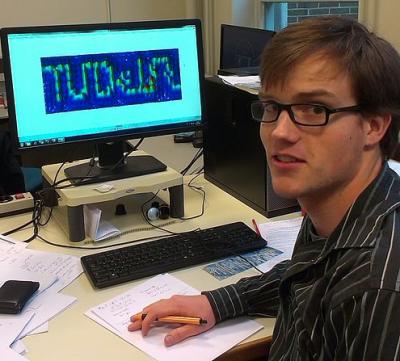
Simulating electromagnetic or acoustic wave propagation in complex open structures is extremely important in many areas of science and engineering. In a wide range of applications, ranging from photonics and plasmonics to seismic exploration, efficient wave field solvers are required in various design and optimization frameworks.?? ?
In this talk, a Krylov subspace projection methodology is presented to efficiently solve wave propagation problems on unbounded domains. To model the extension of the computational domain to infinity, an optimal complex scaling method is introduced. Traditionally, complex scaling has been used to simulate open quantum systems. Here, an optimized complex scaling method is implemented that allows us to simulate wave propagation on unbounded domains provided we compute the propagating waves via a stability-corrected wave function. In our Krylov subspace framework, this wave function is approximated by polynomial or rational functions, which are obtained via Krylov subspace projection. We show that the field approximations are actually expansions in terms of approximate open resonance modes of the system and we present a novel and highly efficient Krylov subspace implementation for media exhibiting second-order relaxation effects. Numerical examples for one-, two-, and three-dimensional problems illustrate the performance of the method and show that our Krylov resonance expansions significantly outperform conventional solution methods.
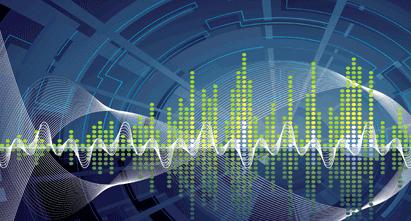
ISCAS 2014
- Sunday, 1 -- Thursday, 5 June 2014
- Melbourne Convention and Exhibition Centre, Melbourne, Australia
2014 IEEE International Symposium on Circuits and Systems
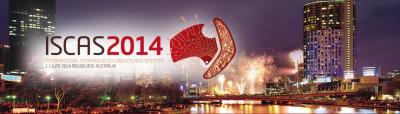
Welcome from the General Chairs of the Organising Committee
On behalf of the Organising Committee we welcome you to Melbourne, ranked by the Economist Intelligence Unit in 2011, 2012 and 2013 as the most liveable City in the world, to Australia, and to the 2014 IEEE International Symposium on Circuits and Systems.
ISCAS?2014 is sponsored by the Institute of Electrical and Electronic Engineers Circuits and Systems Society (IEEE CASS), and generously supported by the State Government of Victoria and the Melbourne Convention Bureau.
As you all know, ISCAS is the flagship annual conference of IEEE CASS, and it is well established as the world?s premier networking forum in the fields of theory, design and implementation of circuits and systems. As a result of the release of its 2012 Vision and Mission (see ieee-cas.org), the CASS goal is to develop ISCAS also as the leading forum for pioneering circuits and systems contributions to humanity?s grand challenges.
Accordingly, the special theme of ISCAS 2014 is nano/bio circuits and systems applied to enhancing living and lifestyles, particularly in relation to the multidisciplinary grand challenges in healthcare and wellbeing, the environment and climate change.
Keynotes
ISCAS?2014 has four keynote presentations, two of which address crucial aspects of high priority grand challenges, in health and in sustainability, while the other two describe frontier work at the extreme ends, in terms of scale, of circuits and systems engineering ? new devices that promise to sustain the remarkable advances in semiconductors that we have enjoyed for over 60 years, and design methods for systems of systems, which are relevant to so many grand challenge problems:
Dr Donald E. Ingber from Harvard University on Monday will present Microengineered Human Organs On Chips, describing advances he and his team have made in the engineering of microfluidic ?Organs-on-Chips??microchips lined by living human cells created with microfabrication techniques that recapitulate organ-level structure and functions as a way to replace animal testing for drug development and mechanistic discovery.
Professor Iven Mareels from The University of Melbourne in his talk on Wednesday, titled Circuits and Systems for Modern Irrigation Management, describes work over 15 years on circuits and systems research, development and commercialisation of an internet-of-things dedicated to smart irrigation water management.
Professor Victor Zhirnov from the Semiconductor Research Corporation, in Scaling Limits of Nanoionic Devices, elaborates how recognition that crystal ?defects? could be used as controllable entities, rather than being seen as imperfections, leads to the possibility that nanoionic resistive switching devices may be scalable down to ~ 1nm and thus may offer a promising path to replace the foundation of today?s computing technologies.
