dr. B. Hunyadi
Signal Processing Systems (SPS), Department of Microelectronics
Expertise: Biomedical signal processing, Tensor decompositions
Themes: Biomedical signal processingBiography
Borbála (Bori) Hunyadi was born in Budapest, Hungary. She received a MSc degree in electrical and computer engineering from the Pazmany Peter Catholic University in 2009 and a PhD in electrical engineering from the Department of Electrical Engineering at KU Leuven in 2014, where she continued to work as a postdoctoral researcher.
In 2018 she was awarded one of the “Delft Technology Fellowships” for outstanding female academic researchers. In October 2018 she joined the Circuits and Systems (now Signal Processing Systems) group at TU Delft as an assistant professor. She is the co-director of the Delft Tensor AI Lab (DeTAIL).
Her research interests include biomedical signal processing and machine learning for biomedical pattern recognition. More specifically, she is interested in multichannel and multimodal signal processing and fusion, blind source separation, tensor decompositions and wearable signal processing to better understand healthy and pathological physiology, in particular in neuroscience applications.
She was the secretary of the IEEE EMBC Benelux chapter between 2019-2024. Currently she serves the vice-chair of the EURASIP technical area committee on biomedical signal and image processing. She is associate editor for the IEEE Signal Processing Magazine (Columns and Forum) and Frontiers in Neuroscience (Brain Imaging Methods).
EE4530 Applied convex optimization
Applied convex optimization: role of convexity in optimization, convex sets and functions, Canonical convex problems (SDP, LP, QP), second-order methods, first-order methods for large-scale problems.
EE4750 Tensor networks for green AI and signal processing
Introduction to multilinear algebra, tensor decompositions, and their applications for green AI and biomedical signal processing
Education history
EE2S31 Signal processing
(not running) Digital signal processing; stochastic processes
Prostate cancer detection using ultrasound
Tensor techniques to improve the analysis of (3D+time) ultrasound images
Delft Tensor AI Lab
Tensor-based AI methods for biomedical signals
Projects history
Multimodal, multiresolution brain imaging
Developing a novel brain imaging paradigm combining functional ultrasound and EEG
Medical Delta Cardiac Arrhythmia Lab
Part of a larger program (with Erasmus MC) to unravel and target electropathology related to atrial arrhythmia
- Speckle Denoising of Dynamic Contrast-enhanced Ultrasound using Low-rank Tensor Decomposition
M. Calis; M. Mischi; A.-J. van der Veen; B. Hunyadi;
accepted for publication by IEEE Trans. Medical Imaging,
March 2025. DOI: 10.1109/TMI.2025.3551660 - A Singular-value-based Map to Highlight Abnormal Regions Associated with Atrial Fibrillation Using High-resolution Electrograms and Multi-lead ECG
H. Moghaddasi; R.C. Hendriks; B. Hunyadi; P. Knops; M.S. van Schie; N.M.S. de Groot; A.J. van der Veen;
IEEE Trans. Biomedical Eng.,
2024. DOI: 10.1109/TBME.2024.3420412
document - Tensor decomposition-based data fusion for biomarker extraction from multiple EEG experiments
Kenneth Stunnenberg; Richard Hendriks; Jantien Vroegop; Marloes Adank; Borbala Hunyadi;
In International Conference on Acoustics, Speech, and Signal Processing (ICASSP),
2024. - Denoising of the Speckle Noise by Robust Low-rank Tensor Decomposition
M. Calis; B. Hunyadi;
In 32nd European Signal Processing Conference (EUSIPCO),
pp. 1157-1161, September 2024. DOI: 10.23919/EUSIPCO63174.2024.10715120
document - Modeling nonlinear evoked hemodynamic responses in functional ultrasound
S. Kotti; A. Erol; B. Hunyadi;
In IEEE ICASSPW 2023 Workshop proceedings,
2023.
document - Classification of De Novo Post-Operative and Persistent Atrial Fibrillation Using Multi-Channel ECG Recordings
Hanie Moghaddasi; Richard C. Hendriks; Alle-Jan van der Veen; Natasja M.S. de Groot; Borbala Hunyadi;
Computers in Biology and Medicine,
Volume 143, April 2022. DOI: 10.1016/j.compbiomed.2022.105270
document - Denoising of Dynamic Contrast-enhanced Ultrasound Sequences: a Multilinear Approach
M. Calis; M. Mischi; A.-J. van der Veen; B. Hunyadi;
In Proceedings of the 15th International Joint Conference on Biomedical Engineering Systems and Technologies,
pp. 192-199, February 2022. DOI: 10.5220/0010838000003123
document - Surface Electrocardiogram Reconstruction Using Intra-operative Electrograms
H. Moghaddasi; B. Hunyadi; A.J. van der Veen; N.M.S. de Groot; R.C. Hendriks;
In 42nd WIC Symposium on Information Theory and Signal Processing in the Benelux (SITB 2022),
Louvain la Neuve, Belgium, pp. 136, 2022.
