Machine learning for image and video understanding
Contact: Justin Dauwels
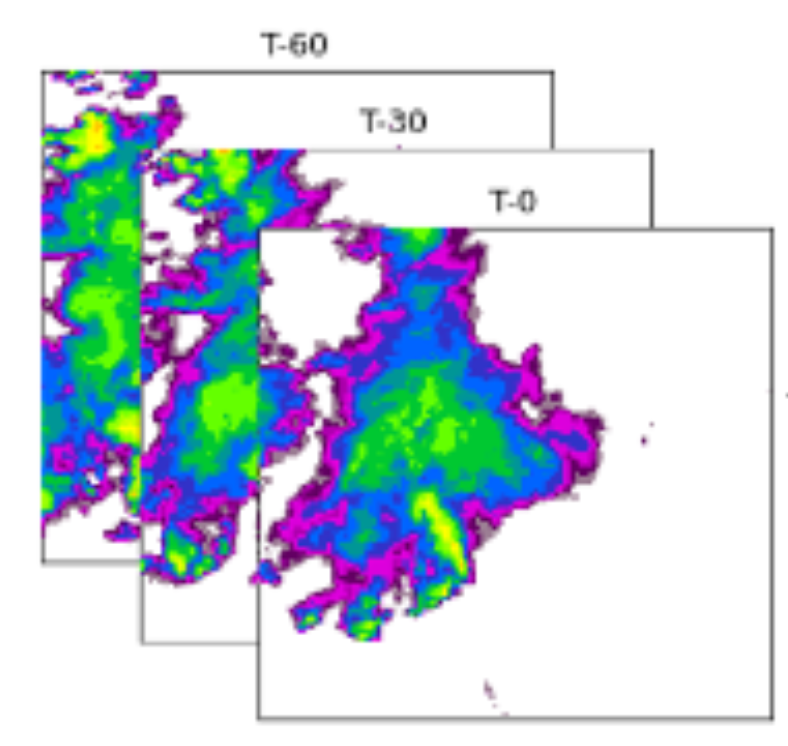
Human intelligence can easily discover object components, learn abstract concepts, and reason about entity relationships from natural scenes, while artificial intelligence still lags behind. This theme aims at bridging this gap in practical applications by incorporating prior domain knowledge. Specific research topics include object detection, person tracking, 3D scene reconstruction, spatial-temporal radar image prediction, representation learning, and image (video) generation. Instead of considering synthetic images or manually cleaned simple datasets, this research theme focuses on more challenging real-world scenes such as room interiors, office buildings, and precipitation images, to name just a few, where occlusion, incomplete observations, or extreme values may appear. To cope with these challenges, the interplay of flexible deep neural networks with multi-view information fusion approaches, neural radiance field models, extreme value theory, and probabilistic graphical models will be explored. We closely collaborate with a variety of research institutes (e.g., NTU) and companies (e.g., Deepmind, Philips, TÜV SÜD, Nexans).
Projects under this theme
Model-driven decision lab
Data and model-driven decision making for the Dutch police
Towards robust perception of radar images
Machine learning and Signal Processing for radar systems (digital radar, millimeter-wave radar), automotive sensors, and related applications
Neural Radiance Fields for 3D Reconstruction of Scenes from Images
This project aims at synthesizing novel views of scenes given only sparse views.
Nowcasting of Extreme Rainfall
This project explores nowcasting of extreme rainfall with deep generative models and extreme value theory.
Machine learning for Optimizing Workflow in the Operating Room
This project is concerned with object detection, person tracking and camera calibration in scenes with severe occlusion.