Dr. Stephan C. Stilkerich from Airbus Group will present Model Based Engineering of Highly Mobile Systems of Systems: Safe Aeroplanes; Safer Automobiles, with an introduction and post-talk discussion moderated by Dr Graham Hellestrand from Embedded Systems Technology. This keynote deals with front-line approaches to engineering electronic systems and their software, that are required to perform real-time control critical for the safe operation of airplanes and cars, including while operating in dense traffic and simultaneously reducing environmental impact.
Technical Program Regular Sessions
The technical program consists of tutorials, lecture papers, poster papers and demonstrations accepted based on peer review of the submission from regular open calls. We have retained many of the ISCAS features that have evolved in recent years, and added new features, some in response to ISCAS feedback, to continue to improve attendees? experience of the event.
We are very pleased to report that ISCAS?2014 will be first time that the new CASS Conference App will be made available to all attendees, and we look forward to your feedback to improve it. The CAS Society has supported the development of the Conference App, through Conference4Me, to facilitate the navigation of the conference agenda and venues, secure access to proceedings, micro-blogging, live discussion and ranking of papers, providing feedback to organizers and general improvement of attendees? experience at CAS conferences.
Lecture papers follow the traditional ISCAS format. There are nine lecture sessions over three days, with session having 11 parallel streams. Sessions are 90 minutes with up to five papers, allowing 18 minute for each including introduction, presentation and discussion.
The Demonstration session and Poster sessions are held over 3 hours commencing at the 3pm coffee break on Monday, Tuesday and Wednesday. The Demonstration session is Monday only. There are no competing parallel lecture sessions during the first 90 minutes of each day?s Posters/Demonstrations, allowing increased attention to them from all attendees.
We have increased the length of the lunch break to 90 minutes. This will allow more time for the CASS side meetings, particularly the annual meetings of the 15 CASS Technical Committees, which are playing an increasingly important role in leadership of the Society. The longer break will also provide a more relaxed walk to the nearby restaurants for the lunch break, and we hope it will facilitate a greater level of networking.
Following the ISCAS?2013 lead we continue the trial of offering free attendance at Tutorial and CAS-FEST sessions for all ISCAS?2014 registrants. We have also expanded both the tutorial program and CAS-FEST. CASS? goals in these moves are both to widen the reach of and to increase participation in the tutorial program and CAS-FEST. We will greatly appreciate feedback from attendees on the value you perceive in these offerings.
Tutorials
ISCAS?2014 commences on Sunday with 19 half-day and 1 full-day Tutorial sessions.
We have included two Tutorial sessions on Technology Management in response to feedback from CASS industry members:
T19 ? Interfacing Organisations: How to successfully manage organizational interfaces by Felix Lustenberger; and T20 - Managing Technology Professionals by Tuna B. Tarim: Transitioning from Individual Contributor to Management. Felix and Tuna are CASS members and also leaders of IEEE?s Technology Management Council, which was recently approved to transition to an IEEE Society.
Also in response to feedback, from the Women in CAS (WiCAS) and Young Professionals Program (formerly GoLD) groups, is a tutorial on career development, social skills, collaboration and networking:
T7 ? Engineering Networks that Work: Design Tools for Your Career by Dr Margaret Collins Margaret is a Cardiff-based research consultant, professional coach and trainer with extensive experience in helping people achieve their career goals. Come ready to get involved ? this is an active workshop session!
A third initiative in the Tutorials is a full day introduction to Memristive devices, circuits, systems and applications, the topic of this year?s CAS-FEST. This will cover all aspects of this emerging technology, namely: theory, practical nanodevices, physical switching mechanisms, circuits and emerging applications:
T21 ? If it?s Pinched it?s a memristor (AM), Professor Leon Chua T22 ? ReRAM Memristive Devices: Electrochemical Systems at the Atomic Scale (AM), Dr Ilia Valov T23 ? Analog and Mixed-Signal Applications of Memristive Devices (PM), Professor Dmitri Strukov T24 Integrating memristive devices in CMOS neuromorphic computing architectures (PM), Professor Giacomo Indiveri The aim of these sessions is to provide sufficient introduction to enable a typical ISCAS attendee to appreciate the state of the art material that will be presented in the CAS-FEST sessions.
