Nowcasting of Extreme Rainfall (NER)
Extreme precipitation often causes serious hazards such as flooding and landslides, which pose threats to human lives and cause substantial economic loss. In order to give early warnings of such hazards, nowcasting systems have been widely used to forecast the future weather condition in the short term (typically less than 6 hours). In this project, we adopt deep generative models to achieve more accurate precipitation prediction than existing methods. For precipitation nowcasting, weather conditions are usually represented by the radar precipitation fields produced by weather radars. The inputs of a nowcasting system are precipitation fields of the previous timestamps and the outputs are forecasting of future radar precipitation fields. Different from earlier studies in the literature that either adopt physical models or deep learning models, the prediction models developed in this project explore the combination of the best of two worlds. Specifically, we design deep generative models to avoid blurry generation and incorporate extreme value theory into those models to capture extreme patterns. We test our models on radar data from the Royal Netherlands Meteorological Institute (KNMI). The selected dataset contains the radar reflectivity data from 2008 to 2021, with a spatial resolution of 1 km and a temporal resolution of 5 minutes. The rainfall rate is estimated from the radar reflectivity using a Z-R transformation.
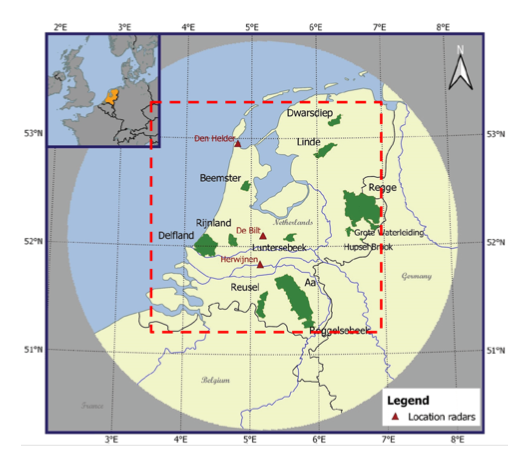
Project data
Starting date: | October 2021 |
---|---|
Closing date: | November -0001 |
Contact: | Justin Dauwels |