News
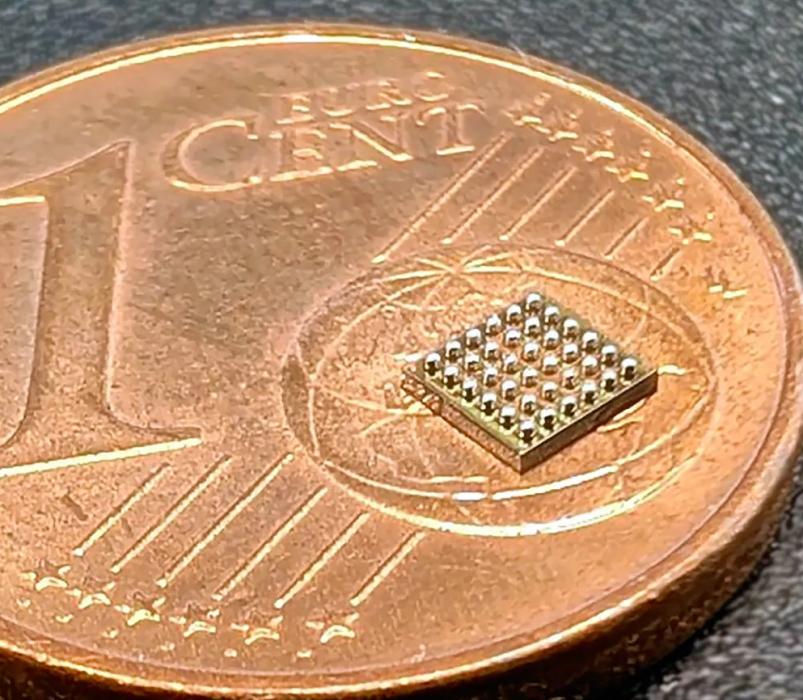
Startup Innatera covered in IEEE Spectrum
Introducing Pulsar, a Spiking Neural Networks chip
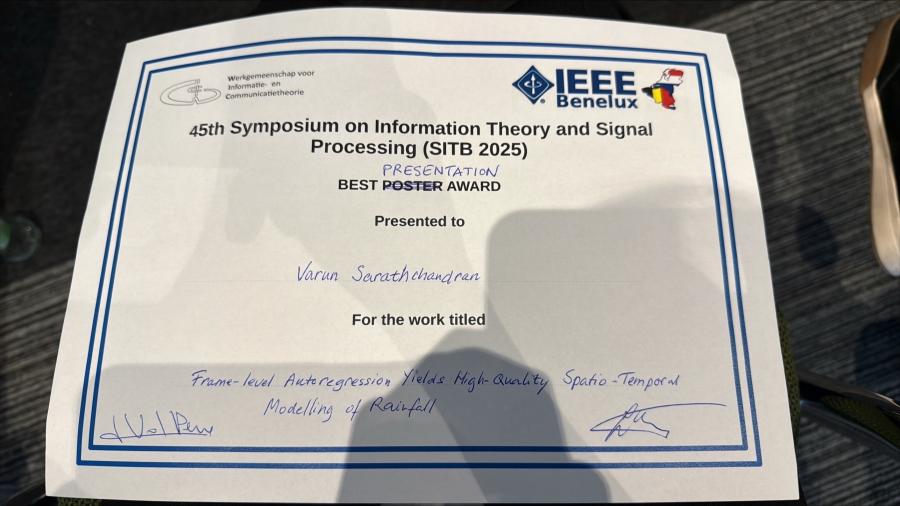
SITB presentation award
MSc students
Alumni
- Konstantinos Andriopoulos (2024)
- Zeineh Bou Cher (2024)
- Junzhe Yin (2024)
- Ankush Roy (2024)
- Yiheng Chang (2024)
- Zipeng Wang (2024)
- Doruk Barokas Profeta (2023)
- Shuoyan Zhao (2023)
- Sinian Li (2023)
- Alan Hamo (2023)
- Jonathan Dijkstra (2023)
- Luuk Bolhuis (2022)
- Chuhan Wang (2022)
- Haoran Bi (2022)
- Renjie Dai (2022)
- Enpu Chen (2022)
- Jinchen Zeng (2022)
- Zhiyi Wang (2022)
- Yanan Hu (2022)
- Yingfeng Jiang (2022)
- Qi Zhang (2022)
- Nan Lin (2022)
- Yuanyuan Yao (2022)
- Pallas Koers (2022)