CAS-FEST
Since its inception in 2010, the Circuits and Systems ? Forum on Emerging and Selected Topics has progressively become more closely integrated with ISCAS. This year?s topic was again selected from an open call and the winning proposal, from members of the Nonlinear Circuits and Systems (NCAS) Technical Committee, has taken still further this level of integration with ISCAS. This includes the presentation of invited introductory tutorials in the regular ISCAS Tutorials program (see above), the inclusion of three Special Sessions in the regular ISCAS Lecture Papers program, a full day of CAS-FEST Special Sessions on Wednesday, and the highlight full day of CAS-FEST Keynote talks on Thursday.
With this additional integration, CAS-FEST 2014 will bring together leading experts and provide a thorough coverage of the field of memristors, from an introduction to those unfamiliar with the field, through solidifying existing knowledge, to highlighting developments at the forefront of the field, and pointing to future challenges and promising directions for research. We hope that this coordinated approach will result in a landmark event in the development of the field.
Social Events and Awards Dinner
We are planning the now standard set of ISCAS evening events, with the Welcome Reception on Sunday evening soon after the conclusion of Tutorials, the WiCAS/YPP (formerly GoLD) event on early Monday evening, the Awards Dinner on Tuesday evening, and the Closing Reception immediately following the last session on Wednesday. Watch out for the Australian twists!
We hope that you will have a rewarding and enjoyable time in Melbourne at ISCAS?2014 and look forward to meeting as many of you as we can!
Professor Jugdutt (Jack) Singh & Dr David Skellern General Co-Chairs, ISCAS 2014
Additional information ...
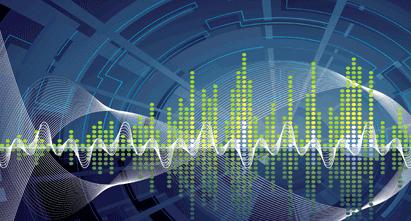
MSc ME Thesis Presentation
- Wednesday, 21 May 2014
- 09:30-10:30
- Snijderszaal LB 01.010
Physical design of a 3D router
Milovan VasicWith the use of multi-core architectures, the Network-on-Chip (NoC) became an important research topic. The most important benefit of a NoC compared to a communication bus is that it is scalable. The heart of the NoC is the router, which provides the communication between different computational units. This component is highly suitable to be a 3D component, which means that the connection can go into a vertical direction. This way the NoC is extended, with the same area footprint. This thesis describes the physical design of the 3D router, where various design problems are solved. An existing router architecture is used as a start-point. One of the problems which this thesis is trying to solve, is the reduction of the number of needed data lines. This is especially useful for the vertical data lines, which are implemented with Through Silicon Vias (TSVs). A TSV has a large footprint compared to a transistor, which means it takes up a lot of chip area. This results in increased cost. The reduction of the data lines is accomplished by the serialization of the data. It is determined that the best serialization ratio is 4. The 3D router is also adjusted for asynchronous operation. This is accomplished with the use of FIFOs, two-flop synchronizers and Gray Encoders.
Joint clock synchronization and delay estimation under quadratic clock model in wireless networks
- Thursday, 15 May 2014
- 13:00-14:00
- HB 17.150
"Joint clock synchronization and delay estimation under quadratic clock model in wireless networks"
Yan XieAdditional information ...
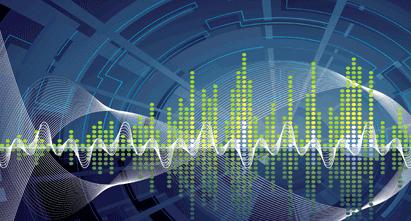
Conferences
- Sunday, 4 -- Friday, 9 May 2014
- Florence, Italy
IEEE ICASSP'2014
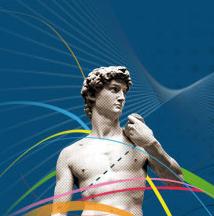
IEEE ICASSP is the world's largest and most comprehensive technical conference focused on signal processing and its applications. The series is sponsored by the IEEE Signal Processing Society and has been held annually since 1976. The conference features world-class speakers, tutorials, exhibits, a Show and Tell event, and over 120 lecture and poster sessions.
Additional information ...
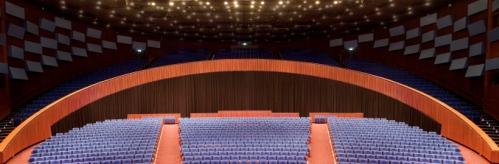
Signal Processing Seminar
- Thursday, 24 April 2014
- 13:00-14:00
- HB 17.150
Compressive Cyclic Spectrum Reconstruction
Additional information ...