document - Multiparametric ultrasound and machine learning for prostate cancer localization
P. Chen; M. Calis; H. Wijkstra; P. Huang; B. Hunyadi; M. Mischi;
In 30th European Signal Processing Conference (EUSIPCO),
September 2022. DOI: 10.23919/EUSIPCO55093.2022.9909729
document - Novel rank-based features of atrial potentials for the classification between paroxysmal and persistent atrial fibrillation
H. Moghaddasi; R.C. Hendriks; A.J. van der Veen; N.M.S. de Groot; B. Hunyadi;
In 2022 Computing in Cardiology (CinC),
IEEE, September 2022.
document - Augmenting interictal mapping with neurovascular coupling biomarkers by structured factorization of epileptic EEG and fMRI data
Van Eyndhoven, Simon; Dupont, Patrick; Tousseyn, Simon; Vervliet, Nico; Van Paesschen, Wim; Van Huffel, Sabine; Hunyadi, Borbala;
NeuroImage,
Volume 228, pp. 117652, 2021. DOI: 10.1016/j.neuroimage.2020.117652 - The power of ECG in multimodal patient‐specific seizure monitoring: Added value to an EEG‐based detector using limited channels
Vandecasteele, Kaat; De Cooman, Thomas; Chatzichristos, Christos; Cleeren, Evy; Swinnen, Lauren; Macea Ortiz, Jaiver; Van Huffel, Sabine; Dumpelmann, Matthias; Schulze-Bonhage, Andreas; De Vos, Maarten; Van Patschen, Wim; Hunyadi, Borbala;
Epilepsia,
Volume 62, Issue 10, pp. 2333-2343, October 2021. DOI: https://doi.org/10.1111/epi.16990 - Tensors for neuroimaging: A review on applications of tensors to unravel the mysteries of the brain
Aybuke, Erol; Hunyadi, Borbala;
In Tensors for Data Processing: Theory, Methods, and Applications,
Elsevier, October 2021. eBook ISBN 9780323859653. - Personalizing Heart Rate-Based Seizure Detection Using Supervised SVM Transfer Learning
Thomas De Cooman; Kaat Vandecasteele; Carolina Varon; Borbala Hunyadi; Evy Cleeren; Wim Van Paesschen; Sabine Van Huffel;
Frontiers in Neurology,
Volume 11, pp. 145, 2020. DOI: 10.3389/fneur.2020.00145
document - Visual seizure annotation and automated seizure detection using behind-the-ear electroencephalographic channels
Vandecasteele, Kaat; De Cooman, Thomas; Dan, Jonathan; Cleeren, Evy; Van Huffel, Sabine; Hunyadi, Borbala; Van Paesschen, Wim;
Epilepsia,
Volume 61, Issue 4, pp. 766--775, 2020. DOI: 10.1111/epi.16470
document - Zebrafish-based screening of antiseizure plants used in Traditional Chinese Medicine: Magnolia officinalis extract and its constituents Magnolol and Honokiol exhibit potent anticonvulsant activity in a therapy-resistant epilepsy model
Li, Jing; Copmans, Danielle; Partoens, Michele; Hunyadi, Borbala; Luyten, Walter; de Witte, Peter;
ACS chemical neuroscience,
Volume 11, Issue 5, pp. 730--742, 2020. DOI: 10.1021/acschemneuro.9b00610
document - Tensor-based Detection of Paroxysmal and Persistent Atrial Fibrillation from Multi-channel ECG
H. Moghaddasi; A.J. van der Veen; N.M.S. de Groot; B. Hunyadi;
In 29th European Signal Processing Conference (EUSIPCO 2020),
Amsterdam (Netherlands), EURASIP, pp. 1155-1159, August 2020.
document - Joint Estimation of Hemodynamic Response and Stimulus Function in Functional Ultrasound Using Convolutive Mixtures
Aybuke Erol; Simon Van Eyndhoven; Sebastiaan Koekkoek; Pieter Kruizinga; Borbala Hunyadi;
In 2020 54th Asilomar Conference on Signals, Systems, and Computers,
IEEE, 2020. - Development of temporal lobe epilepsy during maintenance electroconvulsive therapy: A case of human kindling?
C. Schotte; E. Cleeren; K. Goffin; B. Hunyadi; S. Buggenhout; K. Van Laere; W. Van Paesschen;
Epilepsia Open,
Volume 4, Issue 1, pp. 200-205, 2019. DOI: 10.1002/epi4.12294
document - Semi-automated EEG enhancement improves localization of ictal onset zone with EEG-correlated fMRI
S. Van Eyndhoven; B. Hunyadi; P. Dupont; W. Van Paesschen; S. Van Huffel;
Frontiers in Neurology,
Volume 10, 2019. DOI: 10.3389/fneur.2019.00805
document - Nonconvulsive epileptic seizure monitoring with incremental learning
Y.R. Rodriguez Aldana; E.J. Maranon Reyes; F. Sanabria Macias; V. Rodriguez Rodriguez; L. Morales Chacon; S. Van Huffel; B. Hunyadi;
Computers in Biology and Medicine,
Volume 114, pp. 103434, 2019. ISSN 0010-4825. DOI: 10.1016/j.compbiomed.2019.103434
Keywords: ...
