Programmable Systems for Intelligence in Automobiles (PRYSTINE)
Among the actual trends that will affect society in the coming years, autonomous driving stands out as having the potential to disruptively change the automotive industry as we know it today. As a consequence, this will also highly impact the semiconductor industry and open new market opportunities, since semiconductors play an indispensable role as enablers for automated vehicles. Fully automated driving has been identified as one major enabler to master the Grand Societal Challenges of safe, clean, and efficient mobility. For this, fail-operational behavior is essential in the sense, plan, and act stages of the automation chain in order to handle safety-critical situations by its own, which currently is not reached with state-of-the-art approaches also due to missing reliable environment perception and sensor fusion.
PRYSTINE will realize Fail-operational Urban Surround perceptION (FUSION) which is based on robust Radar and LiDAR sensor fusion and control functions in order to enable safe automated driving in urban and rural environments.
Within PRYSTINE, TUD will focus on the design of programmable compute hardware to enable automatic driving functions, across two application targets: data fusion for robust perception; and acceleration of AI frameworks for decision making. TUD will investigate the applicability of neuromorphic computing architectures, and programmable hardware fabrics, within PRYSTINE's envelope of objectives: robust perception and dependable embedded control at reduced cost and power-consumption. These efforts will span the flow from architecture exploration, down to system-level design and prototyping, as well as physical design.
TUD will carry out the architectural exploration of a hardware compute solution for multi-sensor data fusion. Input data to this hardware can be a set of correlated data frames (images, numerical data,...), and output data is a consolidated, fused data stream that can be used as the basis for decision-making/scenario assessment. The objective of this task is to realize a programmable system that can robustly fuse arbitrary data streams, together with a methodology to customize the solution to multiple design points. Further, TUD will investigate hardware architectures to accelerate AI kernels and decision-making frameworks, in the context of automatic driving functions. This activity could include hardware neural networks realized either as a full-custom/semi-custom VLSI implementation, or within a programmable fabric. Finally, to facilitate efficient and robust multi-sensor data fusion across disparate sources, TUD will investigate the hardware acceleration of communication stacks within the programmable system. Alongside this, TUD will investigate the reliability and dependability of the E/E architectures designed for sensor fusion and decision making. Key topics include aging, device degradation, as well as fault tolerance and mitigation techniques. The hardware platforms developed will be combined with algorithms and application software from PRYSTINE partners for performance validation and demonstration within a lab environment.
For more information, see the
project homepage.
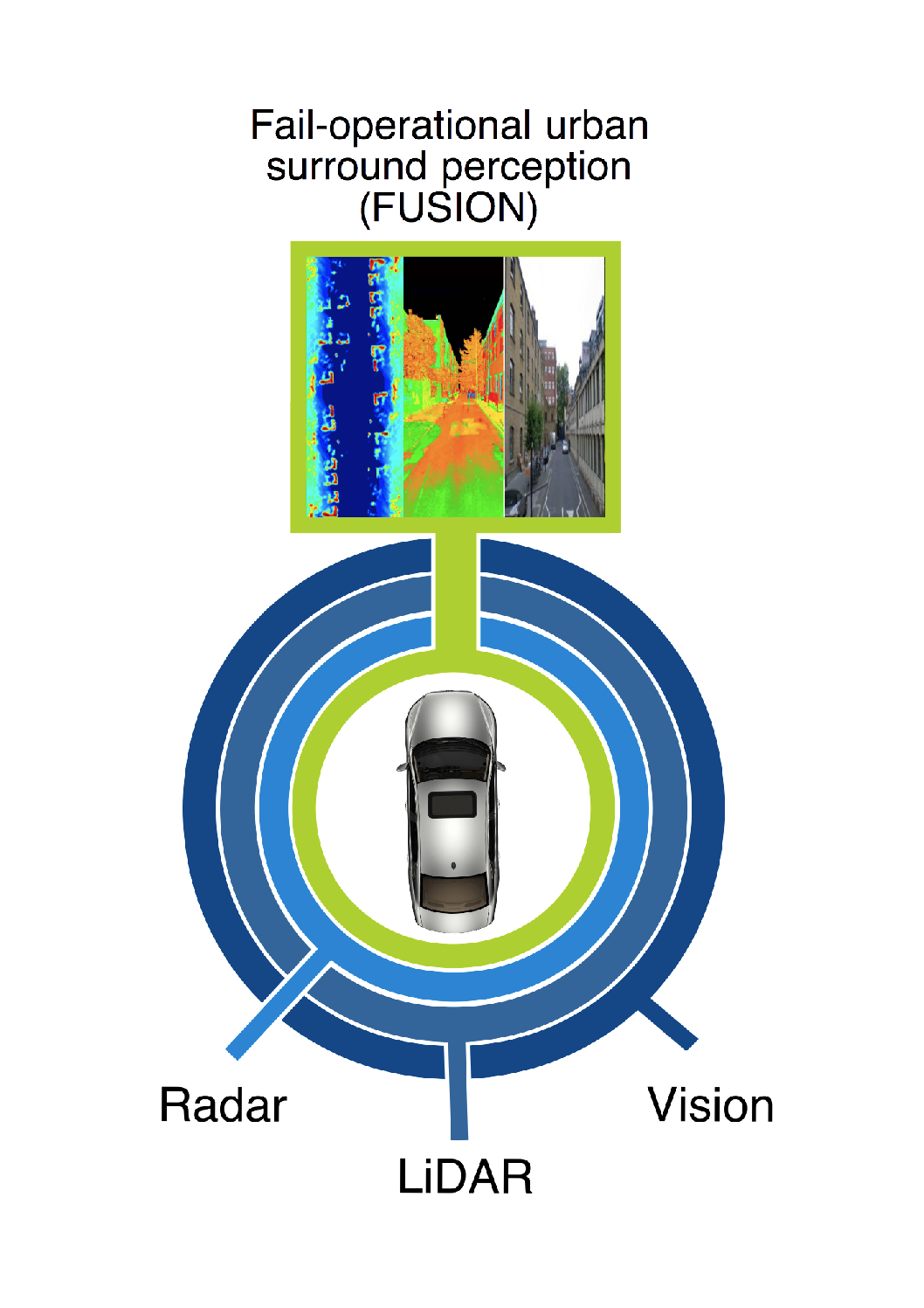
Project data
Researchers: | René van Leuken, Sumeet Kumar, Amir Zjajo, Alexander de Graaf, Johan Mes, Darek Maksimiuk |
---|---|
Starting date: | May 2018 |
Closing date: | November 2021 |
Funding: | 51238 kE; related to group 1747 kE |
Sponsor: | ECSEL/RVO/TKI/ |
Partners: | 60 partners across Europe |
Contact: | René van Leuken |