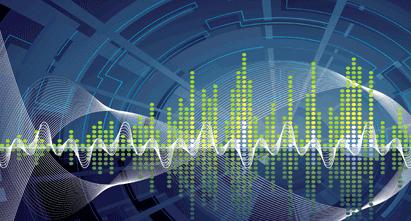
Signal Processing Seminar
- Thursday, 17 April 2014
- 16:00-17:00
- HB 17.150
GPU-Accelerated Adaptive Unstructured Road Detection Using Close Range Stereo Vision
Bugra Ozutemiz, PhD candidate, Middle-East Technical UniversityDetection of road regions is not a trivial problem especially in unstructured and/or off-road domains since traversable regions of these environments do not have common properties. Even the properties and appearance of these environments can change on the run. Hence, an algorithm working under unstructured conditions should have a continuous adaptation capability. To achieve this, a novel unstructured road detection algorithm that can continuously learn the road region is proposed in this work. The algorithm gathers close-range stereovision data using a simple roughness threshold and uses this information to estimate the road region in the far field. The proposed approach simplifies over the approaches in the literature by changing offline supervised learning and pose estimation of the vehicle and sensor with a simple heuristic coming from the nature of the problem: roughness (or smoothness) of the terrain. Thanks to the parallel nature of the algorithm, it is also implemented on a GPU with CUDA and a real-time running performance is achieved even on a low-performance graphics card. The experiments show that the algorithm gives excellent road detection results even under fast-changing light conditions and a running frequency of 50 Hz is achieved even in the worst case.
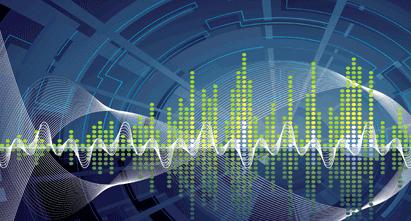
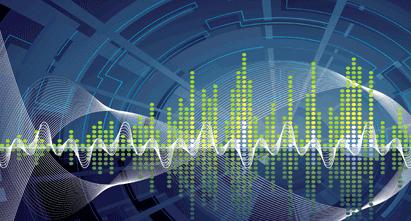
MSc TC Thesis Presentation
- Wednesday, 16 April 2014
- 10:00-10:45
- EWI Snijderszaal
Joint Angle-Frequency Estimation for Multiple Signals with Circular Arrays
Joost GeelhoedCAS, TNO
(Work carried out at TNO Defence, Safety and Security--The Hague)
In electronic warfare information about radio signals is gathered. Parameters as the directionof- arrival (DOA) and the frequency can be estimated from sampled data received on antenna arrays. The objective of this thesis is to design a joint angle-frequency estimation (JAFE) algorithm for a circular uniform antenna array.
A 1-D and 2-D JAFE algorithm is presented. Both algorithms use phase-mode excitation and ESPRIT. The 1-D algorithm with spatial smoothing is introduced. With this algorithm it possible to estimate signals with similar frequencies, when the elevation is 90 degrees. Simulations demonstrate that when two signals are coherent and a spatial smoothing factor of two is applied the mean of the azimuth estimation is the true mean. It is also demonstrated that when two signals have the same DOA a temporal smoothing factor of two is necessary and that more temporal smoothing reduces the standard deviation of the azimuth estimation. It is shown that the phase-mode excitation technique introduces a systematic error that is considerably high for few antenna elements and an even number of elements. It is demonstrated that interpolation can reduce this error in case a UCA of 5 elements or a UCA of 12 elements.
Signal Processing Seminar
- Thursday, 10 April 2014
- 13:00-14:00
- HB 17.150
3ME DCSCAdditional information ...
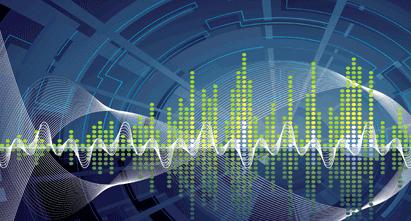
Signal Processing Seminar
- Thursday, 10 April 2014
- 15:00-16:00
- HB 17.150
OFDM Analysis: an Industry Point of View
Earl McCune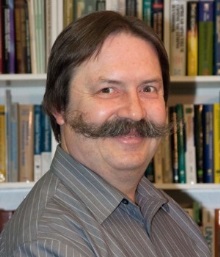
With more than 40 years of experience in wireless communications technology and its associated hardware construction, this presenter finds the current flurry of interest in orthogonal frequency division modulation (OFDM) very interesting. It is surprising that the extremely revolutionary nature of this signal class is actually poorly understood, particularly by the academic community. On the industrial side, there is a general misunderstanding of the economic consequences of building hardware needed to generate and receive an OFDM signal. Here the motivation and physical principles of OFDM are built from fundamental concepts, from which the signal characteristics are evaluated and compared with conventional QAM. Conclusions on the comparative economics of OFDM are drawn.