Nonconvulsive epileptic seizures, Hilbert huang transform, Multiway data analysis, Incremental learning.
Abstract: ...
Nonconvulsive epileptic seizures (NCSz) and nonconvulsive status epilepticus (NCSE) are two neurological entities associated with increment in morbidity and mortality in critically ill patients. In a previous work, we introduced a method which accurately detected NCSz in EEG data (referred here as ‘Batch method’). However, this approach was less effective when the EEG features identified at the beginning of the recording changed over time. Such pattern drift is an issue that causes failures of automated seizure detection methods. This paper presents a support vector machine (SVM)-based incremental learning method for NCSz detection that for the first time addresses the seizure evolution in EEG records from patients with epileptic disorders and from ICU having NCSz. To implement the incremental learning SVM, three methodologies are tested. These approaches differ in the way they reduce the set of potentially available support vectors that are used to build the decision function of the classifier. To evaluate the suitability of the three incremental learning approaches proposed here for NCSz detection, first, a comparative study between the three methods is performed. Secondly, the incremental learning approach with the best performance is compared with the Batch method and three other batch methods from the literature. From this comparison, the incremental learning method based on maximum relevance minimum redundancy (MRMR_IL) obtained the best results. MRMR_IL method proved to be an effective tool for NCSz detection in a real-time setting, achieving sensitivity and accuracy values above 99%.
document
BibTeX support
Last updated: 25 Mar 2025
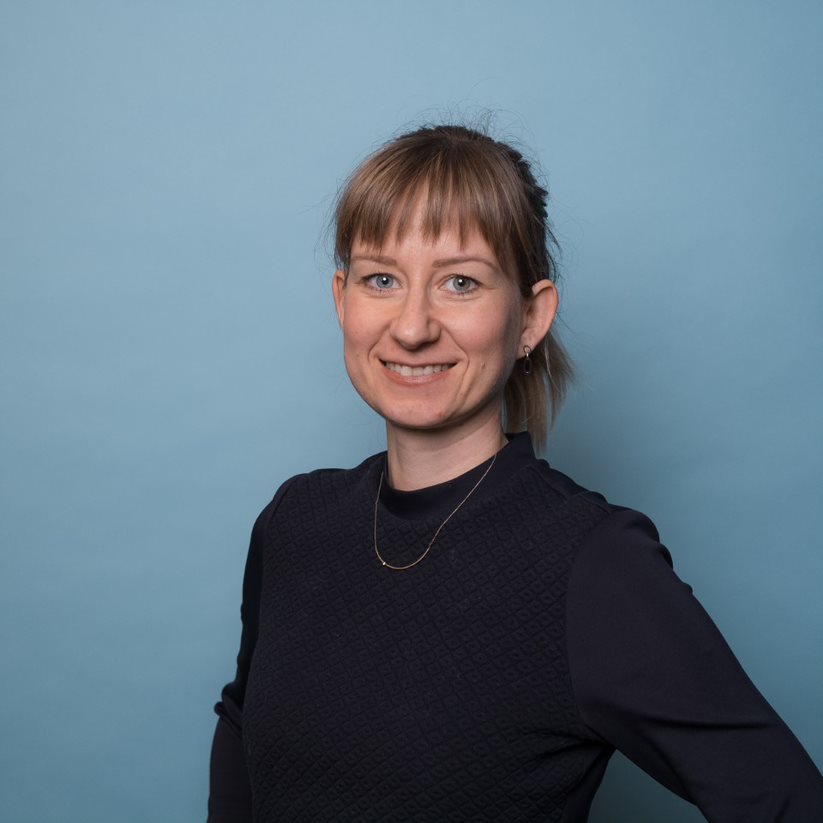
Borbála Hunyadi
PhD students
MSc students
- Kai Grotepass
- Jep Brinkmann
- Yang Xu
Alumni
- Elise Bakker (2025)
- Beatriz Lafuente Alcazar (BE) (2024)
- Joos Vrijdag (2024)
- Christiaan Bot (2024)
- Lieke Roelofs (2024)
- Kenneth Stunnenberg (2023)
- Xuan Gao (2023)
- Pierre-Antoine Denarié (2022)
- Zhuangzhuang Yu (2022)
- Xiaoning Shi (2022)
- Cesar Eduardo Cornejo Ramirez (2022)
- Yitong Tao (2022)
- Ruben Wijnands (2022)
- Arda Kaygan (2021)
- Gaia Zin (2021)
- Maarten Enthoven (2021)
- Mandani (Mado) Ntekouli (2019)
MSc project proposals
- Brain-computer interfaces based on EEG recording of imagined speech
- Contactless determination of vital parameters for improved healthcare (with Intelliprove)
- Dynamic brain network analysis
- Optimizing the analysis of auditory event-related potentials in EEG (with Erasmus Medical Center)
- Nonlinear identification of the hemodynamic response in functional ultrasound