Agenda
Signal Processing Seminar
- Thursday, 29 February 2024
- 11:00-12:00
- HB 17.140
Cauchy-Schwarz Divergence Information Bottleneck for Regression
Shujian YuDepartment of Artificial Intelligence, VU, Amsterdam
The information bottleneck (IB) approach is popular to improve the generalization and robustness of deep neural networks. Essentially, it aims to find a minimum sufficient representation t from input variable x that is relevant for predicting desirable response variable y, by striking a trade-off between I(x;t) and I(y;t), where I refers to the mutual information (MI). However, optimizing IB remains a difficult problem. In this talk, we study the IB principle for the regression problem and develop a new way to parameterize IB with deep neural networks, by leveraging the favorable properties of the Cauchy-Schwarz (CS) divergence. By doing so, we move away from the mean squared error (MSE) loss-based regression and ease estimation of MI terms by avoiding variational approximations or distributional assumptions. We investigate the improved generalization ability of our proposed CS-IB and demonstrate strong adversarial robustness guarantee. We observe that the solutions discovered by CS-IB always achieve the best trade-off between prediction accuracy and compression ratio. We additionally extend CS-IB to structured data such as graphs, and demonstrate its effectiveness to predict the age of patients based on their brain functional MRI (fMRI) data with a graph neural network.
Additional information ...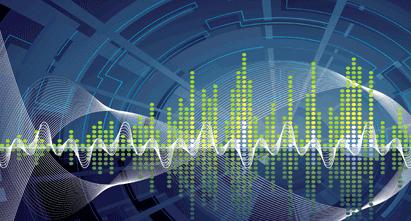
Agenda
- Wed, 30 Jul 2025
- 10:00
- HB17.140 (Seminar room)
MSc SPS Thesis presentation
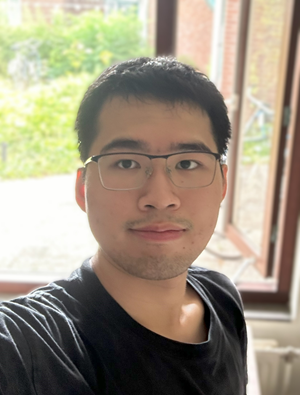
Xingyuan Ma
Wideband Ambiguity Function Shaping and Sonar Imaging
- Thu, 18 Sep 2025
- 09:03
- Aula Senaatszaal
PhD Thesis Defence
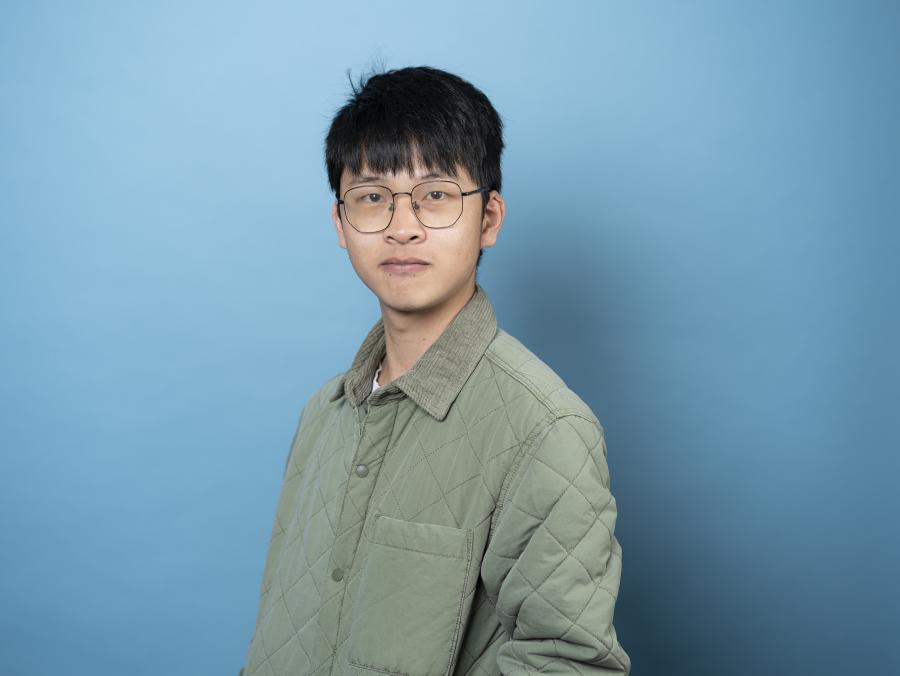
Changheng Li
Multi-Microphone Signal Parameter Estimation in Various Acoustic Scenarios
Low Complexity Approaches Utilizing Temporal Information