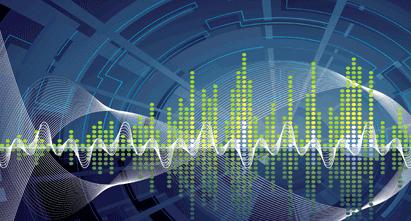
EUCAP14 conference on antennas and propagation
- Sunday, 6 -- Tuesday, 8 April 2014
- The Hague
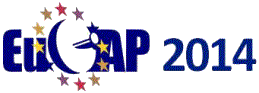
Abstract submission: 13 Oct 2013
Conference dates: 6-8 April 2014
Application areas:
- Fundamental research
- Satcom on-the-move terminal antennas
- Navigation, localisation, positioning and tracking
- Cellular mobile communications (includes: base station, handheld devices)
- Machine to machine, internet on devices
- Wireless networks (includes: WLAN, indoor communication)
- High data-rate transfer and backbone networks
- RFID and sensor networks
- Biomedical (includes: human body interaction, on-body antennas, electromagnetic exposure and interactions)
- Satellite communications
- Satellite passive and active remote sensing
- RADAR
- Radio astronomy
- Signal and image processing
- Defense and security
- Short-range Giga-bit communications
- Commercial software
Signal Processing Seminar
- Thursday, 27 March 2014
- 13:00-14:00
- HB 17.150
EWI, Embedded Systems group

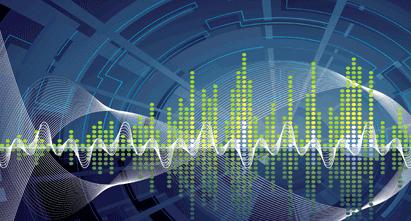
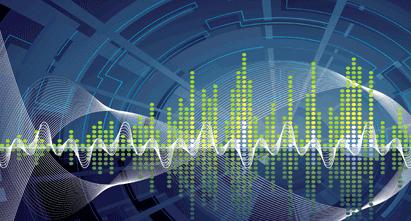
Signal Processing Seminar
- Thursday, 13 March 2014
- 13:00-14:00
- HB 17.150
Speech reinforcement in noisy reverberant environments using a perceptual distortion measure
Joao CrespoAdditional information ...
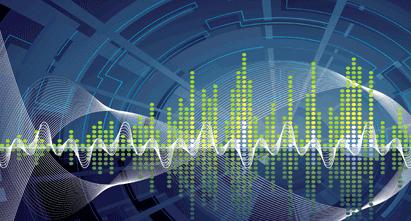
Signal Processing Seminar
- Thursday, 6 March 2014
- 13:00-14:00
- HB 17.150
On indoor localization based on acoustic signals and channel fingerprinting
Yongwei (Enki) WangNWPU, ChinaAdditional information ...
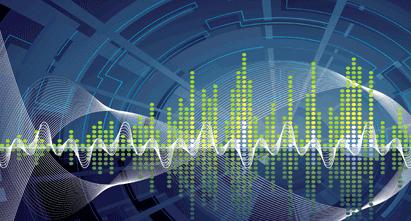
MSc CE Thesis Presentation
- Tuesday, 4 March 2014
- 10:30-11:30
- HB 17.150
Modeling of Olivocerebellar Neurons using SystemC and High-Level Synthesis
M.F. van EijkNeuro-scientific experiments often require a heavy amount of computational power to achieve a more efficient research process. Speeding up neuron models, used in such experiments, facilitates faster testing of scientific hypotheses and faster model refinement in order to better replicate the biological-cell behavior in question. To be able to simulate realistic behavior, high-detail neuron models need to be built and typically require maximal computing power. Hardware acceleration can be used to execute such simulations in a realistic amount of time. However, building a hardware implementation is very time-consuming and error-prone. Recent trends in RTL design have resulted in tools which can convert a high-level behavioral hardware description to an RTL description targeting an FPGA, which makes them ideal tools for complex FPGA designs, such as high-detail neuron models. In this thesis, a model of the Inferior-Olivary Nucleus (ION) network has been implemented with SystemC RTL and mapped onto an FPGA using a High-Level Synthesis (HLS) tool-flow. A shared-bus architecture has been used as proof of concept to interconnect the various cells in the modeled network. SystemC Transaction Level Modeling (TLM) facilitates fast network-interconnect modeling and verifying of model functionality. We have thus developed a SystemC TLM model that can predict trends of our RTL implementation. With this TLM model we were able to quickly model large network sizes and assess the model?s scalability with respect to utilized resources and performance. The complete network model has, subsequently, also been synthesized. Xilinx Vivado HLS has been used to convert the SystemC implementation to an RTL description mapped on a Virtex 7 (XC7VX550T) FPGA device. The resulting design achieved a speed-up of x6 compared to a reference C model, making it possible to simulate a network of 48 cells in real-time. Because HLS tools are used, the model can be easily modified to accommodate last-minute changes and models updates by the neuroscientific community.
