Neural Radiance Fields for 3D Reconstruction of Scenes from Images (NeRF)
It is highly challenging to reconstruct 3D scenes given only (sparse) multi-view images of scenes. The recent development of Neural Radiance Fields (NeRF) is a significant breakthrough in addressing this challenge. Many NeRF-based models have been proposed using graphics techniques to create photorealistic visuals from hand-crafted scene representations. However, several aspects of NeRF still need further improvement, especially for real-world scenes. For example, the main limitation of NeRF is its inability to scale well to wild scenes. Compared to small-scale objects, many details of large-scale objects in wild scenes may be lost because of the complicated outdoor environments and the interference between camera views. Furthermore, the positional encoding technique used in NeRF for capturing a high-fidelity representation of high-frequency content in the scenes usually leads to undesired artifacts. How to alleviate or remove such artifacts is still unexplored. This project will approach these challenges by developing novel approaches using activation functions. We test our model on multi-view RGB images of real-world office buildings captured by a drone.
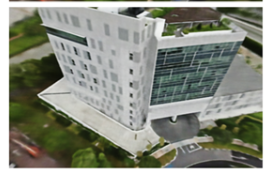
Project data
Starting date: | November 2021 |
---|---|
Closing date: | November -0001 |
Contact: | Justin Dauwels |