Signal Processing Seminar
- Thursday, 27 February 2014
- 13:00-14:00
- HB 17.150
Current and future research lines in audio signal processing
Richard Heusdens
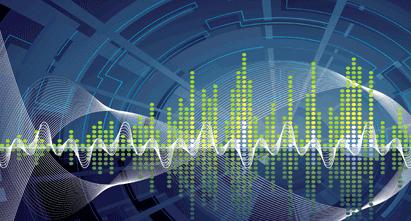
Signal Processing Seminar
- Thursday, 20 February 2014
- 13:00-14:00
- HB 17.150
AIS ship identification signal separation using GSVD and the Signed URV algorithm
Alle-Jan van der VeenSeparating partially overlapping data packets using subspace intersection.
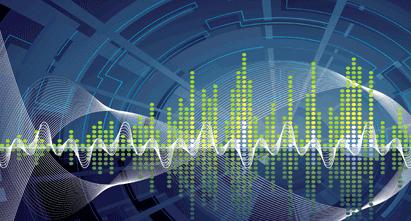
Signal Processing Seminar
- Thursday, 28 November 2013
- 16:00-17:00
- HB 17.150
Low-complexity computer simulation of multichannel room impulse responses
CAS

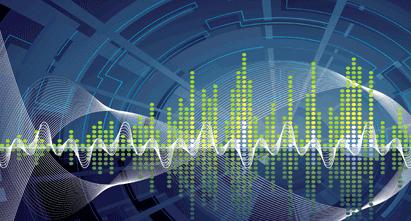
Signal Processing Seminar
- Thursday, 21 November 2013
- 16:00
- HB 17.150
CAS

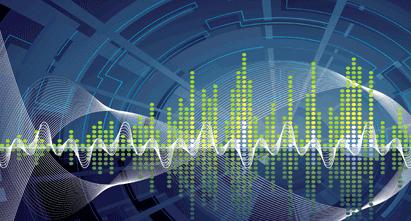
Signal Processing Seminar
- Thursday, 7 November 2013
- 16:00-17:00
- HB 17.150
Relative velocity estimation using Multidimensional Scaling
Raj Thilak RajanCAS


Signal Processing Seminar
- Thursday, 31 October 2013
- 16:00-17:00
- HB 17.150
Tracking Position and Orientation of a Mobile Rigid Body
Sundeep Prabhakar ChepuriCAS


PhD Thesis Defence
- Friday, 25 October 2013
- 10:00-11:00
- Aula
Spectrum sensing for cognitive sensor networks
Sina Maleki
Signal Processing Seminar
- Thursday, 24 October 2013
- 16:00-17:00
- HB 17.150
Resource allocation for mobile ad hoc networks
Philippe CiblatTELECOM ParisTech
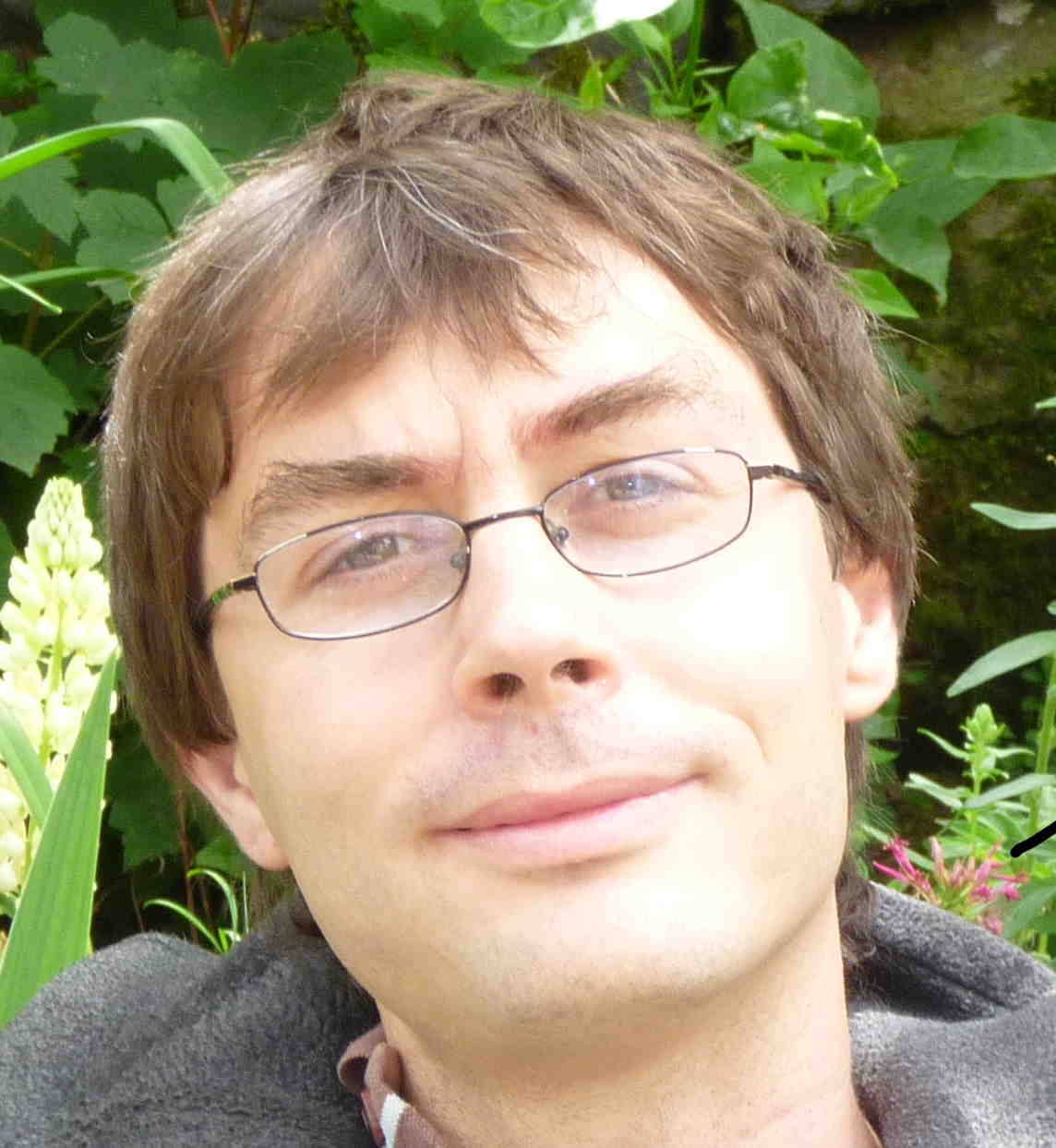

Signal Processing Seminar
- Friday, 18 October 2013
- 11:00
- HB 17.150
ICA and IVA: Theory, Connections, and Applications to Medical Imaging
Tulay AdaliUniversity of Maryland at Baltimore County

Data-driven methods are based on a simple generative model and hence can minimize the assumptions on the nature of data. They have emerged as promising alternatives to the traditional model-based approaches in many applications where the underlying dynamics are hard to characterize. Independent component analysis (ICA), in particular, has been a popular data-driven approach and an active area of research. Starting from a simple linear mixing model and imposing the constraint of statistical independence on the underlying components, ICA can recover the linearly mixed components subject to only a scaling and permutation ambiguity. It has been successfully applied to numerous data analysis problems in areas as diverse as biomedicine, communications, finance, geophysics, and remote sensing.
This talk reviews the fundamentals and properties of ICA, and provides a unified view of two main approaches for achieving ICA, those that make use of non-Gaussianity and sample dependence. Then, the generalization of ICA for analysis of multiple datasets, independent vector analysis (IVA), is introduced and the connections between ICA and IVA are highlighted, in particular in the way both approaches make use of signal diversity. Several key problems for achieving a successful decomposition, such as matrix optimization and density matching are discussed as well, along with examples of their application to medical image analysis.
Additional information ...

Signal Processing Seminar
- Thursday, 17 October 2013
- 16:00-17:00
- HB 17.150
CAS


Signal Processing Seminar
- Thursday, 10 October 2013
- 16:00-17:00
- HB 17.150
Constrained Imaging with Active-set methods
Millad Mouri SardarabadiCAS
Can radio astronomy imaging be improved by incorporating constraints such as non-negativity and known upper bounds?

Signal Processing Seminar
- Thursday, 3 October 2013
- 16:00-17:00
- HB 17.150
Secure Signal Processing: Challenges and Opportunities
Zekeriya ErkinTU Delft ISP Lab


Short Course
- Wednesday, 4 -- Wednesday, 11 September 2013
- 10:30-15:00
- HB 10.230
Network Information Theory: Cooperation and Interference
Prof.dr. M.C. GastparEPFL
Information Theory has proved to be a relevant and successful foundation for the understanding of point-to-point digital communication systems. In today's networked world, information flows in more complex patterns: We can think of networks of sensors or cameras that are wirelessly connected or of cooperating base stations in mobile telephony systems. Over the past decade, significant progress has been made towards extending information theory to such communication systems. In this short course, we survey both classical results and recent progress in Network Information Theory.
Registration:
This course is intended for interested PhD/MSc students with some background in information theory. They should register before Monday, September 2, 2013, via e-mail to J.H.Weber@tudelft.nlCredit:
Upon completion of the class, including homework, PhD students in the TU Delft Graduate School will earn 2 credits for their doctoral education program. Other students can receive a certificate.Outline:
The short course will be taught mostly on the blackboard, along with some slides. It contains four modules:- Review of Basic Information Theory
- Broadcast and Multiple Access
- Techniques for Relaying and Cooperation
- Managing Interference
Textbook:
Recommended (but not necessary) is the book by Abbas El Gamal and Young- Han Kim, Network Information Theory, Cambridge University Press, 2013.Additional information ...
MSc TC Thesis Presentation
- Tuesday, 27 August 2013
- 10:30-11:15
- HB 17.150
Compressive power spectral density estimation with non-uniform sampling
Fernando de la Hucha Arce
MSc TC Thesis Presentation
- Monday, 12 August 2013
- 14:00-15:00
- HB 17.150
Photo-acoustic imaging
Victor SloevA new non-invasive imaging modality is based on transmitting a laser pulse on the skin; the created heat will cause an acoustic pulse that is detected with an ultrasonic array. How can an image be formed?
Signal Processing Seminar
- Thursday, 18 July 2013
- 15:30-16:30
- HB 17.150
Optimal power allocation for energy harvesting cognitive radio networks
NingCAS
Signal Processing Seminar
- Tuesday, 9 April 2013
- 15:00-16:00
- HB 17.150
Location tracking
Raj Thilak RajanCAS
Signal Processing Seminar
- Sunday, 20 January 2013
- 15:00-16:00
- HB 17.150
Underwater localization
Hamid RamezaniCAS
PhD Thesis Defence
- Tuesday, 15 January 2013
- 12:30-14:00
- Aula Senaatszaal
Wireless Transceiver Design For High Velocity Scenarios
Tao Xu
This thesis is dedicated to transceiver designs for high data-rate wireless communication systems with rapidly moving terminals. The challenges are two-fold. On the one hand, more spectral bandwidth of the transmitted signals is required by future wireless systems to obtain higher transmission rates, which can result in the frequency selectivity of the communication channels. On the other hand, Doppler effects emerge when high mobile speeds are present, which can result in the time selectivity of the communication channels. Therefore, it is likely that future wireless communication systems operate in doubly-selective channels, which impose many difficulties on transceiver designs. In this thesis, we investigate these challenges in the four scenarios, and propose a number of corresponding solutions.
- OFDM over Narrowband Channels;
- OFDM over Wideband Channels;
- Multi-Rate Transmissions over Wideband Channels;
- Robust Multi-band Transmissions over Wideband Channels.

MSc Thesis Presentation
- Sunday, 7 October 2012
- 14:00-14:30
- HB17.150
Underwater Ultra-Wideband Fingerprinting-Based Localization
Siavash ShakeriIn this work a new location fingerprinting-based localization algorithm is proposed for an underwater medium by utilizing ultra-wideband (UWB) signals. In many conventional underwater systems, localization is accomplished by utilizing acoustic waves. On the other hand, electromagnetic waves haven?t been employed for underwater localization due to the high attenuation of the signal in water. However, it is possible to use UWB signals for short-range underwater localization. In this work, the feasibility of performing localization for an underwater medium is illustrated by utilizing a location-based fingerprinting approach. Existing algorithms for an indoor environment are evaluated in this project for an underwater medium. These algorithms are based on a neural networks or maximum likelihood estimator. Further, we also consider a classical k-nearest neighbors (KNN) approach. In addition, by employing the concept of compressive sampling, we propose a sparsity-based localization approach for which we define a system model exploiting the spatial sparsity. Moreover, a recently proposed grid mismatching algorithm is also adapted to the current localization framework and its performance is evaluated. Finally, the performance of the proposed methods is compared with the existing fingerprinting-based localization approaches.